Machine Learning History Timeline
Machine learning is an evolving field that has seen significant advancements since its inception. This article provides a comprehensive timeline of the key milestones in the history of machine learning, showcasing its progress and impact on various industries.
Key Takeaways
- Machine learning has a rich history of development and innovation.
- Significant advancements have been made in machine learning algorithms and techniques.
- Machine learning has revolutionized industries such as healthcare, finance, and transportation.
- Data availability and computing power have greatly contributed to the growth of machine learning.
The Early Beginnings: 1940s – 1950s
In the late 1940s and early 1950s, the foundations of machine learning were laid down. **Pioneering researchers** like Alan Turing and Arthur Samuel developed early computer programs capable of learning from data. Samuel’s checkers-playing program, which improved its performance through experience, was a prime example of early machine learning. *These early efforts set the stage for future developments in the field.*
Advancements in Neural Networks: 1950s – 1970s
The 1950s witnessed the development of **perceptron**, a single-layer artificial neural network capable of learning simple tasks. This breakthrough laid the groundwork for further advancements in neural network research. *The perceptron algorithm became the foundation for future complex neural network architectures.* However, by the late 1960s, the limitations and computational challenges posed by neural networks led to a decline in interest in this field.
Renewed Interest: 1980s – 1990s
The 1980s and 1990s saw a resurgence of interest in machine learning. Researchers focused on developing **new algorithms** and techniques, such as decision tree learning and support vector machines, to improve the accuracy and performance of machine learning models. *This period marked a turning point for machine learning, with increased applications in areas like image recognition and natural language processing.*
Year | Milestone |
---|---|
1943 | Warren McCulloch and Walter Pitts propose the first computational model of a neural network. |
1956 | John McCarthy coins the term “artificial intelligence” and organizes the Dartmouth Conference, where machine learning is discussed. |
1986 | Geoffrey Hinton introduces the backpropagation algorithm, enabling training of deep neural networks. |
Big Data and Deep Learning Revolution: 2000s – Present
The 2000s marked a significant shift in machine learning, driven by the availability of **big data** and the advancement of **computing power**. This era witnessed the rise of **deep learning**, a subfield of machine learning focused on large neural networks with multiple layers. Deep learning models, designed to mimic the human brain, achieved remarkable success in tasks such as image classification and speech recognition. *The ability to extract valuable insights from massive datasets has transformed countless industries.*
Industry | Applications |
---|---|
Healthcare | Personalized medicine, disease detection, drug discovery. |
Finance | Stock market analysis, fraud detection, risk assessment. |
Transportation | Autonomous vehicles, route optimization, traffic prediction. |
Continuing Advancements and Ethical Considerations
Machine learning continues to advance at a rapid pace. **Researchers and practitioners** are exploring state-of-the-art algorithms, such as reinforcement learning and generative adversarial networks, to tackle more complex problems. *These innovations offer exciting possibilities, but also raise ethical concerns related to privacy, bias, and algorithmic transparency.* It is crucial to navigate these challenges as machine learning becomes more integrated into various aspects of our lives.
Year | Development |
---|---|
2012 | Geoffrey Hinton’s team at Google achieves breakthrough results in image recognition using deep convolutional neural networks. |
2016 | AlphaGo, a computer program developed by DeepMind, defeats the world champion Go player, marking a major milestone in artificial intelligence. |
2019 | OpenAI’s GPT-2 language model demonstrates remarkably human-like text generation capabilities, raising concerns about the potential misuse of such technology. |
As technological advancements in machine learning **continue to shape our future**, it is important to stay abreast of the latest developments. The history of machine learning highlights the exponential growth and immense potential of this field, which will undoubtedly have a transformative impact on society.
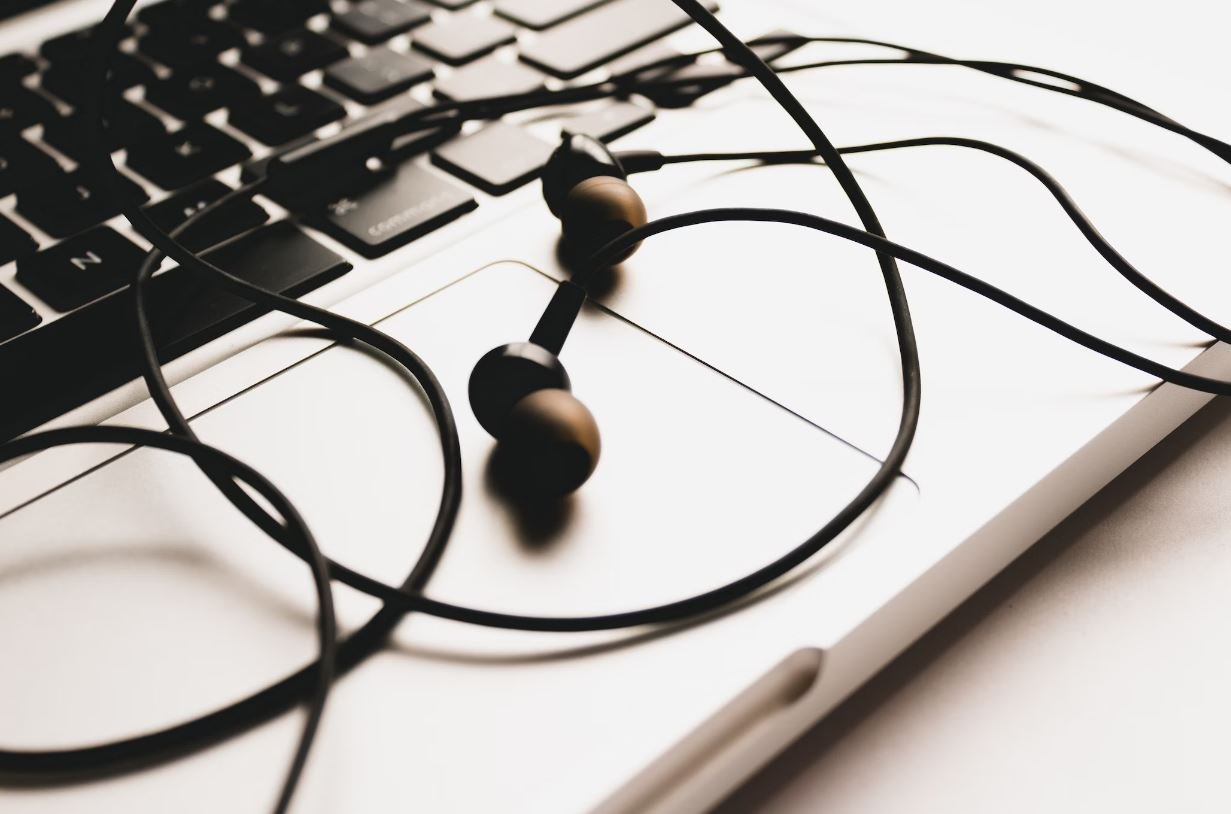
Common Misconceptions
Machine Learning History Timeline
There are several common misconceptions around the topic of machine learning history. One of the most prevalent misconceptions is that machine learning is a relatively new field that emerged only in the last decade. Another misconception is that machine learning was exclusively developed by computer scientists and engineers. Lastly, some people may believe that machine learning has not significantly impacted our daily lives. Let’s debunk these misconceptions one by one.
– Machine learning is not a new concept, but rather has its roots in the mid-20th century.
– Machine learning involves a wide range of disciplines beyond computer science, including mathematics, statistics, and neuroscience.
– Machine learning plays a significant role in various aspects of our lives, from recommendation systems to voice assistants.
Contrary to popular belief, machine learning is not a recent phenomenon. While the field has seen explosive growth in recent years, the foundations of machine learning can be traced back to the mid-1900s. Pioneers like Arthur Samuel, who developed the first self-learning program in 1952, laid the groundwork for the field we know today. Additionally, the concept of neural networks, a fundamental aspect of modern machine learning algorithms, was first proposed in the 1940s by Warren McCulloch and Walter Pitts.
– The roots of machine learning date back to the mid-20th century.
– Arthur Samuel created the first self-learning program in 1952.
– Neural networks were first proposed in the 1940s by Warren McCulloch and Walter Pitts.
Machine learning is not solely an effort of computer scientists and engineers. While these professionals have played a crucial role in advancing the field, machine learning draws from a variety of disciplines. Mathematics and statistics provide the foundation for many machine learning algorithms and models, helping to ensure accurate predictions and analyses. Additionally, insights from neuroscience have influenced the development of machine learning algorithms inspired by the workings of the human brain. This interdisciplinary nature allows for collaboration and the integration of diverse perspectives into machine learning research and applications.
– Machine learning draws from disciplines like mathematics, statistics, and neuroscience.
– Mathematics and statistics provide the foundation for machine learning algorithms.
– Insights from neuroscience have influenced the development of machine learning algorithms.
Lastly, it is incorrect to say that machine learning has not had a significant impact on our daily lives. From our interactions with search engines and social media platforms to personalized product recommendations, machine learning algorithms underpin many technologies we rely on each day. Additionally, machine learning has revolutionized industries such as healthcare, finance, and transportation, improving diagnostics, fraud detection, and autonomous vehicle capabilities. The increasing integration of machine learning in society only further highlights its profound impact on our daily lives and the potential it holds for the future.
– Machine learning impacts our daily lives through technologies like search engines and product recommendations.
– Machine learning revolutionizes industries such as healthcare, finance, and transportation.
– The integration of machine learning in society highlights its profound impact and future potential.
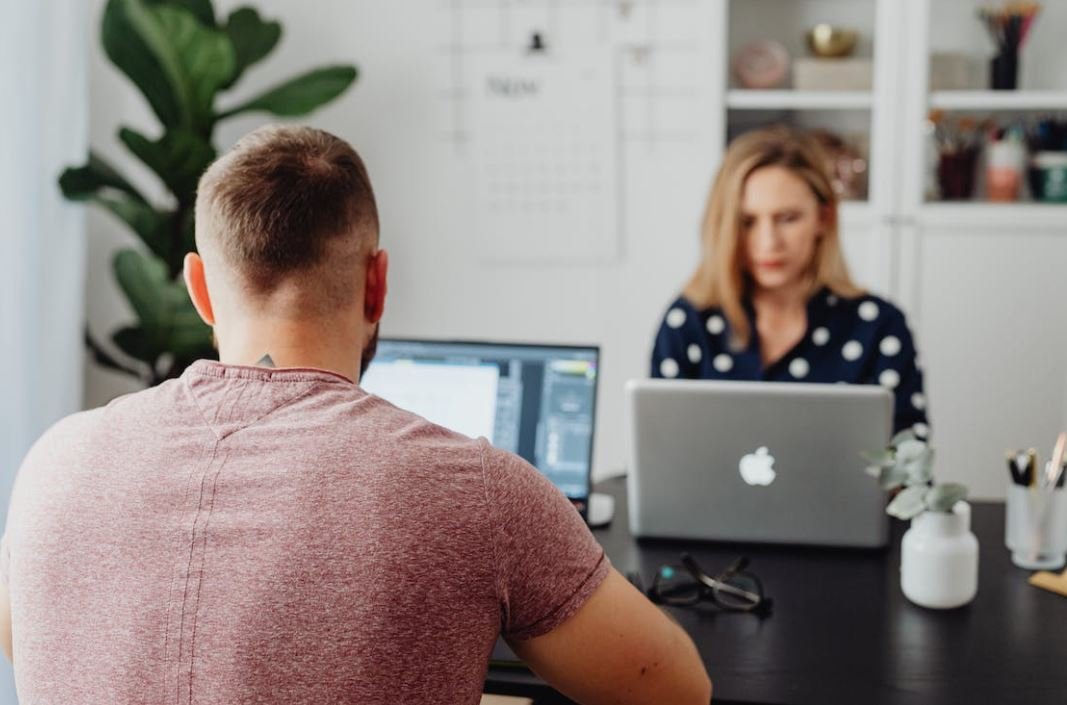
The Early Beginnings
In the early days of artificial intelligence and machine learning, significant breakthroughs and milestones were achieved. The following table presents some key events during this period:
Year | Event |
---|---|
1950 | Alan Turing proposes the “Turing Test” as a measure of machine intelligence. |
1956 | John McCarthy organizes the Dartmouth Conference, officially heralding the field of AI. |
1957 | Frank Rosenblatt invents the “Perceptron,” a single-layer neural network. |
1959 | Arthur Samuel develops the first machine learning program to play checkers. |
1967 | The “Nearest Neighbor Algorithm” is introduced, a landmark in pattern recognition. |
The AI Winter
Following the initial enthusiasm, a period known as the “AI Winter” ensued, where progress and funding were reduced. However, several notable events still occurred:
Year | Event |
---|---|
1970 | The “Neural Network” concept experiences renewed interest. |
1979 | Stanford’s “Knowledge-Based Systems” program demonstrates advanced AI applications. |
1986 | Geoffrey Hinton publishes the “Backpropagation” algorithm, revolutionizing neural network learning. |
1997 | IBM’s “Deep Blue” defeats Garry Kasparov in a chess match, showcasing the power of machine learning. |
1998 | Yann LeCun develops the “LeNet-5” convolutional neural network, advancing computer vision. |
The Renaissance of Machine Learning
In recent years, machine learning has experienced a renaissance, leading to tremendous advancements. These breakthroughs have reshaped various fields, as exemplified by the following table:
Year | Event |
---|---|
2006 | Geoffrey Hinton presents the first successful “Deep Belief Network,” fueling the rise of deep learning. |
2011 | IBM’s “Watson” wins Jeopardy! against human champions, showcasing natural language processing and reasoning. |
2012 | AlexNet, a deep convolutional neural network, achieves a significant leap in image classification performance. |
2014 | Google’s “DeepMind” develops a neural network that learns to play video games, exhibiting reinforcement learning capabilities. |
2018 | GANs (Generative Adversarial Networks) are introduced, enabling the generation of realistic synthetic data. |
The Future of Machine Learning
As machine learning continues to evolve, researchers and experts foresee several exciting possibilities and challenges. The following table presents some potential future implications:
Year | Implication |
---|---|
2022 | Autonomous vehicles become a common sight on roads, revolutionizing transportation. |
2025 | AI-powered virtual personal assistants become nearly indistinguishable from human interactions. |
2030 | Robotic companions for the elderly enhance quality of life and provide emotional support. |
2035 | Machine learning enables significant advancements in medical diagnostics, revolutionizing healthcare. |
2040 | General Artificial Intelligence (AGI) surpasses human capabilities, leading to unprecedented breakthroughs. |
The Impact on Industries
Machine learning has had a transformative effect on diverse industries, introducing new possibilities and efficiencies. The table below highlights some sectors significantly impacted:
Industry | Impact of Machine Learning |
---|---|
Healthcare | Enhances disease diagnosis, drug development, and personalized treatment plans. |
E-commerce | Enables personalized recommendations, targeted advertising, and demand forecasting. |
Finance | Improves fraud detection, stock market prediction, and algorithmic trading. |
Transportation | Optimizes route planning, autonomous vehicle navigation, and traffic management. |
Manufacturing | Enhances quality control, predictive maintenance, and optimizing production processes. |
The Ethical Considerations
The advancements in machine learning also raise ethical considerations surrounding data privacy, bias, and transparency. The table below sheds light on these concerns:
Concern | Implication |
---|---|
Data Privacy | Increased data collection raises concerns about the security and use of personal information. |
Bias in Algorithms | Machine learning models can reflect biases present in training data, leading to unfair or discriminatory outcomes. |
Transparency | The complexity of some machine learning algorithms makes it challenging to explain how decisions are reached, raising transparency concerns. |
Human Replacement | The rise of automation and AI-driven systems may lead to job displacement and socioeconomic implications. |
Accountability | Clarifying responsibility for AI-driven decisions and establishing appropriate legal frameworks becomes vital. |
The Contributions of Notable Figures
Throughout history, numerous influential figures have contributed to the advancement of machine learning. The table below highlights some key individuals:
Name | Contribution |
---|---|
Alan Turing | Developed the concept of the “Turing Test” and laid the foundation for modern computer science. |
John McCarthy | Pioneered the field of AI and organized the Dartmouth Conference. |
Geoffrey Hinton | Revolutionized neural network learning with the “Backpropagation” algorithm and significantly advanced deep learning. |
Yann LeCun | Developed the “LeNet-5” convolutional neural network, essential for computer vision advancement. |
Fei-Fei Li | Advocated for large-scale datasets and contributed to the expansion of deep learning in computer vision. |
Machine learning‘s historical timeline showcases not only the progression of the field but also the transformative impact it has had on various aspects of our lives. These advancements hold great promise for the future, but also require careful consideration of ethical implications and responsible implementation.
Machine Learning History Timeline
Frequently Asked Questions
What is machine learning?
When was machine learning first introduced?
What are the key milestones in machine learning history?
Who are some influential figures in the history of machine learning?
What are some real-world applications of machine learning?
What are the different types of machine learning algorithms?
What are some challenges in machine learning?
How is machine learning related to artificial intelligence?
What is the future of machine learning?
How can I start learning machine learning?