Data Mining is a Technique Where a Company
Data mining is a technique used by companies to extract valuable knowledge and insights from large datasets. By analyzing this data, businesses can make informed decisions, identify trends, and gain a competitive edge. In this article, we will explore the concept of data mining and how it benefits organizations.
Key Takeaways
- Data mining is a technique used by companies to extract knowledge from large datasets.
- It helps businesses make informed decisions and gain a competitive edge.
- Data mining identifies patterns, trends, and relationships within the data.
- Companies can use data mining to improve customer satisfaction and optimize operations.
- Data mining requires advanced algorithms and tools for efficient analysis.
**Data mining** involves the process of **extracting** and **analyzing** large datasets to uncover **hidden patterns** and **relationships**. *This technique allows companies to go beyond simple data retrieval, enabling them to gain valuable insights from complex data structures.* By utilizing advanced algorithms and tools, businesses can identify trends, predict future outcomes, and solve complex problems.
**Data mining** can benefit organizations in various ways. It enables businesses to analyze their __customer behavior__, identify purchasing patterns, and optimize marketing strategies accordingly. For example, a company may analyze its sales data to understand which products are frequently purchased together, allowing cross-selling opportunities to be exploited. *This technique also facilitates precise targeting of potential customers, resulting in increased conversion rates and customer satisfaction.* Additionally, data mining assists in identifying fraudulent activities, improving risk management, and optimizing operational processes.
The Data Mining Process
**Data mining** typically follows a structured process to ensure efficient and effective analysis. The key steps involved in the data mining process are:
- **Problem Definition**: Clearly define the goals and objectives of the data mining project.
- **Data Gathering**: Collect relevant data from various sources, ensuring its accuracy and completeness.
- **Data Preprocessing**: Cleanse and transform the data to remove noise, inconsistencies, or missing values.
- **Exploratory Data Analysis**: Understand and summarize the data through statistical techniques and visualization.
- **Modeling**: Develop a data mining model using appropriate algorithms, such as decision trees or clustering techniques.
- **Evaluation**: Assess the model’s performance and validity by testing it on independent datasets.
- **Deployment**: Implement the model to make predictions or apply the gained knowledge in practical scenarios.
Data Mining Techniques
Data mining encompasses a variety of techniques that can be employed based on the complexities and nature of the data. Some common data mining techniques include: **association rule mining**, **classification**, **clustering**, and **sequential pattern mining**. *These techniques allow organizations to derive valuable insights and patterns from their data, enabling better decision-making and problem-solving*.
Transaction ID | Items Purchased |
---|---|
1 | Bread, Milk, Eggs |
2 | Bread, Cheese |
3 | Milk, Eggs |
4 | Bread, Milk |
**Association rule mining** is a data mining technique that discovers relationships or associations among items in a dataset. It is commonly used in market basket analysis and has applications in recommendation systems. For example, analyzing purchasing patterns can help retailers identify products that are frequently bought together, leading to strategically planned product placements and targeted promotions.
Customer ID | Age | Income | Loan Approved |
---|---|---|---|
1 | 27 | 40,000 | Yes |
2 | 45 | 60,000 | Yes |
3 | 32 | 35,000 | No |
4 | 20 | 28,000 | No |
**Classification** predicts the class or category of a dataset based on its attributes or features. It is widely used in credit scoring, customer segmentation, and spam email filtering. For instance, financial institutions use classification to evaluate loan applications and predict customers’ creditworthiness based on factors such as age, income, and employment status.
Conclusion
Data mining is a powerful technique that helps companies gain valuable insights from their data, enabling them to make informed decisions and improve business performance. By analyzing large datasets, businesses can uncover hidden patterns, identify trends, and optimize various aspects of their operations. With the right tools and algorithms, data mining allows organizations to stay ahead in an increasingly competitive world.
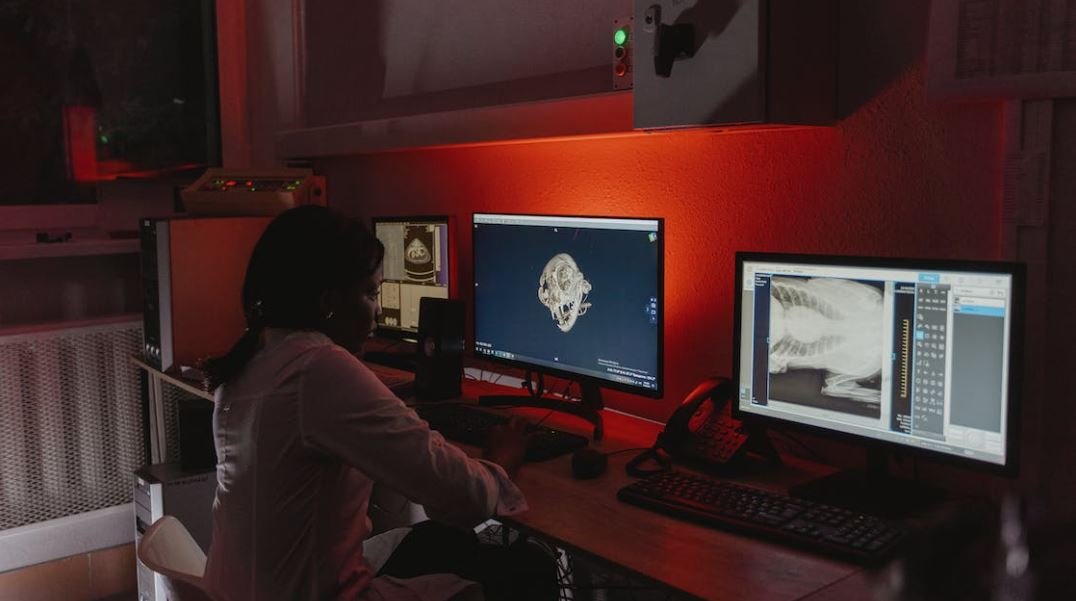
Common Misconceptions
Data Mining is a Technique Where a Company Will
Data mining is often misunderstood and leads to many misconceptions. One common misconception is that data mining is exclusively used by companies to gather and analyze customer data. However, data mining goes beyond just customer information and can be used for various purposes.
- Data mining can be applied in fields like healthcare to analyze patient data and identify patterns or trends in diseases.
- Data mining is used in finance to detect fraudulent activities or identify investment opportunities.
- Data mining can also be utilized in education to create personalized learning experiences for students based on their performance and behavior.
Another prevalent misconception is that data mining involves extracting information without consent or knowledge. Data mining is a legitimate practice that typically involves the use of anonymized data or data that has been collected with proper consent from individuals.
- Data mining often involves processing large datasets that have been properly curated and collected through legal means.
- Data mining adheres to ethical guidelines and privacy regulations to protect individuals’ information.
- Data mining can only access information to which it has been given authorized access.
Some people may also think that data mining is solely about finding correlations in data, without uncovering any deeper insights. However, data mining aims to discover meaningful patterns and relationships that can be used to make informed decisions and predictions.
- Data mining can identify hidden patterns that may not be apparent on the surface.
- Data mining can unveil trends and associations that help companies understand customer preferences and behavior.
- Data mining can provide valuable insights into market trends, allowing businesses to adjust strategies accordingly.
Another misconception surrounding data mining is the idea that it is a quick and effortless process that guarantees accurate results. In reality, data mining involves complex algorithms and meticulous analysis, requiring expertise and careful interpretation of the results.
- Data mining requires thorough data cleaning and preparation to ensure reliable results.
- Data mining models need to be adjusted and refined to improve accuracy and effectiveness.
- Data mining is an ongoing process that requires continuous monitoring and evaluation.
Lastly, some people may assume that data mining is primarily used for malicious purposes, such as invading privacy or manipulating data. However, data mining‘s primary purpose is to extract valuable insights and knowledge from vast amounts of data to benefit businesses, industries, and society as a whole.
- Data mining helps in improving product development and enhancing customer experience.
- Data mining can aid in detecting and preventing potential risks in various industries, such as cybersecurity.
- Data mining can contribute to scientific research and advancements in numerous fields.
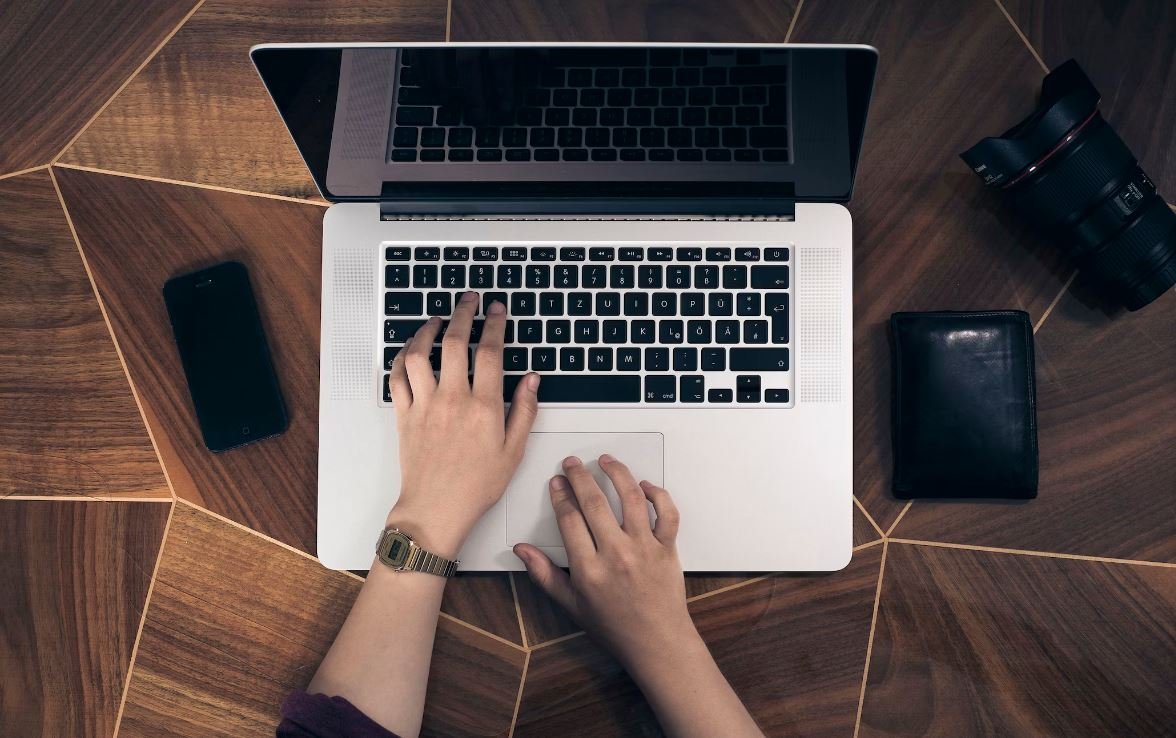
Data Mining is a Technique Where a Company Will Make the table VERY INTERESTING to read.
Data mining is a powerful technique used by companies to extract valuable insights and patterns from large sets of data. By analyzing these datasets, companies can uncover hidden trends, patterns, and relationships, allowing them to make informed decisions and improve their operations. In this article, we present ten interesting tables that showcase the various applications and benefits of data mining.
Table: Global Market Share of Leading Smartphone Brands
This table illustrates the global market share of the top five smartphone brands. It highlights the dominance of certain brands in the industry and the intense competition among manufacturers. Understanding the market share helps companies strategize their marketing efforts and stay ahead in this highly competitive market.
Table: Customer Satisfaction Scores for E-commerce Platforms
This table showcases the customer satisfaction scores for popular e-commerce platforms. It provides an overview of customer preferences, evaluating factors such as user experience, delivery speed, and product quality. Companies can use this data to identify areas for improvement and enhance customer satisfaction.
Table: Employee Performance Metrics by Department
By analyzing employee performance metrics, companies gain valuable insights into individual and departmental productivity. This table highlights key performance indicators such as average sales, customer satisfaction ratings, and task completion rates across different departments. This allows management to identify areas for employee development and enhance overall performance.
Table: Online Ad Click-Through Rates by Campaign Type
This table presents the click-through rates (CTR) for different types of online ad campaigns. It allows companies to compare the effectiveness of various campaign strategies and optimize their advertising efforts. Analyzing CTR helps businesses identify successful ad formats, target audiences more effectively, and increase overall ad engagement.
Table: Customer Churn Rate by Subscription Plan
Understanding customer churn rates is essential for businesses that offer subscription services. This table displays the churn rates for different subscription plans, providing insights into customer retention strategies. By identifying which plans have the highest churn rates, companies can tailor their offerings and implement targeted retention efforts.
Table: Product Sales by Geographic Region
Analyzing product sales by geographic region provides companies with a clear understanding of market demand across different areas. This table showcases the sales figures by region, highlighting potential growth opportunities and areas for market expansion. Utilizing this data, companies can allocate resources strategically and tailor marketing efforts to specific regions.
Table: Website Bounce Rates by Page Category
Website bounce rates refer to the percentage of visitors who leave a site after viewing only one page. This table breaks down the bounce rates by page category, such as blogs, product pages, and FAQs. By identifying high bounce rates, businesses can address website design and content issues to improve user engagement and increase conversion rates.
Table: Fraudulent Transactions Detected by Payment Method
For companies operating in the online marketplace, combating fraud is crucial. This table displays the number of fraudulent transactions detected for different payment methods, providing insights into potential vulnerabilities. Analyzing this data enables businesses to enhance security measures, protect customer data, and reduce financial losses.
Table: Social Media Engagement Metrics by Platform
Social media has become a vital tool for companies to engage with their target audience. This table presents key engagement metrics, including likes, shares, comments, and click-through rates, across various social media platforms. Analyzing this data helps businesses identify the most effective platforms and optimize their social media marketing strategies.
Table: Customer Lifetime Value by Acquisition Channel
Customer lifetime value (CLV) is a crucial metric for businesses aiming to maximize long-term customer loyalty. This table demonstrates the CLV based on the acquisition channel, such as organic search, social media, or email marketing. By understanding CLV per channel, companies can allocate resources effectively and focus on acquiring customers with the highest lifetime value.
Through data mining, companies can harness the power of data to make informed decisions, streamline operations, and improve overall performance. The tables presented in this article highlight the diverse applications of data mining across various industries. By leveraging these insights, businesses can fuel innovation, gain a competitive edge, and drive meaningful growth.
Data Mining FAQs
What is data mining?
Data mining is a technique used by companies to extract useful and meaningful patterns from large datasets. It involves various methods such as statistical analysis, machine learning, and database systems to identify trends, relationships, and anomalies. These insights can then be utilized for decision-making, predictive modeling, and enhancing business strategies.
How does data mining work?
Data mining works by applying algorithms and techniques to vast amounts of raw data collected from different sources. The process involves multiple steps, starting with data collection, preprocessing, and transformation. Then, various data mining techniques are applied to uncover patterns, relationships, and correlations within the data. Finally, the results are evaluated, interpreted, and used to make informed business decisions.
What are the benefits of data mining?
Some benefits of data mining include:
- Identifying customer buying patterns and preferences
- Improving targeted marketing and advertising campaigns
- Enhancing customer segmentation and personalization
- Detecting fraud and minimizing risks
- Optimizing business operations and resource allocation
- Improving product recommendations and cross-selling
- Forecasting future trends and demands
- Streamlining decision-making processes
- Identifying and mitigating potential bottlenecks or issues
- Gaining a competitive advantage in the market
What challenges can arise during data mining?
Some challenges that can arise during data mining include:
- Data quality issues, such as missing or inaccurate values
- Dealing with large and complex datasets
- Privacy concerns and ethical considerations
- Selection of appropriate data mining techniques
- Interpretation and validation of results
- Computational requirements and scalability
- Data integration and compatibility issues
- Maintaining data security and confidentiality
- Legal and regulatory compliance
- Effective communication of findings to stakeholders
What are some popular data mining techniques?
Some popular data mining techniques include:
- Decision Trees
- Association Rule Mining
- Clustering
- Regression Analysis
- Neural Networks
- Text Mining
- Time Series Analysis
- Classification Algorithms
- Sequential Pattern Mining
- Support Vector Machines
What industries commonly use data mining?
Data mining has applications across various industries, including:
- Retail and E-commerce
- Finance and Banking
- Healthcare and Pharmaceuticals
- Telecommunications
- Manufacturing and Supply Chain
- Insurance
- Transportation and Logistics
- Marketing and Advertising
- Government and Public Sector
- Social Media and Internet
How can data mining impact customer experience?
Data mining can impact customer experience by:
- Improving personalization and tailored recommendations
- Identifying and resolving customer pain points or issues
- Predicting customer needs and preferences
- Enhancing customer support and satisfaction
- Anticipating and addressing future customer demands
- Optimizing pricing strategies based on customer behavior
- Creating loyalty programs and targeted promotions
- Identifying and retaining high-value customers
What are the ethical considerations of data mining?
Some ethical considerations of data mining include:
- Respecting privacy rights and data protection regulations
- Ensuring data security and confidentiality
- Obtaining informed consent for data collection
- Using the data only for intended purposes
- Avoiding bias and discrimination in algorithmic decision-making
- Providing transparency and clear communication to individuals
- Minimizing the potential for unintended consequences or harm
What is the future of data mining?
The future of data mining is likely to witness advancements in areas such as:
- Integration of artificial intelligence and machine learning
- Handling larger volumes and varieties of data
- Real-time data mining and analysis
- Improved automation of data preprocessing
- Enhanced visualization tools for data exploration
- Advanced predictive modeling and anomaly detection
- Increased focus on interpretability and explainability of models
- Privacy-preserving techniques in data mining
- Utilizing data mining for social good and public interest
- Continuous evolution and adaptation to emerging challenges