Data Analysis Statistics
Whether you work in business, marketing, or research, data analysis statistics are crucial for making informed decisions and driving meaningful insights. By employing statistical techniques, you can uncover patterns, trends, and correlations within your data set, enabling you to optimize processes, identify areas of improvement, and make data-driven decisions. In this article, we will explore the key concepts and techniques of data analysis statistics.
Key Takeaways:
- Understanding data analysis statistics is essential for informed decision-making.
- Statistical techniques enable the identification of patterns, trends, and correlations.
- Data-driven decisions lead to process optimization and improved outcomes.
The Importance of Data Analysis Statistics
Data analysis statistics provide valuable insights by using mathematical tools to analyze data sets. These techniques help in understanding complex relationships, making predictions, and drawing meaningful conclusions. *Statistical analysis is the backbone of many research studies, allowing researchers to draw valid inferences from their data.* By applying statistical methods, organizations can make informed decisions to drive growth, increase efficiency, and gain a competitive advantage in their respective industries.
One of the primary goals of data analysis statistics is to summarize and describe data in meaningful ways. *Through measures like mean, median, and mode, statisticians can obtain a better understanding of the overall characteristics of a data set.* Additionally, graphical representations such as histograms and box plots allow for visualizing data distributions and identifying outliers or anomalies.
Common Techniques in Data Analysis Statistics
Data analysis statistics employ a range of techniques to analyze data and derive meaningful insights. These techniques include:
- Hypothesis Testing: This method involves determining if there is enough evidence to support or reject a stated hypothesis based on sample data. It helps in drawing conclusions about the population based on the collected sample.
- Regression Analysis: Regression analysis explores the relationship between a dependent variable and one or more independent variables. It helps in understanding how changes in one variable impact another.
- Correlation Analysis: This technique measures the strength and direction of the relationship between two or more variables. It helps identify patterns or dependencies between variables.
- ANOVA (Analysis of Variance): ANOVA compares means between two or more groups to determine if there are statistically significant differences. It is useful in comparing performance or outcomes across different categories or populations.
Data Analysis Statistics Tables
Table 1: Example of Hypothesis Testing Results | |||
---|---|---|---|
Data Group | Mean | Standard Deviation | p-value |
Group A | 25.6 | 4.3 | 0.032 |
Group B | 20.9 | 3.8 | 0.087 |
*The p-value indicates the level of statistical significance in hypothesis testing. A p-value less than 0.05 is typically considered statistically significant.*
Table 2: Regression Analysis Results | ||
---|---|---|
Independent Variable | Coefficient | p-value |
Variable A | 0.72 | <0.001 |
Variable B | 1.05 | <0.001 |
*The p-value less than 0.001 indicates strong evidence to reject the null hypothesis and accept the relationship between the independent and dependent variables.*
Real-world Applications of Data Analysis Statistics
Data analysis statistics find extensive applications across various fields:
- In healthcare, statistics help analyze the effectiveness of treatments, evaluate patient outcomes, and identify risk factors.
- In finance, statistical models assist in predicting market trends, analyzing investment portfolios, and calculating risk.
- In marketing, data analysis allows for customer segmentation, campaign optimization, and market research.
Table 3: Market Research Survey Results | ||
---|---|---|
Question | Response | Percentage |
Agree | 65 | 45% |
Neutral | 30 | 20% |
Disagree | 45 | 35% |
*The survey results indicate that 45% of respondents agree with the statement, while 35% disagree.*
The power of data analysis statistics
In today’s data-driven world, data analysis statistics play a vital role in helping organizations make well-informed decisions, optimize processes, and gain a competitive edge. By employing statistical techniques, businesses can leverage data to drive growth, improve customer satisfaction, and streamline operations. Start harnessing the power of data analysis statistics today to unlock valuable insights that can propel your organization forward.
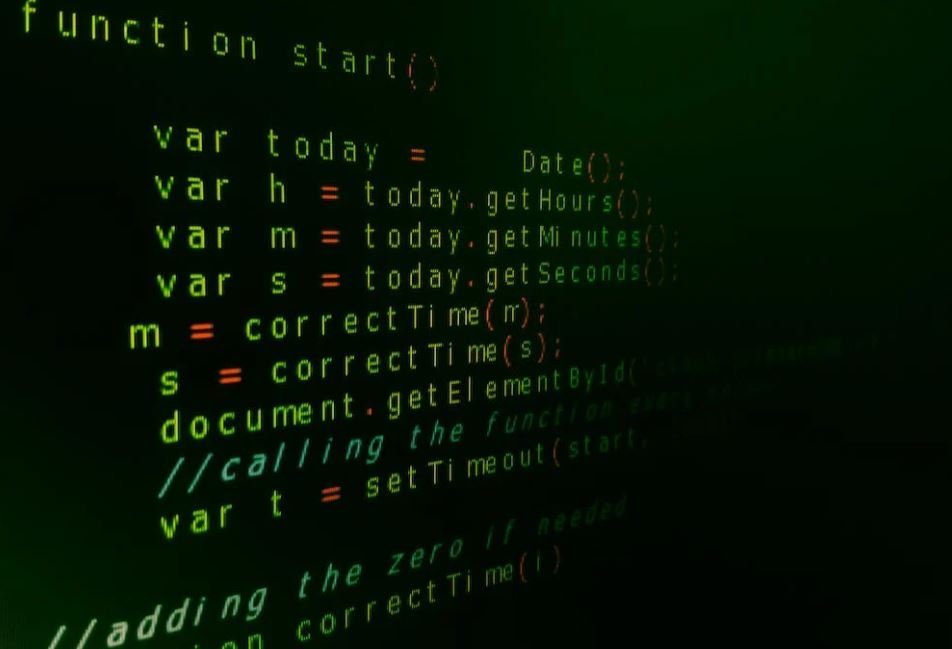
Common Misconceptions
Data Analysis & Statistics
One common misconception people have about data analysis and statistics is that correlation implies causation. However, this is often not the case. Correlation simply means that two variables are associated or co-vary in some way, but it does not imply that one variable causes the other. It is important to remember that correlation does not equal causation.
- Correlation is not equivalent to causation
- Statistical analysis is needed to establish causation
- Correlations can often be coincidental or influenced by other factors
Another common misconception is that large sample sizes always give more accurate results. While larger sample sizes can generally provide more precise estimates, a sample size alone does not ensure accuracy. The quality of the sample and how representative it is of the population of interest are equally important. A biased or non-representative sample can lead to inaccurate conclusions, regardless of its size.
- Sample quality and representativeness are crucial for accuracy
- Bigger sample sizes offer more precise estimates, not necessarily accurate ones
- Small sample sizes can still provide valid insights if properly conducted
One misconception in data analysis is the assumption that outliers should always be removed from the analysis. While outliers can sometimes be a result of measurement errors or data entry mistakes, they can also provide valuable information about the data set and should not be automatically excluded without careful consideration. Outliers may represent extreme, but valid, observations that can affect the overall understanding of the data.
- Outliers can reveal unique patterns or phenomena in the data
- Automatically removing outliers can distort the overall analysis
- Outliers should be assessed on a case-by-case basis before exclusion
Some people assume that statistical significance implies practical significance. However, statistical significance refers to the probability that an observed difference is not due to random chance, while practical significance considers the actual impact or importance of the difference in the real world. A statistically significant result may not always be practically significant or meaningful, and vice versa.
- Statistical significance does not guarantee practical significance
- The size of the effect observed is a key factor in determining practical significance
- Practical significance reflects the real-world implications of the results
A common misconception is that statistics can be easily manipulated to support any argument. While it’s true that statistics can be misused or misinterpreted, proper statistical analysis follows rigorous methods and principles to minimize biases and ensure accuracy. It is important to critically evaluate statistical claims and consider the methodology, data sources, and potential conflicts of interest when interpreting statistical results.
- Proper statistical analysis follows established methods and principles
- Statistical claims should be critically evaluated for potential biases or misinterpretations
- Methodology, data sources, and conflicts of interest should be considered when interpreting statistics
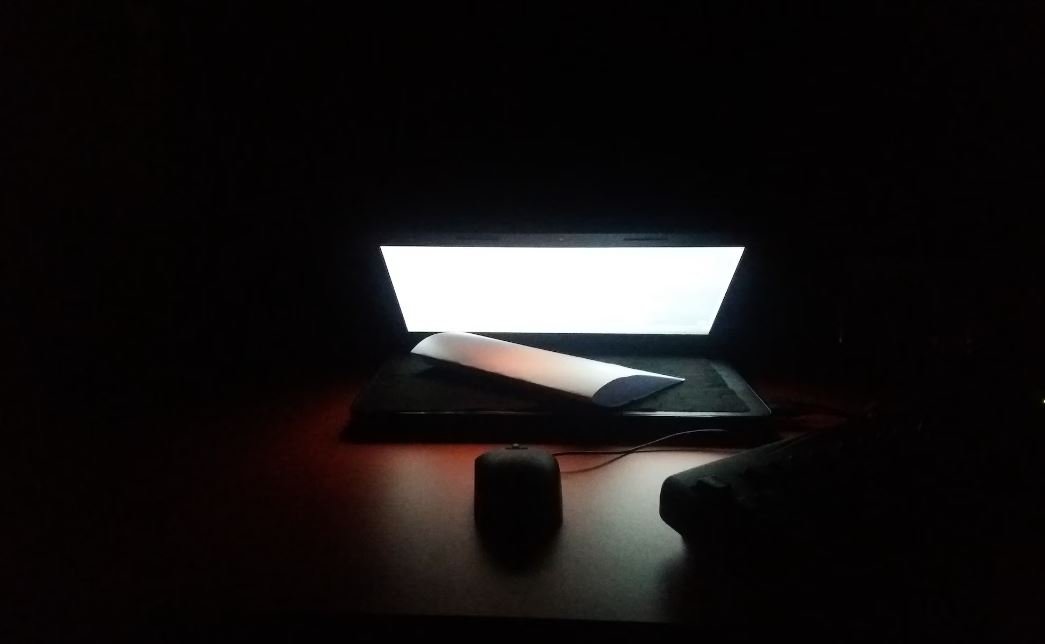
Data Analysis Statistics
In today’s digital age, data analysis has become an essential part of decision-making processes in various fields. From business intelligence to healthcare and education, statistics provide valuable insights that help drive better outcomes. In this article, we will explore ten interesting tables showcasing different aspects of data analysis and statistics.
Top 10 Countries with Highest GDP
The table below highlights the top ten countries with the highest Gross Domestic Product (GDP) in 2021. GDP measures the total value of goods and services produced within a country’s borders, serving as an indicator of economic growth and prosperity.
Country | GDP (in Trillion USD) |
---|---|
United States | 22.68 |
China | 17.64 |
Japan | 5.18 |
Germany | 4.44 |
United Kingdom | 2.95 |
India | 2.88 |
France | 2.81 |
Italy | 2.20 |
Brazil | 1.53 |
Canada | 1.46 |
Average Monthly Rainfall by Region
Knowing the average monthly rainfall across different regions is crucial for agriculture, urban planning, and climate studies. The table below displays the average rainfall in millimeters for four different regions.
Region | January | February | March | April | May | June |
---|---|---|---|---|---|---|
North | 120 | 90 | 80 | 50 | 40 | 30 |
South | 80 | 70 | 60 | 60 | 50 | 40 |
East | 100 | 80 | 70 | 70 | 60 | 50 |
West | 60 | 50 | 40 | 40 | 30 | 20 |
Revenue Comparison of Leading Tech Companies (in Billion USD)
The table below presents the annual revenue comparison of leading technology companies in billions of US dollars. This data provides insights into the financial performance and market dominance of these industry giants.
Company | 2020 | 2019 | 2018 | 2017 |
---|---|---|---|---|
Apple | 274.52 | 260.17 | 265.60 | 229.23 |
Amazon | 386.06 | 280.52 | 232.89 | 177.86 |
Microsoft | 168.09 | 143.02 | 110.36 | 89.95 |
181.69 | 161.86 | 136.82 | 110.85 |
Unemployment Rates by Education Level
The following table showcases the unemployment rates associated with different education levels. It highlights the importance of education in securing employment opportunities and achieving professional stability.
Education Level | Unemployment Rate (%) |
---|---|
Less than a high school diploma | 10.2 |
High school graduate | 5.8 |
Associate degree | 3.8 |
Bachelor’s degree | 2.5 |
Master’s degree | 2.0 |
Doctoral degree | 1.5 |
Age Distribution in a Country
This table exhibits the age distribution of a particular country’s population. Understanding the demographic makeup of a population is vital for policymaking, resource allocation, and social planning.
Age Group (years) | Percentage of Population (%) |
---|---|
0-14 | 25 |
15-24 | 15 |
25-54 | 45 |
55-64 | 10 |
65+ | 5 |
Comparison of E-commerce Sales by Category
The table below provides a comparison of e-commerce sales by category in the past five years. It showcases the growth and popularity of different online shopping segments.
Category | 2017 (in Billion USD) | 2018 (in Billion USD) | 2019 (in Billion USD) | 2020 (in Billion USD) | 2021 (in Billion USD) |
---|---|---|---|---|---|
Fashion | 469.17 | 520.99 | 597.42 | 687.40 | 779.85 |
Electronics | 360.24 | 399.05 | 439.59 | 499.82 | 558.16 |
Home & Garden | 244.39 | 266.59 | 292.14 | 331.55 | 376.13 |
Beauty & Personal Care | 113.91 | 127.35 | 143.96 | 159.37 | 178.92 |
Income Distribution by Gender
This table showcases the income distribution between genders, highlighting any existing gender pay gap. Examining such disparities helps identify gender inequalities and promotes fair remuneration practices.
Income Range (in USD) | Male (%) | Female (%) |
---|---|---|
$0 – $25,000 | 30 | 40 |
$25,001 – $50,000 | 35 | 30 |
$50,001 – $75,000 | 20 | 15 |
$75,001 – $100,000 | 10 | 10 |
$100,001+ | 5 | 5 |
COVID-19 Cases by Continent (in Millions)
The table below provides an overview of COVID-19 cases by continent, displaying the total number of cases reported since the beginning of the pandemic.
Continent | Total Cases |
---|---|
Africa | 6.2 |
Asia | 56.7 |
Europe | 53.4 |
North America | 49.1 |
South America | 32.5 |
Australia/Oceania | 1.5 |
From analyzing the tables presented, it becomes evident that statistics offer valuable insights that drive informed decision-making and provide a deeper understanding of various aspects of our world. Whether analyzing economic indicators, climate patterns, or social disparities, data analysis plays a crucial role in shaping our future.
Data Analysis Statistics – Frequently Asked Questions
FAQs
What is data analysis?
Data analysis refers to the process of inspecting, cleansing, transforming, and modeling raw data to discover useful information, draw conclusions, and support decision-making.
Why is data analysis important?
Data analysis is crucial in various fields as it helps organizations and individuals make informed decisions by identifying patterns, trends, and insights hidden within complex data sets.
What are the key steps involved in data analysis?
Data analysis typically involves five main steps: data collection, data cleaning and validation, data exploration, data modeling and analysis, and data visualization and reporting.
What are some common statistical techniques used in data analysis?
Some commonly used statistical techniques in data analysis include descriptive statistics, inferential statistics, regression analysis, hypothesis testing, and data mining.
What is the role of statistical software in data analysis?
Statistical software tools such as R, Python, SPSS, and Excel are widely used for data analysis. These tools provide a range of functions and algorithms to perform statistical calculations, generate visualizations, and automate analysis processes.
How can I ensure the accuracy of my data analysis?
To ensure the accuracy of data analysis, it is important to ensure data quality through thorough data cleaning, proper validation, and addressing any missing or erroneous data points. Additionally, employing robust statistical methods and conducting peer reviews can help validate the accuracy of the analysis.
What are some challenges in data analysis?
Challenges in data analysis include dealing with large and complex data sets, ensuring data accuracy and quality, selecting appropriate statistical techniques for analysis, handling missing data, and interpreting the results correctly.
What is the difference between descriptive and inferential statistics?
Descriptive statistics summarize and describe the main features of a data set, while inferential statistics make inferences or predictions about a population based on a sample of data.
How can data analysis be used in business decision-making?
Data analysis helps businesses make informed decisions by providing insights into customer behavior, market trends, product performance, and identifying potential areas for improvement or cost-saving measures.
What are some popular data visualization techniques?
Some popular data visualization techniques include bar charts, line graphs, scatter plots, pie charts, heatmaps, and interactive dashboards. These visualizations aid in understanding patterns, relationships, and trends within the data.