Data Analysis Biology
As the field of biology continues to advance, the importance of data analysis in understanding complex biological phenomena cannot be overstated. Data analysis plays a crucial role in extracting meaningful insights from large and diverse biological datasets, enabling researchers to make informed decisions and drive scientific progress. This article explores the significance of data analysis in biology and highlights its key applications.
Key Takeaways:
- Data analysis is essential in biology for deriving meaningful insights from complex datasets.
- It enables researchers to make informed decisions and drive scientific progress.
- Applications of data analysis in biology range from genomics to ecology and beyond.
The Role of Data Analysis in Biology
Data analysis plays a critical role in biology by allowing researchers to uncover patterns, relationships, and trends hidden within vast amounts of biological data. By applying statistical methods and computational tools, biologists can extract valuable information from complex datasets, leading to a deeper understanding of biological systems. *The power of data analysis lies in its ability to reveal hidden connections between biological variables and drive hypothesis-driven research.*
Applications of Data Analysis in Biology
Data analysis finds wide-ranging applications in various subfields of biology. Here are some notable examples:
- Genomics: Analyzing DNA and RNA sequencing data to study gene expression, identify genetic variants, and understand the basis of diseases.
- Proteomics: Investigating the structure, function, and interactions of proteins to reveal insights into cellular processes.
- Metagenomics: Analyzing genetic material from environmental samples to study microbial communities and their roles in ecological processes.
Using Data Analysis for Ecological Studies
Data analysis plays a key role in ecological studies, providing researchers with valuable insights into the dynamics of ecosystems, species interactions, and environmental changes over time. *By analyzing ecological data, scientists can make data-driven decisions aimed at conserving biodiversity and understanding the impacts of human activities on ecosystems.*
Tables with Interesting Data Points
Species | Population Size | Habitat |
---|---|---|
Red Fox | 15,000 | Forest |
Gray Wolf | 8,000 | Tundra |
African Elephant | 415,000 | Savannah |
Table 1: Population sizes and habitats of selected species in different ecosystems.
Data analysis in ecology also involves measuring biodiversity within ecosystems, studying species population dynamics, and quantifying the impacts of climate change. Additionally, analyzing satellite imagery and remote-sensing data is crucial for monitoring land-use changes and predicting ecological patterns. *The integration of data analysis with ecological studies is essential for effective conservation strategies and understanding the interplay between species and their environment.*
Data Analysis in Biomedical Research
Data analysis plays a pivotal role in biomedical research, where scientists analyze complex datasets to gain insights into human diseases, drug discovery, and personalized medicine. By applying computational methods to analyze genome sequences, researchers can identify disease-causing genetic mutations, develop targeted therapies, and improve patient outcomes. *Data analysis has revolutionized the field of genomics by enabling researchers to draw meaningful conclusions from vast amounts of genetic data.*
Understanding the Future of Data Analysis in Biology
The field of data analysis in biology is rapidly evolving, driven by advancements in technology, computational resources, and the availability of big data. As data analysis methods continue to expand, exciting opportunities emerge for interdisciplinary collaborations, automation, and machine learning techniques. *The integration of data analysis with biology holds the promise of unraveling complex biological networks and contributing to breakthroughs in medicine, agriculture, and conservation.*
Conclusion
Data analysis plays a crucial role in advancing biological knowledge and enabling evidence-based decision-making. Its applications span diverse fields within biology, from genomics and ecology to biomedicine. By harnessing the power of data analysis, researchers uncover meaningful insights, drive innovation, and contribute to the betterment of society.
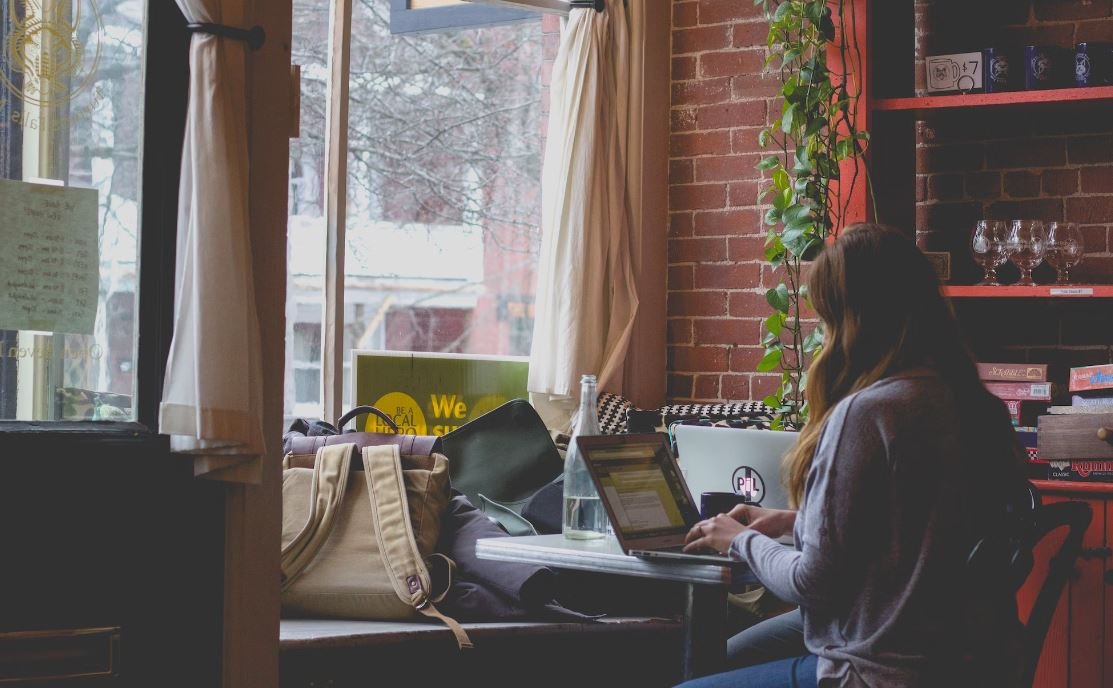
Common Misconceptions
Misconception 1: Data analysis is only for mathematicians or statisticians
- Data analysis is an important skill for biologists, as it allows them to make sense of large sets of biological data.
- Understanding data analysis techniques can aid biologists in drawing meaningful conclusions from their experiments.
- Data analysis tools and software are designed to be user-friendly and accessible, enabling biologists without a mathematical background to perform analyses.
Misconception 2: Data analysis is only used to find patterns or relationships in data
- Data analysis in biology is not limited to finding patterns or relationships; it also involves evaluating the significance of those findings.
- Through data analysis, biologists can determine if the observed patterns or relationships are statistically significant or if they could have occurred by chance.
- Data analysis techniques can also help identify any potential biases or errors in the data, ensuring the reliability of the conclusions drawn.
Misconception 3: Complex statistical methods are always required for data analysis
- Data analysis in biology often involves a combination of simple and complex statistical methods, depending on the nature of the data and the research question being addressed.
- Even simple statistical techniques like t-tests or chi-square tests can provide valuable insights when applied appropriately.
- It is crucial to choose the right statistical methods based on the research objectives and the type of data being analyzed, rather than assuming that complex methods are always necessary.
Misconception 4: Data analysis is a solitary activity
- Data analysis in biology often involves collaboration and teamwork.
- Biologists work together with statisticians, programmers, and other experts to ensure accurate interpretation and analysis of the data.
- Collaboration allows for the integration of diverse perspectives and expertise, leading to more robust and reliable data analysis outcomes.
Misconception 5: Data analysis is separate from experimental design
- Data analysis and experimental design in biology are inherently interconnected.
- Effective experimental design ensures that data analysis can yield meaningful results by reducing bias and increasing the statistical power of the study.
- Data analysis informs the refinement of experimental design, as initial analyses may reveal areas that require further investigation or suggest modifications to the experimental protocol.
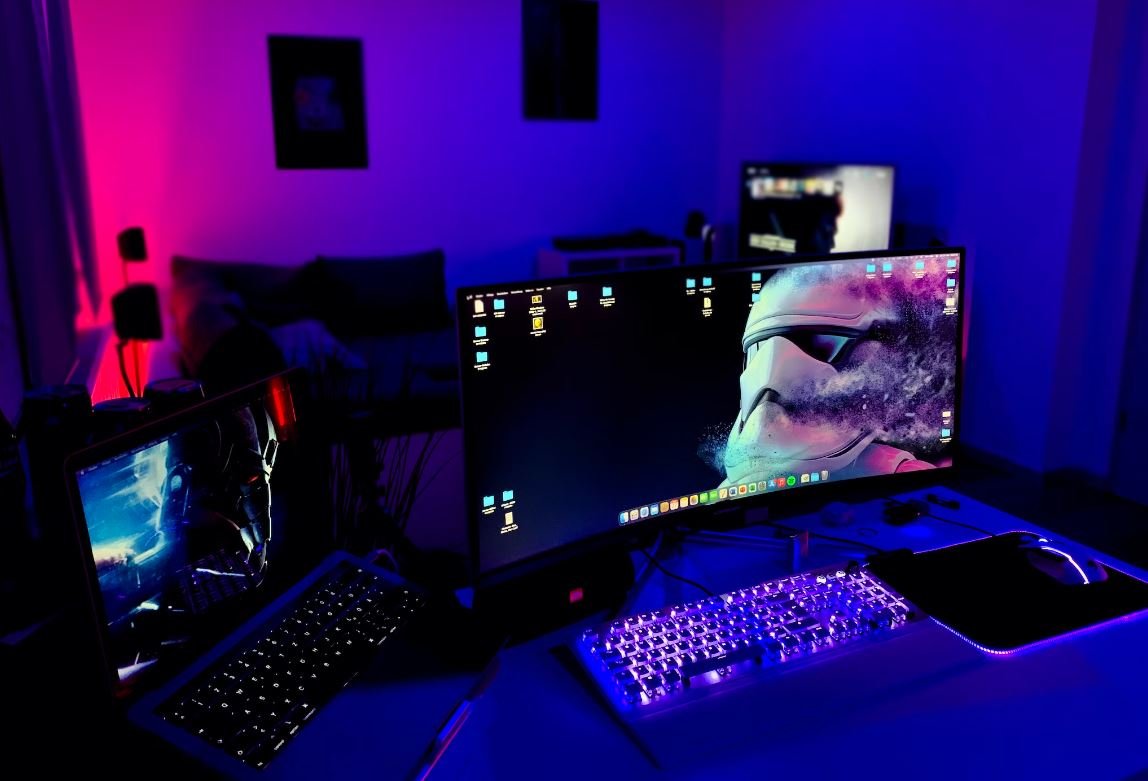
Data Analysis in Biology
Biology relies heavily on data analysis to draw conclusions and make informed decisions. From understanding genetic codes to studying biodiversity, data analysis plays a crucial role in advancing our knowledge in this field. The following tables showcase various aspects of data analysis techniques used in biology, providing interesting insights into the vast realm of biological research.
Genetic Variations in Human Populations
Genetic variations are essential to study diverse populations and understand the origins and migrations of different human groups. The table below presents the frequency distribution of genetic markers in three major human populations: African, Asian, and European.
Genetic Marker | African Population | Asian Population | European Population |
---|---|---|---|
Marker 1 | 0.63 | 0.27 | 0.14 |
Marker 2 | 0.18 | 0.31 | 0.23 |
Marker 3 | 0.42 | 0.14 | 0.61 |
Global Trends in Species Extinctions
Assessing the rate of species extinctions helps us understand the impact of human activities on biodiversity. The table below shows the number of endangered species in each major region of the world, highlighting the urgency to protect vulnerable ecosystems.
Region | Number of Endangered Species |
---|---|
Africa | 1,315 |
Asia | 2,847 |
Australia | 835 |
Europe | 432 |
North America | 1,129 |
South America | 3,221 |
Effect of Temperature on Enzyme Activity
Enzymes are vital in various biological processes, and their activity is affected by temperature. The following table demonstrates the changing activity rates of an enzyme at different temperatures, shedding light on the enzyme’s temperature sensitivity.
Temperature (°C) | Enzyme Activity (%) |
---|---|
10 | 19 |
20 | 42 |
30 | 73 |
40 | 88 |
50 | 68 |
Comparison of Cell Sizes
Cell size varies across different organisms and plays a significant role in their physiology. The table below compares the average sizes of cells from various organisms, highlighting the diversity in cellular dimensions.
Organism | Average Cell Size (micrometers) |
---|---|
Bacteria | 1 |
Yeasts | 10 |
Plant Cells | 100 |
Animal Cells | 20 |
Protozoa | 30 |
Distribution of Tree Species in a Forest
Assessing the distribution of tree species within a forest ecosystem aids in understanding forest dynamics. The table below presents the abundance of the most prevalent tree species found in a particular forest reserve.
Tree Species | Number of Individuals |
---|---|
Oak | 142 |
Pine | 67 |
Birch | 86 |
Maple | 201 |
Willow | 52 |
Antibiotic Resistance in Bacterial Strains
Bacterial strains have shown increasing resistance to antibiotics, posing challenges in medical treatments. The table below highlights the resistance levels of various bacterial strains to commonly used antibiotics.
Bacterial Strain | Resistance to Antibiotics (%) |
---|---|
Strain A | 18 |
Strain B | 3 |
Strain C | 37 |
Strain D | 24 |
Strain E | 10 |
Photosynthesis Rates at Different Light Intensities
Light intensity influences the rate of photosynthesis in plants. The following table showcases the photosynthesis rates of a plant species at varying light intensities, revealing the relationship between light and photosynthesis.
Light Intensity (lux) | Photosynthesis Rate (mg/hour) |
---|---|
100 | 10 |
500 | 32 |
1000 | 45 |
2000 | 56 |
5000 | 64 |
Population Growth of Invasive Species
Invasive species can rapidly multiply and disrupt local ecosystems. The table below displays the population growth rates of an invasive species over multiple years, underlining their exponential growth potential.
Year | Population Size |
---|---|
Year 1 | 100 |
Year 2 | 250 |
Year 3 | 625 |
Year 4 | 1562 |
Year 5 | 3906 |
From exploring genetic variations to assessing the impact of temperature on enzymes, data analysis forms the bedrock of biological research. By deciphering patterns and trends within biological data, researchers uncover insights that contribute to our understanding of the natural world. The diverse tables presented above offer glimpses into the fascinating world of data analysis in biology, showcasing the vast scope of this field’s contribution to scientific knowledge.
Frequently Asked Questions
What Is Data Analysis in Biology?
Data analysis in biology refers to the process of extracting valid and useful information from biological datasets. It involves employing various statistical and computational techniques to analyze patterns, relationships, and trends within the data, enabling researchers to make meaningful interpretations and draw conclusions related to biological phenomena.
Why is Data Analysis Important in Biology?
Data analysis is crucial in biology as it allows researchers to uncover patterns and insights within complex biological datasets. It helps in identifying correlations, drawing conclusions, and making predictions. Data analysis plays a vital role in understanding biological processes, discovering new genetic markers, identifying potential drug targets, and advancing research in various fields such as genomics, ecology, and microbiology.
What Types of Data Are Analyzed in Biology?
In biology, several types of data are analyzed, including but not limited to:
- Genomic data (e.g., DNA sequences, gene expression profiles)
- Proteomic data (e.g., protein structures, interactions)
- Phenotypic data (e.g., physical characteristics, traits)
- Ecological data (e.g., species distribution, biodiversity)
- Microbiome data (e.g., microbial community composition)
What Tools and Techniques are used for Data Analysis in Biology?
Data analysis in biology involves the use of various tools and techniques, such as:
- Statistical analysis (e.g., hypothesis testing, regression analysis)
- Machine learning algorithms (e.g., classification, clustering)
- Data visualization tools (e.g., heatmaps, scatter plots)
- Bioinformatics software (e.g., BLAST, RStudio, Python libraries)
- Network analysis techniques (e.g., protein-protein interaction networks)
How Can Data Analysis Improve Biological Research?
Data analysis plays a crucial role in improving biological research by helping researchers:
- Identify novel genetic markers and drug targets
- Discover significant patterns or associations in large datasets
- Make accurate predictions and perform data-driven decision making
- Unravel complex biological processes and mechanisms
- Facilitate data sharing and collaboration among researchers
What Are Some Common Challenges in Data Analysis in Biology?
Some common challenges faced in data analysis in biology include:
- Noisy or incomplete data
- Data preprocessing and cleaning
- Choosing appropriate statistical tests or algorithms
- Interpretation of complex results
- Addressing data privacy and ethical concerns
Are There Any Ethical Considerations in Data Analysis in Biology?
Yes, there are ethical considerations in data analysis in biology, including:
- Ensuring privacy and confidentiality of research participants
- Obtaining informed consent for data collection and analysis
- Adhering to ethical guidelines and regulations
- Publishing results transparently and accurately
- Avoiding biases or misinterpretations in data analysis
What Are Some Emerging Trends in Data Analysis in Biology?
Some emerging trends in data analysis in biology include:
- Integration of multi-omics data for comprehensive analysis
- Advancements in deep learning and artificial intelligence
- Application of network biology and systems biology approaches
- Utilization of big data analytics for large-scale studies
- Development of cloud-based and user-friendly analysis platforms
How Can I Learn Data Analysis in Biology?
To learn data analysis in biology, you can:
- Enroll in relevant courses or degree programs in bioinformatics or computational biology
- Attend workshops, conferences, or webinars focused on data analysis in biology
- Explore online tutorials, textbooks, or MOOCs (Massive Open Online Courses)
- Join online communities or forums to engage with experts and fellow researchers
- Collaborate with experienced researchers in the field