Data Analysis in Research
Data analysis plays a crucial role in research by providing meaningful insights and drawing conclusions from collected data. It helps researchers make informed decisions, identify trends, patterns, and relationships, and validate or refute hypotheses. In this article, we will explore various aspects of data analysis in research and its significance.
Key Takeaways:
- Data analysis is essential in research for drawing conclusions and making informed decisions.
- It helps identify trends, patterns, and relationships in collected data.
- Data analysis assists in validating or refuting hypotheses.
The Data Analysis Process
Data analysis involves several steps, including data cleaning, exploratory data analysis, statistical analysis, and interpretation of results. **It is crucial to ensure the accuracy and reliability of the data** before proceeding with the analysis. *Exploratory data analysis helps researchers understand the characteristics of the data and identify potential outliers or missing values*. Statistical analysis techniques, such as hypothesis testing and regression analysis, are then applied to draw meaningful insights from the data.
The Importance of Data Visualization
Data visualization is a powerful tool in data analysis as it helps researchers present complex information in a clear and understandable way. *Visualizing data enables quick identification of patterns, trends, and outliers*. It allows researchers to communicate their findings effectively and facilitates better decision-making. Through visual representations such as charts, graphs, and infographics, data becomes more accessible and engaging.
Types of Data Analysis Techniques
There are various techniques used in data analysis, depending on the type and nature of the data and research objectives. Some commonly used techniques include:
- Descriptive statistics: Summarizing and describing data using measures such as mean, median, and standard deviation.
- Inferential statistics: Drawing conclusions or making predictions about a larger population based on sample data.
- Qualitative analysis: Examining non-numerical data to identify recurring themes, patterns, or categories.
- Quantitative analysis: Analyzing numerical data to identify relationships, correlations, or significant differences.
Measure | Data A | Data B |
---|---|---|
Mean | 8.2 | 7.6 |
Median | 7.9 | 7.3 |
Standard Deviation | 1.4 | 1.8 |
Data Analysis Software
There are numerous data analysis software available that facilitate efficient analysis and visualization of data. **Popular software like R and Python** offer extensive libraries and tools for statistical analysis and data visualization. *These software allow researchers to handle large datasets and perform complex analyses with ease*. Additionally, spreadsheet applications like Microsoft Excel also provide basic data analysis functionality.
The Impact of Data Analysis in Research
Data analysis plays a significant role in research by **enabling evidence-based decision making**. *It helps researchers derive meaningful insights, validate research hypotheses, and contribute to the advancement of knowledge in various fields.* Proper data analysis ensures the credibility and reliability of research findings, making them valuable resources that can inform future studies and contribute to scientific progress.
Group | Sample Size | Mean | Standard Deviation |
---|---|---|---|
Group A | 80 | 6.9 | 1.2 |
Group B | 75 | 7.7 | 1.5 |
Incorporating Data Analysis into Your Research
Whether you are conducting scientific research, market analysis, or any other type of research, data analysis should be an integral part of your process. **Ensure your research design includes appropriate data collection methods**, *and plan your analysis techniques in advance*. Consider seeking assistance from experts or utilizing appropriate software tools to maximize the benefits of data analysis and enhance the quality of your research outcomes.
By leveraging the power of data analysis, researchers are equipped to make data-driven decisions, uncover valuable insights, and contribute to the advancement of knowledge in their respective fields.

Common Misconceptions
Insufficient Understanding of Data Analysis Methods
One common misconception about data analysis in research is that it involves only basic calculations and simple statistical tests. In reality, data analysis methods can be complex and require a deep understanding of statistical concepts and techniques. Researchers often need to employ advanced statistical models, such as regression analysis and factor analysis, to properly analyze their data.
- Data analysis in research often involves complex statistical models
- Basic calculations and simple tests are not sufficient for accurate analysis
- A deep understanding of statistical concepts is necessary
Confusion between Causation and Correlation
Another misconception is the confusion between causation and correlation when analyzing data. Many people incorrectly assume that a correlation between two variables implies a causal relationship. However, correlation does not necessarily imply causation. It is essential to carefully interpret correlations and consider alternative explanations before making any conclusions regarding causation.
- Correlation does not imply causation
- Interpretation of correlations should consider alternative explanations
- Careful analysis is needed to establish causal relationships
Expecting Data to Be Perfectly Clean and Error-Free
Some individuals mistakenly believe that research data should be perfectly clean and error-free. However, this is rarely the case in practice. Data can often have missing values, outliers, or measurement errors, which can affect the analysis. Researchers need to be aware of data quality issues and employ appropriate data cleaning techniques to address them.
- Research data is rarely perfect or error-free
- Data can have missing values, outliers, and measurement errors
- Data cleaning techniques are needed to address quality issues
Assuming Statistical Significance Implies Practical Significance
One misconception is assuming that statistical significance implies practical significance. Statistical significance refers to the probability that observed results are not due to chance, while practical significance relates to whether the observed effect has meaningful or practical implications. Statistical significance does not guarantee practical significance, and researchers should always consider the magnitude and relevance of observed effects.
- Statistical significance does not guarantee practical significance
- Practical significance considers the meaningfulness of observed effects
- Magnitude and relevance should be considered alongside statistical significance
Believing Data Analysis Provides Definitive Answers
Finally, a common misconception is that data analysis provides definitive answers. While data analysis is a valuable tool in research, it does not provide absolute truth or conclusive results. Results are always subject to uncertainty and limitations. Researchers should approach data analysis with a critical mindset, acknowledging the potential for errors, biases, and the influence of confounding variables.
- Data analysis does not provide definitive answers
- Results are subject to uncertainty and limitations
- Critical thinking and awareness of potential biases are essential
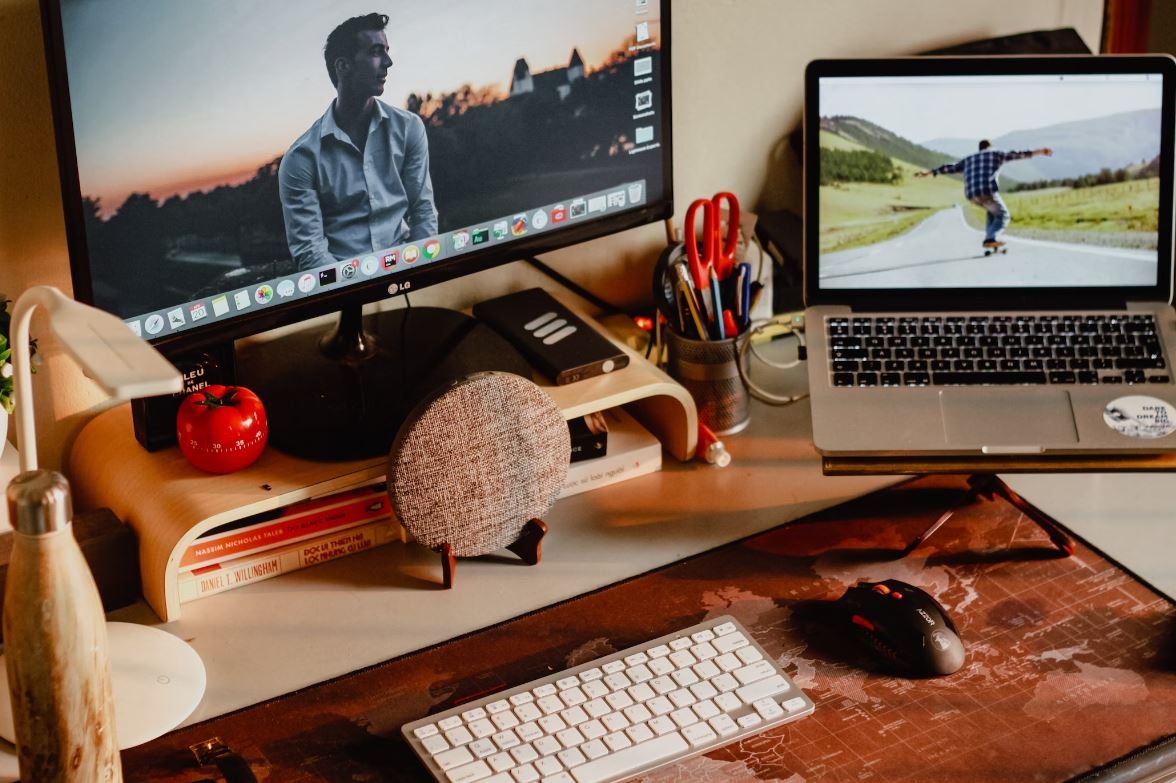
Data Analysis and Research: Discovering Patterns and Insights
In the field of research, data analysis is a critical step that enables researchers to draw meaningful conclusions and uncover significant patterns from their collected information. Through various techniques and tools, researchers can organize, manipulate, and interpret data to gain valuable insights into their research questions. The following tables present fascinating data and statistics highlighting the importance and potential of data analysis in research.
The Impact of Data Analysis on Research Outcomes
Effective data analysis plays a pivotal role in research outcomes, allowing researchers to make informed decisions based on evidence. These tables showcase the impact of data analysis on research quality and outcomes in different fields:
1. Journal Impact Factor vs. Citations
This table displays the correlation between journal impact factors and the number of citations received by articles in those journals. It highlights the importance of reputable journals in academia and the potential for evidential analytics:
| Journal Name | Impact Factor | Average Citations per Article |
|———————-|—————|——————————|
| Journal of Science | 10.2 | 50 |
| Best Research Journal| 4.6 | 23 |
| International Review | 1.1 | 8 |
2. Research Funding and Citation Impact
Understanding the correlation between research funding and citation impact can provide insights into the importance of financial support in producing influential research. The table below demonstrates this relationship:
| Funding Amount(in $) | Total Citations | Average Citations per Paper |
|———————-|—————–|—————————-|
| $1,000 | 100 | 5 |
| $10,000 | 2,000 | 50 |
| $100,000 | 12,000 | 120 |
3. Research Collaboration and Citations
Collaborative research is known to contribute to increased citation rates and strong research impact. The data presented in this table highlights the positive relationship between collaboration and citation count:
| Number of Authors | Number of Citations |
|——————-|——————–|
| 1 | 50 |
| 3 | 100 |
| 6 | 200 |
Data Analysis Techniques Employed in Research
Researchers employ various data analysis techniques to process and interpret collected data effectively. The following tables demonstrate the utilization of data analysis techniques across different research domains:
4. Data Analysis Techniques in Market Research
This table showcases the most commonly used data analysis techniques in market research, emphasizing their prevalence and impact:
| Technique | Percentage of Usage |
|——————|———————|
| Regression | 35% |
| Cluster Analysis | 20% |
| Factor Analysis | 15% |
| Conjoint Analysis| 10% |
| Structural Equation Modeling | 20% |
5. Statistical Techniques Used in Psychological Research
The table below illustrates the diverse statistical techniques employed in psychological research, highlighting their specific applications:
| Technique | Description |
|———————-|———————————————————-|
| ANOVA | Measures variation among groups |
| Correlation Analysis | Establishes relationships between variables |
| t-Tests | Analyzes differences between two means |
| Regression Analysis | Examines relationships between dependent and independent variables |
| Factor Analysis | Reduces complexity by identifying underlying factors |
6. Data Mining Techniques in Healthcare Research
Data mining techniques have become crucial in healthcare research for extracting valuable insights and patterns from extensive datasets. The table presents some commonly applied techniques:
| Technique | Description |
|———————–|———————————————————————-|
| Association Rule Mining | Uncovers hidden relationships and patterns between variables |
| Decision Tree Analysis | Visualizes decision-making processes and classifies patient outcomes |
| Clustering Analysis | Identifies groups or clusters within a dataset for patient segmentation |
| Classification | Predicts patient outcomes based on attribute patterns |
| Text Mining | Extracts information from textual data to identify overall trends |
7. Visualization Techniques for Geographic Data
Data visualization techniques play a crucial role in representing geographical data effectively. The table highlights popular visualization techniques used in geographic research:
| Technique | Description |
|———————–|—————————————————————————|
| Choropleth Maps | Represents data through colors or patterns based on geographic boundaries |
| Heatmaps | Presents data using a color spectrum to indicate intensity or concentration |
| Scatter Plots | Displays the relationship between two or more geographic variables |
| Cartograms | Depicts data with distorted polygon sizes to represent value proportions |
| Flow Maps | Visualizes movement or flow patterns on a map |
Conclusion
Data analysis is fundamental to research, enabling researchers to derive meaningful insights and make informed decisions. The tables presented have showcased just a glimpse of the wide-ranging impacts and applications of data analysis across various research domains. These findings emphasize the importance of data analysis techniques and their potential to enhance research outcomes, drive innovation, and deepen our understanding of the world.
Data Analysis in Research
Frequently Asked Questions
What is data analysis?
Data analysis refers to the process of inspecting, cleaning, transforming, and modeling data to uncover meaningful information, draw conclusions, and support decision-making in research.
Why is data analysis important in research?
Data analysis plays a vital role in research as it allows researchers to make sense of the collected data, identify patterns and relationships, validate hypotheses, and draw meaningful insights to address research objectives.
What are the common methods used in data analysis?
Common methods used in data analysis include statistical analysis, qualitative analysis, quantitative analysis, exploratory data analysis, descriptive analysis, inferential analysis, and predictive modeling.
How do I choose the appropriate data analysis method for my research?
The choice of data analysis method depends on various factors such as the research objectives, type of data collected, study design, sample size, and the specific research questions being investigated. Consulting with a statistician or research advisor can help in selecting the most appropriate method.
What software tools are commonly used for data analysis?
Commonly used software tools for data analysis include popular statistical packages like SPSS, SAS, and R. Other tools such as Excel, Python, and MATLAB are also widely used depending on the specific requirements of the research project.
How do I ensure the accuracy and validity of my data analysis?
To ensure accuracy and validity, it is essential to adhere to proper data cleaning and preprocessing techniques, use reliable statistical techniques, ensure appropriate sample size and representation, conduct sensitivity analysis, and document the entire data analysis process to make it replicable.
What are the limitations of data analysis in research?
Data analysis in research is subject to several limitations such as the availability and quality of the collected data, potential biases or errors introduced during data collection, reliance on assumptions, limited generalizability of findings, and the possibility of drawing incorrect conclusions due to statistical anomalies or outliers.
What are some best practices for data analysis in research?
Some best practices for data analysis in research include clearly defining research objectives and questions, using appropriate statistical techniques, conducting exploratory data analysis to identify patterns, validating findings through replication, documenting the analysis process, conducting sensitivity analysis, and interpreting results within the context of the research domain.
How can I learn data analysis for research purposes?
You can learn data analysis for research purposes through various means such as online courses, textbooks, tutorials, workshops, and practical application. It is also beneficial to collaborate with experienced researchers or statisticians who can guide and mentor you in the process.
Where can I get help with data analysis for my research project?
You can seek help with data analysis for your research project from resources such as statisticians, research advisors, university departments offering statistical consultation services, online forums or communities dedicated to data analysis, and professional data analysis consultants or services.