Data Mining Bias
As our world becomes increasingly data-driven, it’s important to understand the potential biases that can arise in data mining. Data mining is the process of analyzing large sets of data to uncover patterns, relationships, and insights. However, if not performed carefully, data mining can unintentionally introduce bias into the results, leading to skewed or inaccurate conclusions. In this article, we will explore the concept of data mining bias, its causes, and its implications.
Key Takeaways:
- Data mining can introduce bias into the results, skewing the analysis.
- Biases can arise due to imperfect data collection, sample selection, or algorithms.
- Data mining bias can have significant implications, including perpetuating inequality and discrimination.
Causes of Data Mining Bias
Data mining bias can arise from various sources, including:
- Imperfect Data Collection: If the data collected is incomplete, contains errors, or is skewed towards certain demographics, it can introduce bias into the analysis.
- Sample Selection Bias: If the sample used for data mining does not accurately represent the target population or is disproportionately weighted towards certain groups, the results will reflect the biases present in the sample.
- Algorithmic Bias: Algorithms used in data mining can be biased if they are trained on biased data or designed with certain assumptions that may not hold true in all contexts.
Identifying and addressing data mining bias is crucial to ensure fair and accurate analyses.
Implications of Data Mining Bias
Data mining bias can have far-reaching implications, including:
- Perpetuating Inequality: Biased data mining can reinforce existing inequalities by favoring certain groups or discriminating against others.
- Unfair Decision-Making: If data mining is used to inform decision-making processes, biased results can lead to unfair outcomes, such as in hiring or loan approvals.
- Eroding Trust: The presence of bias in data mining undermines trust in the results and the organizations performing the analysis.
Data Mining Bias in Practice
Let’s take a closer look at some real-world examples of data mining bias:
Example | Description |
---|---|
Gender Pay Gap Analysis | A study that analyzes salary data may overlook factors contributing to the gender pay gap, such as societal biases and occupational segregation. |
Racial Profiling | Data mining used in law enforcement can inadvertently target certain racial or ethnic groups due to biased data or algorithms. |
Addressing Data Mining Bias
Awareness and proactive steps can help address data mining bias:
- Data Audit: Conduct regular audits to assess the quality and bias potential of the data used in data mining.
- Transparent Documentation: Document the data collection and analysis process to identify potential sources of bias and promote transparency.
- Diverse Representation: Ensure diverse representation within the data mining team to minimize biases and consider different perspectives.
Solutions | Description |
---|---|
Algorithmic Fairness | Develop algorithms that account for biases and are designed to mitigate bias in their decision-making processes. |
Regular Evaluation | Continuously evaluate and monitor the data mining process for potential biases and make necessary adjustments. |
Data mining bias is a critical issue that deserves attention and action. By recognizing and addressing biases in data mining, we can strive for fairer and more accurate analyses, promoting equality and trust in data-driven decision making.
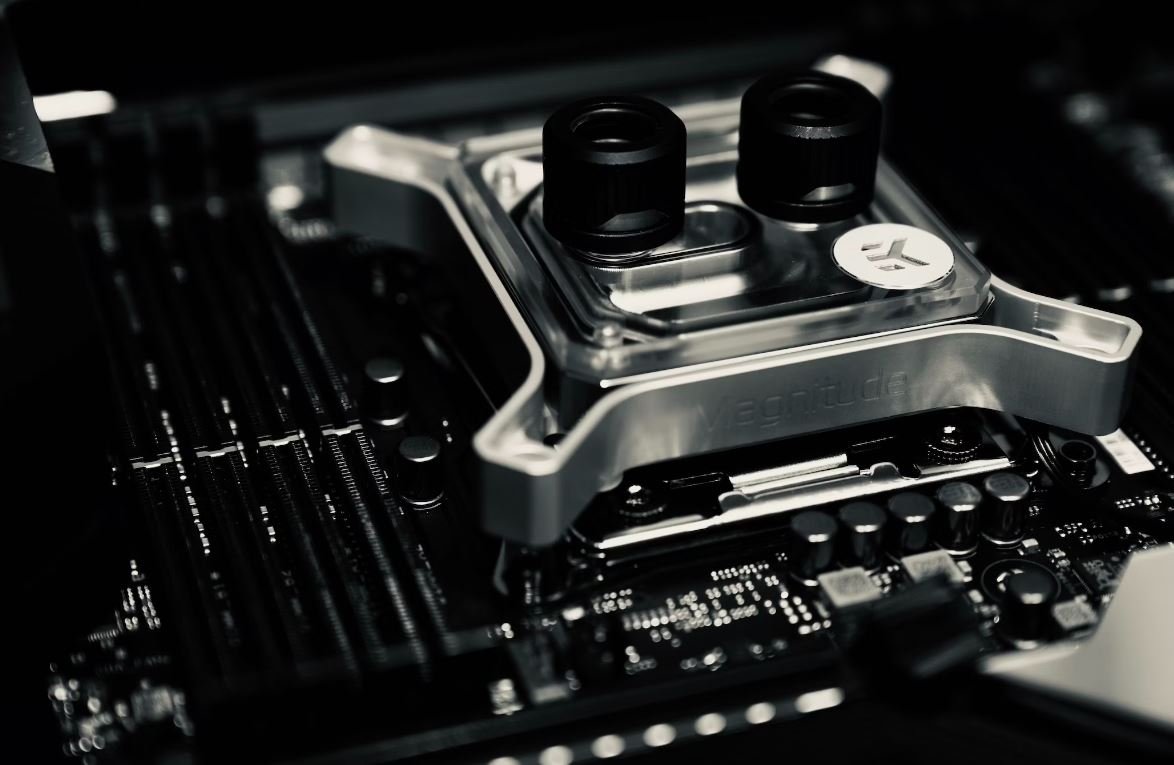
Common Misconceptions
Data Mining Bias
There are several common misconceptions surrounding data mining bias that people often have. One of the most prevalent misconceptions is that data mining is an entirely objective process that produces unbiased results. However, data mining is heavily influenced by the data that is input into the system, and if the data itself is biased, the results will be biased as well.
- Data mining results are influenced by the quality and relevance of the input data.
- Data mining algorithms can magnify existing biases present in the data.
- Data mining bias can lead to inaccurate predictions and unfair outcomes.
Another common misconception is that data mining bias is solely the result of intentional discrimination or prejudice. While intentional bias can certainly be a cause, there are also many instances where bias emerges unintentionally due to factors such as incomplete or incorrect data, sampling bias, or inherent limitations of the algorithms used.
- Data mining bias can occur unintentionally due to incomplete or incorrect data.
- Sampling bias can introduce bias into the data mining process.
- Limitations of the algorithms used in data mining can contribute to bias.
Some people also mistakenly believe that bias in data mining can be completely eliminated or neutralized. While efforts can be made to minimize bias, it is often difficult to completely eliminate it. Bias can be deeply ingrained in the data itself or in the systems and processes that produce the data. Additionally, the algorithms used in data mining can introduce their own biases, making complete neutrality challenging to achieve.
- Minimizing bias in data mining is possible, but complete elimination is challenging.
- Data and system biases can persist even with mitigation efforts.
- Data mining algorithms themselves may introduce biases that are difficult to neutralize.
Another misconception is that data mining bias only affects certain groups or individuals, particularly those who are traditionally disadvantaged or marginalized. In reality, bias in data mining can impact all individuals and groups, regardless of their background. Data mining bias has the potential to perpetuate existing inequalities and reinforce discriminatory practices, regardless of who is being targeted.
- Data mining bias can impact all individuals and groups, not just specific demographics.
- Data mining bias can perpetuate existing inequalities and discriminatory practices.
- All users and stakeholders can be affected by bias in data mining processes.
Lastly, there is a misconception that data mining bias is a rare occurrence. However, bias in data mining is prevalent and can be found in various domains such as law enforcement, healthcare, finance, and hiring practices. The increasing reliance on data-driven decision-making makes it crucial to address and mitigate bias in data mining to ensure fair and equitable outcomes for all individuals.
- Data mining bias is present in numerous domains, including law enforcement, healthcare, finance, and hiring practices.
- Addressing bias in data mining is crucial due to its prevalence in decision-making processes.
- Data mining bias can undermine the fairness and equity of outcomes.
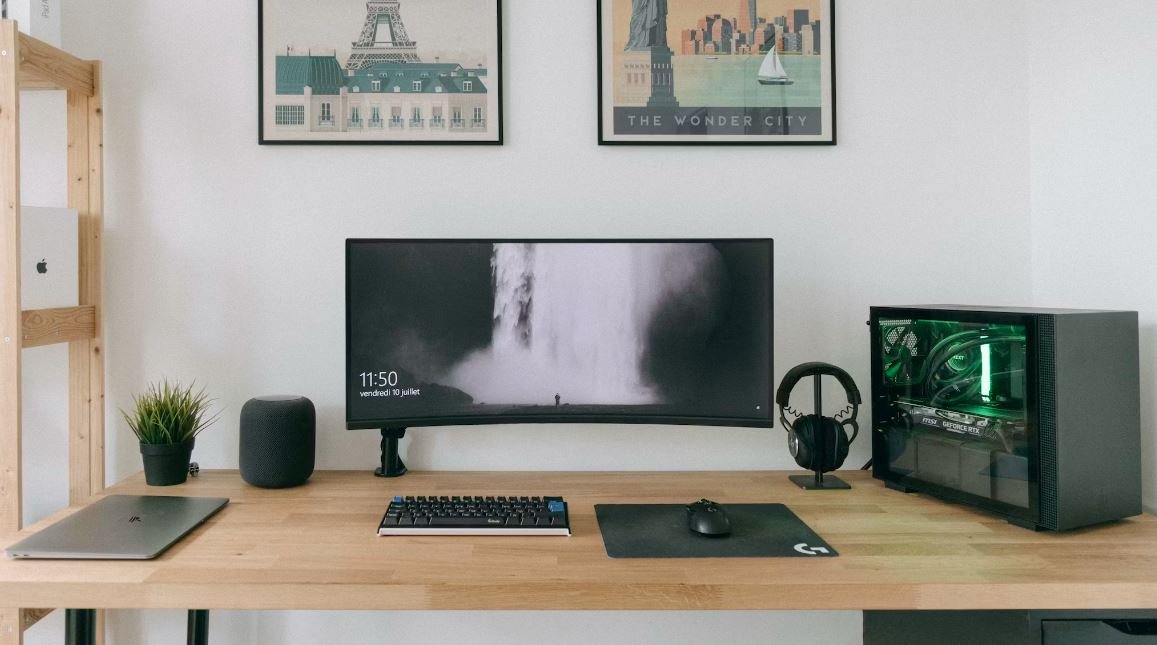
Introduction
Data mining is a powerful tool that allows us to extract valuable insights from large datasets to make informed decisions. However, it is important to be aware of the biases that can be inherent in the data and potential pitfalls of data mining. In this article, we explore 10 intriguing tables that highlight the presence of bias in data mining and shed light on the need for a well-rounded approach in analyzing and interpreting data.
Table 1: Representation of Gender in Tech Companies
This table presents the gender distribution across various tech companies, showing the percentage of male and female employees. It highlights the significant lack of female representation in the industry, calling attention to possible biases in hiring practices.
Table 2: Historical Election Results by Political Party
Displaying the historical election results by political party, this table demonstrates the popularity of certain parties over time. It raises the question of whether the data truly reflects the public’s choice, as the outcome may be influenced by factors such as media coverage and campaign funds.
Table 3: Average Income Levels by Ethnicity
Examining the average income levels across different ethnicities, this table gives insight into income disparities that may perpetuate biases and inequalities. It emphasizes the importance of accounting for socio-economic factors when analyzing data.
Table 4: Sentencing Disparities by Offense Type
Illustrating the sentencing disparities for various offense types, this table brings attention to potential biases within the criminal justice system. It prompts a critical examination of the fairness and impartiality of sentencing decisions across different groups.
Table 5: Ranking of News Outlets by Political Bias
Ranking news outlets based on political bias, this table reveals the extent to which media sources may shape public opinion. It highlights the need to approach information from diverse sources to avoid being influenced by a single perspective.
Table 6: Representation of Body Types in Fashion Advertising
Showcasing the representation of body types in fashion advertising, this table exposes the narrow standards of beauty perpetuated by the industry. It calls for a more inclusive and representative portrayal of diverse body shapes to combat biases in beauty standards.
Table 7: Research Funding Allocation by Topic
Presenting research funding allocation by topic, this table draws attention to potential biases in scientific research. It raises the question of whether certain areas of study receive more funding, leading to an imbalance in knowledge advancement.
Table 8: Student Performance by Socioeconomic Background
Comparing student performance based on socioeconomic background, this table highlights the influence of family income on educational outcomes. It underscores the importance of equal access to resources and opportunities to counteract systemic biases.
Table 9: Availability of Healthcare Services by Geographical Location
This table reveals the availability of healthcare services based on geographical location, exposing potential biases in access to quality healthcare. It emphasizes the need for equitable healthcare distribution to address disparities in well-being.
Table 10: Customer Purchase Patterns by Advertising Medium
Examining customer purchase patterns based on advertising medium, this table demonstrates the effectiveness of various marketing channels. It encourages businesses to be mindful of potential biases when targeting specific demographic groups, ensuring fair representation of all potential customers.
Conclusion
These 10 tables provide compelling evidence of the presence of biases in data mining and its impact across various domains. By being aware of these biases, we can ensure that data-driven decisions and policies are implemented responsibly. It is crucial to critically examine data sources, question underlying assumptions, and consider diverse perspectives to minimize biases and promote fairness in our analyses. Only through a rigorous and inclusive approach can we make meaningful progress towards a more equitable and unbiased society.
Frequently Asked Questions
What is data mining bias?
Data mining bias refers to the systematic errors or prejudices that occur during the data mining process, resulting in biased or skewed outcomes. Bias can occur at various stages, including data selection, preprocessing, algorithmic design, and interpretation of results.
How does data mining bias affect decision-making?
Data mining bias can have significant consequences on decision-making processes. Biased data can lead to inaccurate insights, discriminatory patterns, and unfair or biased decisions. This can perpetuate and reinforce existing biases present in the data, resulting in negative impacts on individuals or groups.
What are the common sources of data mining bias?
Data mining bias can arise from various sources, including unrepresentative or incomplete datasets, biased sampling techniques, algorithmic biases, human biases in feature selection or labeling, and lack of diversity in data sources. These sources can introduce biases that reflect socially constructed inequities, leading to biased outcomes.
How can data mining bias be mitigated?
To mitigate data mining bias, several strategies can be employed. These include ensuring diverse and representative datasets, using fair and unbiased sampling techniques, identifying and addressing algorithmic biases, involving domain experts in data preprocessing, providing transparency and interpretability of algorithms, and employing fairness-aware evaluation metrics.
Can data mining techniques contribute to reducing bias?
Data mining techniques can play a role in reducing bias by enabling the identification and mitigation of biases in datasets and algorithms. Fairness-aware machine learning algorithms and techniques, such as inverse propensity weighting and fair data preprocessing, can help in addressing bias and promoting fairness in decision-making processes.
What are some real-world examples of data mining bias?
Real-world examples of data mining bias include gender or racial biases in hiring algorithms, biased loan approval systems that disproportionately affect certain groups, facial recognition systems that exhibit racial biases, and recommendation algorithms that perpetuate stereotypes. These examples highlight the potential harm caused by biased data mining practices.
How does data mining bias relate to ethics and fairness?
Data mining bias is closely linked to ethics and fairness in decision-making. Biased outcomes violate principles of fairness, equal opportunity, and non-discrimination. Detecting and addressing data mining bias is essential to ensure that algorithms and models do not perpetuate or amplify societal inequities.
What role do data scientists play in addressing data mining bias?
Data scientists have a crucial role in addressing data mining bias. They are responsible for understanding and mitigating biases throughout the data mining process. This involves being aware of biases, implementing fair data collection and preprocessing methods, selecting unbiased algorithms, and rigorously evaluating the impact of data mining on fairness and ethics.
Are there legal implications of data mining bias?
There can be legal implications resulting from data mining bias. Discrimination based on protected characteristics, such as race or gender, can lead to violations of anti-discrimination laws. In certain domains, biased decision-making systems may face legal challenges and sanctions for their discriminatory outcomes.
How can individuals protect themselves from data mining bias?
Individuals can take several steps to protect themselves from data mining bias. It includes being aware of the potential biases present in automated decision-making systems, advocating for transparency and fairness in algorithmic systems, and demanding accountability and regulations to prevent discriminatory practices.