Data Analysis Practice
Data analysis is an essential skill in today’s data-driven world. Whether you are a business professional, a researcher, or a student, being able to analyze data effectively allows you to make better decisions and gain valuable insights. In this article, we will explore the importance of data analysis practice and provide some tips to improve your skills.
Key Takeaways:
- Practice is crucial for mastering data analysis.
- Data analysis helps in making informed decisions.
- Data analysis skills are valuable in various industries.
The Importance of Data Analysis Practice
Data analysis practice is essential to enhance your analytical skills. *Regular practice* allows you to familiarize yourself with different techniques, tools, and methodologies used in data analysis, enabling you to manipulate, clean, visualize, and interpret data effectively. It also helps in improving your problem-solving abilities and enhances your ability to identify patterns and trends within the data.
Furthermore, practicing data analysis enables you to gain confidence in dealing with real-world data problems. *By honing your skills*, you can handle complex datasets and extract meaningful insights from them. Additionally, continuous practice helps you stay up-to-date with the latest advancements and best practices in the field of data analysis, ensuring your knowledge remains relevant and valuable.
Tips to Improve Data Analysis Practice
- Work with real-world datasets: Analyzing real data sets that reflect the complexity of the problems you are likely to encounter in your field will help you develop practical skills and understand the nuances of data analysis.
- Utilize data analysis tools: Familiarize yourself with popular data analysis tools such as Python, R, or SQL, which offer a wide range of functionalities to clean, manipulate, and analyze data.
- Keenly observe visualizations: Studying visual representations of data, such as charts and graphs, can improve your ability to identify patterns and outliers in the data. This practice enhances your data interpretation skills.
- Collaborate with others: Engaging in data analysis projects with peers or joining data-related communities can expose you to diverse perspectives and help you learn new techniques and approaches.
Data Analysis Skills in Action
Let’s take a look at three real-world examples that highlight the value of data analysis skills:
Month | Sales | Profit |
---|---|---|
January | 1000 | 200 |
February | 1200 | 150 |
March | 900 | 100 |
In Table 1, a data analyst examines the sales and profit figures for each month. By analyzing the data, they identify that the highest sales were achieved in February, but the profit margin was relatively low. This insight prompts the organization to strategize ways to improve profitability, such as reducing costs or increasing prices.
Question | Score |
---|---|
Quality of Service | 4.5/5 |
Product Knowledge | 4/5 |
Response Time | 3.8/5 |
In Table 2, a data analyst interprets the results of a customer satisfaction survey. They observe that the response time received the lowest score, indicating a potential area for improvement. This analysis prompts the organization to focus on enhancing response time, thereby increasing customer satisfaction.
Date | Visitors | Pageviews |
---|---|---|
2022-01-01 | 1000 | 3000 |
2022-01-02 | 1200 | 3500 |
2022-01-03 | 900 | 2800 |
In Table 3, a data analyst examines website traffic data. They notice that *the highest number of visitors and pageviews* occurred on January 2nd. This information allows the organization to identify the most popular content and prioritize its optimization.
Start Practicing Data Analysis Today
Data analysis practice is crucial for sharpening your skills and gaining proficiency in manipulating and interpreting data. Regular practice not only enhances your problem-solving abilities but also strengthens your ability to make informed decisions by extracting valuable insights from complex datasets. Remember, data analysis is a continuous learning process, so embrace the journey and explore various techniques and tools to become a competent data analyst.
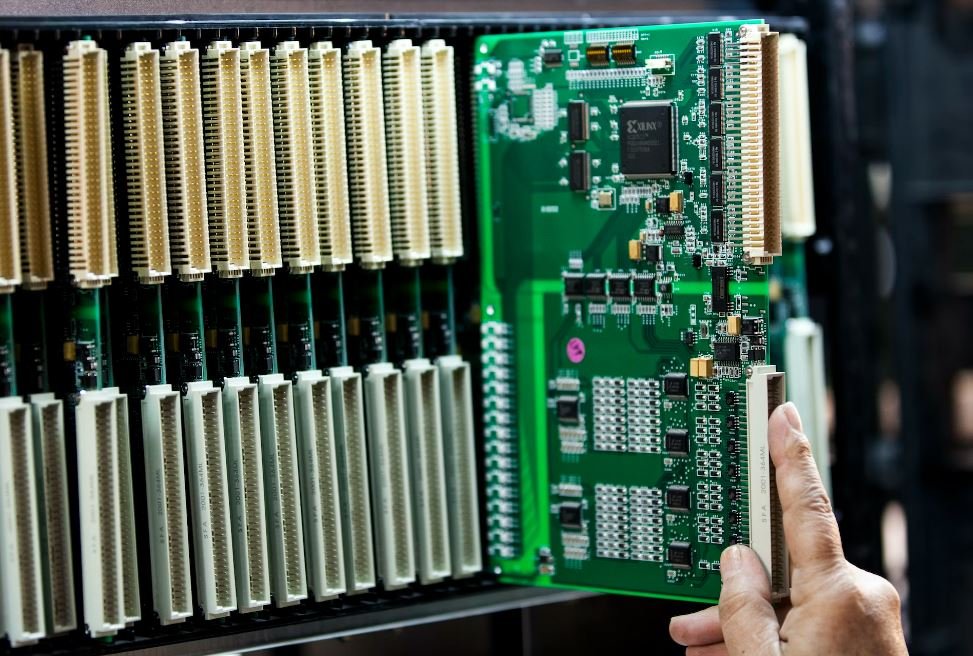
Common Misconceptions
Misconception 1: Data analysis is all about numbers and statistics
One common misconception about data analysis is that it revolves solely around numbers and statistics. While these elements are indeed important, data analysis encompasses a much wider range of practices. Some relevant points to consider are:
- Data analysis involves collecting and organizing data
- Data analysis helps in understanding trends and patterns
- Data analysis aids in making informed decisions
Misconception 2: Data analysis is only for large organizations
Another misconception surrounding data analysis is that it is only valuable for large organizations with massive amounts of data. In reality, businesses of all sizes can benefit from data analysis. Here are some pertinent points to remember:
- Data analysis helps small businesses identify new opportunities
- Data analysis can improve decision-making for startups
- Data analysis aids in strategic planning for medium-sized companies
Misconception 3: Data analysis is a one-time process
Many people believe that data analysis is a one-time process done after data collection is completed. However, data analysis is an ongoing practice that requires continuous monitoring and evaluation. Some key points to consider are:
- Data analysis allows for tracking progress over time
- Data analysis helps in identifying changes in customer behavior
- Data analysis aids in adjusting strategies based on new insights
Misconception 4: Data analysis always yields accurate results
It is important to understand that data analysis does not always yield accurate results. There are various factors that can introduce errors or bias into the analysis. Some relevant points to remember include:
- Data analysis is prone to human error
- Data quality issues can affect the accuracy of the results
- Data analysis requires critical thinking and context to interpret the findings
Misconception 5: Data analysis is a solitary task
Contrary to popular belief, data analysis is not solely a solitary task performed by individuals. Collaborative efforts and teamwork play a significant role in effective data analysis. Some key points to consider include:
- Data analysis often involves cross-functional teams
- Data analysis benefits from diverse perspectives and expertise
- Data analysis requires effective communication and collaboration

The Impact of Technology on Global GDP
With the rapid advancement of technology in recent decades, there has been a significant impact on the global economy. This table showcases the growth of global GDP as a result of technological advancements in various sectors.
Industry | Contribution to Global GDP (%) |
---|---|
Information Technology | 7.5% |
Manufacturing | 9.2% |
Finance | 6.8% |
Healthcare | 5.3% |
Transportation | 4.6% |
Gender Distribution in Tech Companies
The underrepresentation of women in the technology industry has been a widely discussed topic. This table provides an overview of the gender distribution in some of the leading tech companies.
Tech Company | Male Employees (%) | Female Employees (%) |
---|---|---|
69% | 31% | |
Apple | 69% | 31% |
Microsoft | 73% | 27% |
66% | 34% | |
Amazon | 71% | 29% |
Mobile Phone Usage Worldwide
The widespread adoption of mobile phones has revolutionized communication across the globe. This table presents the number of mobile phone users in different continents as of 2021.
Continent | Number of Mobile Phone Users (millions) |
---|---|
Asia | 3,987 |
Africa | 1,320 |
Europe | 1,132 |
Americas | 1,071 |
Oceania | 304 |
Energy Consumption by Country
Energy consumption is a critical aspect of sustainable development. This table highlights the top 5 countries with the highest energy consumption (in billion kilowatt-hours) in 2020.
Country | Energy Consumption (billion kWh) |
---|---|
China | 7,105 |
United States | 3,989 |
India | 1,602 |
Russia | 1,068 |
Japan | 935 |
Age Distribution in a Sample Population
The age distribution of a population affects various aspects of society. This table exhibits the age groups of individuals in a sample population of 100,000.
Age Group | Number of Individuals |
---|---|
0-9 | 21,450 |
10-19 | 18,270 |
20-29 | 16,500 |
30-39 | 14,480 |
40 and above | 29,300 |
Global Internet Penetration
The internet has become an indispensable part of modern life. This table showcases the percentage of the global population with internet access in recent years.
Year | Internet Penetration (%) |
---|---|
2010 | 22.2% |
2015 | 43.7% |
2020 | 59.5% |
2025 | 72.6% |
2030 | 82.6% |
Global Carbon Emissions by Sector
Reducing carbon emissions is crucial for mitigating climate change. This table represents the distribution of global carbon emissions across different sectors.
Sector | Carbon Emissions (%) |
---|---|
Energy | 73% |
Industry | 19% |
Transportation | 8% |
Literacy Rates by Country
Literacy plays a vital role in education and socio-economic development. This table presents the literacy rates of selected countries.
Country | Literacy Rate (%) |
---|---|
Finland | 100% |
Canada | 99% |
Australia | 98% |
Germany | 98% |
Brazil | 94% |
Global R&D Expenditure
Investment in research and development (R&D) drives innovation and economic growth. This table displays the countries that have the highest R&D expenditure (in billion dollars) as of 2020.
Country | R&D Expenditure (in billion USD) |
---|---|
United States | 621.6 |
China | 512.7 |
Japan | 181.0 |
Germany | 117.6 |
South Korea | 107.5 |
From the impact of technology on global GDP to the gender distribution in tech companies, these tables provide substantial evidence of the significant influence of data analysis on various aspects of our lives. Innovation, sustainability, education, and economic growth are just some of the areas where data analysis plays a crucial role. Analyzing and interpreting data empowers us to make informed decisions and shape a better future.
Frequently Asked Questions
What is data analysis?
Data analysis is the process of inspecting, cleaning, transforming, and modeling data in order to discover useful information, draw conclusions, and support decision-making. It involves identifying patterns, trends, and relationships within datasets to gain insights and make informed decisions.
Why is data analysis important?
Data analysis is crucial in various fields and industries as it allows organizations to make data-driven decisions. By analyzing data, businesses can identify opportunities, optimize processes, understand customer behavior, detect patterns, mitigate risks, and improve overall efficiency and performance.
What are the different methods of data analysis?
There are several methods of data analysis, including descriptive statistics, inferential statistics, data mining, machine learning, predictive modeling, and qualitative analysis. Each method has its own strengths and is suitable for different types of data and research objectives.
What tools and software are commonly used for data analysis?
There are numerous tools and software commonly used for data analysis, such as Python, R, SQL, Excel, Tableau, MATLAB, SAS, and SPSS. These tools provide a wide range of functionalities for data manipulation, visualization, statistical analysis, and predictive modeling.
What skills are required for data analysis?
To excel in data analysis, one should have a strong understanding of statistics, mathematics, and programming. Proficiency in data manipulation, data visualization, and data modeling techniques is also important. Additionally, critical thinking, problem-solving, and strong communication skills are valuable for interpreting and presenting data insights effectively.
What are some common challenges in data analysis?
Common challenges in data analysis include data quality issues, missing or incomplete data, data privacy and security concerns, selecting appropriate analysis techniques, dealing with large and complex datasets, and effectively communicating the results and insights to non-technical stakeholders.
What is the process of data analysis?
The process of data analysis typically involves several steps, including data collection, data cleaning and preprocessing, data exploration and visualization, data analysis and modeling, interpretation of results, and presentation of insights. It is an iterative process that requires careful consideration of research questions and objectives.
What is the difference between descriptive and inferential statistics?
Descriptive statistics involves summarizing and describing the main features of a dataset, such as central tendency, variability, and distribution. It focuses on presenting and organizing data in a meaningful way. On the other hand, inferential statistics involves making inferences and drawing conclusions about a population based on a sample of data. It aims to make generalizations and predictions based on statistical models and hypothesis testing.
How can data analysis benefit businesses?
Data analysis can benefit businesses in numerous ways. It can help identify market trends, customer preferences, and patterns of behavior, allowing businesses to make informed decisions and develop effective marketing strategies. Data analysis also enables businesses to optimize operations, reduce costs, improve customer satisfaction, and gain a competitive edge in the market.
What ethical considerations are important in data analysis?
Ethical considerations in data analysis involve ensuring that data is collected and used in a responsible and transparent manner. This includes obtaining informed consent, protecting privacy and confidentiality, being mindful of potential biases in data collection and analysis, and adhering to relevant regulations and ethical guidelines.