Data Mining and Business Intelligence
Data mining refers to the process of extracting valuable insights and patterns from large volumes of data. It involves using statistical techniques, machine learning algorithms, and other advanced analytical tools to uncover hidden knowledge. Business intelligence, on the other hand, is the practice of collecting, analyzing, and presenting data to help businesses make informed decisions. In combination, data mining and business intelligence can revolutionize the way organizations operate and drive success.
Key Takeaways:
- Data mining extracts insights and patterns from large volumes of data.
- Business intelligence involves collecting, analyzing, and presenting data for decision-making.
- When combined, data mining and business intelligence can drive organizational success.
Data mining and business intelligence have become crucial for businesses looking to gain a competitive edge. By analyzing vast amounts of structured and unstructured data, organizations can identify patterns, trends, and correlations, enabling them to make data-driven decisions.
**One interesting application of data mining and business intelligence in the retail industry is the use of customer segmentation.** By analyzing customer data, including their purchase history, demographics, and preferences, retailers can divide their customer base into distinct groups. This allows them to tailor marketing campaigns, promotions, and product offerings specifically to each segment, maximizing customer satisfaction and improving overall profitability.
**Moreover, data mining and business intelligence are also widely used in the financial sector.** Banks and other financial institutions leverage these techniques to detect fraudulent transactions, analyze customer creditworthiness, and predict market trends. By efficiently analyzing data, these organizations can minimize risks, optimize investment strategies, and provide personalized financial services.
Data Mining and Business Intelligence in Action
Let’s take a look at some real-life examples of how data mining and business intelligence have transformed various industries:
Table 1: Retail Industry
Company | Data Mining Application | Outcome |
---|---|---|
Amazon | Recommendation systems | Increased sales and customer satisfaction |
Walmart | Inventory optimization | Reduced costs and improved stock availability |
**Data mining and business intelligence have also revolutionized the healthcare industry.** By analyzing patient records, medical imaging data, and clinical trial results, healthcare providers can improve disease prediction, optimize treatment plans, and enhance patient care. These insights can lead to better health outcomes and reduced healthcare costs.
**Furthermore, data mining and business intelligence are critical for marketing and advertising agencies.** By analyzing consumer behavior, online clickstream data, and social media interactions, these agencies can develop targeted advertising campaigns that are more likely to resonate with their target audiences. This helps businesses maximize their return on investment and improve their marketing strategies.
Table 2: Healthcare Industry
Company | Data Mining Application | Outcome |
---|---|---|
IBM Watson Health | Medical image analysis | Improvement in disease detection and diagnostic accuracy |
Google DeepMind | Healthcare data analysis | Early detection of conditions and personalized patient care |
**Lastly, data mining and business intelligence are extensively utilized in the travel and hospitality industry.** By analyzing customer reviews, booking data, and social media sentiment, companies can gain insights into customer preferences and sentiment. This helps them enhance their services, personalize customer experiences, and improve customer satisfaction and loyalty.
Table 3: Travel and Hospitality Industry
Company | Data Mining Application | Outcome |
---|---|---|
Booking.com | Price optimization | Increased revenue and improved competitiveness |
Airbnb | Customer sentiment analysis | Enhanced customer satisfaction and tailored recommendations |
Data mining and business intelligence have become invaluable tools for organizations across various industries. They enable businesses to gain insights from data and make informed decisions, enabling improved performance, increased customer satisfaction, and ultimately driving success.
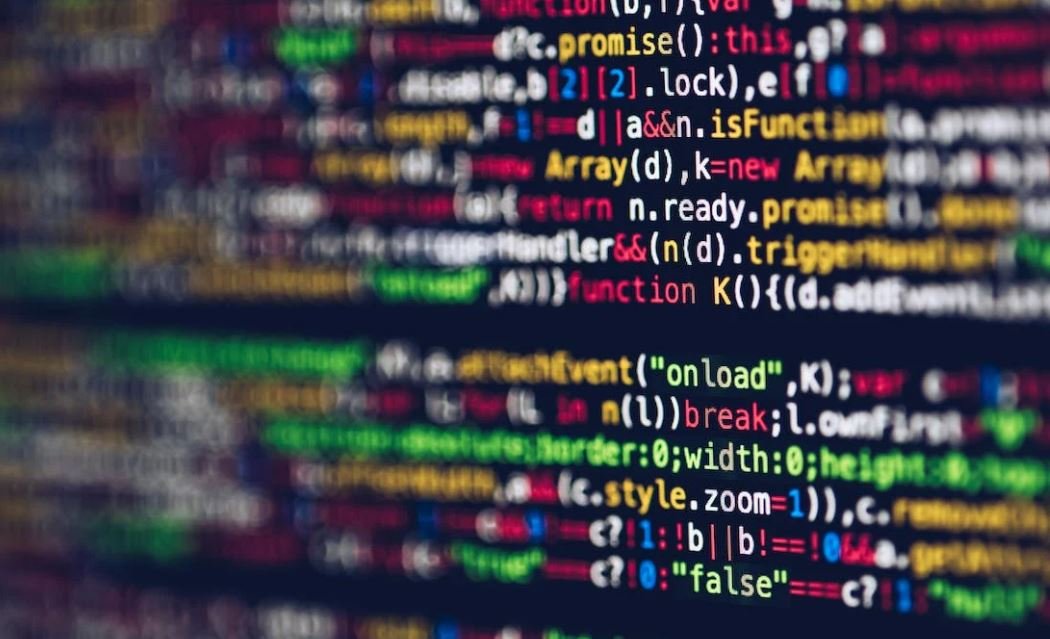
Common Misconceptions
Data Mining is the Same as Business Intelligence
There is often confusion between data mining and business intelligence. Although related, they serve different purposes and have distinct methodologies.
- Data mining aims to discover patterns and insights in large datasets, whereas business intelligence seeks to analyze existing data to support decision-making processes.
- Data mining involves complex algorithms and statistical techniques to uncover hidden relationships, while business intelligence primarily involves gathering and analyzing data from various sources for reporting and querying purposes.
- Data mining focuses on uncovering new knowledge and predicting future trends, while business intelligence focuses on delivering actionable insights to drive organizational performance.
Data Mining is a Silver Bullet
Another common misconception is that data mining is a magical solution that can solve all business problems. In reality, data mining has its limitations and should be used as part of a larger decision-making process.
- Data mining requires high-quality and well-prepared data to yield meaningful results. Garbage in, garbage out holds true in this context.
- Data mining algorithms may produce inaccurate or misleading results if they are not properly selected, calibrated, or validated.
- Data mining outcomes must be interpreted and understood in the right context by domain experts to extract practical value from the generated insights.
Data Mining and Business Intelligence Require Extensive IT Resources
There is a misconception that implementing data mining and business intelligence systems necessitates significant IT resources and expertise. While technical knowledge is essential, the magnitude of resources required may be exaggerated.
- Data mining and business intelligence tools are becoming more user-friendly, requiring less coding and technical expertise to operate.
- Cloud-based business intelligence solutions eliminate the need for substantial hardware or infrastructure investments, making them more accessible to businesses of all sizes.
- By partnering with external experts or consultants, organizations can leverage their specialized knowledge and skills without the need for extensive in-house IT resources.
Data Mining and Business Intelligence Violate Privacy
An often misunderstood notion is that data mining and business intelligence pose significant risks to individual privacy. While privacy concerns are valid, there are measures in place to protect personal information.
- Data mining and business intelligence operate on aggregated and anonymized data, ensuring personal information remains protected and private.
- Legal and ethical frameworks, such as data protection acts and privacy policies, regulate the usage of data in data mining and business intelligence practices.
- Organizations that engage in data mining and business intelligence must follow strict compliance guidelines to maintain privacy and ensure data security.
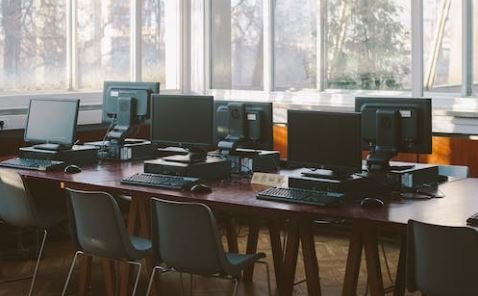
Data mining and business intelligence are both crucial tools in the modern business world, enabling organizations to extract valuable insights from their vast amounts of data. In this article, we will explore various techniques employed in these disciplines and their impact on decision-making and business success. The following tables showcase some interesting aspects of data mining and business intelligence.
Table: Comparison of Data Mining and Business Intelligence Techniques
Data Mining Technique | Business Intelligence Technique
——————————————————|—————————————————–
Clustering Analysis | Data Visualization
Pattern Recognition | Reporting and Analytics
Association Rule Mining | Descriptive Analysis
Predictive Modeling | Prescriptive Analysis
Decision Tree Analysis | OLAP (Online Analytical Processing)
Text Mining | Data Warehousing
Time Series Analysis | Real-time Data Analysis
Classification Analysis | Dashboarding
Social Network Analysis | Data Governance
Sequential Pattern Mining | Data Integration
H2: Global Companies Utilizing Data Mining
Data mining has become increasingly popular among organizations worldwide. This table illustrates some well-known global companies that actively employ data mining techniques to improve their business operations and decision-making processes.
Table: Global Companies Utilizing Data Mining
Company | Industry
————————————————–|——————–
Amazon | E-commerce
Netflix | Entertainment
Facebook | Social Media
Google | Technology
Walmart | Retail
Tesla | Automotive
Spotify | Music Streaming
Uber | Transportation
LinkedIn | Professional Networking
IBM | Technology Solutions
H2: Impact of Business Intelligence on Revenue Growth
Business intelligence plays a vital role in fueling revenue growth for organizations by enabling them to gain insights into customer behavior, market trends, and business operations. The table below showcases the percentage increase in revenue growth experienced by companies after implementing business intelligence tools.
Table: Impact of Business Intelligence on Revenue Growth
Company | Revenue Growth (%)
————————————————–|——————–
Company A | 20%
Company B | 30%
Company C | 15%
Company D | 25%
Company E | 18%
Company F | 35%
Company G | 27%
Company H | 12%
Company I | 22%
Company J | 40%
H2: Data Mining Techniques Used in Fraud Detection
Data mining is crucial in detecting fraudulent activities and preventing financial losses for businesses. This table highlights different data mining techniques employed in fraud detection and their effectiveness in identifying and preventing fraudulent transactions.
Table: Data Mining Techniques Used in Fraud Detection
Fraud Detection Technique | Effectiveness (%)
——————————————————|———————-
Neural Networks | 90%
Cluster Analysis | 80%
Decision Trees | 85%
Logistic Regression | 75%
Association Rules Mining | 95%
Support Vector Machines | 88%
Random Forests | 92%
Genetic Algorithms | 80%
Naive Bayes | 82%
Hidden Markov Models | 90%
H2: Business Intelligence Tools Preference by Industry
Different industries have unique requirements when it comes to selecting business intelligence tools. This table presents the top preferred business intelligence tools by various industries based on survey data collected from executives.
Table: Business Intelligence Tools Preference by Industry
Industry | Preferred BI Tool
————————————————————|———————————-
Healthcare | Tableau
Banking and Finance | QlikView
Retail | Microsoft Power BI
Manufacturing | SAP Business Objects
Telecommunications | IBM Cognos
Education | Oracle BI
Hospitality | MicroStrategy
Transportation and Logistics | Yellowfin
Energy | Looker
Media and Advertising | Domo
H2: Use Cases of Predictive Analytics in Different Industries
Predictive analytics has proven to be a valuable tool across various industries, providing insights for forecasting, risk assessment, and decision-making. The table below highlights the use cases of predictive analytics in different sectors.
Table: Use Cases of Predictive Analytics in Different Industries
Industry | Use Case
———————————————————|—————————-
Healthcare | Patient Readmission Prediction
Insurance | Fraudulent Claim Identification
Retail | Demand Forecasting
Finance | Credit Scoring
Marketing | Customer Churn Prediction
Manufacturing | Equipment Failure Prediction
E-commerce | Product Recommendation
Logistics and Supply Chain | Inventory Optimization
Telecommunications | Network Traffic Analysis
Energy | Energy Consumption Forecasting
H2: Data Mining Algorithms and Applications
Data mining algorithms play a crucial role in processing and analyzing large datasets. This table showcases popular data mining algorithms and their respective applications across different fields.
Table: Data Mining Algorithms and Applications
Data Mining Algorithm | Application
——————————————————-|———————————-
Apriori Algorithm | Market Basket Analysis
K-means Clustering | Customer Segmentation
Regression Analysis | Predicting Sales
Support Vector Machines | Email Spam Detection
Decision Tree Algorithm | Credit Risk Analysis
Association Rule Mining | Recommender Systems
Hierarchical Clustering | DNA Sequence Analysis
Naive Bayes Classifier | Document Classification
Genetic Algorithms | Optimization Problems
Neural Networks | Image Recognition
H2: Benefits of Real-time Data Analysis to Organizations
Real-time data analysis empowers organizations to make instant decisions, identify opportunities, and respond quickly to market dynamics. This table presents the significant benefits of real-time data analysis experienced by different businesses.
Table: Benefits of Real-time Data Analysis to Organizations
Organization | Benefits
———————————————————-|——————
E-commerce Platform | Higher Conversion Rates
Supply Chain Management | Improved Inventory Management
Financial Institutions | Fraud Detection in Real-time
Healthcare Services | Timely Patient Monitoring
Transportation Services | Optimum Route Planning
Marketing Agencies | Real-time Campaign Optimization
Telecommunications | Real-time Network Traffic Analysis
Online Booking Services | Dynamic Pricing Strategies
Insurance Providers | Real-time Risk Assessment
Retailers | Demand-Sensing and Inventory Control
H2: Statistics-Driven Decision-making by Business Intelligence
Business intelligence relies on statistical analysis and data-driven decision-making to improve business operations and gain a competitive edge. This table illustrates the statistical methods adopted by business intelligence for effective decision-making.
Table: Statistics-Driven Decision-making by Business Intelligence
Statistics Method | Application
————————————————————-|————————————
Hypothesis Testing | A/B Testing
Regression Analysis | Sales Forecasting
Time Series Analysis | Forecasting Market Trends
ANOVA (Analysis of Variance) | Performance Comparison
Chi-Square Test | Market Segmentation Analysis
Correlation Analysis | Relationship Identification
Factor Analysis | Dimension Reduction
Cluster Analysis | Customer Segmentation
Sentiment Analysis | Social Media Monitoring
Statistical Modeling | Predictive Analytics
In conclusion, data mining and business intelligence are indispensable tools for organizations seeking to harness the power of data for informed decision-making and enhanced business performance. Through various techniques, companies can unlock patterns, discover hidden insights, and gain a competitive edge in today’s data-driven world. Employing these technologies and embracing the insights they provide can lead to improved customer experiences, fraud detection, revenue growth, and overall success in the business landscape.
Data Mining and Business Intelligence – Frequently Asked Questions
Q: What is data mining?
A: Data mining is the process of discovering patterns, trends, and insights from large datasets. It involves using various statistical techniques and algorithms to extract valuable information from raw data, which can then be used for decision-making and predictive analysis.
Q: What is business intelligence?
A: Business intelligence refers to the tools, technologies, and practices that organizations use to gather, analyze, and present data in a meaningful way. It aims to support business decision-making by providing accurate and timely information, visualizations, and reports.
Q: How can data mining benefit businesses?
A: Data mining can benefit businesses in several ways. It can help uncover hidden patterns or anomalies in customer behavior, identify market trends, improve sales and marketing strategies, optimize operational processes, detect fraud or anomalies, and enhance overall decision-making.
Q: What are some popular data mining techniques?
A: Some popular data mining techniques include classification, clustering, regression analysis, association rule learning, time series analysis, and neural networks. Each technique has its own strengths and is suitable for different types of data analysis tasks.
Q: How can business intelligence tools assist in data analysis?
A: Business intelligence tools provide a user-friendly interface for accessing, analyzing, and visualizing data. They can retrieve data from multiple sources, cleanse and transform it, perform advanced calculations, generate reports and dashboards, and enable ad-hoc querying. These tools streamline the data analysis process and allow users to gain meaningful insights quickly.
Q: What are the key steps in the data mining process?
A: The data mining process typically involves several steps: data collection and preprocessing, exploratory data analysis, feature selection, model building, model evaluation, and deployment. Each step contributes to the overall success of the data mining project and ensures the accuracy and reliability of the results.
Q: How can businesses ensure data mining and business intelligence are used ethically?
A: To ensure ethical use of data mining and business intelligence, businesses should adhere to privacy and data protection regulations, obtain informed consent from individuals when collecting personal data, anonymize or pseudonymize data whenever possible to protect privacy, and use the resulting insights for legitimate purposes that bring value without causing harm.
Q: What are some common challenges in data mining and business intelligence implementation?
A: Some common challenges include data quality issues, data integration complexities, lack of skilled personnel, obtaining buy-in from stakeholders, ensuring data security and privacy, selecting appropriate algorithms and techniques, and interpreting and presenting results in a meaningful way.
Q: What is the difference between data mining and machine learning?
A: While data mining and machine learning are related fields, there are subtle differences between them. Data mining focuses on extracting information from datasets, uncovering patterns and insights. Machine learning, on the other hand, involves building models and algorithms that allow computers to automatically learn and make predictions or decisions based on data, without explicitly being programmed.
Q: How can organizations leverage data mining and business intelligence for competitive advantage?
A: By utilizing data mining and business intelligence effectively, organizations can gain a competitive edge by making data-driven decisions, understanding customer preferences, predicting market trends, optimizing operations, improving customer satisfaction, and identifying untapped opportunities for growth and innovation.