Data Analysis vs Results
When it comes to understanding and interpreting data, both data analysis and results play crucial roles. Data analysis involves the process of examining and interpreting data to uncover patterns, trends, and insights. On the other hand, results refer to the outcomes or findings derived from data analysis. While data analysis focuses on the methodology and techniques used to analyze data, results provide actionable information and guide decision-making. In this article, we will explore the relationship between data analysis and results, their importance, and how they complement each other in various fields.
Key Takeaways:
- Data analysis involves examining and interpreting data to uncover patterns and insights.
- Results refer to the outcomes or findings derived from data analysis.
- Data analysis and results are both crucial in guiding decision-making.
- Data analysis provides the methodology and techniques, while results offer actionable information.
Data analysis is the process by which raw data is transformed into meaningful information through various techniques and methodologies. It involves organizing, cleaning, and analyzing large datasets to identify patterns, relationships, and trends. *Data analysis plays a vital role in gaining insights from the data that can be used to inform decision-making and drive business strategies.* Whether it’s analyzing consumer behavior, market trends, or performance metrics, data analysis offers a powerful toolkit to extract valuable knowledge from complex datasets.
Results, on the other hand, represent the outcomes or findings obtained from data analysis. They provide specific answers to research questions or address the goals and objectives of a study. *Results translate the data analysis into actionable information and provide evidence to support decision-making.* These findings often take the form of statistical summaries, visualizations, or predictive models, depending on the nature of the data and the intended audience.
The Relationship between Data Analysis and Results
Data analysis and results are interconnected processes that complement each other. Data analysis provides the foundation for deriving meaningful results, while results validate and inform further data analysis. *Data analysis serves as the backbone of result interpretation, enabling researchers and analysts to draw accurate conclusions.* It involves applying statistical techniques, data mining algorithms, and machine learning models to extract relevant information and gain a deeper understanding of the dataset.
Once data analysis is complete, the results obtained can be used to evaluate hypotheses, verify research objectives, and draw meaningful insights. *Results validate the accuracy and effectiveness of the data analysis process.* Researchers and analysts interpret the results in light of the research question or problem statement, making connections and drawing conclusions based on the evidence provided by the data analysis. *Effective interpretation of results requires a combination of domain knowledge, analytical skills, and critical thinking.*
The Importance of Data Analysis and Results
Data analysis and results are crucial components in various fields, including business, healthcare, finance, and research. *Data analysis helps organizations uncover patterns and trends, gain insights into customer behavior, and make informed decisions.* It assists in identifying bottlenecks, detecting outliers, and optimizing processes to enhance performance and efficiency. Results, on the other hand, provide evidence-based conclusions and recommendations that guide decision-making and can impact the success of projects, campaigns, or policies.
Moreover, data analysis and results play a significant role in research and academia. Researchers rely on data analysis to support their hypotheses, draw conclusions from research studies, and contribute to existing knowledge. *By analyzing data and obtaining meaningful results, researchers can provide empirical evidence and contribute to the advancement of their respective fields.* Results help verify or refute existing theories, challenge assumptions, and open avenues for further exploration and investigation.
Data Analysis vs Results: Real-Life Examples
Let’s look at some real-life examples where data analysis and results play a crucial role:
Table 1: Sales Performance Comparison
Product | Region | Q1 2020 Sales | Q1 2021 Sales |
---|---|---|---|
Product A | North | 1000 | 1200 |
Product B | North | 800 | 900 |
Product A | South | 700 | 750 |
Product B | South | 600 | 700 |
In this example, data analysis involves comparing sales performance between different regions and quarters. The results obtained from the analysis show an increase in sales for both Product A and Product B from Q1 2020 to Q1 2021, indicating positive growth in these regions.
Table 2: Patient Outcome Analysis
Patient ID | Treatment Group | Outcome |
---|---|---|
001 | A | Improved |
002 | B | Unchanged |
003 | A | Improved |
004 | B | Improved |
In this healthcare example, data analysis is performed to evaluate the effectiveness of two different treatment groups. The results indicate that patients in treatment group A had an improved outcome in comparison to treatment group B, suggesting a potential advantage of using treatment group A for similar cases.
Table 3: Survey Results
Question | Response |
---|---|
Q1 | Agree |
Q2 | Disagree |
Q3 | Neither Agree nor Disagree |
In this market research example, data analysis is carried out to analyze survey responses. The results provide insights into customer opinions and attitudes towards specific questions, helping businesses uncover trends and preferences among their target audience.
In summary, data analysis and results are inseparable components of understanding and interpreting data. Data analysis provides the necessary methods and techniques to uncover patterns, while results translate the analysis into actionable information that guides decision-making. Both elements are essential in various fields, helping organizations draw insights, researchers contribute to existing knowledge, and businesses make informed decisions. When utilized effectively, data analysis and results can be powerful tools for success in today’s data-driven world.
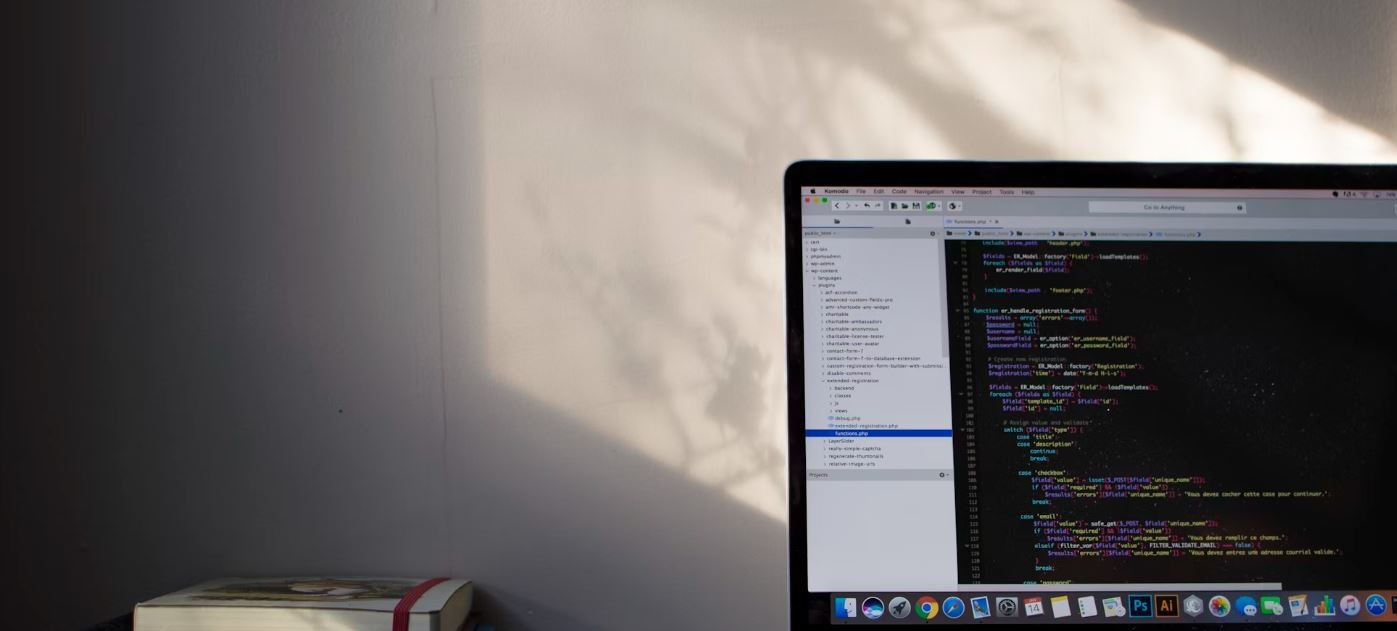
Common Misconceptions
Data Analysis vs Results
There are several common misconceptions surrounding the concepts of data analysis and the results derived from it. These misconceptions often arise due to a lack of understanding or misinformation. By clarifying these misconceptions, we can gain a better understanding of the true nature of data analysis and its results.
- Data analysis is purely technical: One common misconception is that data analysis is solely a technical process that involves complex algorithms and statistical methods. While technical skills are important in data analysis, it is equally crucial to have a solid understanding of the domain or subject matter being analyzed.
- Data analysis provides definite answers: Another misconception is that data analysis always provides clear and definitive answers to questions. In reality, data analysis is an iterative process that involves exploring and interpreting data to gain insights. The results obtained from data analysis are often subject to interpretation and require further investigation.
- Data analysis is applicable only to large datasets: Many people believe that data analysis is only useful for large, complex datasets. However, data analysis can be applied to datasets of any size, including small or medium-sized datasets. The key is to use appropriate statistical techniques and tools that are suitable for the dataset being analyzed.
Interpretation vs Manipulation
Another set of common misconceptions revolves around the distinction between interpretation and manipulation of data. These misconceptions can lead to misunderstandings about the integrity and objectivity of data analysis results.
- Data analysis is manipulative: One misconception is that data analysis is used to manipulate data and manipulate results to fit a particular narrative or agenda. In reality, data analysis aims to uncover patterns and insights within the data, providing objective and evidence-based information.
- Data interpretation is subjective: Another misconception is that data interpretation is purely subjective and open to individual biases. While interpretation can involve subjective elements, it is grounded in statistical methods, logical reasoning, and a rigorous understanding of the data.
- Data manipulation can only produce desired outcomes: Some people believe that data manipulation can always produce desired outcomes. However, manipulation of data can lead to unreliable and misleading results. Reliable data analysis requires adherence to ethical practices and the use of valid statistical techniques.
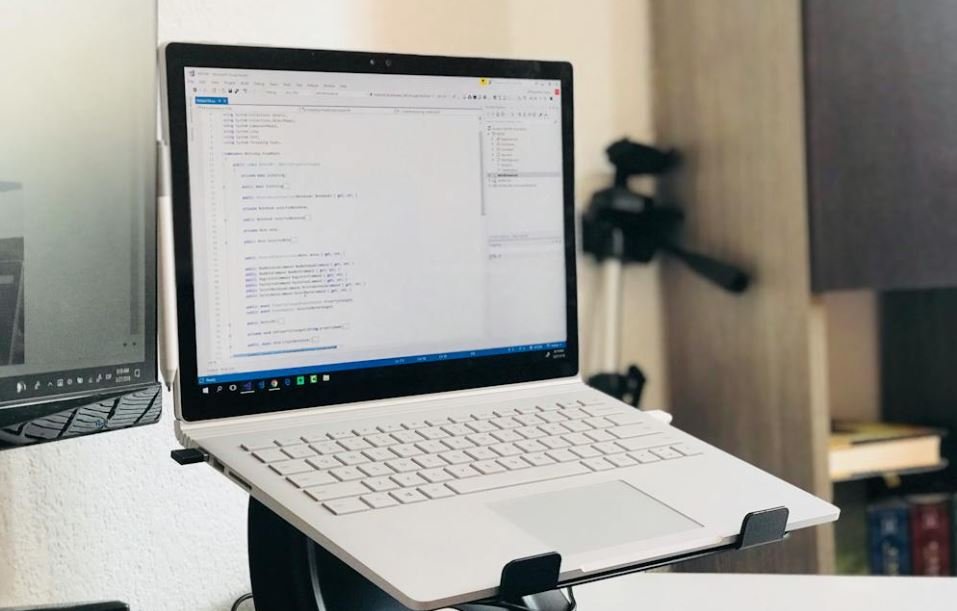
Introduction:
The field of data analysis and the interpretation of its results play vital roles in various domains such as business, research, and academia. This article aims to shed light on this topic by presenting 10 interesting tables that highlight different aspects of data analysis and the outcomes it generates.
1. The Top 5 Sales Figures for Q2 2021:
In this table, we present the top five sales figures for the second quarter of 2021. These numbers provide insights into the most successful products or services during this specific time period. Understanding sales data aids in identifying market trends and helps in making strategic decisions to enhance business performance.
2. Average Monthly Temperature Variations:
By showcasing the average monthly temperature variations in this table, we can evaluate climatic changes over a specific duration. This data analysis is crucial for climatologists and environmental scientists working towards understanding climate patterns and predicting future weather scenarios.
3. Student Performance Comparison in Mathematics and Science:
In this table, we compare the academic performance of students in mathematics and science subjects. Such analysis allows educators and policymakers to identify areas that require improvement within the education system. Addressing the disparities between subjects aids in enhancing overall learning outcomes.
4. Research Funding Allocation by Sector:
This table presents the distribution of research funding among different sectors, such as healthcare, technology, and social sciences. The allocation of resources in research is essential for promoting innovation and addressing societal challenges effectively.
5. Stock Market Performance Comparison:
By comparing the performance of various stocks in this table, investors can evaluate the profitability of their investment portfolio. Data analysis provides meaningful insights into market trends, allowing individuals to make informed decisions regarding buying or selling stocks.
6. Crime Rate Analysis by City:
This table illustrates the crime rates in different cities, enabling the evaluation of safety and security levels across regions. Such analysis helps law enforcement agencies and policymakers in developing effective crime prevention strategies and allocating resources appropriately.
7. Customer Satisfaction Ratings by Product Category:
By analyzing customer satisfaction ratings based on product categories, businesses can identify areas for improvement and enhance overall customer experience. This table helps companies make data-driven decisions to boost customer loyalty and retention.
8. COVID-19 Vaccination Rate by Age Group:
In this table, we present the vaccination rates by age group to evaluate the effectiveness of vaccination campaigns. Data analysis in the context of public health helps policymakers determine priority groups, allocate resources efficiently, and achieve vaccination targets.
9. Website Traffic Analysis by Source:
By analyzing website traffic data based on different sources, marketers can assess the effectiveness of their marketing campaigns. Evaluating the origins of website visitors helps in optimizing marketing strategies to attract the desired target audience effectively.
10. Annual Energy Consumption by Country:
This table allows us to compare the annual energy consumption of various countries, shedding light on the energy usage patterns globally. Understanding energy consumption trends is crucial for policymakers and scientists working towards sustainable development and reducing carbon emissions.
Conclusion:
Data analysis is a powerful tool for uncovering patterns, making informed decisions, and deriving actionable insights across various fields. From business strategies to healthcare planning, education reforms to climate modeling, data analysis helps us navigate the complexities of our world more effectively. By delving into the results of data analysis, we can harness the power of information to drive positive change and foster growth in both personal and professional domains.
Data Analysis vs Results – Frequently Asked Questions
FAQ 1: What is data analysis?
Data analysis refers to the process of inspecting, cleansing, transforming, and modeling data to uncover useful information, draw conclusions, and support decision-making. It involves applying various techniques and tools to extract meaningful insights from a dataset.
FAQ 2: How are data analysis and results related?
Data analysis is the systematic approach used to derive results from raw data. Results, in the context of data analysis, refer to the meaningful information or findings obtained after analyzing the data through various statistical and analytical methods.
FAQ 3: What is the importance of data analysis in generating results?
Data analysis is crucial in generating reliable and accurate results. It helps to identify patterns, trends, correlations, and dependencies within the data, allowing for informed decision-making, problem-solving, and actionable insights.
FAQ 4: What are some commonly used data analysis techniques?
Some commonly used data analysis techniques include descriptive statistics, inferential statistics, data mining, data visualization, regression analysis, hypothesis testing, clustering, and machine learning. The choice of technique depends on the nature of the data and the specific objectives of the analysis.
FAQ 5: Can data analysis alone provide meaningful results?
Data analysis alone cannot provide meaningful results. The interpretation and understanding of the analyzed data are crucial in deriving useful insights and meaningful conclusions. Data analysis merely helps in uncovering patterns and relationships within the data.
FAQ 6: How are the results of data analysis presented?
The results of data analysis are typically presented in a structured manner, including tables, charts, graphs, and reports. Visualization techniques such as bar charts, pie charts, line graphs, and scatter plots are often employed to represent the findings in a visually appealing and easily understandable manner.
FAQ 7: Are there any limitations to data analysis?
Yes, data analysis has certain limitations. It relies heavily on the quality and relevance of the data being analyzed. Inaccurate or incomplete data can result in unreliable findings. Additionally, data analysis is influenced by inherent biases, assumptions, and constraints that may impact the validity of the results.
FAQ 8: How can data analysis contribute to decision-making?
Data analysis plays a vital role in decision-making by providing objective and evidence-based insights. It helps decision-makers identify trends, patterns, and relationships, enabling them to make informed choices, address challenges, optimize processes, and achieve desired outcomes.
FAQ 9: Can data analysis be used across different industries?
Absolutely! Data analysis is applicable in various industries, including finance, marketing, healthcare, education, retail, manufacturing, and more. Regardless of the sector, organizations can use data analysis to gain a competitive advantage, improve operations, enhance customer experiences, and drive business growth.
FAQ 10: What are some popular software tools for data analysis?
There are several popular software tools available for data analysis, such as Python (with libraries like Pandas, NumPy, and SciPy), R, SAS, SPSS, MATLAB, Excel (with data analysis add-ins), Tableau, Power BI, and more. These tools provide a wide range of functionalities to aid in data processing, analysis, and visualization.