Data Analysis in Qualitative Research
Qualitative research aims to understand individual experiences, behaviors, and attitudes through in-depth interviews, focus groups, and observations. Analyzing qualitative data is a crucial step in the research process, as it helps researchers draw meaningful conclusions and insights. This article explores the importance of data analysis in qualitative research, key methodologies, and best practices.
Key Takeaways:
- Data analysis is a crucial step in qualitative research, helping researchers make sense of complex and rich data.
- Qualitative data analysis involves the identification of themes, patterns, and relationships.
- Various strategies and software tools can assist researchers in analyzing qualitative data effectively.
Methods of Data Analysis in Qualitative Research
Qualitative data analysis involves several methods that aid in organizing and interpreting data. By employing these approaches, researchers can identify recurring themes, factors, and connections within the data. Some commonly used methods include:
- Thematic Analysis: This approach involves identifying and analyzing recurring patterns or themes within the data, offering important insights into participants’ experiences and opinions.
Thematic analysis helps uncover underlying patterns that would otherwise remain unnoticed. - Content Analysis: In this method, the text is systematically coded and categorized to identify themes or commonalities, structures, and meanings within the data.
Content analysis provides a systematic and objective means of analyzing textual data. - Grounded Theory: This method involves constructing theories and developing concepts from the data, allowing the emergence of new perspectives and theoretical frameworks.
Grounded theory helps researchers develop a deep understanding of a phenomenon through iterative data analysis.
Best Practices for Analyzing Qualitative Data
Effective analysis of qualitative data requires adherence to certain best practices. Following these guidelines promotes consistency, rigor, and validity in the research findings:
- Transcribe and organize data: Transcribing interviews or focus group discussions ensures that data is readily accessible for analysis and comparison.
Organizing data ensures easier retrieval and supports efficient analysis. - Use a data coding system: Developing a coding system helps categorize and organize data, facilitating its analysis and interpretation.
A well-defined coding system enhances the consistency and transparency of data analysis. - Triangulate data: Triangulation involves using multiple data sources or methods to validate or corroborate findings, enhancing the trustworthiness of the analysis.
Triangulation strengthens the credibility and dependability of qualitative research findings.
Data Analysis Tools and Software
Advancements in technology have brought forth numerous tools and software that assist researchers in analyzing qualitative data effectively. These tools offer various features and functionalities that simplify the process, such as coding, data visualization, and collaboration. Some commonly used software tools for qualitative data analysis include:
Software | Features |
---|---|
NVivo |
|
ATLAS.ti |
|
Dedoose |
|
Choosing the appropriate software depends on the research objectives, available resources, and the complexity of the data.
Conclusion
Data analysis is a vital component of qualitative research, enabling researchers to uncover insights, patterns, and relationships within the data. By employing appropriate methods and following best practices, researchers can draw meaningful conclusions from qualitative data. Advancements in data analysis software further facilitate the process, offering researchers efficient tools for coding, visualization, and collaboration.
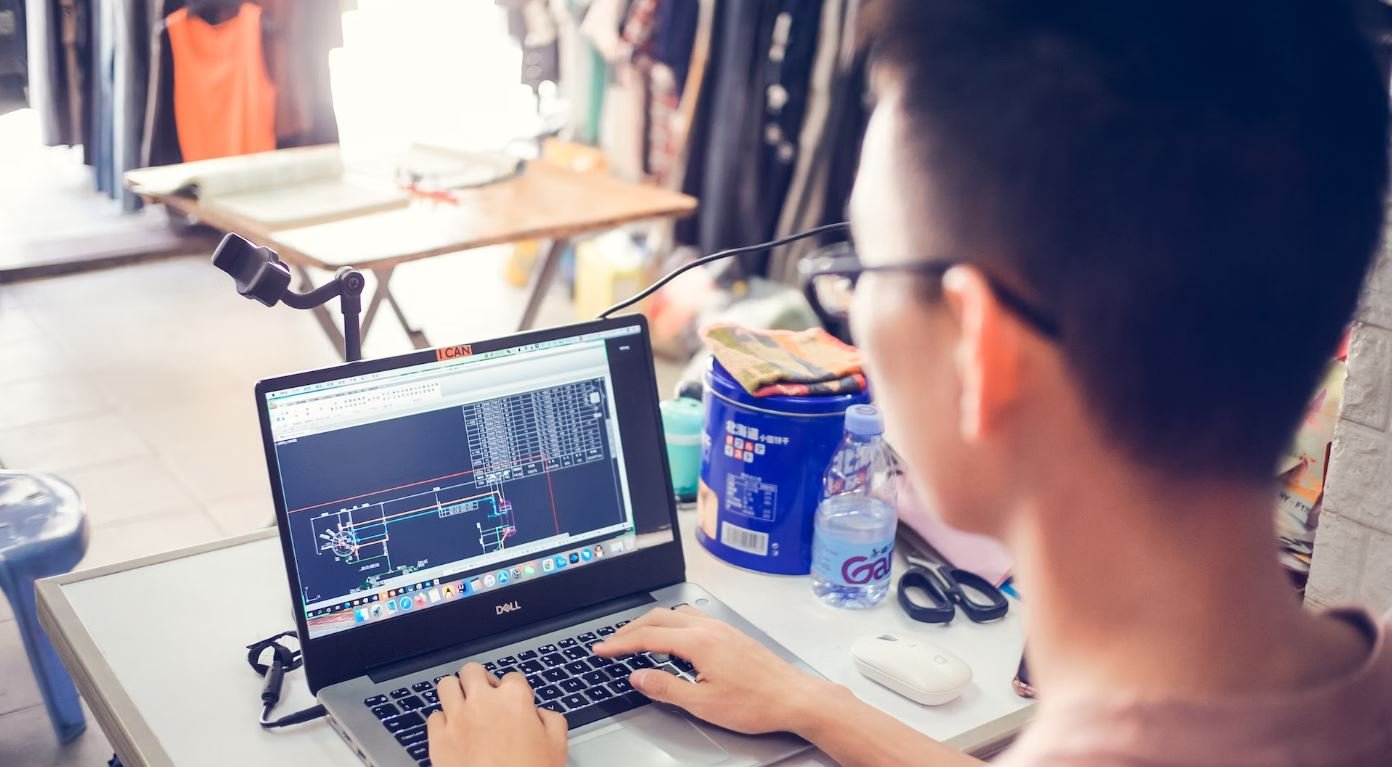
Common Misconceptions
Data Analysis in Qualitative Research
Qualitative research involves the systematic collection, interpretation, and analysis of non-numeric data such as interviews, observations, and open-ended survey responses. However, there are several common misconceptions surrounding the data analysis process in qualitative research.
- Qualitative data analysis is purely subjective and lacks reliability.
- Qualitative analysis is only used for exploratory purposes and cannot yield concrete findings.
- Qualitative research analysis is time-consuming and impractical for large-scale studies.
One misconception is that qualitative data analysis is purely subjective and lacks reliability. While qualitative research does involve interpretation and subjectivity, rigorous methods are employed to establish trustworthiness and reliability. Techniques like member checking, peer review, and maintaining an audit trail are used to enhance the validity and objectivity of the analysis.
- Qualitative researchers strive for intersubjective agreement in data interpretation.
- Some qualitative analysis methods, such as content analysis, follow systematic and transparent procedures.
- Reliability in qualitative analysis comes from the consistent application of methods and techniques.
Another misconception is that qualitative analysis is only used for exploratory purposes and cannot yield concrete findings. In reality, qualitative analysis can provide rich and detailed insights into complex phenomena. It can uncover patterns, themes, and relationships that quantitative methods may miss. Qualitative research can produce evidence-based conclusions that inform theory development and decision-making.
- Qualitative research can lead to the discovery of new theories or concepts.
- It allows for an in-depth understanding of people’s experiences and perspectives.
- Qualitative findings can be used to generate hypotheses for future quantitative studies.
A common misconception is that qualitative research analysis is time-consuming and impractical for large-scale studies. While it is true that qualitative analysis can be time-intensive because of the detailed examination of data, there are numerous software programs available that can aid in the organization, coding, and analysis of qualitative data. These tools help streamline the process and make it feasible for large amounts of qualitative data to be analyzed efficiently.
- Software programs like NVivo and ATLAS.ti facilitate data organization and analysis.
- Using software reduces the time required for manual coding and retrieval of data.
- Technology allows for the analysis of larger datasets while maintaining rigor.
In conclusion, there are common misconceptions surrounding the data analysis process in qualitative research. It is important to recognize that qualitative analysis is not entirely subjective and lacking in reliability. It can yield concrete findings and provide valuable insights. Additionally, with the aid of software tools, qualitative analysis can be time-efficient and suitable for larger studies. Understanding these misconceptions helps individuals appreciate the effectiveness and rigor of qualitative data analysis in research.
- Qualitative research analysis is not purely subjective and can be reliable.
- Qualitative analysis can yield valuable and concrete findings.
- Software tools make qualitative analysis more practical for large-scale studies.
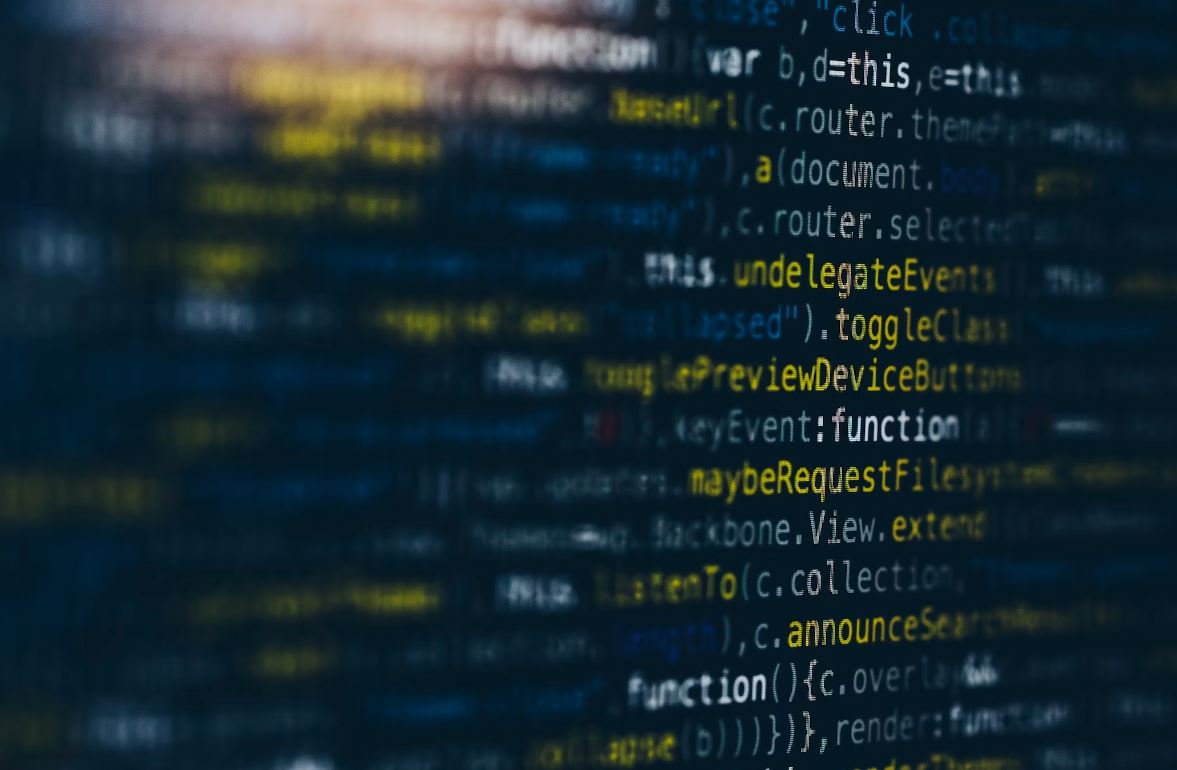
Data Analysis in Qualitative Research
Qualitative research is a method that involves collecting and analyzing data in order to gain an in-depth understanding of a particular phenomenon. Data analysis in qualitative research relies on the interpretation of non-numerical data, such as interviews, observations, and documents. In this article, we present 10 tables that illustrate different aspects of data analysis in qualitative research, highlighting the richness and unique insights that this approach can provide.
Table: Segmentation of Interview Participants by Age Group
This table showcases the distribution of interview participants according to age groups. Understanding the demographics of the participants can allow researchers to identify potential variations in experiences and perspectives.
| Age Group | Number of Participants |
|———–|———————–|
| 18-25 | 12 |
| 26-35 | 15 |
| 36-45 | 9 |
| 46-55 | 8 |
| 56+ | 6 |
Table: Main Themes Evolving from Content Analysis
This table summarizes the main themes that emerged from the content analysis of participant interviews. These themes provide insights into the different aspects and perspectives related to the research topic.
| Themes | Number of Occurrences |
|————————|———————-|
| Challenges | 43 |
| Opportunities | 26 |
| Strategies | 32 |
| Recommendations | 18 |
| Impact | 38 |
Table: Verbatim Excerpts from Observations
This table presents verbatim excerpts from the observation notes. These excerpts provide a snapshot of the participants’ behaviors, interactions, and environmental context, helping to capture the essence of the observed phenomenon.
| Excerpt | Participant | Context |
|—————————————————-|————-|—————–|
| “Participant A expressed frustration with the task” | A | Group discussion|
| “Participants B and C engaged in a lively debate” | B, C | Workshop setting|
| “Participant D seemed hesitant but eventually shared their opinion” | D | Interview room |
Table: Example Quotes Supporting Research Findings
This table presents illustrative quotes from participant interviews that support the research findings. These quotes provide real-life examples and add depth to the understanding of the phenomenon under investigation.
| Quote | Participant | Context |
|————————————————–|————-|——————–|
| “I often feel overwhelmed by the workload” | Participant A| Interview |
| “This program has opened up new opportunities for me” | Participant B| Focus group discussion|
| “I believe that clear communication is key” | Participant C| In-depth interview |
Table: Comparison of Themes Across Subgroups
This table compares the occurrence of themes across different subgroups of participants to identify similarities and differences in their perspectives. This analysis enables researchers to explore potential variations within the data.
| Themes | Subgroup 1 | Subgroup 2 |
|—————–|————|————|
| Engagement | 12 | 9 |
| Satisfaction | 8 | 10 |
| Barriers | 7 | 11 |
| Motivation | 11 | 10 |
| Collaboration | 10 | 8 |
Table: Frequency of Non-Verbal Cues in Observations
This table displays the frequency of non-verbal cues captured during observations. Non-verbal cues, such as gestures and facial expressions, can offer valuable insights into participants’ emotions and attitudes.
| Non-Verbal Cues | Frequency |
|——————-|———–|
| Nodding | 28 |
| Smiling | 16 |
| Frowning | 10 |
| Hand gesturing | 20 |
| Eye contact | 23 |
Table: Distribution of Document Types
This table illustrates the distribution of different types of documents analyzed in the research. By categorizing documents, researchers can gain a comprehensive understanding of the phenomenon from various perspectives.
| Document Type | Number of Documents |
|——————–|———————|
| Reports | 37 |
| Memos | 21 |
| Emails | 12 |
| Meeting minutes | 16 |
| Surveys | 10 |
Table: Summary of Ethnographic Field Notes
This table provides a summary of the key observations and insights from ethnographic field notes. These notes record researchers’ observations of daily activities, interactions, and cultural practices within a specific context.
| Observations | Insights |
|———————————————————————–|——————————————————–|
| Daily routine of community members revolves around agricultural activities | Cultural significance of agriculture in the community |
| High level of interpersonal cooperation and support among community members | Importance of social bonds in the community |
| Symbolic rituals and ceremonies play a central role in community cohesion | Role of traditions in strengthening community ties |
Table: Comparison of Findings from Literature Review
This table compares the findings from the literature review conducted for the research. Comparing the research findings with existing knowledge helps establish the novelty and contribution of the current study.
| Findings | Study A | Study B |
|———————————-|—————–|—————–|
| Lack of standardized procedures | Yes | No |
| Emerging themes | 7 | 5 |
| Recommendations for future research | Yes | No |
| Methodological limitations | No | Yes |
| Consistency with existing theories | Yes | Yes |
Conclusion
Data analysis in qualitative research offers a rich and insightful approach to understanding complex phenomena. The tables presented in this article highlight the diverse elements involved in data analysis, including participant demographics, emerging themes, verbatim excerpts, and comparisons across subgroups. By employing qualitative methods, researchers can uncover nuanced insights that may not be captured through quantitative analysis alone. The exploration of non-verbal cues, document analysis, and ethnographic field notes further enriches the understanding of the phenomenon under investigation. These tables demonstrate the value of data analysis in qualitative research and its ability to provide meaningful and context-specific knowledge. By embracing the interpretive nature of qualitative research, researchers can gain deeper insights into the perspectives, experiences, and nuances of the researched phenomena.
Frequently Asked Questions
What is qualitative data analysis?
Qualitative data analysis is the process of systematically organizing, interpreting, and making sense of non-numerical data, such as interview transcripts, observations, and textual documents. It involves identifying patterns, themes, and relationships to generate insights and understanding.
What are the main approaches to qualitative data analysis?
There are various approaches to qualitative data analysis, including content analysis, thematic analysis, grounded theory, and narrative analysis. Each approach has its own distinct methods and techniques for analyzing qualitative data.
How do I choose the most suitable qualitative analysis approach for my research?
Choosing the most suitable qualitative analysis approach depends on the nature of your research question, the type of data you have collected, and the theoretical framework guiding your study. It is recommended to review the characteristics and requirements of different approaches before selecting the one that aligns best with your research objectives.
What software can I use for qualitative data analysis?
There are several software options available for qualitative data analysis, such as NVivo, ATLAS.ti, MAXQDA, and Dedoose. These tools provide features for coding, organizing, and analyzing qualitative data, making the analysis process more efficient and systematic.
How do I code qualitative data?
Coding is a key step in qualitative data analysis. It involves assigning labels or tags to segments of data that represent concepts, themes, or categories. Coding can be done manually by using highlighters or index cards, or it can be facilitated through software tools specifically designed for qualitative analysis.
What is the difference between inductive and deductive coding?
Inductive coding is a bottom-up approach where codes and themes emerge directly from the data. It allows new insights and patterns to emerge during the analysis process. Deductive coding, on the other hand, is a top-down approach that uses predetermined codes or theoretical frameworks to guide the analysis. It is more hypothesis-driven and focused on testing prior theories or concepts.
How do I ensure the reliability and validity of qualitative data analysis?
To ensure reliability and validity in qualitative data analysis, it is crucial to establish clear and transparent procedures. This can include using multiple coders to independently analyze the data, conducting member checks with participants to verify interpretations, maintaining an audit trail of analytical decisions, and seeking feedback from peers or experts in the field.
What are some common challenges in qualitative data analysis?
Some common challenges in qualitative data analysis include managing large volumes of data, dealing with subjectivity and bias, interpreting multiple perspectives, maintaining reflexivity throughout the analytical process, and balancing the need for depth and breadth in analysis. It is important to acknowledge and address these challenges to enhance the rigor and credibility of qualitative research.
How do I report the findings of qualitative data analysis?
Reporting the findings of qualitative data analysis typically involves organizing and presenting the results in a clear and coherent manner. This can include writing a narrative description of key themes, providing illustrative quotes or excerpts, using data visualizations such as charts or diagrams, and linking the findings back to the research objectives and theoretical framework.
Can qualitative data analysis be used in combination with quantitative methods?
Yes, qualitative data analysis can be used in combination with quantitative methods in a research study. This is known as mixed-methods research. It allows researchers to gain a more comprehensive understanding of a phenomenon by triangulating qualitative and quantitative data, enabling a deeper exploration of complex research questions and enhancing the overall validity of the findings.