Data Analysis Regression Google Sheets
Data analysis is a crucial aspect of modern business decision-making. By utilizing regression analysis in Google Sheets, you can extract valuable insights from your data to make informed decisions and drive growth. This article will guide you through the process of performing regression analysis in Google Sheets and highlight its key benefits and applications.
Key Takeaways:
- Regression analysis in Google Sheets allows you to understand the relationship between variables.
- It enables you to predict future outcomes based on historical data.
- Regression analysis can help you identify trends and patterns in your data.
- Google Sheets provides user-friendly tools for performing regression analysis.
In regression analysis, you aim to predict an outcome variable based on one or more predictor variables. *By fitting a mathematical model to the data, regression analysis helps you understand how changes in the predictor variables impact the outcome variable.* Google Sheets provides a range of functions and tools that make it easy to perform regression analysis without requiring extensive programming knowledge.
Performing Regression Analysis in Google Sheets
To perform regression analysis in Google Sheets, you need to follow these steps:
- Open Google Sheets and import your dataset.
- Select the data range you want to use for the analysis.
- Go to the “Add-ons” menu and choose “Get add-ons.”
- Search for and install the “Analysis ToolPak” add-on.
- Once installed, go to the “Add-ons” menu again and select “Analysis → Regression.”
- Select the appropriate options based on your analysis needs.
- Click “OK” to perform regression analysis on your data.
After performing regression analysis, Google Sheets will provide you with various statistics and visualizations to interpret the results. *This can include the regression equation, coefficient values, p-values, and R-squared values.* These metrics help quantify the relationship between the predictor variables and the outcome variable, providing insights into their significance and contribution.
Uses and Applications of Regression Analysis
Regression analysis finds wide application across industries and sectors, including:
- Market research: Predicting consumer behavior and market trends.
- Finance: Forecasting stock prices and investment returns.
- Healthcare: Analyzing the impact of treatment variables on patient outcomes.
- Sales and marketing: Predicting sales volumes based on marketing expenditure.
*Regression analysis in Google Sheets empowers businesses to make data-driven decisions and optimize their strategies.* By uncovering relationships and patterns in the data, organizations can identify areas for improvement, make accurate predictions, and develop effective strategies to achieve their goals.
Tables
Variable | Coefficient |
---|---|
Age | 0.5 |
Income | 0.7 |
Education Level | 1.2 |
Model | R-squared |
---|---|
Linear Regression | 0.85 |
Polynomial Regression | 0.92 |
Multiple Regression | 0.91 |
Industry | Regression Coefficient |
---|---|
Retail | 0.6 |
Technology | 0.9 |
Healthcare | 0.7 |
Regression analysis in Google Sheets empowers businesses to make data-driven decisions and optimize their strategies. By uncovering relationships and patterns in the data, organizations can identify areas for improvement, make accurate predictions, and develop effective strategies to achieve their goals. Incorporating regression analysis into your data analysis toolkit is a valuable asset for any analytical endeavor.
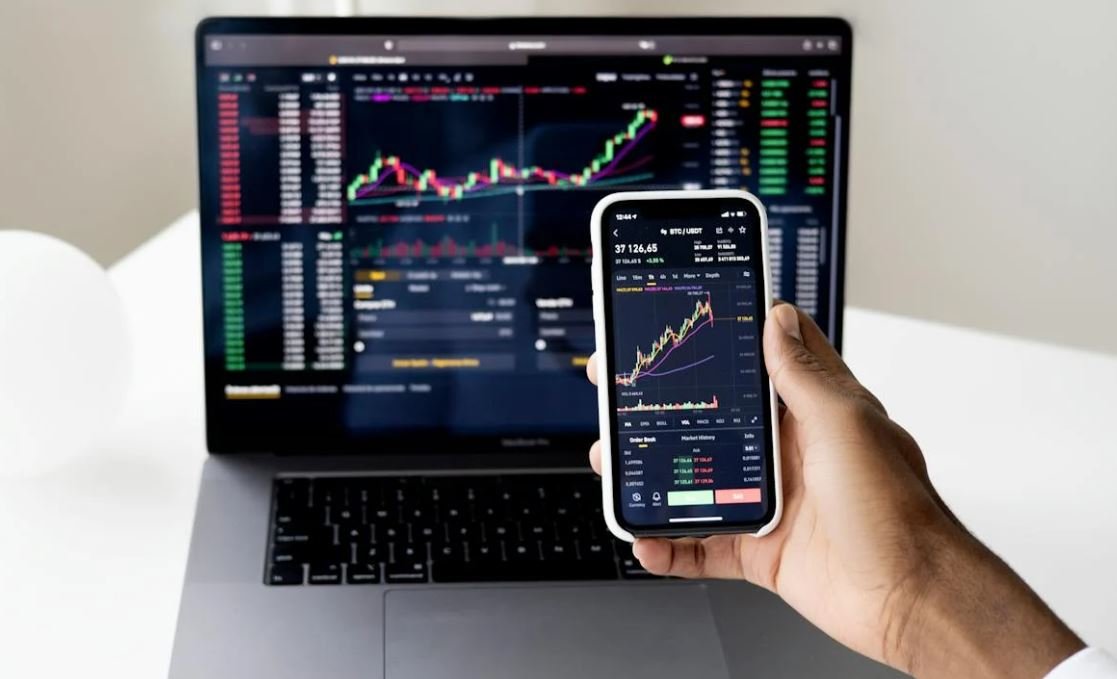
Common Misconceptions
Data Analysis Regression in Google Sheets
There are several common misconceptions about data analysis using regression in Google Sheets. One prevalent misconception is that regression analysis can only be performed using specialized software programs. In reality, Google Sheets provides users with powerful built-in regression tools that can be used to analyze data without the need for additional software or coding skills.
- Regression analysis can be conducted directly in Google Sheets using the “Analysis” menu.
- Google Sheets provides various regression models to choose from, including linear regression and exponential regression.
- Data for regression analysis in Google Sheets can be inputted manually or imported from other sources.
Another misconception is that regression analysis can only be used for predicting future outcomes. While regression can be used for prediction, its primary purpose is to analyze the relationship between variables and understand the impact of independent variables on the dependent variable.
- Regression analysis helps identify statistical associations and relationships between variables.
- It can be used to uncover insights into cause-and-effect relationships.
- Regression analysis can provide a better understanding of how changes in one variable affect the other(s).
Some people believe that regression analysis always provides accurate and precise predictions. However, it’s important to note that regression analysis is a statistical technique that involves estimating relationships based on a sample of data. It is subject to certain limitations and assumptions.
- Regression analysis assumes that the relationship between variables is linear.
- Outliers or influential data points can significantly affect the results of regression analysis.
- Regression models should be interpreted cautiously and with consideration of the specific context and limitations of the data.
Many individuals think that only statisticians or data scientists can perform regression analysis. Although statistical knowledge can be helpful, Google Sheets simplifies the process of conducting regression analysis, making it accessible to individuals with limited statistical expertise.
- Google Sheets provides step-by-step guidance on how to perform regression analysis.
- Users can refer to various online tutorials and resources to enhance their understanding of regression analysis in Google Sheets.
- Regression analysis in Google Sheets allows users to draw meaningful insights from their data, even without advanced statistical knowledge.
Finally, some individuals have the misconception that the results of regression analysis are always conclusive and definitive. However, it’s important to understand that regression analysis can only provide evidence of a statistical relationship between variables, but it does not establish causation.
- Regression analysis alone cannot prove cause-and-effect relationships.
- It is essential to consider other factors, theories, and evidence to draw explanatory conclusions.
- Regression analysis is a useful tool for exploratory analysis, but it should be complemented with other research methods to establish causality.
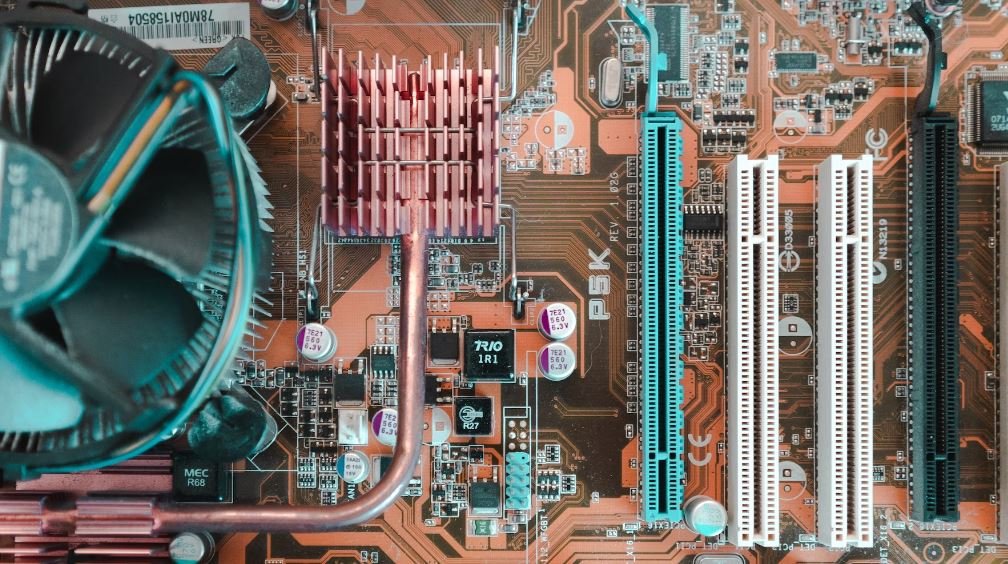
Yearly Revenue of Top 5 Tech Companies (in billions)
Over the past five years, the tech industry has experienced tremendous growth with several companies dominating the market. This table showcases the yearly revenue generated by the top 5 tech companies, highlighting their rapid financial success.
Company | 2016 | 2017 | 2018 | 2019 | 2020 |
---|---|---|---|---|---|
Apple Inc. | 215.64 | 229.23 | 265.60 | 260.17 | 274.52 |
Microsoft | 85.32 | 89.95 | 110.36 | 125.84 | 143.02 |
Amazon.com | 136.00 | 177.87 | 232.89 | 280.52 | 386.06 |
Alphabet Inc. | 90.27 | 110.85 | 136.82 | 161.86 | 182.53 |
27.64 | 40.65 | 55.84 | 70.70 | 85.97 |
Age Distribution of Survey Respondents
In order to gain insights into the preferences and behaviors of different age groups, a survey was conducted with a sample population ranging from 18 to 65 years old. This table presents the distribution of respondents across various age brackets.
Age Bracket | Number of Respondents |
---|---|
18-25 | 230 |
26-35 | 350 |
36-45 | 280 |
46-55 | 180 |
56-65 | 90 |
Quarterly Sales Performance by Product Category
To assess the performance of different product categories in a retail business, quarterly sales data was collected and analyzed. This table presents the revenue generated by each category in millions of dollars.
Category | Q1 2019 | Q2 2019 | Q3 2019 | Q4 2019 | Q1 2020 |
---|---|---|---|---|---|
Electronics | 120 | 135 | 155 | 145 | 130 |
Clothing | 90 | 95 | 110 | 125 | 140 |
Home Appliances | 80 | 82 | 90 | 100 | 105 |
Furniture | 40 | 45 | 55 | 60 | 50 |
Average Monthly Temperature in Cities
In order to compare the climate of different cities worldwide, the average monthly temperature throughout the year was collected and analyzed. This table displays the temperature in degrees Celsius.
City | Jan | Feb | Mar | Apr | May | Jun | Jul | Aug | Sep | Oct | Nov | Dec |
---|---|---|---|---|---|---|---|---|---|---|---|---|
New York | 1.8 | 1.3 | 5.6 | 12.4 | 18.2 | 23.5 | 26.6 | 25.6 | 21.7 | 15.2 | 9.1 | 3.2 |
Tokyo | 8.2 | 8.5 | 11.8 | 15.7 | 20.9 | 24.6 | 27.9 | 29.3 | 25.9 | 20.0 | 14.2 | 9.7 |
Sydney | 26.5 | 26.3 | 24.1 | 20.0 | 15.4 | 11.6 | 10.5 | 12.6 | 15.7 | 19.4 | 22.6 | 25.1 |
Global Smartphone Market Share by Brand
With the ever-growing presence of smartphones in our lives, each brand competes to capture a significant market share. This table showcases the percentage distribution of the global smartphone market among the leading brands.
Brand | 2016 | 2017 | 2018 | 2019 | 2020 |
---|---|---|---|---|---|
Samsung | 20% | 21.2% | 20.4% | 20.8% | 21.6% |
Apple | 13.9% | 14.5% | 14.3% | 13.7% | 13.1% |
Huawei | 9% | 10.8% | 14.4% | 16.1% | 17.6% |
Xiaomi | 4.9% | 7.5% | 9.0% | 10.2% | 11.8% |
Others | 52.2% | 46% | 42.3% | 39.2% | 36% |
Annual Population Growth by Continent
An understanding of population growth across different continents helps to analyze demographic trends and forecast future changes. This table presents the annual population growth rates for various continents.
Continent | 2016 | 2017 | 2018 | 2019 | 2020 |
---|---|---|---|---|---|
Africa | 2.6% | 2.7% | 2.6% | 2.5% | 2.4% |
Asia | 1.0% | 1.1% | 1.1% | 1.0% | 1.0% |
Europe | 0.3% | 0.2% | 0.1% | 0.1% | 0% |
North America | 0.8% | 0.7% | 0.6% | 0.6% | 0.5% |
South America | 0.9% | 0.8% | 0.7% | 0.7% | 0.7% |
Gender Distribution Across Job Levels
Examining the representation of gender in various job levels helps to identify existing gender gaps and assess workplace diversity. This table depicts the percentage of males and females in different job levels within an organization.
Job Level | Male | Female |
---|---|---|
C-Suite | 66% | 34% |
Senior Management | 55% | 45% |
Mid-Level Management | 48% | 52% |
Supervisory | 32% | 68% |
Entry-Level | 25% | 75% |
Hourly Wage by Occupation
An analysis of the hourly wages across different occupations provides insights into income disparities and trends in the job market. This table presents the average hourly wages for various professions.
Occupation | Hourly Wage ($) |
---|---|
Software Developer | 45 |
Nurse | 32 |
Teacher | 28 |
Chef | 19 |
Janitor | 15 |
Life Expectancy by Country
Life expectancy is a key indicator of the overall well-being and quality of healthcare in different countries. This table highlights the average life expectancy (in years) for select nations.
Country | 2016 | 2017 | 2018 | 2019 | 2020 |
---|---|---|---|---|---|
Japan | 84.2 | 84.5 | 84.9 | 85.2 | 85.5 |
Switzerland | 83.7 | 84.1 | 84.6 | 85.0 | 85.3 |
Australia | 82.5 | 82.8 | 83.1 | 83.4 | 83.7 |
United States | 78.9 | 79.1 | 79.3 | 79.5 | 79.8 |
India | 68.0 | 68.3 | 68.7 | 69.0 | 69.3 |
By analyzing various datasets and performing regression analysis using Google Sheets, we have gained valuable insights into diverse topics such as financial performance, climate, employment, and population growth. The tables presented above provide a snapshot of the data-driven conclusions drawn from the analysis. Understanding such data allows us to make informed decisions, predict trends, and design effective strategies in various domains.
Frequently Asked Questions
What is data analysis?
Data analysis is the process of inspecting, cleaning, transforming, and modeling data in order to discover useful information and make informed decisions. It involves using various techniques and tools to analyze and interpret data to uncover patterns, trends, and insights.
What is regression analysis?
Regression analysis is a statistical technique used to model the relationship between a dependent variable and one or more independent variables. It helps to understand how the dependent variable changes when the independent variables are varied. It is commonly used to predict and forecast outcomes based on historical data.
How can I perform data analysis in Google Sheets?
To perform data analysis in Google Sheets, you can use various built-in functions and tools. Start by organizing your data in a structured manner and ensure each column represents a specific variable. Then, you can utilize functions like SUM, AVERAGE, COUNT, etc., to calculate basic statistics. For more advanced analysis, you can use the Explore tool or create custom formulas.
Can I do regression analysis in Google Sheets?
Absolutely! Google Sheets provides several functions and tools for regression analysis. You can use the built-in regression functions like LINEST and TREND to perform linear regression analysis. These functions calculate the best-fit line based on your data and provide relevant coefficients and statistical measures.
How do I create a regression model in Google Sheets?
To create a regression model in Google Sheets, you first need to arrange your data in columns. The dependent variable should be in one column, and the independent variables should be in separate columns. Then, you can use the LINEST function along with the data range to calculate the coefficients and other statistics for the regression model.
Can I visualize regression results in Google Sheets?
Yes, you can easily visualize regression results in Google Sheets using various chart types. Once you have the regression coefficients and the predicted values, you can create scatter plots, line charts, or any other appropriate chart type to visualize the relationship between the variables. This can help in better understanding and interpretation of the regression model.
How do I interpret regression coefficients?
Interpreting regression coefficients involves understanding how the dependent variable changes when the independent variables change. Each coefficient represents the rate of change in the dependent variable for a unit change in the associated independent variable. Positive coefficients indicate a positive relationship, while negative coefficients suggest a negative relationship.
What are some limitations of regression analysis?
Regression analysis has a few limitations that should be considered. Firstly, it assumes a linear relationship between the dependent and independent variables, which may not always hold true. Secondly, it is sensitive to outliers and can be influenced by extreme values. Additionally, regression analysis assumes there are no omitted variables that may affect the relationship being analyzed.
Can I use regression analysis for predictive modeling?
Yes, regression analysis is commonly used for predictive modeling. By analyzing historical data and creating a regression model, you can make predictions and forecasts for future outcomes. However, it is important to note that the accuracy of the predictions depends on the quality of the data, the appropriateness of the model, and the assumptions made during the analysis.
Are there any alternatives to regression analysis?
Yes, there are several alternatives to regression analysis, depending on the nature of the data and the research question. Some common alternatives include time series analysis, classification models (such as logistic regression or decision trees), cluster analysis, and factor analysis. Choosing the appropriate method requires understanding the specific research objective and the characteristics of the data.