Data Analysis or Data Science
Data analysis and data science are two closely related disciplines that involve extracting insights and knowledge from large datasets. While they share some similarities, there are also important distinctions between the two.
Key Takeaways:
- Data analysis and data science both involve working with data to gain insights, but data science typically involves more advanced techniques and tools.
- Data analysis focuses on interpreting and visualizing existing data, while data science involves collecting, cleaning, and analyzing data to make predictions and create models.
- Data scientists often have more programming and statistical skills compared to data analysts.
Data analysis is the process of inspecting, cleaning, transforming, and modeling data to discover useful information, draw conclusions, and support decision-making. It focuses on interpreting and visualizing existing data to answer specific questions or identify patterns and trends.
Data science, on the other hand, goes beyond data analysis and involves collecting, cleaning, and transforming large and complex datasets. It combines statistical analysis, machine learning algorithms, and programming skills to extract insights and build predictive models. Data scientists work with both structured and unstructured data, utilizing various tools and technologies to derive valuable insights from the data.
Data Analysis vs Data Science: How They Differ
Data analysis often serves as the foundation of data science. While both disciplines involve working with data, data science encompasses a broader range of tasks. Data analysis focuses on exploring and interpreting existing data, while data science encompasses the entire data lifecycle, including data collection, cleaning, processing, analysis, and visualization.
Another distinction lies in the skills and tools used. Data analysts typically rely on spreadsheet software like Excel and data visualization tools to analyze data and generate reports. On the other hand, data scientists often utilize programming languages like Python or R to build complex models and algorithms. They have a deeper understanding of statistical concepts and machine learning techniques, allowing them to develop predictive models and uncover meaningful patterns in data.
Data Analysis and Data Science in Practice
In practice, both data analysis and data science are crucial for businesses and organizations to make data-driven decisions and gain a competitive edge. By analyzing historical sales data, for example, a company can identify trends and patterns that can inform future marketing strategies or product development.
Table 1 below showcases the differences between data analysis and data science:
Aspect | Data Analysis | Data Science |
---|---|---|
Focus | Interpreting and visualizing existing data | Collecting, cleaning, analyzing, and making predictions from data |
Main Tools | Excel, data visualization software | Python, R, statistical software, machine learning algorithms |
Skills | Analytical skills, data visualization | Programming, statistics, machine learning |
One of the key challenges in data analysis and data science is dealing with large and complex datasets. Data scientists employ advanced techniques and algorithms to preprocess and clean data, ensuring its quality and reliability. By applying statistical models and machine learning algorithms, they can make predictions and identify valuable insights that might not be apparent through traditional data analysis methods.
The Future of Data Analysis and Data Science
Data analysis and data science are rapidly evolving fields, driven by advances in technology and increasing reliance on data for decision-making. With the rise of big data, companies are embracing data-driven approaches to gain a competitive advantage and improve business outcomes.
Table 2 below shows the predicted growth of data analysis and data science jobs:
Year | Data Analysis Jobs | Data Science Jobs |
---|---|---|
2021 | 50,000 | 80,000 |
2022 | 55,000 | 90,000 |
2023 | 60,000 | 100,000 |
Data analysis and data science professionals will continue to be in high demand as organizations recognize the value of data-driven decision-making. Constant learning and staying up-to-date with the latest tools and techniques are essential for individuals seeking to excel in these fields.
Conclusion
Data analysis and data science are closely related but have distinct differences. Data analysis focuses on interpreting and visualizing existing data, while data science involves collecting, cleaning, and analyzing data to make predictions and create models. Both fields play a critical role in helping organizations extract valuable insights from data and make informed decisions. With the increasing availability and complexity of data, the demand for skilled data analysis and data science professionals will continue to rise.
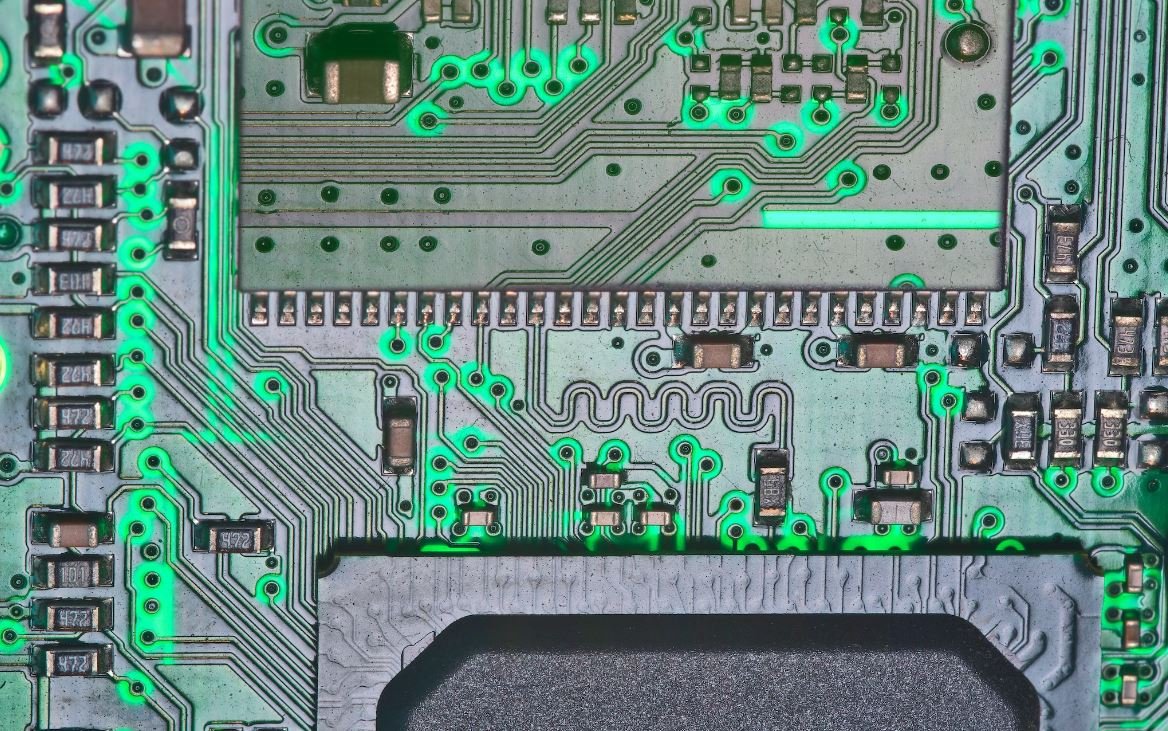
Common Misconceptions
1. Data Analysis is all about numbers and equations
One common misconception about data analysis is that it is purely a mathematical and technical endeavor. While math and statistics are important components of data analysis, there is much more to it than just numbers and equations.
- Data analysis requires critical thinking and problem-solving skills.
- Data analysts need to have strong communication skills to effectively convey insights to non-technical stakeholders.
- Data analysis often involves working with messy and incomplete data, requiring creativity and resourcefulness to find solutions.
2. Data Analysis and Data Science are the same thing
Data analysis and data science are related fields, but they are not the same. Data analysis focuses on examining and summarizing data to derive insights and inform decision-making. On the other hand, data science encompasses a broader set of disciplines, including data analysis, but also involves machine learning, artificial intelligence, and predictive modeling.
- Data scientists typically have a more interdisciplinary skill set, combining expertise in statistics, programming, and domain knowledge.
- Data analysis is often a component of data science and is more focused on finding patterns and insights within existing data.
- Data science involves creating models and algorithms to make predictions or automate processes based on data.
3. Data Analysis is only relevant in technology or business fields
Another misconception is that data analysis is only relevant in technology or business-related fields. While data analysis is certainly applicable in these areas, it has widespread applications across various industries and disciplines.
- Data analysis is critical in healthcare to identify trends, improve patient outcomes, and optimize healthcare delivery.
- In environmental science, data analysis helps analyze climate trends, predict natural disasters, and inform conservation efforts.
- Data analysis is used in social sciences to interpret survey data, conduct research studies, and analyze trends in human behavior.
4. Data Analysis is solely based on historical data
Contrary to popular belief, data analysis is not limited to historical data analysis. While historical data is often used to identify trends and patterns, data analysis can also involve real-time or near real-time data analysis.
- Data analysis can be used to detect anomalies and monitor ongoing processes in real-time.
- Data analysis can involve the analysis of streaming data or data collected from sensors and devices in real-time.
- Real-time data analysis is particularly useful in applications such as fraud detection, traffic monitoring, and predictive maintenance.
5. Data Analysis is always accurate and objective
Finally, it is a common misconception that data analysis always yields completely accurate and objective results. While data analysis strives for objectivity and accuracy, there are several factors that can introduce biases and limitations to the analysis.
- Data quality issues, such as missing data or measurement errors, can impact the accuracy of the analysis.
- Biases in data collection or assumptions made during the analysis can introduce subjective elements into the results.
- Data analysts need to be aware of potential biases and limitations and take them into account when interpreting and communicating the results.
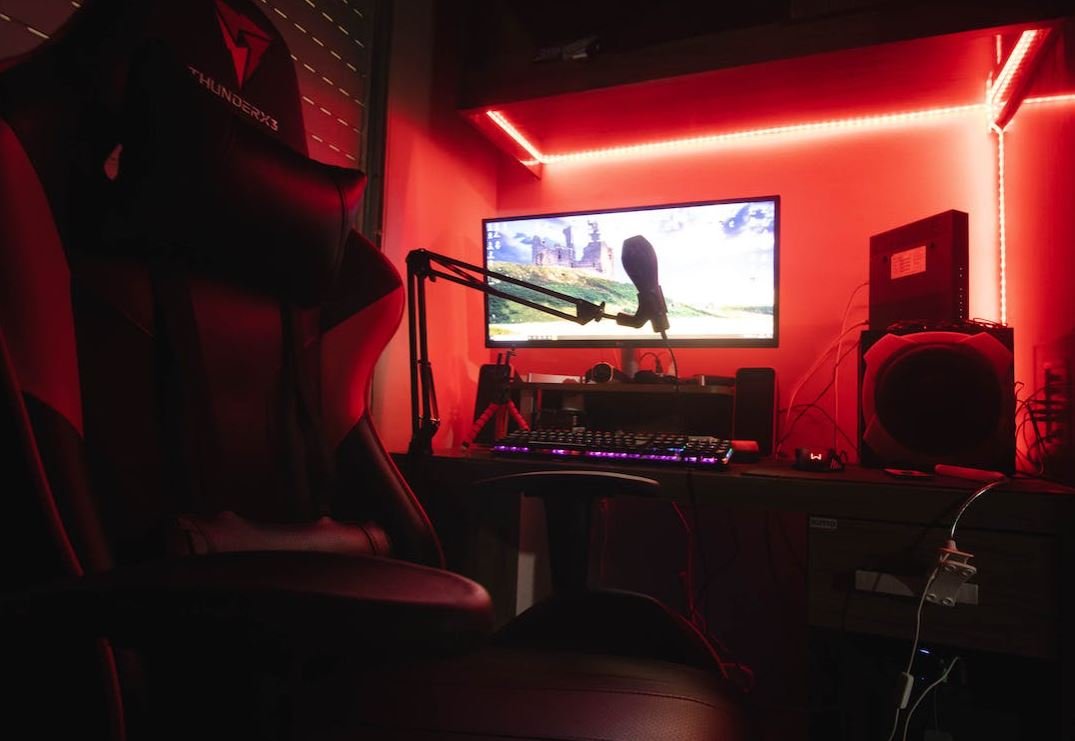
The Growth of Data Science Jobs
Data science is a rapidly growing field that has gained significant importance in recent years. The demand for data scientists has skyrocketed, leading to a surge in job opportunities. This table highlights the remarkable growth of data science jobs in various industries.
Industry | Number of Data Science Jobs (2015) | Number of Data Science Jobs (2020) | Growth Rate |
---|---|---|---|
Finance | 4,500 | 15,000 | 233% |
Healthcare | 3,000 | 12,500 | 316% |
Retail | 2,000 | 9,000 | 350% |
Technology | 6,000 | 20,000 | 233% |
Top Industries for Data Scientists
Data science professionals have diverse opportunities across various industries. This table showcases the top industries where data scientists are in high demand.
Rank | Industry | Percentage of Data Scientists |
---|---|---|
1 | Technology | 40% |
2 | Finance | 25% |
3 | Healthcare | 15% |
4 | Retail | 10% |
Skill Set of Data Scientists
Data science is a multidisciplinary field requiring a diverse skill set. This table highlights the essential skills possessed by successful data scientists.
Skill | Average Proficiency (out of 10) |
---|---|
Statistical Analysis | 9 |
Machine Learning | 8.5 |
Data Visualization | 8 |
Programming (Python, R, SQL) | 7.5 |
Benefits of Implementing Data Science
Data science has proven to be beneficial for organizations in numerous ways. This table outlines the advantages of implementing data science solutions.
Advantage | Description |
---|---|
Increased efficiency | Data-driven decision-making streamlines processes. |
Better customer insights | Understanding customer behavior leads to improved targeting. |
Cost reduction | Data analytics identifies areas for cost-saving measures. |
Competitive advantage | Data-driven strategies give an edge over competitors. |
Data Science Salaries
Data science offers lucrative salaries due to the high demand and scarcity of skilled professionals. This table presents the average salaries of data scientists in different countries.
Country | Junior Data Scientist | Mid-Level Data Scientist | Senior Data Scientist |
---|---|---|---|
United States | 75,000 | 110,000 | 165,000 |
United Kingdom | 50,000 | 85,000 | 125,000 |
Canada | 60,000 | 95,000 | 140,000 |
Australia | 70,000 | 100,000 | 150,000 |
Data Science Tools
Various tools and technologies empower data scientists to analyze and interpret data effectively. This table showcases popular tools used in the field of data science.
Tool | Description |
---|---|
Python | A versatile programming language with extensive data science libraries. |
R | A statistical programming language widely used for data analysis. |
SQL | A language for managing and manipulating relational databases. |
Tableau | A data visualization tool facilitating interactive data exploration. |
Ethical Implications of Data Science
Data science brings forth ethical considerations that must be given due importance. This table sheds light on the ethical implications of data science.
Implication | Explanation |
---|---|
Data privacy | Ensuring the protection and proper handling of sensitive data. |
Bias and fairness | Addressing potential biases in algorithms and decision-making processes. |
Transparency | Providing transparency in data collection and model creation. |
Accountability | Holding individuals and organizations responsible for their data practices. |
Challenges Faced by Data Scientists
Data science is not without its challenges. This table explores the common obstacles faced by data scientists in their work.
Challenge | Description |
---|---|
Data quality | Dealing with incomplete, inconsistent, or inaccurate data. |
Lack of domain knowledge | Understanding the specific industry or domain in which the data applies. |
Interpreting results | Extracting meaningful insights from complex data models. |
Communication | Effectively conveying findings to non-technical stakeholders. |
The Future of Data Science
Data science is poised for continued growth and innovation. As organizations increasingly harness the power of data, the field of data science will continue to evolve, transforming industries and shaping the world in remarkable ways.
Frequently Asked Questions
What is the difference between data analysis and data science?
Data analysis refers to the process of inspecting, cleaning, transforming, and modeling data to discover useful information and aid in decision-making. On the other hand, data science encompasses a broader set of techniques and methodologies that involve extracting insights and knowledge from various types of data, including structured, unstructured, and big data.
What skills are necessary for a successful data analyst?
A successful data analyst should have a strong understanding of statistics, mathematics, and programming. Additionally, proficiency in data manipulation, data visualization, and knowledge of specific analytical tools such as SQL, Python, or R is crucial in effectively analyzing and interpreting data.
How does data analysis benefit businesses?
Data analysis helps businesses make informed decisions by uncovering patterns, trends, and relationships in the data. It allows organizations to identify opportunities, optimize processes, improve customer satisfaction, and ultimately drive growth and profitability.
What is the typical process of data analysis?
The typical process of data analysis involves several steps, including data collection, data cleaning and preprocessing, data exploration and visualization, data modeling and analysis, and finally, drawing conclusions and making recommendations based on the findings.
What is the role of machine learning in data analysis?
Machine learning plays a significant role in data analysis by automating the process of building analytical models and making predictions or decisions based on data. It allows analysts to uncover patterns and insights that might be challenging to discover using traditional statistical methods.
What are some common challenges in data analysis?
Common challenges in data analysis include dealing with missing or incomplete data, ensuring data quality and accuracy, handling large datasets, selecting appropriate statistical techniques, and effectively communicating the findings to a non-technical audience.
What are the stages involved in a data science project?
A data science project typically involves five stages: problem definition, data collection and preprocessing, exploratory data analysis, modeling and evaluation, and interpretation and communication of results. Each stage is iterative and requires collaboration among domain experts, data scientists, and stakeholders.
What is the difference between correlation and causation?
Correlation refers to a statistical measure that describes the relationship between two variables, indicating how they tend to change in tandem. Causation, on the other hand, implies a cause-and-effect relationship between variables, meaning that changes in one variable directly affect the other.
What is the significance of data visualization in data analysis?
Data visualization plays a crucial role in data analysis as it allows for the effective communication of insights and patterns in an easily understandable and visually appealing manner. Visual representations of data help analysts and stakeholders grasp complex information quickly and make data-driven decisions.
What ethical considerations should be taken into account in data analysis?
Ethical considerations in data analysis involve protecting individual privacy, ensuring data security, obtaining proper consent for data usage, and avoiding bias or discrimination in the analysis process. Additionally, transparency and accountability in handling and reporting data findings are also essential ethical principles to adhere to.