Data Mining Is Best Described As
In today’s digital age, the world is generating an immense amount of data every second. This vast amount of data holds immense value, but it can also be overwhelming to make sense of it all. That’s where data mining comes in. Data mining is the process of extracting meaningful insights and patterns from large datasets, allowing businesses to make informed decisions and gain a competitive edge. Let’s delve deeper into what data mining is all about and how it can benefit various industries.
Key Takeaways
- Data mining is the process of extracting valuable information from large datasets.
- It helps businesses make informed decisions, improve efficiency, and gain a competitive edge.
- Data mining techniques include classification, clustering, and association rule mining.
- The benefits of data mining span across various industries, including finance, healthcare, and retail.
Data mining involves using advanced algorithms and statistical techniques to analyze large datasets. It helps businesses find hidden patterns, relationships, and insights that are often not apparent at first glance. By analyzing vast amounts of data, organizations can identify trends, make data-driven decisions, and uncover valuable information. *Data mining is like being a detective, searching for hidden gems of knowledge in a vast sea of information.*
There are several key techniques utilized in data mining to extract meaningful information from datasets:
- Classification: This technique involves categorizing data into predefined classes based on specified parameters. It helps predict unknown classes based on existing knowledge.
- Clustering: Clustering involves grouping similar data together based on similarities and differences. This technique helps identify relationships within datasets without predefined classes.
- Association rule mining: This technique identifies relationships and connections between variables in large datasets. It helps businesses understand the associations and dependencies between different data points.
*Data mining can reveal unexpected insights and correlations that may not be immediately apparent.* This knowledge can significantly impact decision-making processes and lead to more effective strategies, cost reductions, and improved efficiency. By harnessing the power of data mining, businesses can uncover hidden opportunities and gain a competitive advantage in their respective industries.
The Benefits of Data Mining
Data mining has widespread applications across various industries, bringing immense advantages to businesses. Let’s explore some key benefits:
1. Finance:
Data mining plays a crucial role in the finance industry. By analyzing historical financial data, businesses can identify patterns and predict market trends. This helps financial institutions manage risks, detect fraud, and make better investment decisions.
2. Healthcare:
Data mining has revolutionized the healthcare industry. It enables the analysis of vast amounts of patient data such as medical records, clinical trials, and genetic profiles. This helps healthcare providers improve patient outcomes, predict disease patterns, and develop personalized treatment plans.
3. Retail:
In the retail sector, data mining is used to analyze customer purchasing patterns, preferences, and behavior. This information helps businesses create targeted marketing campaigns, optimize pricing strategies, and improve overall customer satisfaction.
Data Mining in Action: Interesting Data Points
To showcase the power of data mining, let’s take a look at some interesting data points:
Industry | Data Point |
---|---|
Online Retail | Customers who bought item A also bought item B, resulting in a 30% increase in sales of both items. |
Telecommunications | Analyzing customer complaints led to identifying a common issue, allowing the company to address it and significantly reduce customer churn. |
Data Mining Techniques: Classification Model Evaluation
To illustrate the evaluation of a classification model in data mining, consider the following table:
Actual Class | Predicted Class |
---|---|
Positive | Positive |
Negative | Positive |
Negative | Negative |
Positive | Negative |
In this evaluation, we can calculate metrics such as accuracy, precision, recall, and F1-score to assess the performance of the classification model.
Data Mining: Empowering Businesses with Insights
Data mining has revolutionized the way businesses extract value from their data. By utilizing sophisticated techniques and algorithms, organizations can uncover hidden patterns, relationships, and insights that can drive strategic decision-making. *With data mining, businesses gain a competitive edge by transforming data into actionable knowledge.* So, whether you’re in finance, healthcare, retail, or any other industry, harnessing the power of data mining can lead to improved efficiency, increased profits, and enhanced customer satisfaction.
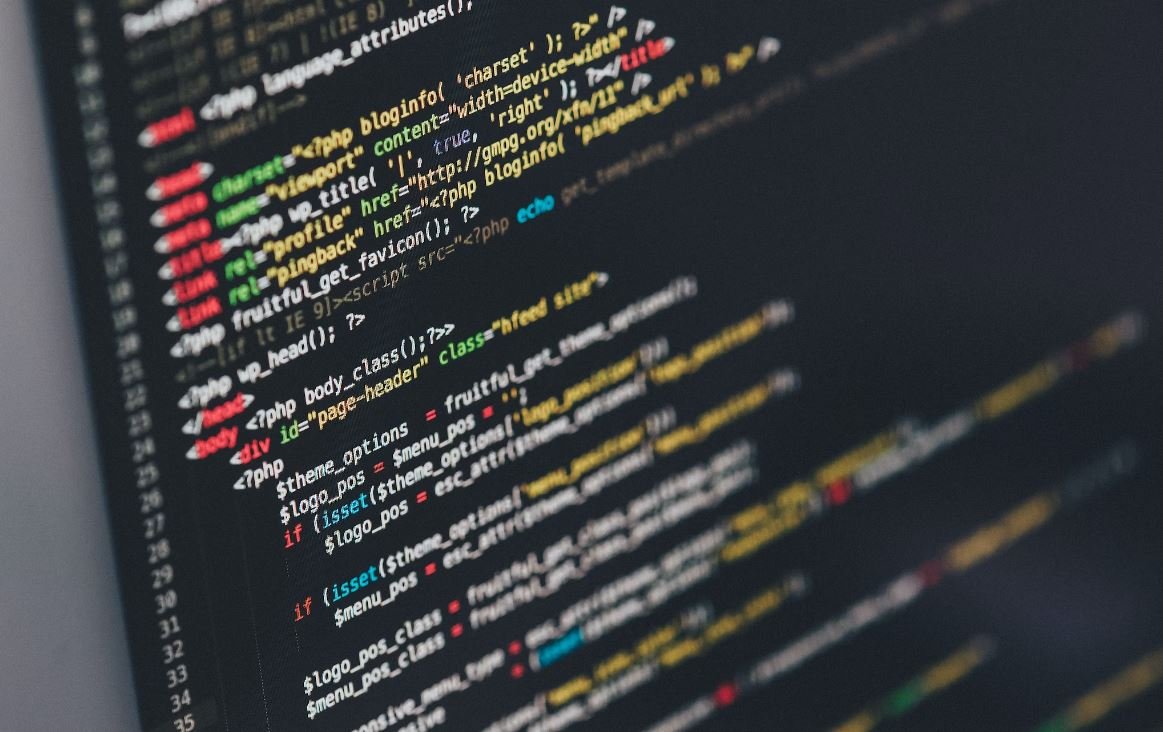
Common Misconceptions
1. Data Mining Requires Access to Large Datasets
One common misconception about data mining is that it requires access to vast amounts of data in order to be effective. However, this is not entirely true. While having access to large datasets can certainly be advantageous, data mining techniques can still be applied to smaller datasets with success.
- Data mining techniques can uncover valuable insights even from relatively small datasets.
- Data quality is often more important than data quantity when it comes to the effectiveness of data mining.
- Data mining can provide meaningful results even with limited data availability.
2. Data Mining Always Provides Definitive Answers
Another common misconception is that data mining always provides definite and concrete answers. In reality, data mining is an exploratory process that involves analyzing data to uncover patterns and relationships, rather than providing absolute truths.
- Data mining results are often probabilistic in nature and should be interpreted accordingly.
- Data mining assists in generating insights and hypotheses, which may require further analysis and validation.
- Data mining results should be used as inputs for decision-making rather than being considered as final and conclusive answers in themselves.
3. Data Mining is Only Used for Predictive Analytics
Data mining is often mistakenly thought of as solely being used for predictive analytics. While predictive modeling is indeed a significant application of data mining, it is not the only one. Data mining techniques can also be employed for descriptive analytics, where the focus is on summarizing and understanding data.
- Data mining facilitates the discovery of patterns and relationships within data, regardless of the predictive or descriptive nature of the analysis.
- Data mining can be used for various purposes such as anomaly detection, clustering, and pattern recognition.
- Data mining techniques can provide valuable insights into historical data, aiding in understanding past trends and patterns.
4. Data Mining is All About Technology
Many people mistakenly believe that data mining is solely a technological process that requires advanced tools and software. While technology is undoubtedly an essential component of data mining, it is not the sole factor in achieving successful outcomes.
- Data mining also involves expertise in statistics, mathematics, and domain knowledge to extract meaningful insights from data.
- Data mining requires proper planning, data preparation, and interpretation of results to derive value from the process.
- Data miners need to understand the context and goals of the analysis to apply appropriate techniques and interpret the findings accurately.
5. Data Mining is Intrusive and Violates Privacy
A misconception about data mining is that it is invasive and infringes on individuals’ privacy. However, data mining can be performed in a way that respects privacy regulations and protects sensitive information.
- Data mining can be conducted using anonymized or aggregate data, ensuring individual identities and personal information remain protected.
- Data mining can be guided by ethical principles and legal frameworks to safeguard privacy rights.
- Data mining can offer benefits to individuals and society while still maintaining privacy and data security.
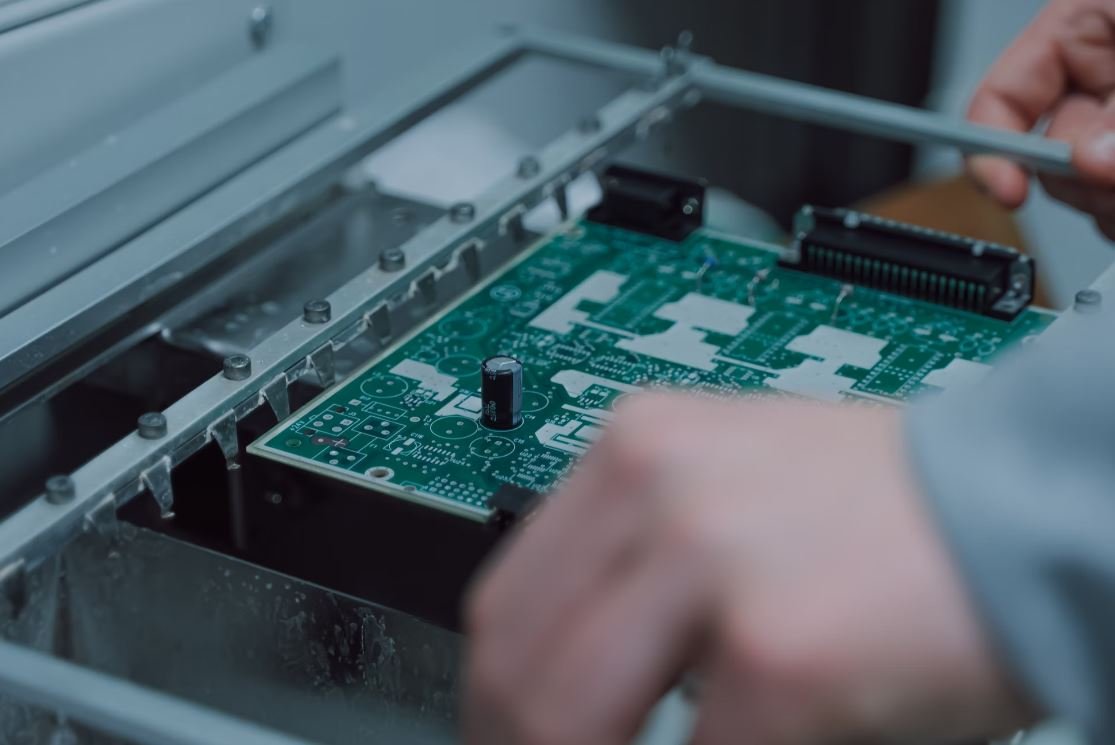
Data Mining in the Healthcare Industry
Data mining has been widely used in various industries, including healthcare. By analyzing large datasets and extracting valuable knowledge, data mining enables healthcare professionals to make more informed decisions and improve patient outcomes. The following table presents an overview of different applications of data mining in the healthcare industry and their corresponding benefits.
Data Mining Techniques
Data mining techniques are employed to discover patterns and relationships within datasets. This table highlights some commonly used data mining techniques and provides a brief description of each technique’s purpose.
Data Mining vs. Machine Learning
Data mining and machine learning are often used interchangeably, but they have distinct differences. This table illustrates the contrasting characteristics of data mining and machine learning, shedding light on their respective methodologies and applications.
Data Mining Algorithms
Data mining algorithms play a crucial role in uncovering hidden patterns and trends within datasets. The following table provides an overview of several popular data mining algorithms and showcases their specific functionalities.
Data Mining in Marketing
Data mining has revolutionized the field of marketing by allowing organizations to gain valuable insights into consumer behavior. This table highlights different marketing activities where data mining can be applied, along with the benefits that can be achieved through its utilization.
Data Mining Applications in Finance
Data mining has proven to be instrumental in the financial industry, enabling organizations to model trends, predict market fluctuations, detect fraud, and much more. The table below outlines various applications of data mining in finance and the positive impact it can have on the industry.
Data Mining in E-commerce
E-commerce platforms generate vast amounts of data daily, making data mining essential for extracting meaningful information. This table showcases how data mining can enhance various aspects of e-commerce, such as personalized recommendations, targeted advertising, and fraud detection.
Data Mining in Social Media
Data mining techniques can be used to analyze social media data, providing valuable insights on trends, sentiment analysis, and user preferences. The table below presents different applications of data mining in social media and their corresponding benefits for marketers and researchers.
Data Mining in Education
Data mining has revolutionized education by offering insights into student performance, identifying at-risk individuals, and enhancing learning outcomes. The table provides examples of how data mining can be applied in education, leading to more personalized and effective teaching approaches.
Data Mining in Customer Relationship Management
Capturing and analyzing customer data has become essential for businesses aiming to enhance customer relationships. The table below demonstrates various customer relationship management aspects where data mining can be deployed, resulting in improved customer satisfaction and loyalty.
In conclusion, data mining has emerged as a powerful tool in various industries, facilitating decision-making processes and providing valuable insights. By employing data mining techniques and algorithms, organizations can unlock hidden patterns, predict future trends, and make data-driven decisions. As technology and data continue to evolve, data mining will undoubtedly play an increasingly significant role in shaping the future of industries and improving overall performance.
Frequently Asked Questions
1. What is data mining?
Data mining is the process of extracting valuable insights and patterns from large datasets. It involves analyzing and discovering patterns, relationships, and trends within the data to facilitate decision-making or gain deeper understanding.
2. How is data mining different from data analysis?
Data mining and data analysis are closely related but have distinct differences. While data analysis focuses on exploring, cleaning, and transforming data, data mining goes beyond that by utilizing advanced algorithms to uncover hidden patterns and relationships in the data.
3. What are the key steps involved in data mining?
Data mining typically involves the following steps: data collection, data preprocessing, feature selection, data transformation, choosing appropriate data mining algorithms, applying the algorithms to the dataset, evaluating the results, and interpreting the findings.
4. In what industries is data mining commonly used?
Data mining finds applications in various industries such as finance, healthcare, retail, marketing, telecommunications, and manufacturing. It can be used for fraud detection, customer segmentation, market analysis, demand forecasting, and many other purposes.
5. What are the benefits of data mining?
Data mining offers several benefits including improved decision-making, enhanced marketing strategies, better customer segmentation, increased operational efficiency, reduced costs, and identification of new business opportunities.
6. What are some common data mining techniques?
Common data mining techniques include classification, regression, clustering, association, and anomaly detection. Classification involves assigning data instances into predefined categories, regression predicts a numerical value based on input variables, clustering groups similar data points together, association discovers relationships among variables, and anomaly detection identifies unusual patterns in the data.
7. What challenges are faced in data mining?
Some challenges in data mining include dealing with large and complex datasets, handling missing or noisy data, selecting appropriate variables or features, ensuring data privacy and security, dealing with ethical considerations, and interpreting and validating the results obtained from the data mining process.
8. What tools and technologies are commonly used in data mining?
There are several popular tools and technologies used in data mining, such as Python, R, MATLAB, SQL, Apache Hadoop, Apache Spark, and various machine learning libraries like Scikit-learn and TensorFlow. These tools provide a wide range of functionalities and algorithms to support data mining tasks.
9. How does data mining relate to machine learning?
Data mining and machine learning are interconnected fields. Data mining can be seen as an initial step in the knowledge discovery process, where patterns are extracted from the data. Machine learning algorithms are then used to build models and make predictions based on these patterns. In other words, data mining provides valuable insights for machine learning models.
10. What are some ethical considerations in data mining?
Some ethical considerations in data mining include ensuring data privacy and security, obtaining informed consent from individuals whose data is being analyzed, avoiding discrimination or bias, maintaining transparency in data usage, and adhering to legal and regulatory requirements associated with data handling.