Data Analysis vs Discussion
Data analysis and discussion are two critical components of any research or decision-making process. While both are essential, they serve different purposes and have distinct characteristics. Understanding the difference between them is crucial to make informed decisions based on data-driven insights.
Key Takeaways
- Data analysis involves examining, cleaning, transforming, and modeling data to extract meaningful information.
- Discussion interprets and communicates the findings resulting from data analysis, providing insights and recommendations.
- Both data analysis and discussion are iterative processes that contribute to refining research questions and hypotheses.
Data Analysis
Data analysis is the process of examining large amounts of data to identify patterns, relationships, and trends. It involves several steps, including data cleaning, data transformation, and data modeling. Data cleaning focuses on removing errors, inconsistencies, and outliers from the dataset, ensuring data quality for accurate analysis. *Data analysis can uncover hidden patterns and correlations that may not be immediately obvious*
Different Stages of Data Analysis
- Data Cleaning: Remove errors, inconsistencies, and outliers from the dataset.
- Data Transformation: Convert raw data into a suitable format for analysis, including normalization and aggregation.
- Data Modeling: Apply statistical techniques, algorithms, or machine learning algorithms to explore relationships, make predictions, or identify patterns in the data.
Statistical Technique | Use |
---|---|
T-test | Compare means of two groups. |
Regression Analysis | Examine relationships between dependent and independent variables. |
Discussion
Discussion involves interpreting the findings resulting from data analysis and providing insights and recommendations based on these findings. It involves analyzing patterns, relationships, and trends identified during the data analysis process. Through discussion, researchers or decision-makers can communicate the implications and potential significance of their findings. *Discussion enables stakeholders to make informed decisions based on data-driven insights*
Components of Discussion
- Interpretation: Analyze and explain the meaning and implications of the data analysis results.
- Insights: Extract key insights from the analysis to uncover trends, patterns, or relationships.
- Recommendations: Provide actionable recommendations based on the insights to guide future actions or decisions.
Insight | Recommendation |
---|---|
Increased customer satisfaction with new product features. | Invest in further research and development to enhance customer experience. |
Decreased sales in a specific market segment. | Implement targeted marketing strategies to regain market share. |
Conclusion
In conclusion, data analysis and discussion are integral parts of the data-driven decision-making process. While data analysis focuses on extracting insights from large datasets, discussion interprets and communicates the findings to stakeholders to guide future actions or decisions. Integrating both processes allows organizations to make informed decisions based on accurate, meaningful data analysis results. By understanding the differences and leveraging the strengths of each process, organizations can optimize their decision-making capabilities.
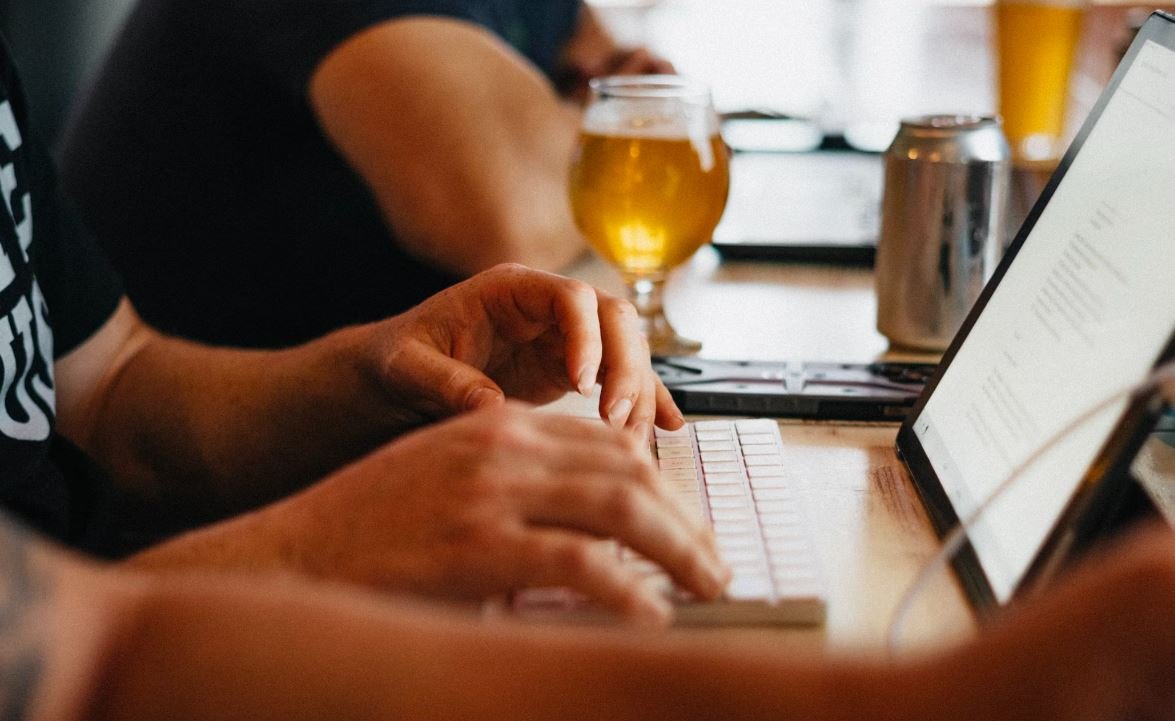
Common Misconceptions
Misconception 1: Data Analysis is only about numbers
One common misconception people have about data analysis is that it involves exclusively working with numbers and statistics. While quantitative data analysis is certainly a critical aspect of the field, data analysis also encompasses the examination and interpretation of qualitative data, such as text, images, and audio. Data analysis involves identifying patterns, trends, and insights from various sources of data, not just numerical ones.
- Data analysis involves both quantitative and qualitative data.
- Data analysis entails identifying patterns and trends from different data sources.
- Data analysis is not limited to working with numbers and statistics.
Misconception 2: Data analysis eliminates the need for human discussion
Another misconception is that data analysis eliminates the need for human discussion. However, data analysis should not be seen as a replacement for human interaction and collaboration. While data can provide valuable insights and inform decision-making processes, it still requires interpretation and contextualization by individuals with domain knowledge. Effective data analysis often involves discussions among team members to share insights, explore different perspectives, and make informed decisions.
- Data analysis should be complemented by human discussion and collaboration.
- Data analysis requires interpretation and contextualization by individuals.
- Data analysis is a tool that informs decision-making but still relies on human input.
Misconception 3: Data analysis is a one-time process
Some people mistakenly believe that data analysis is a one-time process that ends after analyzing a dataset. In reality, data analysis is an iterative and ongoing process. As new data becomes available, it needs to be analyzed and compared to existing insights to uncover new patterns and trends. Continuous data analysis allows organizations to make informed decisions based on the most up-to-date information and adapt their strategies as needed.
- Data analysis is an iterative and ongoing process.
- Data analysis involves analyzing new data to uncover new insights.
- Data analysis enables organizations to adapt their strategies based on the most up-to-date information.
Misconception 4: Data analysis is solely the responsibility of data analysts
There is a common misconception that data analysis is solely the responsibility of data analysts or experts with technical skills. While data analysts play a crucial role in analyzing and interpreting data, effective data analysis involves collaboration between different stakeholders within an organization. Domain experts, managers, and decision-makers also need to actively participate in data analysis processes to ensure the insights generated align with their goals and objectives.
- Data analysis requires collaboration between different stakeholders.
- Domain experts and decision-makers should actively participate in data analysis.
- Data analysis involves the contribution of individuals with different skills and expertise.
Misconception 5: Data analysis guarantees accurate and objective results
While data analysis aims to provide accurate and objective insights, it is important to recognize that biases and limitations can still influence the results. Data quality issues, sampling biases, or human biases in the data collection process can all affect the validity of the analysis. Additionally, the interpretation and framing of the analysis can introduce subjectivity. It is essential to critically evaluate the analysis and consider potential limitations to ensure more reliable and robust conclusions.
- Data analysis is susceptible to biases and limitations.
- Data quality and collection biases can impact the validity of the analysis.
- Interpretation and framing of the analysis can introduce subjectivity.
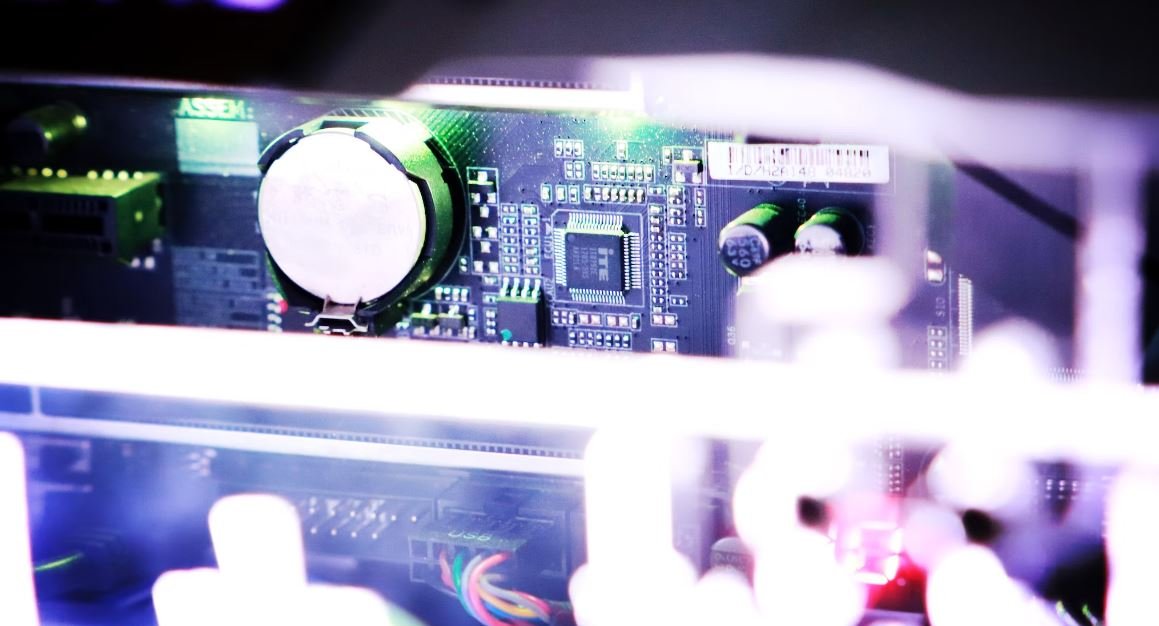
Data Analysis vs Discussion
Data analysis and discussion are two essential components of any research study. While data analysis involves understanding and interpreting raw data to extract meaningful insights, the discussion section provides an opportunity to analyze, interpret, and connect these findings with existing knowledge. In this article, we explore various aspects of data analysis and discussion, showcasing their importance in the research process. The following tables present intriguing data and points related to this topic.
Table 1: Comparative Analysis of Data Analysis and Discussion
The first table presents a comparison between data analysis and discussion, highlighting their distinct purposes and functions within a research study:
Aspect | Data Analysis | Discussion |
---|---|---|
Purpose | Analyze and interpret raw data | Analyze and interpret findings, relate to existing knowledge |
Focus | Numerical analysis | Conceptual analysis |
Process | Statistical techniques, visualization | Interpretation, implication |
Goal | Extract meaningful insights | Provide context and implications |
Table 2: Data Analysis Techniques
This table outlines various data analysis techniques commonly employed in research:
Technique | Description |
---|---|
Descriptive Statistics | Summarizes and describes data using measures such as averages, standard deviation, etc. |
Inferential Statistics | Draws conclusions and makes inferences about the population based on a sample. |
Content Analysis | Systematically categorizes and analyzes textual or visual data. |
Qualitative Analysis | Interprets non-numerical data to uncover underlying meanings and themes. |
Table 3: Role of Data Analysis in Research Studies
This table explores the crucial roles data analysis plays in research studies:
Role | Description |
---|---|
Validation | Verifying research findings through rigorous statistical analysis. |
Identification of Patterns | Uncovering trends, correlations, and relationships within the data. |
Transformation | Converting raw data into meaningful information for interpretation and discussion. |
Visualization | Presenting data in visual formats such as charts and graphs for ease of understanding. |
Table 4: Themes Explored in Discussion Sections
Explore the diverse themes that are frequently examined in the discussion sections of research articles:
Themes | Description |
---|---|
Implications | Discussing the practical consequences and potential applications of the research findings. |
Limitations | Identifying and contextualizing the shortcomings or constraints of the study. |
Comparison with Previous Studies | Comparing and contrasting the current findings with existing literature. |
Suggestions for Future Research | Providing recommendations for further studies and areas of exploration. |
Table 5: Use of Visual Aids in Data Analysis
Visual aids are valuable tools in data analysis to enhance comprehension and facilitate effective communication:
Visual Aid | Description |
---|---|
Bar Chart | Graphical representation of data using vertical bars to illustrate comparisons. |
Pie Chart | Circular chart divided into sectors, representing proportions or percentages. |
Scatter Plot | Displays the correlation between two variables in a Cartesian coordinate system. |
Heatmap | Visual representation of data using color intensity to depict values on a matrix. |
Table 6: Sample Size Considerations in Data Analysis
Sample size is a crucial factor in research studies, impacting the statistical analysis and generalizability:
Sample Size | Data Analysis Considerations |
---|---|
Small Sample | Greater risk of biased results, limited generalizability to the population. |
Large Sample | Increased statistical power, more precise estimates, improved generalizability. |
Optimal Sample | Balance between statistical power, feasibility, and cost-effectiveness. |
Table 7: Common Pitfalls in Data Analysis and Discussion
Avoiding these common pitfalls helps ensure robust data analysis and meaningful discussions:
Pitfalls | Description |
---|---|
Confirmation Bias | Only analyzing data that confirms pre-existing beliefs or hypotheses. |
Overgeneralization | Drawing broad conclusions based on limited or unrepresentative data. |
Under-interpretation | Failure to delve deeper into the data, missing potential insights or patterns. |
Glossing Over Limitations | Downplaying or neglecting the limitations of the study in the discussion. |
Table 8: Impact of Data Analysis on Research Outcomes
The process of data analysis significantly influences the outcomes of research studies:
Outcomes | Description |
---|---|
Significant Findings | Data analysis has a direct impact on discovering significant results or patterns. |
Conclusions | Data analysis helps draw logical conclusions and support or nullify research hypotheses. |
Publication Potential | High-quality data analysis strengthens the chances of publishing the study. |
Research Impact | Robust data analysis enhances the potential impact and applicability of the research. |
Table 9: Importance of Discussion in Research
The discussion section plays a vital role in framing and contextualizing research findings:
Importance | Description |
---|---|
Interpretation | Discussion explains the meaning and implications of the research findings. |
Contextualization | Situating the study within the wider research landscape and existing knowledge. |
Addressing Limitations | Transparently discussing limitations and potential sources of bias or error. |
Future Directions | Providing insights and recommendations for future research endeavors. |
Conclusion
Data analysis and discussion are interdependent components of research studies, with distinct roles and functions. Data analysis involves the utilization of various techniques and methods to extract meaningful insights from raw data. On the other hand, the discussion section helps interpret, contextualize, and connect the findings with existing knowledge. Together, they contribute to the credibility, validity, and impact of research. By employing robust data analysis techniques and presenting thoughtful discussions, researchers can enhance the quality and usefulness of their studies, facilitating progress and advancing knowledge in their respective fields.
Data Analysis vs Discussion
Frequently Asked Questions
- What is data analysis?
-
Data analysis is the process of inspecting, cleaning, transforming, and modeling data to uncover useful information, draw conclusions, and support decision-making. It involves techniques such as statistical analysis, data mining, and data visualization.
- What is discussion?
-
Discussion refers to the exchange of ideas, opinions, and perspectives on a particular topic. In the context of data analysis, discussion involves the interpretation of the findings, sharing of insights, and brainstorming potential implications and next steps.
- How do data analysis and discussion differ?
-
Data analysis focuses on processing and understanding data, whereas discussion centers around the exploration and interpretation of the analysis results. While data analysis provides factual insights, discussion adds context, varying perspectives, and encourages critical thinking.
- Can data analysis be done without discussion?
-
Yes, data analysis can be performed without immediate discussion. However, the insights gained from analysis often benefit from discussion, as it enables different stakeholders to collaborate, validate findings, and generate new ideas.
- Is discussion more important than data analysis?
-
Both data analysis and discussion are important components of the decision-making process. While data analysis provides objective insights, discussion allows for a deeper understanding, contextualization, and consideration of various perspectives, ultimately leading to more informed decisions.
- What skills are required for data analysis?
-
Data analysis requires a range of skills including statistical analysis, data mining, programming, data visualization, critical thinking, problem-solving, and domain knowledge related to the data being analyzed.
- What skills are required for effective discussion?
-
Effective discussion requires strong communication, active listening, analytical thinking, open-mindedness, empathy, and the ability to synthesize information and contribute constructively to the exchange of ideas.
- Are data analysis and discussion iterative processes?
-
Yes, both data analysis and discussion are often iterative processes. As new insights or questions arise during the analysis, they can lead to further discussions and reevaluations of the data. Similarly, discussions may indicate the need for additional data analysis or refinement of analysis methods.
- How does data analysis contribute to the discussion?
-
Data analysis contributes to the discussion by providing objective findings, visualizations, and statistical evidence. This enables participants to have a common understanding of the data and facilitates a more focused and informed discussion.
- Can data analysis and discussion be automated?
-
Certain aspects of data analysis, such as data processing and basic statistical calculations, can be automated using software tools. However, the interpretive nature of discussion, with its context-driven insights, perspectives, and decision-making, remains predominantly human-driven and challenging to automate.