Data Analysis UX
Data analysis is a critical process in any organization that deals with large amounts of data. It involves extracting valuable insights and patterns from raw data to make informed business decisions. However, the process of analyzing data can be complex and time-consuming. That’s where data analysis UX (User Experience) comes in. It focuses on improving the usability and efficiency of data analysis tools and software, making it easier for users to interact with and understand data.
Key Takeaways:
- Data analysis UX aims to improve the usability and efficiency of data analysis tools.
- It focuses on making data analysis tools user-friendly and intuitive.
- Effective data analysis UX reduces the learning curve and increases productivity.
- UX design principles, such as simplicity and consistency, play a crucial role in data analysis UX.
Data analysis tools can range from basic spreadsheet software to advanced statistical analysis packages. Regardless of the complexity of these tools, their success heavily depends on the UX design. A well-designed data analysis tool should enable users to easily import, clean, and manipulate data. It should provide intuitive and efficient data visualization options to help users uncover patterns and trends in the data they are analyzing. **By prioritizing usability and user satisfaction, data analysis UX ensures that analysts can effectively perform their tasks.**
Data analysis UX is backed by extensive research and testing. UX designers apply various research methods, such as interviews and user observations, to understand the needs and pain points of data analysts. This research helps UX designers create personas and user journey maps, which provide insights into user motivations and behaviors. *Understanding the user perspective is crucial in designing data analysis tools that meet their needs.* UX designers also conduct usability testing to identify areas of improvement and iterate on their designs. This iterative process ensures that the final product is user-centered and aligned with the goals of data analysis.
In addition to usability, data analysis UX also focuses on visual aesthetics. Well-designed data visualizations can effectively communicate complex information in a concise and understandable manner. **By leveraging principles of visual hierarchy, color theory, and information design, data analysis UX enhances the readability and clarity of data visualizations, making it easier for analysts to interpret the results.**
Data analysis UX emphasizes simplicity and consistency in design. *A cluttered and complicated user interface can overwhelm users and hinder their productivity.* UX designers strive to create streamlined and intuitive interfaces that guide users through the data analysis process. They ensure that common actions and features are easily accessible, reducing the time spent on repetitive tasks. Consistent design patterns and conventions across different parts of the tool make it easier for users to navigate and learn the software. **By simplifying the user experience, data analysis UX helps analysts focus on deriving insights from data instead of struggling with the tool itself.**
Data Analysis UX | Traditional Data Analysis |
---|---|
Focuses on usability and user satisfaction | Primarily focused on data processing and analysis |
Emphasizes visual aesthetics and clarity | Visual design is secondary to data accuracy |
Simplifies complex data analysis tasks | Requires manual coding and complex formulas |
To successfully implement data analysis UX, organizations need to invest in both skilled UX designers and robust data analysis tools. The collaboration between data analysts, UX designers, and developers is crucial in understanding the unique requirements of the data analysis process and translating them into a user-friendly interface. **Continuous improvement and user feedback are key in refining and optimizing data analysis UX to meet the evolving needs of users.**
Final Thoughts
Data analysis UX is a vital component of effective data analysis. By focusing on usability, visual aesthetics, and simplicity, it enhances the overall experience of data analysts and promotes more efficient decision-making. With the increasing reliance on data in today’s business landscape, organizations that prioritize data analysis UX will have a competitive advantage in harnessing the power of data. **Investing in data analysis UX is investing in the future success of your organization.**
Benefits of Data Analysis UX |
---|
Improved user productivity |
Easier data interpretation |
Enhanced decision-making |
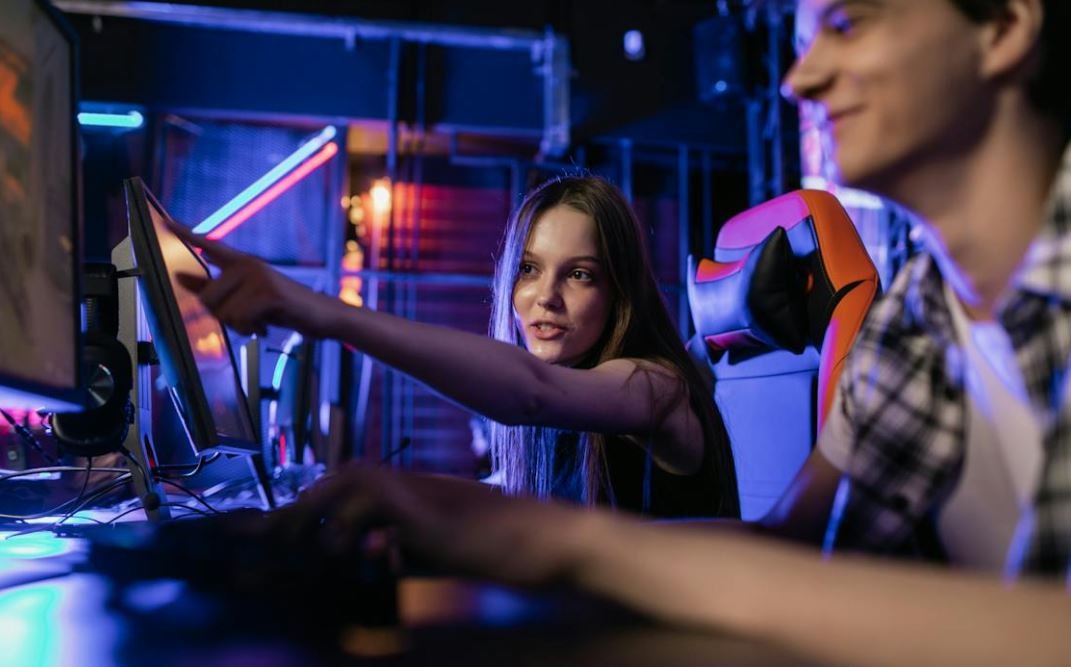
Common Misconceptions
Misconception 1: Data analysis is only for experts
One common misconception about data analysis is that it’s a complex and technical field that can only be understood by experts. While it’s true that there are advanced techniques and tools used in data analysis, the fundamentals are relatively easy to grasp. With some basic understanding of statistics and access to user-friendly software, anyone can start analyzing data to gain valuable insights.
- Data analysis can be learned by following online tutorials and courses.
- Free and user-friendly software like Google Sheets and Tableau are available for beginners.
- Data analysis skills are becoming increasingly important in many professional fields.
Misconception 2: Data analysis is only about numbers
Another misconception is that data analysis is purely a numbers game. While numbers play a significant role in data analysis, it’s not the only aspect to consider. Data analysis involves understanding the context behind the data and interpreting it to make informed decisions. This includes qualitative data, such as customer feedback, user behavior, and market trends.
- Qualitative data can provide valuable insights into user preferences and behavior.
- Data visualization techniques help to present data in a more understandable way.
- Data analysis involves storytelling and communicating insights effectively.
Misconception 3: Data analysis is a one-time process
Many people believe that data analysis is a one-time process that provides immediate answers. However, data analysis is an iterative process that requires continuous monitoring and evaluation. It involves defining specific goals, collecting relevant data, analyzing it, and then using the insights to improve decision-making. Regularly updating and refining data analysis methods can lead to more accurate and actionable results.
- Data analysis should be an ongoing process to ensure up-to-date insights.
Misconception 4: Data analysis is only for large organizations
Some people believe that data analysis is only applicable to large organizations with extensive resources. However, data analysis can be valuable for businesses of all sizes, including small startups and even individuals. In fact, strategic data analysis can help small businesses gain a competitive advantage by identifying market trends, understanding customer behavior, and optimizing operations.
- Small businesses can leverage publicly available data to perform analysis.
Misconception 5: Data analysis is the end goal
Lastly, one misconception is that data analysis is the ultimate goal or solution in itself. However, data analysis is a means to an end—an enabler of informed decision-making. The insights gained from data analysis should be used to drive action and make improvements in various areas, such as marketing strategies, product development, customer experience, and operational efficiency.
- Actionable insights obtained from data analysis require implementation to drive real change.
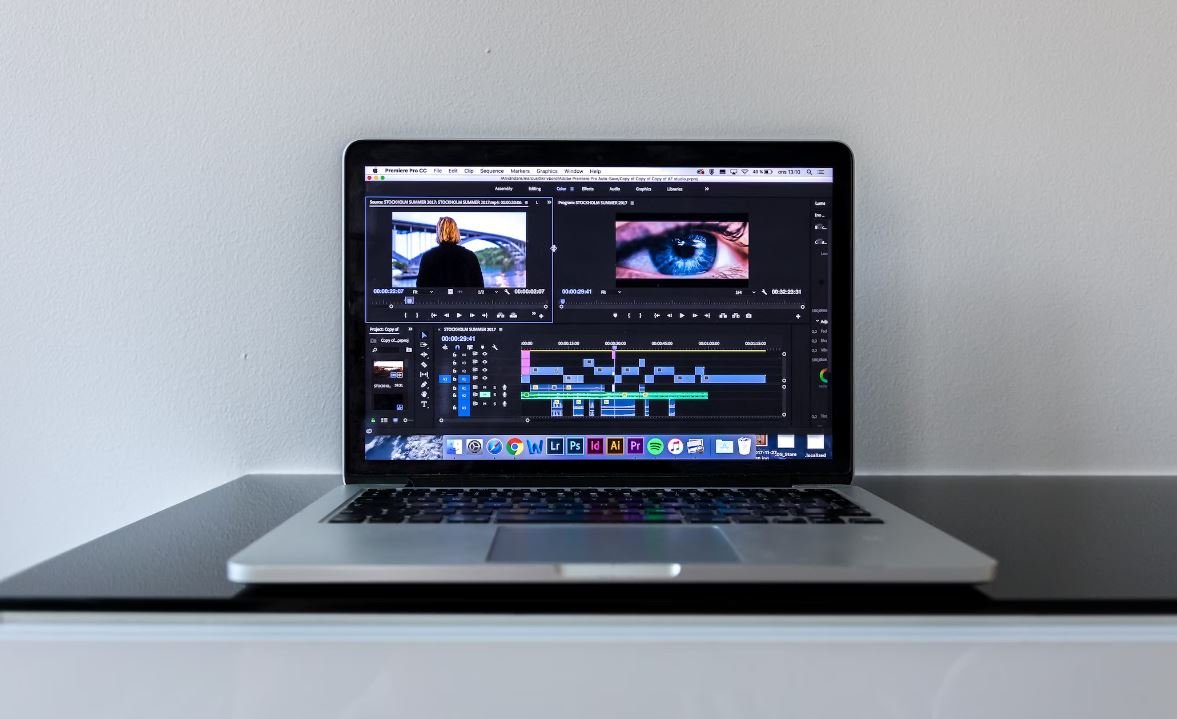
In today’s digital age, data analysis has become an integral part of understanding user experience (UX). By examining data patterns, designers and researchers can gain valuable insights into how users interact with digital products and make informed decisions to improve UX. Let’s delve into ten captivating tables that illustrate key points and data related to data analysis in the realm of UX.
Table 1: User Engagement Metrics for a Mobile App
This table showcases the average time spent by users on a specific mobile app and the corresponding engagement metrics such as the number of sessions, screen views, and average session duration. It provides a snapshot of how users engage with the app and highlights areas for improvement.
Table 2: Conversion Rates by Platform and Device
By analyzing conversion rates across various platforms and devices, this table reveals valuable insights about user behavior. It compares conversion rates between desktop, mobile, and tablet devices, shedding light on optimization opportunities for each platform.
Table 3: Customer Satisfaction Scores by Interaction Type
This table presents customer satisfaction scores based on different interaction types, such as social media support, live chat, or phone calls. It helps identify which interactions provide the most satisfactory user experiences, enabling businesses to focus their efforts accordingly.
Table 4: A/B Test Results for Call-to-Action Buttons
Through A/B testing, this table reveals the performance of different call-to-action buttons. By comparing conversion rates, click-through rates, and bounce rates, designers can make data-driven decisions on which button design resonates best with users.
Table 5: User Demographics and UX Preferences
Analyzing user demographics in relation to UX preferences can be immensely valuable. This table showcases user age groups and their preferred design elements, allowing designers to tailor the experience to specific target audiences.
Table 6: Time Spent on Core Features vs. Secondary Features
This table displays the amount of time users spend on core features compared to secondary features of a product, providing insights into which features should receive more attention and refinement.
Table 7: Website Loading Times by Device and Browser
The loading time of a website influences user satisfaction and engagement. This table presents loading times across various devices and browsers, helping designers prioritize optimization efforts to enhance user experience.
Table 8: User Feedback Distribution by Product Features
By categorizing user feedback based on specific product features, this table illustrates the distribution of positive, neutral, and negative feedback. It facilitates understanding user sentiment towards different aspects of a digital product.
Table 9: User Retention Rates Over Time
Tracking user retention rates over time can help assess the effectiveness of UX optimizations. This table visualizes retention rates for various time frames, enabling designers to identify trends and adjust strategies accordingly.
Table 10: User Flow Visualization for a Web Application
This table presents a visual representation of user flows within a web application, portraying the most common paths taken by users. It highlights potential bottlenecks or areas where users may encounter difficulties, prompting further investigation for UX enhancements.
In conclusion, data analysis plays a pivotal role in understanding and improving user experience. The insightful tables presented in this article demonstrate the power of data-driven decisions in designing and optimizing digital products. By harnessing the insights provided by data analysis, businesses and designers can create enjoyable and engaging experiences that resonate with users.
Frequently Asked Questions
Q1: What is data analysis?
A1: Data analysis is the process of inspecting, cleaning, transforming, and modeling data with the goal of discovering useful information, drawing conclusions, and supporting decision-making.
Q2: Why is data analysis important for UX?
A2: Data analysis plays a crucial role in UX as it helps identify user needs, preferences, and behaviors, enabling informed design decisions and optimal user experiences.
Q3: What are some common data analysis techniques used in UX?
A3: Some common data analysis techniques used in UX include qualitative analysis, quantitative analysis, user segmentation, A/B testing, heatmaps, click tracking, and sentiment analysis.
Q4: How can data analysis help improve conversion rates?
A4: Data analysis allows UX professionals to identify bottlenecks, optimize user flows, and understand user interactions, leading to data-driven changes and interventions to improve conversion rates.
Q5: What is user testing in data analysis?
A5: User testing is a data analysis method where users are observed while interacting with a product or prototype to collect data on behavior, preferences, and satisfaction for UX improvement.
Q6: How can data analysis help in identifying user pain points?
A6: By analyzing user interactions, feedback, and relevant data, UX professionals can identify pain points in the user experience, enabling targeted improvements.
Q7: What is the role of data visualization in data analysis for UX?
A7: Data visualization presents complex data in a visual format, such as charts and graphs, facilitating effective communication of trends, patterns, and insights to stakeholders.
Q8: How can data analysis contribute to personalization of user experiences?
A8: By analyzing user data, UX professionals can personalize experiences by segmenting users and delivering targeted content or customization based on individual needs and preferences.
Q9: What are some challenges in data analysis for UX?
A9: Challenges include collecting accurate data, ensuring privacy and security, dealing with large volumes of data, selecting appropriate analysis techniques, and effectively communicating results.
Q10: Can data analysis be applied to both web and mobile app UX?
A10: Absolutely! Data analysis is applicable to both web and mobile app UX, adapting principles and techniques to improve user experiences across platforms and devices.