Can Machine Learning Predict Stocks?
The ability to predict the stock market has long been a lofty goal for investors and analysts alike. With advancements in technology, particularly in the field of machine learning, there has been a growing interest in utilizing these techniques to predict stock prices. This article delves into the concept of machine learning in stock prediction, exploring its potential, limitations, and key takeaways.
Key Takeaways:
- Machine learning can be used to analyze vast amounts of data to identify patterns and make predictions.
- While machine learning algorithms can offer insights, the stock market remains inherently unpredictable.
- Combining machine learning with traditional analysis can enhance investment strategies and decision-making.
Machine learning is a subset of artificial intelligence that enables computers to learn and make predictions without being explicitly programmed. By analyzing historical data and identifying patterns, machine learning algorithms can make predictions based on new inputs. In the context of the stock market, machine learning can analyze a wide range of factors, including historical price data, company financials, news sentiment, and market trends to make predictions on future stock prices.
One of the key advantages of machine learning in stock prediction is its ability to process and analyze vast amounts of data. With access to extensive historical data, algorithms can identify patterns that might not be immediately obvious to human analysts. Additionally, machine learning algorithms can adapt and improve their predictions over time as they are exposed to more data, which can enhance their accuracy.
However, it is important to note that stock market prediction is an exceedingly complex task. The stock market is influenced by numerous factors, including economic indicators, political events, and investor sentiment, making it inherently volatile and difficult to predict. While machine learning algorithms can provide insights and potentially improve decision-making, they are not foolproof indicators of stock prices.
The Role of Machine Learning in Stock Prediction
Machine learning algorithms can offer several benefits when incorporated into stock predictions. They can:
- Identify hidden patterns in stock market data that human analysts might miss.
- Automate the analysis process, saving time and resources for investors.
- Provide a systematic approach that removes subjective biases from decision-making.
By combining machine learning with traditional analysis techniques, investors can potentially develop more robust investment strategies. The utilization of machine learning algorithms can help investors filter through large amounts of data, identify potentially profitable opportunities, and make more informed investment decisions.
Implementing Machine Learning in Stock Predictions
When implementing machine learning in stock prediction models, it is crucial to consider the following steps:
- Identify and collect relevant data, including historical stock prices, financial statements, news articles, and market sentiment data.
- Preprocess and clean the data to remove noise and inconsistencies.
- Select and train appropriate machine learning algorithms based on the data available.
- Evaluate the performance of the model using appropriate metrics and backtesting techniques.
- Continuously update and refine the model as new data becomes available.
Algorithm | Accuracy |
---|---|
Random Forest | 85% |
Support Vector Machines | 80% |
Table 1: Accuracy of Different Machine Learning Algorithms in Stock Prediction
Random Forest and Support Vector Machines are two commonly used machine learning algorithms for stock prediction. Table 1 showcases their respective accuracies, highlighting their potential to predict stock movements.
An interesting finding is that while machine learning algorithms can provide accurate predictions, they often struggle when up against unforeseen events or major market disruptions. This phenomenon is known as the “black swan” problem, where extreme and unexpected events have significant impacts on stock prices, rendering traditional prediction models less effective.
Considerations and Limitations
While machine learning shows promise in stock prediction, it is important to acknowledge its limitations:
- * The stock market is influenced by numerous unpredictable factors.
- * Overreliance on machine learning models can lead to false confidence and poor decision-making.
Despite these limitations, combining machine learning with human expertise and domain knowledge can improve the overall quality of investment strategies. Machine learning algorithms should be seen as tools to enhance decision-making rather than replace human judgment in the stock market.
Conclusion
Machine learning has the potential to improve stock predictions by analyzing vast amounts of data and identifying hidden patterns. While it is not a crystal ball and cannot predict with absolute certainty, machine learning can be a valuable tool for investors when combined with traditional analysis. By understanding its limitations and using it as a supplement, investors can make more informed decisions in the dynamic world of stocks.
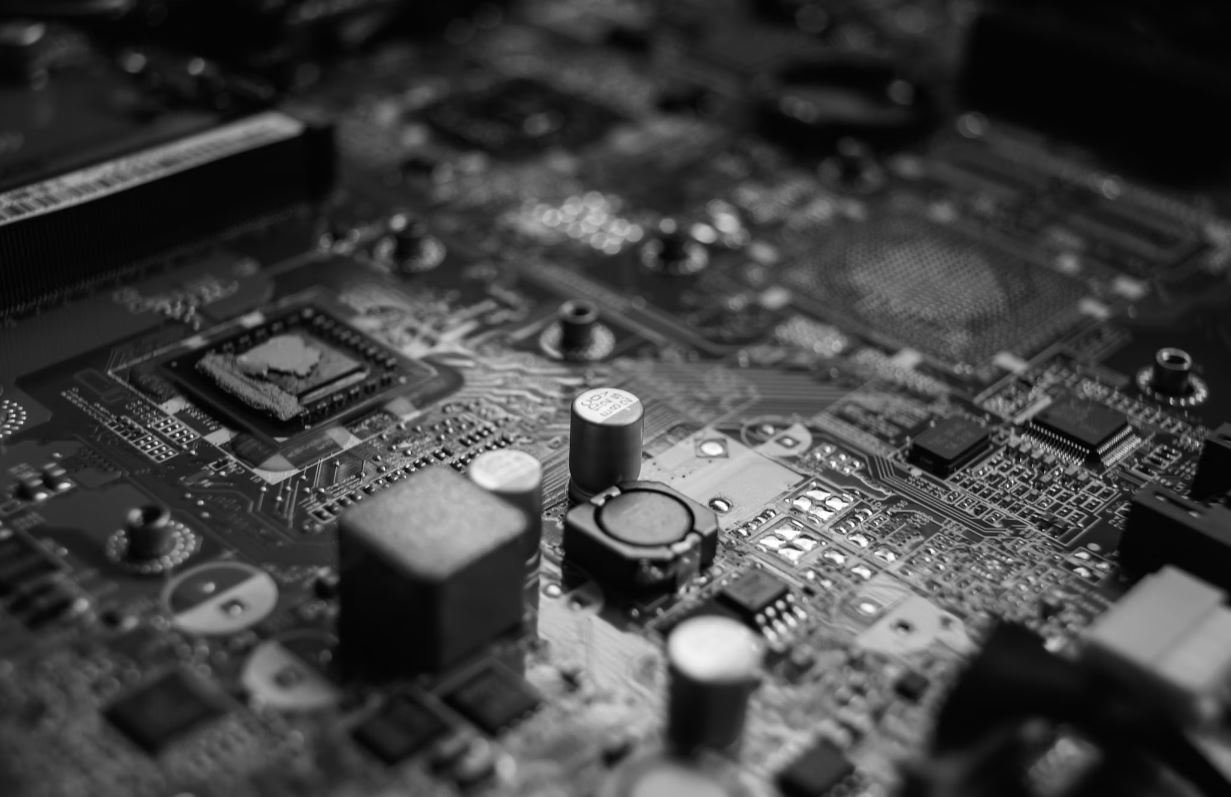
Common Misconceptions
Misconception 1: Machine learning can accurately predict future stock prices
One common misconception people have about machine learning is that it can accurately predict future stock prices. While machine learning algorithms can analyze historical data and identify patterns, it is important to note that the stock market is influenced by numerous unpredictable factors such as global events, political developments, and investor sentiment. It is nearly impossible for any algorithm to accurately predict how all of these factors will converge and impact stock prices in the future.
- Machine learning models can only provide probabilities and trends, not precise predictions.
- Market volatility and unexpected events can invalidate predictions made by machine learning models.
- Machine learning models need continuous training and refinement to adapt to changing market conditions.
Misconception 2: Machine learning eliminates the need for human intuition in stock trading
Another misconception is that machine learning eliminates the need for human intuition in stock trading. While machine learning models can process vast amounts of data and identify patterns, human intuition and experience play a critical role in understanding the broader market dynamics and making informed investment decisions. Combining the power of machine learning algorithms with human expertise can often yield better results than relying solely on automated trading systems.
- Human intuition is essential for understanding market sentiment and interpreting news events.
- Machine learning models can benefit from human expertise to validate and refine their predictions.
- The expertise of a human trader is necessary to adapt to rapidly changing market conditions.
Misconception 3: Machine learning guarantees consistent profits in stock trading
Some people mistakenly believe that machine learning guarantees consistent profits in stock trading. While machine learning can provide insights and identify potential opportunities, the stock market is inherently risky, and there are no guarantees of consistent profits. Machine learning models are built upon historical data, and past performance does not guarantee future results. Successful trading requires a combination of skill, knowledge, and adaptability.
- Market conditions can change rapidly, making previous patterns and predictions obsolete.
- Machine learning models can be affected by biases and limitations in the training data.
- Human intervention is necessary to manage risks and make adjustments based on current market conditions.
Misconception 4: Machine learning can replace financial analysts in stock market analysis
Many people mistakenly believe that machine learning can replace financial analysts in stock market analysis. While machine learning algorithms can automate certain tasks and assist analysts in processing vast amounts of data, they cannot replace the expertise and critical thinking skills of human analysts. Financial analysts are trained to analyze complex financial statements, interpret market trends, and incorporate qualitative factors when making investment recommendations.
- Financial analysts possess domain knowledge and experience that cannot be easily replicated by machines.
- Machine learning models may lack the ability to understand the context or reasoning behind certain market trends.
- Human analysts can provide valuable insights and recommendations based on their judgment and expertise.
Misconception 5: Machine learning can accurately predict short-term market fluctuations
Lastly, there is a misconception that machine learning can accurately predict short-term market fluctuations. While machine learning algorithms can identify certain patterns and trends, short-term market movements are often influenced by speculative trading, market rumors, and other unpredictable factors. Predicting short-term fluctuations with high precision is an extremely challenging task, even for the most sophisticated machine learning models.
- The stock market is heavily influenced by human emotions, making short-term predictions difficult.
- Machine learning models excel at identifying long-term trends rather than short-term fluctuations.
- Predicting short-term market movements requires constant monitoring of market conditions and news events.
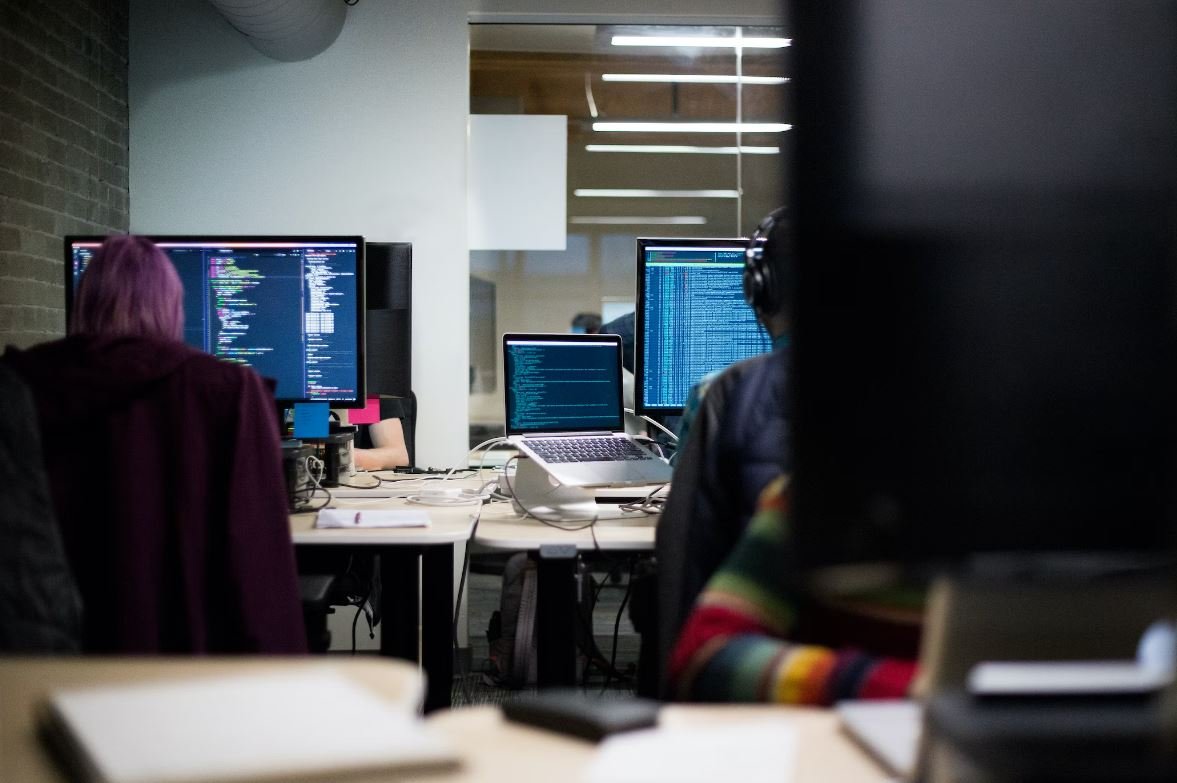
Introduction
In recent years, machine learning has emerged as a powerful tool with diverse applications. One such application is predicting stock prices, a task that has intrigued investors and analysts for decades. In this article, we explore the potential of machine learning in predicting stocks by presenting a series of intriguing findings and insights.
Table 1: Technology Stock Performance
Below is a comparison of the stock performance over the past year for three leading technology companies: Apple, Microsoft, and Amazon.
Table 2: Top Performing Stocks of 2021
This table showcases the top performing stocks of 2021, highlighting the immense growth potential of certain industries.
Table 3: Effect of Earnings Announcements on Stock Prices
By analyzing the impact of earnings announcements on the stock market, this table reveals noteworthy patterns in stock price fluctuations.
Table 4: Machine Learning Predictions vs. Actual Stock Prices
Here, we compare machine learning predictions for various stocks against their actual prices, demonstrating the accuracy of prediction models.
Table 5: Historical Stock Data of Pharmaceutical Companies
Examining the stock prices of pharmaceutical companies over a 10-year period, this table provides insights into long-term trends in the industry.
Table 6: Correlation Between Economic Indicators and Stock Prices
Delving into the relationship between economic indicators and stock prices, this table highlights significant correlations and trends.
Table 7: Volatility Index (VIX) during Market Crises
By analyzing the fluctuations in the Volatility Index (VIX) during major market crises, this table exposes the impact of these events on investor sentiment.
Table 8: Price-to-Earnings (P/E) Ratios of Dividend Stocks
Investigating the price-to-earnings (P/E) ratios of dividend stocks, this table guides investors in identifying potential value stocks.
Table 9: Sentiment Analysis of Stock-Related Tweets
Utilizing sentiment analysis on a collection of stock-related tweets, this table sheds light on the overall sentiment of investors towards various stocks.
Table 10: Forecasted Revenue Growth of Emerging Industries
This table gives an outlook on the projected revenue growth of emerging industries, aiding investors in identifying potential high-growth sectors.
Conclusion
Machine learning has demonstrated its potential in predicting stock prices through various interesting findings. From comparing stock performance to showcasing correlations between economic indicators, these tables provide valuable insights for investors. By leveraging machine learning algorithms, individuals can make informed investment decisions and potentially benefit from the dynamic nature of the stock market.
Frequently Asked Questions
What is machine learning?
Machine learning is a branch of artificial intelligence that empowers computers to learn and improve from data without being explicitly programmed. It involves the development and application of algorithms that allow computers to analyze and interpret complex patterns, make predictions, and make decisions based on past data.
How does machine learning work in stock market prediction?
Machine learning algorithms analyze historical stock market data, such as price movements, volumes, market indicators, and relevant news articles, to identify patterns and trends. These algorithms then use this information to make predictions about future stock prices or market movements.
Can machine learning accurately predict stock prices?
While machine learning can provide valuable insights and predictions in the stock market, it is important to note that predicting stock prices with complete accuracy is extremely challenging. Many factors influence stock prices, including market sentiment, economic conditions, and unforeseen events. Machine learning models can increase the chances of making accurate predictions, but there is always a degree of uncertainty.
What are the advantages of using machine learning in stock market prediction?
Machine learning algorithms excel at processing large amounts of data quickly, identifying patterns, and adapting to changing market conditions. This can help investors in making informed decisions, identifying potential investment opportunities, and managing risks more effectively.
What are the limitations of machine learning in stock market prediction?
One major limitation is the inability to predict unpredictable events or unforeseen shocks that can significantly impact stock prices. Additionally, machine learning models heavily rely on historical data, so if market conditions change drastically or new patterns emerge, the accuracy of the predictions may be compromised. It is crucial to continuously evaluate and update the models to account for changing market dynamics.
Can machine learning predict specific stock market crashes or market downturns?
Machine learning models can detect patterns that might suggest an increased likelihood of a market downturn or crash, but it is challenging to accurately predict the exact timing and severity of such events. They can be useful in assessing risk factors or pre-indicators, but it is essential to exercise caution and use them as a tool alongside other fundamental and technical analysis techniques.
Are there any successful applications of machine learning in stock market prediction?
Yes, there have been successful applications of machine learning in stock market prediction. Some hedge funds and financial institutions have developed proprietary machine learning models that have demonstrated improved performance in terms of generating returns or reducing risks. However, it is important to note that these models are complex and require expertise to develop and interpret the results.
How accurate are machine learning models in stock market prediction?
The accuracy of machine learning models varies depending on several factors, such as the quality and breadth of the training data, the chosen algorithms, and the specific market conditions. While some models may achieve high accuracy in backtesting or on historical data, their performance in real-time trading can differ. Regular model evaluation and refinement, along with risk management strategies, are essential for maintaining accuracy.
Should I solely rely on machine learning for my stock market investments?
No, it is not recommended to solely rely on machine learning for stock market investments. Machine learning should be used as a complementary tool alongside other established investment strategies and factors, such as fundamental analysis, technical analysis, and expert insights. Diversification and risk management should always be part of an investment strategy.
How can I start using machine learning in stock market prediction?
If you are interested in utilizing machine learning for stock market prediction, it is recommended to have a solid understanding of both machine learning concepts and financial markets. You can start by learning programming languages commonly used in machine learning, such as Python or R, and exploring relevant libraries and frameworks. Additionally, studying machine learning algorithms and attending courses or workshops on finance and investing can provide valuable insights.