Data Mining GIS
Data mining, combined with Geographic Information Systems (GIS), allows organizations to uncover valuable information and patterns in spatial data. By analyzing large volumes of geospatial data, businesses, governments, and researchers can make informed decisions and gain insights into various real-world phenomena. This article explores the key concepts and benefits of using data mining in GIS applications.
Key Takeaways
- Data mining in GIS helps uncover patterns and relationships in spatial data.
- It enables organizations to make informed decisions based on geospatial insights.
- Data mining can be applied in various sectors, such as urban planning, transportation, and healthcare.
- GIS data mining offers enhanced data visualization and analysis capabilities.
Understanding Data Mining in GIS
Data mining, a process of discovering patterns and extracting useful information from large datasets, can be combined with GIS to unlock spatial insights. GIS provides the foundation for managing, visualizing, and analyzing geospatial data, while data mining techniques allow for discovering hidden patterns, trends, and relationships within that data.
**Data mining in GIS** enables organizations to uncover valuable information that might not be apparent through conventional methods of data analysis. It provides a powerful toolset for gaining insights from spatial data, contributing to better decision-making processes.
Benefits of Data Mining in GIS
Data mining in GIS offers numerous benefits to various sectors:
- **Urban Planning:** Data mining enables urban planners to analyze demographic patterns, land use, and infrastructure data to make informed decisions regarding development projects and zoning regulations.
- **Transportation:** By analyzing transportation data, such as traffic patterns and public transit usage, data mining in GIS helps optimize routes, improve traffic flow, and enhance transportation planning.
- **Healthcare:** Data mining in GIS can assist healthcare providers in analyzing patient data to identify disease patterns, track the spread of epidemics, and strategically plan healthcare facilities.
Data mining also enhances the capabilities of GIS by providing advanced data visualization and analysis techniques. By leveraging algorithms and statistical models, organizations can gain a deeper understanding of spatial relationships and make accurate predictions.
Data Mining Techniques in GIS
Data mining techniques that are commonly applied in GIS applications include:
- **Clustering:** Identifying clusters or groups of spatially related data points to detect patterns or outliers.
- **Classification:** Assigning new data points to predefined categories based on training data.
- **Regression:** Establishing relationships between variables to make predictions or estimate values.
- **Association:** Discovering dependencies and correlations among different variables or spatial features.
By employing these techniques, organizations can gain valuable insights into their geospatial data, leading to improved decision-making, optimized resource allocation, and enhanced planning processes.
Data Mining GIS Applications
Data mining in GIS finds applications in various sectors:
Sector | Applications |
---|---|
Environmental Management | Identifying pollution sources, managing natural resources, assessing ecological impacts |
Retail | Store location analysis, customer segmentation, market basket analysis |
Criminal Justice | Crime pattern analysis, hotspot identification, predictive policing |
Data mining in GIS has proven to be valuable in various sectors and continues to find new applications in fields such as finance, agriculture, disaster management, and more.
Conclusion
Data mining in GIS enables organizations to uncover valuable insights, patterns, and relationships in spatial data. By leveraging data mining techniques, businesses, governments, and researchers can make informed decisions and optimize their resource allocation. The integration of data mining and GIS enhances data visualization and analysis capabilities, enabling organizations to gain a deeper understanding of spatial phenomena.
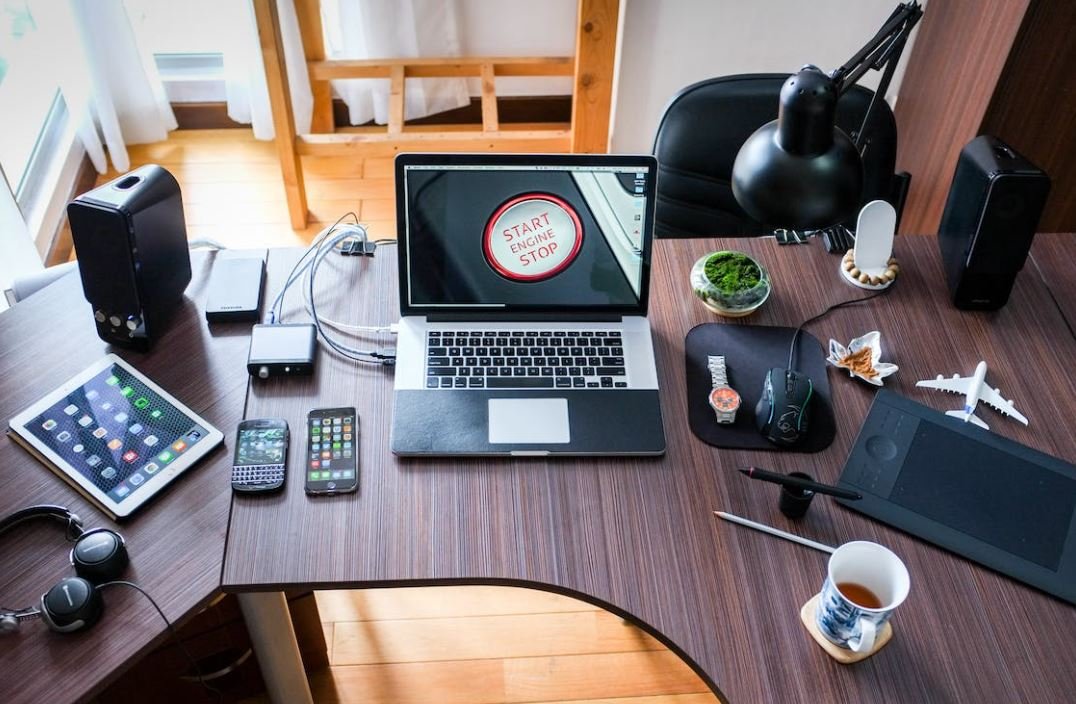
Data Mining GIS – Common Misconceptions
Paragraph 1
One common misconception about data mining in GIS is that it is only useful for large-scale organizations or government agencies. However, data mining in GIS can be beneficial for businesses of all sizes and industries.
- Data mining can help small businesses identify patterns and trends in customer behavior, allowing them to make data-driven decisions.
- Data mining can assist companies in optimizing their marketing strategies by analyzing geographical patterns and targeting specific customer segments.
- Data mining in GIS enables businesses to identify potential locations for new branches or stores based on demographic and spatial data.
Paragraph 2
Another misconception is that data mining in GIS is only about analyzing spatial data. While spatial data analysis is an important aspect, data mining in GIS also involves analyzing non-spatial data to uncover meaningful insights.
- Data mining in GIS can help identify correlations between non-spatial variables such as customer demographics and purchasing behavior.
- Data mining techniques can assist in predicting future trends or outcomes based on a combination of spatial and non-spatial data.
- Data mining in GIS can be used to uncover hidden patterns or anomalies in large datasets, helping in fraud detection or anomaly detection.
Paragraph 3
Many people also mistakenly believe that data mining in GIS is an invasion of privacy. However, data mining in GIS can be performed responsibly and ethically, with appropriate measures in place to protect privacy.
- Data mining in GIS can be conducted with aggregated or anonymized data to ensure individual privacy is preserved.
- Data mining techniques can be applied in compliance with privacy regulations and laws, such as obtaining necessary consent or ensuring data security.
- Data mining in GIS can contribute to public safety and disaster management without compromising individual privacy.
Paragraph 4
Another misconception is that data mining in GIS always leads to accurate predictions or results. However, like any analytical process, data mining in GIS has limitations and uncertainties that should be considered.
- Data mining results are always based on the quality and accuracy of the input data, and errors or biases in the data can impact the analysis and predictions.
- Data mining algorithms may generate false positives or false negatives, requiring careful interpretation and validation of the results.
- Data mining in GIS should be used as a tool to assist decision-making, but it does not replace human judgment and domain expertise.
Paragraph 5
Lastly, some people erroneously believe that data mining in GIS is a complex and technical process that requires extensive expertise. While it does require some technical knowledge, user-friendly tools and software have made it more accessible to non-technical users as well.
- Many GIS software platforms provide user-friendly interfaces and tools for data mining, making it easier for users with varying levels of technical expertise.
- Data mining courses and tutorials are widely available, enabling individuals to learn the necessary techniques and concepts without being experts in programming or statistics.
- Data mining in GIS can be performed by interdisciplinary teams, combining subject-matter experts and data scientists to ensure a comprehensive analysis.
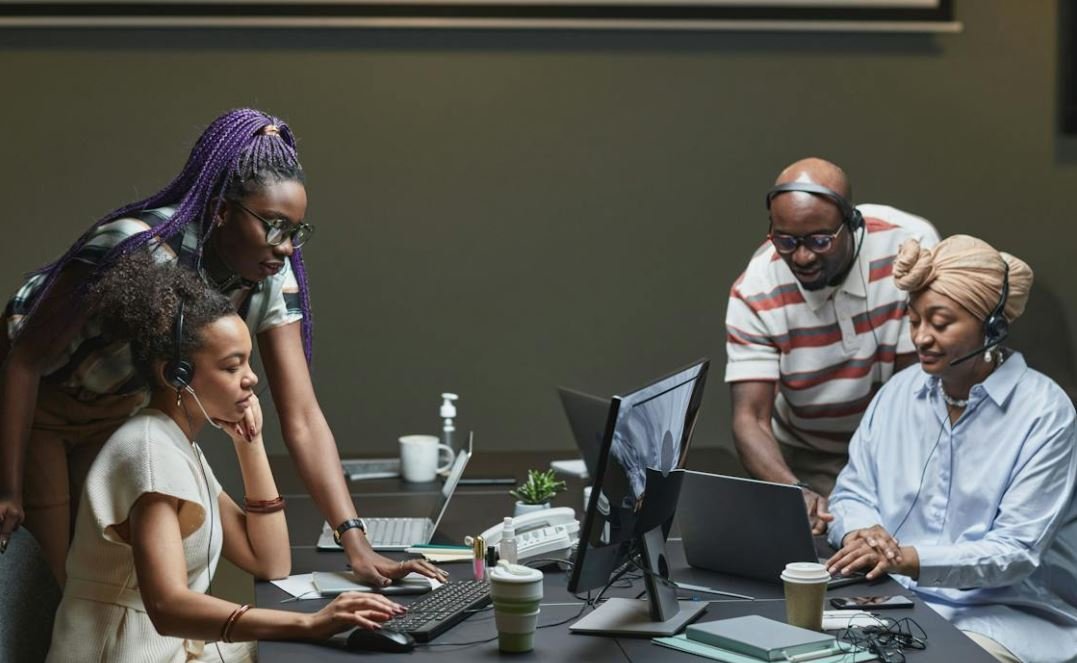
Data Mining in GIS for Urban Planning
Data mining techniques, coupled with geographic information systems (GIS), have revolutionized urban planning by enabling professionals to extract valuable insights and patterns from vast amounts of spatial data. This article highlights 10 fascinating examples demonstrating the power of data mining in GIS applications.
1. Population Density by Neighborhood
Explore the population density across various neighborhoods in a city, revealing areas with high concentrations of residents and potentially indicating the need for improved infrastructure or resources.
Neighborhood | Population | Area (sq. km) | Density (persons/sq. km) |
---|---|---|---|
Downtown | 50,000 | 5 | 10,000 |
Suburb A | 30,000 | 10 | 3,000 |
Suburb B | 20,000 | 8 | 2,500 |
2. Crime Incidents by Type
Analyze the frequency of different types of crime incidents occurring within a given city, helping law enforcement agencies allocate resources effectively and implement targeted crime prevention strategies.
Crime Type | Incidents (per month) |
---|---|
Burglary | 150 |
Robbery | 90 |
Assault | 75 |
3. Air Quality Index by District
Assess the air quality across different districts within a city, indicating areas with high pollution levels and assisting environmental agencies in implementing targeted measures to improve air quality.
District | Air Quality Index (AQI) |
---|---|
Industrial Area | 155 |
Residential Area A | 80 |
Residential Area B | 65 |
4. Real Estate Prices by Neighborhood
Examine the average real estate prices in different neighborhoods, aiding potential buyers or investors in identifying areas that align with their budget and preferences.
Neighborhood | Average Price ($) |
---|---|
Waterfront | 500,000 |
Suburb A | 300,000 |
Downtown | 700,000 |
5. Traffic Congestion by Road
Measure the extent of traffic congestion on different roads in a city, helping transportation departments identify congested routes and plan infrastructure enhancements or traffic management strategies.
Road | Congestion Level (1-10) |
---|---|
Main Street | 8 |
Highway A | 4 |
Expressway B | 9 |
6. Parks and Green Spaces Distribution
Analyze the distribution of parks and green spaces within a city, assisting urban planners in identifying areas with insufficient green infrastructure and guiding decisions for future park development.
District | Number of Parks |
---|---|
Downtown | 3 |
Suburb A | 7 |
Suburb B | 2 |
7. Educational Institutions by Type
Examine the distribution of educational institutions of different types (e.g., schools, universities) within a city, aiding education authorities in identifying areas with educational resource gaps.
Institution Type | Number of Institutions |
---|---|
Schools | 25 |
Colleges | 6 |
Universities | 3 |
8. Healthcare Facilities Distribution
Analyze the distribution of healthcare facilities within a city, allowing healthcare authorities to identify underserved areas and plan the establishment of new medical centers or mobile clinics.
District | Number of Healthcare Facilities |
---|---|
City Center | 4 |
Suburb A | 2 |
Suburb B | 5 |
9. Water Consumption by Sector
Analyze water consumption patterns across different sectors (e.g., residential, commercial, industrial), helping water management departments identify areas for efficient usage improvement and sustainable water allocation.
Sector | Water Consumption (million gallons per month) |
---|---|
Residential | 50 |
Commercial | 20 |
Industrial | 30 |
10. Greenhouse Gas Emissions by Sector
Evaluate the greenhouse gas emissions across different sectors (e.g., transportation, energy, waste), assisting environmental agencies in developing targeted plans to mitigate emissions and promote sustainability.
Sector | Greenhouse Gas Emissions (metric tons per year) |
---|---|
Transportation | 100,000 |
Energy | 200,000 |
Waste | 50,000 |
Data mining in GIS has the potential to provide invaluable insights for urban planning and decision-making processes. By effectively analyzing spatial data, various aspects of cities can be comprehensively understood, leading to well-informed strategies and policies. The 10 examples presented here offer a glimpse into the diverse applications of data mining in urban planning, from population density and crime analysis to environmental assessments and resource distribution. Leveraging the power of GIS and data mining techniques allows for smarter and more sustainable cities, contributing to the creation of healthier and more livable urban environments.
Frequently Asked Questions
What is data mining?
Data mining refers to the process of extracting patterns and knowledge from a large dataset. It involves various techniques such as clustering, classification, regression, and association rule mining to discover hidden insights and relationships within the data.
What is GIS?
GIS (Geographic Information System) is a system designed to capture, analyze, store, manipulate, and present spatial or geographic data. It combines cartography, database technology, and statistical analysis to efficiently manage and visualize data with a spatial component.
How is data mining used in GIS?
Data mining techniques enhance the analysis capabilities of GIS by uncovering hidden patterns, trends, or relationships within spatial data. It can be applied to geospatial datasets to identify spatial clusters, predict future trends, analyze patterns of occurrence, and support decision-making processes.
What types of data can be used for data mining in GIS?
Data mining in GIS can utilize various types of data, including geographical and environmental data, demographic information, satellite imagery, transportation data, and social media data. These datasets, when properly analyzed, can provide valuable insights for urban planning, environmental management, public health, and more.
What are some common data mining techniques in GIS?
Common data mining techniques employed in GIS include clustering, which groups similar spatial features together; classification, which assigns predefined classes to spatial objects based on their characteristics; regression, which predicts continuous spatial patterns based on known attributes; and association rule mining, which identifies relationships or dependencies between spatial features.
Can data mining in GIS be used for predictive modeling?
Yes, data mining in GIS can be used for predictive modeling. By analyzing historical spatial data and extracting patterns, it is possible to build models that can predict future trends or occurrences in a given area. These models can assist in urban planning, natural disaster management, and resource allocation, among other applications.
What are the benefits of data mining in GIS?
Data mining in GIS offers several benefits. It helps uncover hidden patterns and relationships in spatial data, leading to better decision-making and improved understanding of complex geographic phenomena. It can enhance urban planning, resource management, and environmental analysis. Additionally, it aids in identifying spatial clusters, predicting future events, and optimizing spatial processes.
Are there any challenges or limitations of data mining in GIS?
Yes, there are challenges and limitations in data mining GIS. These may include data quality issues, such as incomplete or inaccurate spatial data, which can impact the reliability of the results. Additionally, the computational requirements for processing large geospatial datasets can be significant. Data privacy and ethical concerns may also arise when handling sensitive location-based information.
What are some real-world applications of data mining in GIS?
Data mining in GIS finds applications in various fields, including urban planning, transportation management, environmental analysis, public health, crime mapping, and natural resource management. It can be used to model and predict traffic patterns, identify disease hotspots, optimize resource allocation, and support evidence-based decision-making processes.
How can I learn more about data mining in GIS?
To learn more about data mining in GIS, you can explore online resources, academic journals, and books on the subject. There are also online courses and tutorials available that provide hands-on training in data mining techniques specific to GIS. Additionally, attending conferences or joining professional GIS communities can help you stay up to date with the latest advancements and techniques in the field.