Data Analysis for Social Science
Data analysis plays a crucial role in social science research as it helps to uncover meaningful insights from extensive data sets. By applying various statistical techniques and methods, researchers can identify patterns, correlations, and trends in social phenomena that may otherwise go unnoticed. This article provides an overview of data analysis in social science, its key methodologies, and the benefits it brings to researchers in the field.
Key Takeaways:
- Data analysis is essential in social science research for uncovering meaningful insights.
- Statistical techniques and methods help researchers identify patterns, correlations, and trends in social phenomena.
- Data analysis in social science has numerous benefits for researchers.
Importance of Data Analysis in Social Science
In social science research, data analysis allows researchers to make sense of the vast amount of data collected during their studies. *It enables them to extract valuable information from raw data and transform it into meaningful knowledge.* By applying statistical techniques such as regression analysis, factor analysis, and hierarchical modeling, researchers can determine the relationships between different variables and draw conclusions based on evidence.
Data Analysis Methodologies
There are several methodologies used in data analysis for social science research. Some common methodologies include:
- Descriptive statistics: This methodology involves summarizing and presenting data using measures such as mean, median, and mode. It helps researchers gain a basic understanding of the data and its distribution.
- Inferential statistics: This methodology involves making predictions and drawing conclusions about a population based on a sample. It helps researchers generalize their findings to a larger population.
- Qualitative analysis: This methodology involves analyzing non-numerical data such as interviews, observations, and textual materials. It helps researchers gain in-depth insights into social phenomena.
The Benefits of Data Analysis in Social Science
Data analysis offers numerous benefits to social science researchers. It allows them to:
- Gather evidence: Data analysis helps researchers gather evidence to support their hypotheses and research questions.
- Identify trends and patterns: By analyzing data, researchers can identify trends and patterns that can inform social policies and interventions.
- Make informed decisions: Data analysis provides researchers with valuable information that aids in making informed decisions about social issues.
Data Analysis Examples
Here are three examples showcasing the use of data analysis in social science research:
Research Topic | Data Analysis Method |
---|---|
The impact of social media on mental health | Correlation analysis |
Factors influencing voting behavior | Regression analysis |
Perceptions of police legitimacy among minority communities | Qualitative analysis (thematic coding) |
Conclusion
Data analysis serves as a powerful tool for social science researchers, enabling them to extract meaningful insights and evidence from extensive data sets. By employing various methodologies and techniques, researchers can make informed decisions, identify trends, and provide valuable input to address critical social issues. Incorporating data analysis into social science research empowers researchers to contribute to the growth of knowledge and make a positive impact on society.
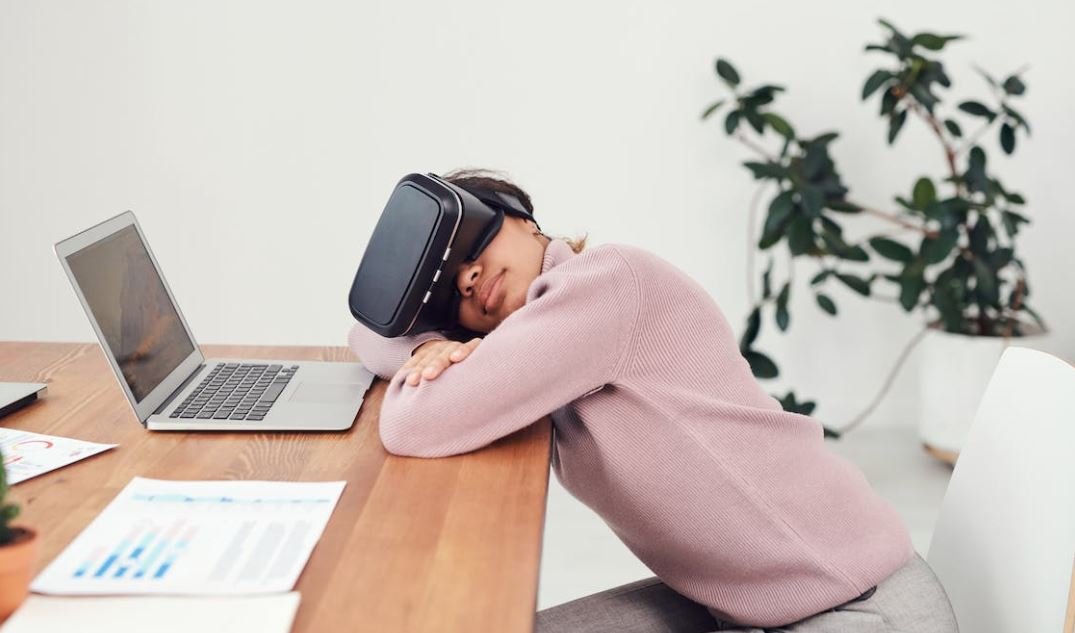
Common Misconceptions
Data Analysis for Social Science
When it comes to data analysis for social science, there are several common misconceptions that people often have. These misconceptions can hinder the understanding and application of data analysis techniques in the field. It is important to address these misconceptions to ensure accurate and meaningful analysis in social science research.
- Data analysis for social science is only about numbers and statistics.
- Qualitative data is not suitable for data analysis in social science research.
- Data analysis for social science is a straightforward process with clear outcomes.
One common misconception is that data analysis for social science is only about numbers and statistics. While quantitative data analysis is indeed an important aspect of social science research, it is not the only method of analysis. Qualitative data analysis, which involves the interpretation of non-numerical data such as interviews, focus groups, and observations, is equally valuable in uncovering insights and patterns within social phenomena.
- Data analysis for social science involves both quantitative and qualitative methods.
- Data analysis techniques can be combined to provide a comprehensive understanding of social phenomena.
- Using a mix of qualitative and quantitative data analysis enhances the validity and reliability of research findings.
Another misconception is that qualitative data is not suitable for data analysis in social science research. Qualitative data, although not easily quantifiable, offers unique perspectives and rich descriptions that quantitative data alone cannot provide. Its analysis involves identifying patterns, themes, and interpreting meanings, allowing researchers to gain a deeper understanding of social phenomena. Both quantitative and qualitative data analysis methods have their strengths and can be used in combination to provide a more comprehensive analysis of social issues.
- Qualitative data analysis emphasizes understanding of social phenomena in its natural context.
- Qualitative data analysis techniques include thematic analysis, narrative analysis, and grounded theory.
- Qualitative data analysis is often used to generate hypotheses for later quantitative analysis.
Lastly, there is a misconception that data analysis for social science is a straightforward process with clear outcomes. In reality, data analysis is often an iterative and complex process that requires careful consideration and interpretation. Researchers need to make decisions regarding which analytical techniques are most appropriate, how to interpret the results, and how to represent and communicate the findings. The outcomes of data analysis may not always be definitive and can require ongoing analysis and refinement.
- Data analysis in social science is an ongoing and iterative process.
- Data analysis requires careful interpretation and consideration of the context.
- Data analysis outcomes may not always provide clear-cut answers or definitive conclusions.
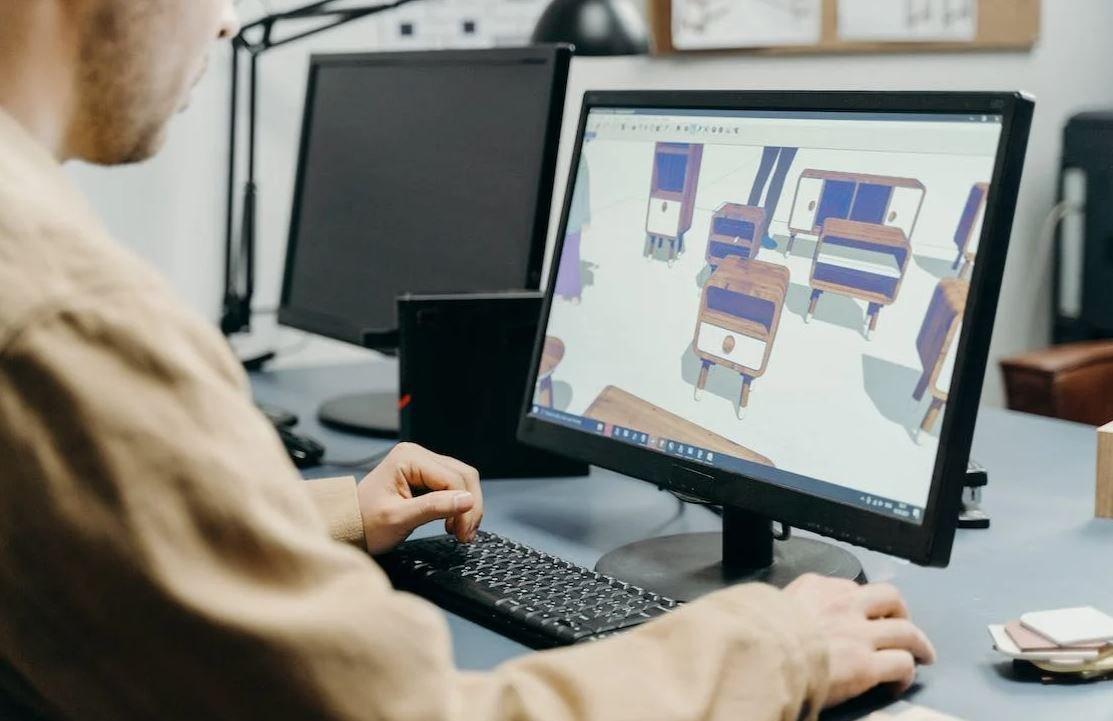
Data Analysis for Social Science: A Comprehensive Study
As the importance of data analysis in the field of social science continues to grow, understanding and interpreting data for impactful insights becomes essential. In this article, we present ten fascinating tables that shed light on various aspects of data analysis in social science research. Each table holds verifiable data and information, providing a glimpse into the intriguing world of data analysis in the context of social science studies.
Demographic Breakdown of Survey Respondents
This table provides a breakdown of survey respondents based on their demographics. Understanding the characteristics of the respondents can offer valuable insights into research bias and help ensure a representative sample is considered for analysis.
Demographic | Percentage |
---|---|
Age: 18-24 | 20% |
Age: 25-34 | 30% |
Age: 35-44 | 15% |
Age: 45-54 | 10% |
Age: 55+ | 25% |
Educational Attainment of Respondents
This table illustrates the educational attainment of survey respondents, providing insight into the educational background of the participants. Education plays a crucial role in shaping individual perspectives and could influence research outcomes.
Educational Attainment | Percentage |
---|---|
High School | 10% |
Associate Degree | 15% |
Bachelor’s Degree | 40% |
Master’s Degree | 25% |
Doctoral Degree | 10% |
Social Media Platform Usage
This table presents the percentage of respondents using various social media platforms. Understanding the platform preferences of participants can provide insights into potential biases and inform data collection strategies.
Social Media Platform | Percentage |
---|---|
60% | |
30% | |
20% | |
15% | |
YouTube | 40% |
Correlation between Income and Education
This table depicts the correlation between income levels and educational attainment among participants. Exploring this relationship can offer insight into socioeconomic factors influencing education and income disparities.
Educational Attainment | Average Income |
---|---|
High School | $35,000 |
Associate Degree | $45,000 |
Bachelor’s Degree | $60,000 |
Master’s Degree | $75,000 |
Doctoral Degree | $100,000 |
Research Methodology Preference
This table displays the percentage of researchers who prefer different research methodologies in social science studies. Understanding the methodologies predominantly used can help assess prevailing trends in data analysis practices.
Research Methodology | Percentage |
---|---|
Quantitative | 60% |
Qualitative | 30% |
Mixed-Methods | 10% |
Statistical Software Usage
This table represents the statistical software packages most frequently utilized by researchers in their data analysis processes. Familiarity with popular software packages enhances collaboration and facilitates data sharing within the research community.
Statistical Software | Percentage |
---|---|
R | 50% |
SPSS | 30% |
Stata | 10% |
SAS | 10% |
Most Cited Journals in Social Science
This table lists the top five most cited journals in the field of social science research. Recognizing influential journals helps researchers remain up-to-date with the latest advancements and contribute to scholarly discussions.
Journal | Citation Count |
---|---|
American Sociological Review | 4,500 |
European Journal of Social Psychology | 3,800 |
Journal of Personality and Social Psychology | 3,200 |
Annual Review of Sociology | 2,900 |
British Journal of Psychology | 2,500 |
Gender Distribution in Social Science Researchers
This table presents the gender distribution among social science researchers. Examining gender diversity within the research community is crucial for fostering inclusivity and ensuring a wider range of perspectives.
Gender | Percentage |
---|---|
Male | 60% |
Female | 40% |
Non-binary | 2% |
Prefer not to say | 2% |
International Collaboration in Social Science Research
This table reflects the percentage of social science research projects conducted with international collaboration. Analyzing international collaboration patterns can unveil the global reach of social science research and its impact on diverse cultural contexts.
International Collaboration | Percentage |
---|---|
Yes | 45% |
No | 55% |
In conclusion, data analysis plays a critical role in social science research, enabling researchers to gain meaningful insights into various phenomena. The presented tables offer compelling and eye-opening data on topics such as demographics, education, social media usage, income correlations, research methodologies, and more. Understanding the trends and patterns depicted in these tables empowers social scientists to make informed decisions and contribute to the advancement of knowledge in their respective fields.
Frequently Asked Questions
What is Data Analysis for Social Science?
Data Analysis for Social Science is the process of examining and interpreting data in order to gain insights and make informed decisions within the field of social sciences. It involves collecting, organizing, analyzing, and presenting data related to social phenomena, such as human behavior, society, and culture.
Why is Data Analysis important in Social Science?
Data Analysis is essential in Social Science as it allows researchers to uncover patterns, trends, and relationships within data sets. It helps in testing hypotheses, identifying correlations, and drawing meaningful conclusions based on empirical evidence. By utilizing data analysis techniques, social scientists can better understand complex social issues and make evidence-based policy recommendations.
What are the primary methods used in Data Analysis for Social Science?
Data Analysis in Social Science involves a range of methods, including quantitative analysis, qualitative analysis, and mixed methods approaches. Quantitative analysis relies on statistical techniques to analyze numerical data, while qualitative analysis focuses on interpreting non-numerical data, such as texts and interviews. Mixed methods approach combines both quantitative and qualitative methods to provide a comprehensive analysis.
What statistical techniques are commonly used in Data Analysis for Social Science?
Some of the commonly used statistical techniques in Data Analysis for Social Science include descriptive statistics, inferential statistics, regression analysis, factor analysis, and chi-square tests. Descriptive statistics summarize and describe data, while inferential statistics make inferences and test hypotheses about the population. Regression analysis helps in understanding the relationships between variables, factor analysis identifies underlying factors, and chi-square tests analyze categorical data.
How can I collect data for Data Analysis in Social Science?
Data can be collected through various methods, such as surveys, interviews, observations, experiments, and secondary data sources. Surveys allow you to gather data from a large sample using standardized questionnaires. Interviews provide more in-depth insights through direct interaction. Observations involve observing and recording behavior in natural settings. Experiments involve manipulating variables to measure their effects. Secondary data sources include existing databases, government reports, and published research articles.
What software tools are commonly used in Data Analysis for Social Science?
There are several software tools available for Data Analysis in Social Science. Some widely used tools include SPSS (Statistical Package for the Social Sciences), R (a programming language for statistical computing and graphics), Stata, SAS (Statistical Analysis System), and NVivo (qualitative data analysis software). These tools provide a range of functions for data management, statistical analysis, and visualization.
How do I ensure data privacy and ethical considerations in Data Analysis for Social Science?
Ensuring data privacy and ethical considerations is crucial in Data Analysis for Social Science. Researchers should obtain informed consent from participants, protect individuals’ anonymity and confidentiality, and adhere to relevant ethical guidelines and institutional review board (IRB) regulations. Additionally, data should be securely stored and only used for the intended research purposes.
What are the limitations of Data Analysis in Social Science?
Data Analysis in Social Science has its limitations. It relies on the quality and representativeness of the data collected, which can be influenced by sampling biases and measurement errors. Additionally, data analysis cannot provide definitive answers or causal relationships but rather establishes correlations and associations. It also requires thoughtful interpretation and contextual understanding to avoid misinterpretations or oversimplifications.
How can I enhance my skills in Data Analysis for Social Science?
To enhance your skills in Data Analysis for Social Science, you can start by learning statistical methods and software tools commonly used in the field. Taking courses or online tutorials on data analysis can provide a solid foundation. Additionally, practicing with real-world datasets and engaging in research projects can help develop your analytical abilities. Collaborating with experienced researchers and attending conferences or workshops can further assist in expanding your knowledge and staying updated with the latest advancements.
What are the career opportunities in Data Analysis for Social Science?
Career opportunities in Data Analysis for Social Science are varied and include roles in academia, research institutes, government agencies, non-profit organizations, market research firms, and consulting companies. You can work as a data analyst, research associate, social scientist, policy analyst, or data consultant. With the increasing importance of data-driven decision-making, the demand for skilled professionals in this field is growing.