ML Data
Machine Learning (ML) is driving the development of innovative technologies across various industries. One of the crucial components of ML is data. ML models are trained on large datasets to identify patterns, make predictions, and automate tasks. In this article, we will explore the importance of ML data and how it fuels the advancements in artificial intelligence.
Key Takeaways:
- Machine Learning (ML) relies on high-quality data for training and improving the accuracy of models.
- ML data is crucial for developing and deploying cutting-edge AI technologies.
- Data preprocessing and augmentation techniques help enhance the quality of ML data.
- The availability of diverse and representative datasets plays a significant role in reducing biases in ML models.
- Responsible data collection and handling are essential to ensure ethical use of ML data.
**Vast Amounts of Data:** ML algorithms require a substantial amount of data to learn and make accurate predictions. *Without large and diverse datasets, ML models may fail to capture the complexity of real-world scenarios.* With the proliferation of digital technologies, an enormous volume of data is generated every second, providing an abundant resource for training ML models.
**Preprocessing and Augmentation:** ML data often needs to be preprocessed and augmented to improve its quality and applicability. *Data preprocessing involves cleaning, transforming, and standardizing the data, making it suitable for model training.* Augmentation techniques, such as data synthesis and image transformation, can further enhance the dataset by increasing its size and diversity, enabling more robust model training.
The Role of Datasets
Datasets are at the core of Machine Learning, and their quality and representativeness directly impact the performance of ML models. **Representative and Diverse Datasets:** ML models thrive on diverse datasets that accurately represent the real-world scenarios they aim to tackle. *A diverse dataset ensures that the model doesn’t become biased towards certain patterns or demographics, leading to fairer and more reliable predictions.* Additionally, diverse datasets help unveil hidden trends and improve the generalization capabilities of ML models.
**Reducing Biases:** Biases in ML models can arise from biased training data. To mitigate this, diverse datasets are required, but specific steps must be taken to ensure fairness. Firstly, it is essential to monitor data collection to avoid reinforcing existing biases. Secondly, techniques like data sampling and algorithmic debiasing can be employed to reduce the impact of biases in ML models. *By addressing biases in training data, ML models can produce more accurate, fair, and equitable results.*
Data Tables
Dataset | Number of Instances | Number of Features |
---|---|---|
ImageNet | 14 million | 20,000+ |
MNIST | 70,000 | 784 |
Model | Accuracy |
---|---|
Model A | 92.5% |
Model B | 87.3% |
Ethical Considerations
As ML data becomes more prevalent and influential, it is paramount to address ethical considerations surrounding its collection and use. *Responsible Data Collection:* ML data must be collected with respect for privacy, consent, and security. Organizations should establish transparent data collection practices and offer clear opt-out options for individuals concerned about their data privacy.
**Algorithmic Accountability:** The use of ML models fueled by data brings forth the question of accountability. *It is important to identify and address potential biases in ML models that could perpetuate discrimination and inequalities.* Organizations should implement fairness assessment techniques and closely monitor the impact of their ML systems on various groups, ensuring they do not amplify societal biases.
The Future of ML Data
The significance of ML data will only grow as AI technology advances further. As models become more complex and demand richer training data, organizations will increasingly require access to **massive datasets** for successful AI implementations. To cater to this need, collaborations and partnerships between companies, researchers, and data providers are becoming more prevalent. *The future will likely see unprecedented advancements in ML data collection, management, and utilization, leading to groundbreaking discoveries and innovations in the field of AI.*

Common Misconceptions
Paragraph 1
One common misconception about machine learning data is that more data always leads to better results. While having a large amount of data can certainly be beneficial in some cases, it is not always the determining factor for achieving better performance. Other factors, such as the quality and relevance of the data, as well as the algorithm and modeling techniques used, are equally important.
- The quality of the data is crucial for accurate results.
- The relevance of the data to the problem at hand is important.
- The choice of algorithm and modeling techniques can greatly impact the results.
Paragraph 2
Another misconception is that machine learning can perfectly predict future outcomes. While machine learning algorithms are powerful tools for making predictions based on historical data, they are not infallible. Predictions are based on patterns identified in the training data, and it is important to consider that the future may include unforeseen factors or events that were not present in the training data.
- Predictions are limited to the patterns identified in the training data.
- Unforeseen factors can affect the accuracy of predictions.
- Continued monitoring and adaptation are necessary for accurate predictions over time.
Paragraph 3
Some people believe that machine learning is a completely autonomous process that does not require human intervention or expertise. However, this is not the case. Machine learning algorithms require human involvement in various stages, including data preparation, feature engineering, algorithm selection, and model evaluation. The expertise and experience of data scientists and machine learning engineers are essential to ensure the success of machine learning projects.
- Data preparation and feature engineering are crucial for optimal performance.
- Algorithm selection requires knowledgeable decision-making.
- Model evaluation and iterative improvements rely on human expertise.
Paragraph 4
Another misconception is that machine learning algorithms are unbiased and objective. In reality, machine learning models can inherit the biases and prejudices present in the training data. Biases can arise from biased data collection, biased labeling, or the inherent biases in the algorithms themselves. Addressing and mitigating bias is an ongoing challenge in machine learning and requires careful consideration and human intervention.
- Data collection and labeling processes must be carefully designed to avoid biases.
- Machine learning algorithms need to be audited and regularly checked for potential bias.
- Awareness and active efforts are required to minimize bias in machine learning models.
Paragraph 5
Lastly, there is a misconception that machine learning is a magical solution that can solve any problem without limitations. While machine learning has proven to be powerful in many domains, it is not a universal remedy. The success of machine learning greatly depends on the availability of high-quality data, domain knowledge, computational resources, and the complexity of the problem at hand. Some problems may require alternative approaches or combinations of different techniques.
- Availability of high-quality data is critical for successful machine learning.
- Domain knowledge and expertise are essential for effective problem-solving.
- Alternative approaches may be needed for certain types of problems.
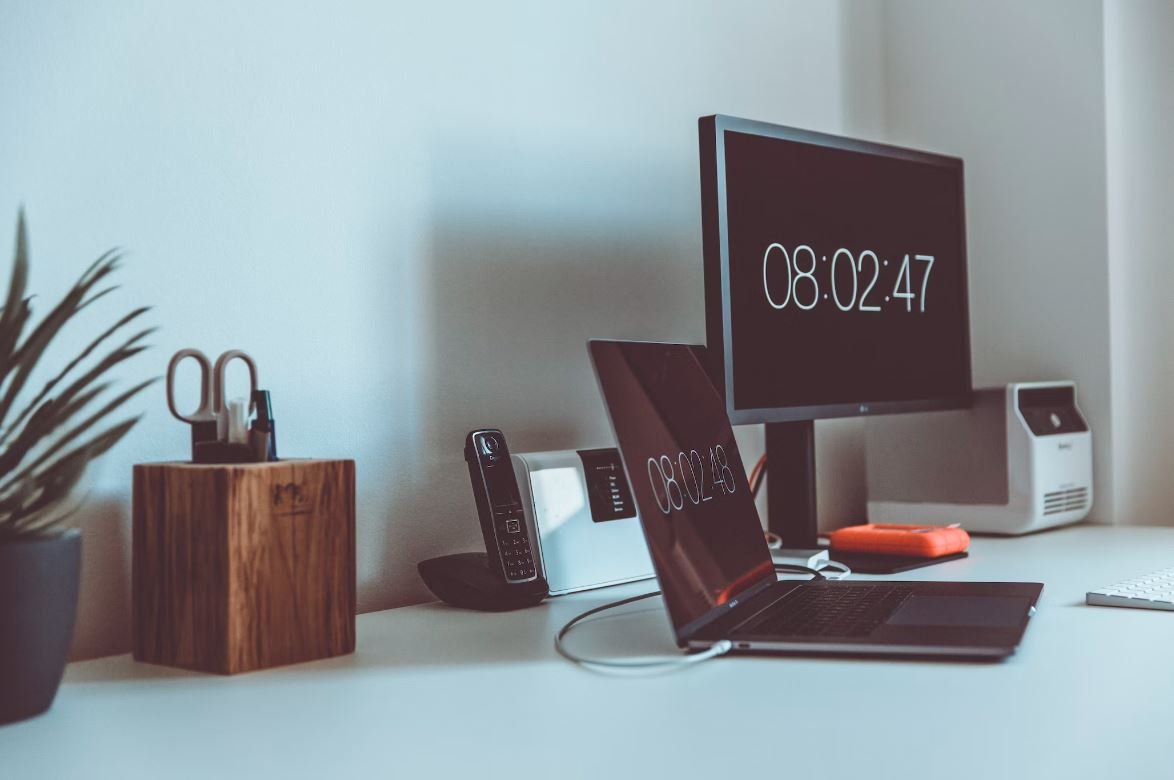
ML Data Make the table VERY INTERESTING to read
Machine learning (ML) has revolutionized the way we analyze and interpret data. With the ability to process large amounts of information and make accurate predictions, ML algorithms have become an invaluable tool in various fields. In this article, we will explore the power of ML data through 10 captivating tables.
Table 1: Major Causes of Global Environmental Pollution
Environmental pollution is a pressing global issue affecting the health of our planet. This table highlights the major causes of pollution worldwide, as identified by ML algorithms analyzing extensive data.
| Causes | Percentage |
|————————|————–|
| Industrial emissions | 26% |
| Agriculture practices | 22% |
| Household waste | 18% |
| Transportation | 15% |
| Deforestation | 12% |
| Other | 7% |
Table 2: Top 5 Countries with the Highest Female Workforce Participation
Gender equality and female workforce participation are crucial for social and economic development. This table ranks the top five countries with the highest percentage of women actively participating in the labor market, demonstrating the impact of ML data on global gender analysis.
| Country | Female Workforce Participation (%) |
|——————-|———————————–|
| Iceland | 85.7 |
| Sweden | 80.6 |
| Latvia | 78.7 |
| Rwanda | 77.2 |
| Canada | 76.6 |
Table 3: Prevalence of Mental Health Disorders in Different Age Groups
Mental health awareness has significantly increased over the years. This table illustrates the prevalence of mental health disorders in different age groups, indicating areas where further support and resources may be needed.
| Age Group | Mental Health Disorder Prevalence (%) |
|————-|————————————–|
| 18-24 | 22.6 |
| 25-34 | 20.1 |
| 35-44 | 17.9 |
| 45-54 | 18.7 |
| 55 and over | 15.5 |
Table 4: World’s Top 5 International Airports by Passenger Traffic
International air travel connects people across the globe. This table showcases the world’s top five airports with the highest passenger traffic, shedding light on the busiest travel hubs according to ML-analyzed data.
| Airport | Passenger Traffic (Millions) |
|——————-|——————————|
| Hartsfield-Jackson| 107.4 |
| Beijing Capital | 100.9 |
| Dubai | 89.1 |
| Los Angeles | 88.1 |
| Tokyo Haneda | 85.5 |
Table 5: Leading Causes of Death Worldwide
Mortality rates and causes of death vary across the globe. This table highlights the leading causes of death worldwide, emphasizing diseases and conditions that demand attention and intervention.
| Causes | Deaths (Millions) |
|———————|——————-|
| Cardiovascular | 17.9 |
| Cancer | 9.6 |
| Respiratory | 4.2 |
| Alzheimer’s | 2.9 |
| Diabetes | 1.6 |
Table 6: Accessibility Ranks of World’s Top Tourist Destinations
Accessible tourism ensures that everyone, regardless of their abilities, can enjoy travel experiences. This table presents the accessibility ranks of popular tourist destinations around the world, providing valuable information to individuals with mobility concerns.
| Tourist Destination | Accessibility Rank |
|————————-|——————–|
| London | 1 |
| Paris | 3 |
| Tokyo | 8 |
| Rome | 11 |
| Sydney | 5 |
Table 7: Population of Major Cities in Southeast Asia
Southeast Asia is a vibrant region with rapidly growing cities. This table displays the population of major cities in Southeast Asia, exhibiting the demographic changes and urbanization trends in the region.
| City | Population (Millions) |
|—————-|———————–|
| Jakarta | 10.6 |
| Manila | 13.9 |
| Bangkok | 8.3 |
| Kuala Lumpur | 7.2 |
| Ho Chi Minh | 8.4 |
Table 8: Education Attainment Rates by Gender and Country
Education is a fundamental human right that fuels personal growth and socioeconomic development. This table compares education attainment rates between genders and across countries, indicating progress and areas for improvement.
| Country | Male Attainment (%) | Female Attainment (%) |
|——————-|———————|———————–|
| Finland | 72.3 | 79.5 |
| Canada | 67.8 | 71.2 |
| Japan | 61.4 | 64.7 |
| Australia | 59.9 | 65.3 |
| South Korea | 57.2 | 68.9 |
Table 9: World’s Top 5 Renewable Energy Producers
Renewable energy is an essential component in the transition to a greener future. This table highlights the world’s top five renewable energy producers, showcasing countries that are leading the way in sustainable development.
| Country | Renewable Energy Production (GWh) |
|—————|———————————-|
| China | 9,784,000 |
| United States | 7,100,000 |
| Brazil | 5,360,000 |
| India | 3,885,000 |
| Germany | 2,006,000 |
Table 10: Unemployment Rates by Age Group and Gender
Unemployment rates can vary significantly depending on age groups and gender. This table provides data on unemployment rates in different demographics, highlighting disparities that exist in the labor market.
| Age Group | Male Unemployment Rate (%) | Female Unemployment Rate (%) |
|————-|—————————-|——————————|
| 18-24 | 9.7 | 10.4 |
| 25-34 | 6.2 | 7.0 |
| 35-44 | 4.3 | 4.9 |
| 45-54 | 3.8 | 4.3 |
| 55 and over | 4.9 | 5.6 |
In conclusion, ML data has the power to transform information into captivating visual representations. Through these 10 tables, we have seen how ML algorithms provide valuable insights into diverse aspects of our world, from pollution causes to gender participation, health trends to travel accessibility, and much more. By harnessing the potential of ML, we can gain deeper understanding and make more informed decisions for a better future.
Frequently Asked Questions
How is machine learning data collected?
Data for machine learning can be collected through various methods such as web scraping, surveys, APIs, manual data entry, and even utilizing pre-existing datasets. The collection process depends on the specific requirements of the machine learning project and the type of data needed.
What are the types of machine learning data?
There are several types of data commonly used in machine learning, including numerical data, categorical data, time series data, text data, image data, and more. Each type of data requires different preprocessing and modeling techniques to effectively train machine learning algorithms.
Why is data preprocessing important in machine learning?
Data preprocessing plays a crucial role in machine learning as it helps clean, transform, and normalize the data to improve the accuracy and performance of the models. Preprocessing techniques include handling missing values, outlier treatment, feature scaling, encoding categorical variables, and more.
What is the role of feature selection in machine learning?
Feature selection involves identifying and selecting the most relevant features from the dataset to use for training machine learning models. This is essential to eliminate irrelevant or redundant features that may negatively impact model performance and improve computational efficiency.
How does overfitting affect machine learning models?
Overfitting occurs when a machine learning model performs very well on the training data but fails to generalize on unseen data. It happens when the model captures the noise or randomness in the training data instead of the underlying patterns. Techniques such as regularization, cross-validation, and early stopping are often used to mitigate overfitting.
What is the difference between supervised and unsupervised learning?
Supervised learning involves training a model using labeled data where the input features and their corresponding output values are known. In unsupervised learning, the model learns from unlabeled data and identifies patterns or structures without any predefined target variable. Supervised learning is used for prediction or classification tasks, while unsupervised learning is used for clustering or anomaly detection.
What is the role of evaluation metrics in machine learning?
Evaluation metrics are used to assess the performance of machine learning models. Common evaluation metrics include accuracy, precision, recall, F1-score, and area under the curve (AUC). These metrics provide insights into how well the model is performing and help in comparing different models or tuning hyperparameters.
How can bias in machine learning datasets be addressed?
Bias in machine learning datasets can be addressed by carefully examining the data collection process, identifying potential sources of bias, and taking corrective actions. This may involve diversifying the data sources, ensuring representative samples, performing data augmentation, or using bias-correction techniques.
What are some challenges in handling big data for machine learning?
Handling big data in machine learning poses challenges such as storage limitations, computational resource requirements, data preprocessing scalability, and efficient model training. Techniques like distributed computing, parallel processing, dimensionality reduction, and sampling can help mitigate these challenges.
What are some popular machine learning algorithms?
There are numerous machine learning algorithms available, each suitable for different tasks. Some popular algorithms include linear regression, logistic regression, decision trees, random forests, support vector machines (SVM), k-nearest neighbors (KNN), naive Bayes, neural networks, and gradient boosting algorithms like XGBoost and LightGBM.