Data Mining in Business
Data mining plays a crucial role in today’s business landscape, helping companies extract valuable insights and patterns from large amounts of data. By analyzing trends and patterns, businesses can make informed decisions and gain a competitive edge. In this article, we will explore the concept of data mining and its applications in various industries.
Key Takeaways:
- Data mining is a process of uncovering patterns and extracting useful insights from large datasets.
- It helps businesses make informed decisions based on data-driven analysis.
- Data mining is used in various industries, including finance, marketing, healthcare, and manufacturing.
Understanding Data Mining
Data mining involves using advanced algorithms and statistical techniques to analyze data and discover patterns, correlations, and relationships that may not be immediately apparent. It allows businesses to gain valuable insights and make predictions based on historical data. *Data mining algorithms can handle complex datasets, including structured, unstructured, and semi-structured data.
Applications of Data Mining in Business
Data mining has numerous applications across industries:
- In finance: Data mining helps financial institutions detect fraudulent activities, analyze market trends, and identify potential investment opportunities.
- In marketing: It aids in customer segmentation, target marketing, and personalized recommendations based on past purchasing behavior.
- In healthcare: Data mining assists in analyzing patient records, identifying disease patterns, and improving treatment outcomes.
- In manufacturing: It optimizes production processes, predicts equipment failures, and improves supply chain management.
Data Mining Techniques
There are several data mining techniques used to uncover patterns and relationships within datasets:
- Classification: This technique categorizes data into predefined classes or groups based on specific attributes.
- Regression: It helps predict a numerical value based on other variables, allowing businesses to forecast trends and analyze relationships.
- Clustering: Clustering divides data into groups based on their similarities, enabling businesses to identify hidden patterns and segment customers.
- Association: This technique identifies relationships between different variables, such as items frequently purchased together.
Data Mining Challenges
Despite its numerous benefits, data mining also faces several challenges:
- Enormous amount of data: With the ever-increasing volume of data being generated, handling and processing vast datasets can be time-consuming and resource-intensive.
- Data quality: Poor data quality, including errors, inconsistencies, and missing values, can negatively impact the accuracy and reliability of the insights derived.
- Privacy concerns: Businesses need to ensure the ethical and secure use of customer data, respecting privacy regulations and protecting sensitive information.
Data Mining Examples
Let’s look at some real-world examples of data mining applications:
Industry | Application |
---|---|
Retail | Market basket analysis to identify product co-occurrence and optimize store layouts. |
Telecommunications | Churn analysis to predict customer attrition and tailor retention strategies. |
Banking | Credit scoring models to determine creditworthiness and assess default risk. |
Industry | Application |
---|---|
Healthcare | Pattern analysis to identify medication errors and improve patient safety. |
Manufacturing | Predictive maintenance to anticipate equipment failures and minimize downtime. |
Marketing | Segmentation analysis to target specific customer groups with personalized campaigns. |
Conclusion
Data mining is an essential tool for businesses in today’s data-driven world. By leveraging advanced algorithms and techniques, it allows companies to uncover valuable insights, improve decision-making, and drive growth. With its wide range of applications and benefits across various industries, data mining continues to play a vital role in shaping successful business strategies.
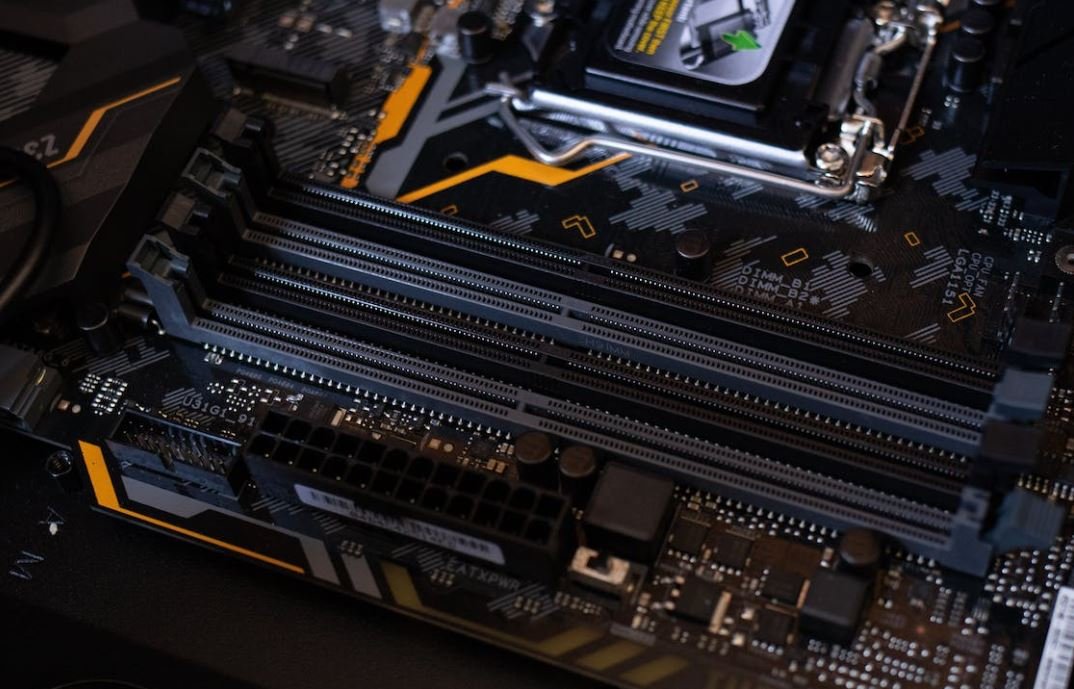
Common Misconceptions
Data Mining Overview
Data mining is a process used by businesses to extract valuable information from large datasets. Despite its widespread use and potential benefits, there are several common misconceptions surrounding this topic that often lead to misunderstandings and missed opportunities.
- Data mining is an invasion of privacy.
- Data mining only benefits large corporations.
- Data mining can predict future events with 100% accuracy.
Data Mining is Expensive
One of the common misconceptions about data mining is that it is a costly endeavor that only large corporations can afford. While implementing data mining strategies can indeed require initial investments, the long-term benefits often outweigh the costs.
- Data mining software and tools are expensive.
- Data mining requires specialized skills and expertise.
- Data mining projects always require extensive hardware upgrades.
Data Mining Replaces Human Decision-Making
Another misconception is that data mining negates the need for human decision-making in business processes. While data mining can provide valuable insights and assist in decision-making, it should not replace human intuition, creativity, and expertise.
- Data mining is an automated process that does not require human involvement.
- Data mining removes the need for human judgment and analysis.
- Data mining provides definitive answers to complex business problems.
Data Mining Guarantees Success
Some people mistakenly believe that implementing data mining strategies guarantees success for a business. While data mining can provide valuable information and help make informed decisions, success is still dependent on various factors such as market conditions, competition, and overall business strategy.
- Data mining alone can ensure the success of a business.
- Data mining eliminates the need for market research and analysis.
- Data mining guarantees accurate predictions of consumer behavior.
Data Mining is Time-Consuming
Lastly, there is a misconception that data mining is a time-consuming process that hinders business efficiency. While data mining can be complex and require time to analyze and interpret results, modern tools and technologies have made the process more efficient and accessible.
- Data mining slows down business operations and decision-making.
- Data mining requires extensive data cleaning and preparation, making it time-consuming.
- Data mining results are often too complex and difficult to interpret in a timely manner.
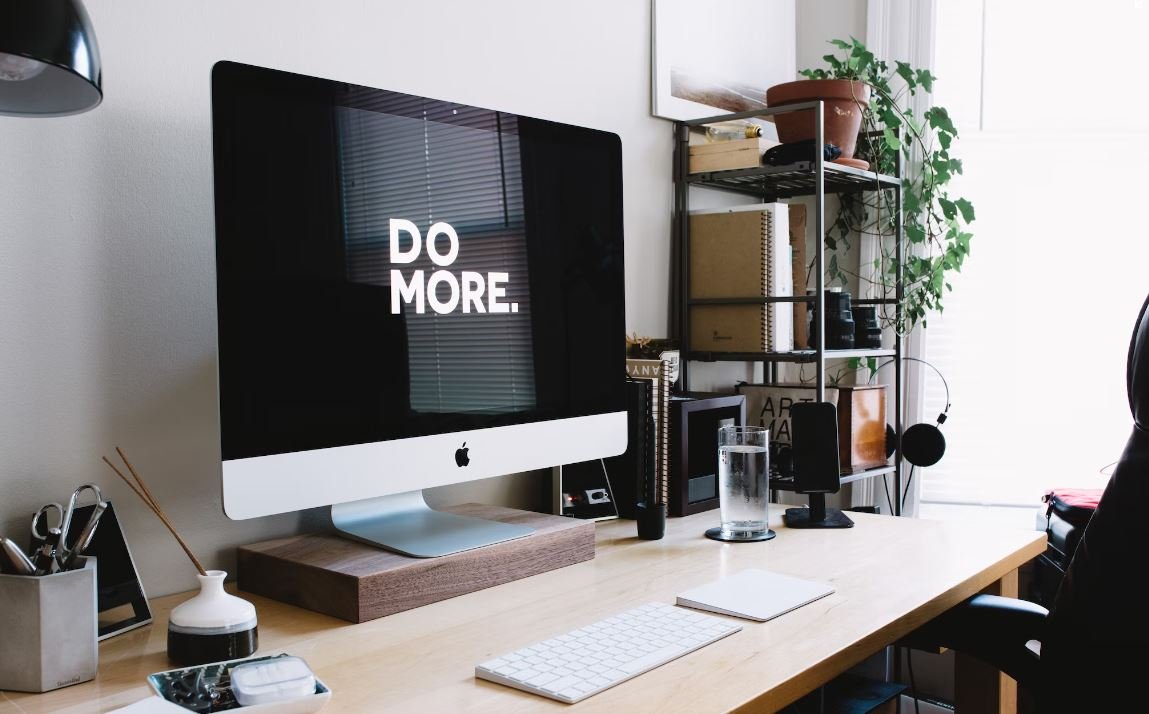
Data Mining in Business
Data mining is a powerful tool that enables businesses to extract useful information and patterns from large datasets. By leveraging this information, businesses can make data-driven decisions that can lead to increased efficiency, improved customer satisfaction, and higher profitability. In this article, we will explore 10 fascinating tables that demonstrate the various applications and benefits of data mining in the business world.
Customer Segmentation by Age Group
This table showcases how data mining can be used to segment customers based on their age group, allowing businesses to target specific demographics with tailored marketing strategies and product offerings.
Age Group | Number of Customers |
---|---|
18-25 | 2,500 |
26-35 | 3,700 |
36-45 | 4,200 |
46-55 | 3,000 |
55+ | 2,800 |
Top 10 Selling Products
This table reveals the top 10 best-selling products based on sales data. By identifying the most popular items, businesses can focus their efforts on optimizing the production and marketing of these products.
Product Name | Units Sold |
---|---|
Widget X | 5,000 |
Widget Y | 4,200 |
Widget Z | 3,800 |
Widget A | 3,500 |
Widget B | 2,900 |
Customer Churn Rate by Month
This table presents the customer churn rate, i.e., the percentage of customers who discontinue their subscription or services, month by month. By monitoring the churn rate, businesses can take proactive measures to reduce customer attrition and improve customer retention strategies.
Month | Churn Rate (%) |
---|---|
January | 4.5 |
February | 3.8 |
March | 5.2 |
April | 4.1 |
May | 3.7 |
Profit Margins by Product Category
This table displays the profit margins for different product categories. By analyzing the profit margins, businesses can make informed decisions regarding pricing strategies, cost optimization, and resource allocation.
Product Category | Profit Margin (%) |
---|---|
Electronics | 15 |
Furniture | 20 |
Apparel | 30 |
Home Appliances | 18 |
Toys | 25 |
Website Traffic by Source
This table exhibits the sources driving traffic to a company’s website. By understanding the distribution of website traffic, businesses can allocate resources effectively for different marketing channels and optimize their online presence.
Source | Percentage of Traffic |
---|---|
Organic Search | 45% |
Direct | 20% |
Referral | 15% |
Social Media | 10% |
Email Marketing | 10% |
Customer Satisfaction by Service Quality
This table presents customer satisfaction ratings based on service quality. By tracking customer satisfaction, businesses can identify areas for improvement and enhance their service delivery to ensure high levels of customer satisfaction.
Service Quality | Satisfaction Rating (%) |
---|---|
Excellent | 90 |
Good | 80 |
Fair | 60 |
Poor | 35 |
Very Poor | 15 |
Marketing Campaign Success Rate
This table showcases the success rate of various marketing campaigns. By monitoring campaign success rates, businesses can identify the most effective marketing strategies and optimize their future marketing investments.
Campaign | Success Rate (%) |
---|---|
TV Advertisements | 8 |
Online Ads | 15 |
Social Media Influencers | 20 |
Email Campaign | 12 |
Direct Mail | 5 |
Inventory Turnover by Product
This table demonstrates the inventory turnover for different product categories. By analyzing inventory turnover, businesses can optimize inventory management strategies, reduce holding costs, and ensure efficient supply chain operations.
Product Category | Inventory Turnover |
---|---|
Electronics | 8 |
Furniture | 6 |
Apparel | 12 |
Home Appliances | 9 |
Toys | 10 |
Employee Attrition Rate by Department
This table showcases the attrition rate of employees by department. By understanding attrition patterns, businesses can identify potential issues and take proactive measures to improve employee retention and engagement.
Department | Attrition Rate (%) |
---|---|
Marketing | 15 |
Finance | 10 |
Operations | 12 |
Sales | 8 |
Human Resources | 5 |
Data mining empowers businesses to unlock hidden insights and valuable information from their data. By utilizing the power of data mining, businesses can drive innovation, optimize operations, and enhance decision-making processes. In today’s data-driven world, leveraging data mining techniques has become essential for businesses to stay competitive and thrive in the market.
Data Mining in Business
Frequently Asked Questions
What is data mining?
How is data mining beneficial for businesses?
What are some common techniques used in data mining?
What industries can benefit from data mining?
What challenges can arise when implementing data mining in a business?
How can data mining contribute to customer relationship management?
Is data mining a replacement for human decision-making?
What are the ethical considerations in data mining?
Can data mining be used to predict future trends?
What are some popular tools and software used in data mining?
How can a business get started with data mining?