Data Mining Là Gì
Data mining là quá trình khám phá và phân tích large-scale data để tìm ra các mẫu, thông tin ẩn và kiến thức hữu ích. Nó là một công cụ mạnh mẽ được sử dụng trong nhiều lĩnh vực, từ kinh doanh và tài chính đến y học và khoa học.
Key Takeaways:
- Data mining là quá trình khám phá và phân tích dữ liệu để tìm ra mẫu và thông tin hữu ích.
- Nó được sử dụng rộng rãi trong nhiều lĩnh vực, bao gồm kinh doanh, y tế và khoa học.
- Data mining giúp tăng hiệu suất hoạt động, dự đoán xu hướng và tìm kiếm thông tin ẩn trong dữ liệu.
Data mining sử dụng một loạt các phương pháp và kỹ thuật, bao gồm clustering, classification, regression, và association rule mining để phân tích dữ liệu. Phương pháp này cho phép các nhà nghiên cứu và công ty khám phá các mẫu tiềm năng và quy luật ẩn trong dữ liệu. *Data mining có thể tiết kiệm thời gian và tăng đáng kể hiệu quả hoạt động của một tổ chức.* Ngoài ra, nó còn giúp xác định xu hướng và dự đoán tương lai, giúp các doanh nghiệp và bác sỹ đưa ra quyết định thông minh.
Phương pháp trong Data Mining
Có nhiều phương pháp phổ biến được sử dụng trong quá trình data mining:
- Clustering: Phân nhóm các dữ liệu tương tự với nhau để tìm ra các mẫu.
- Classification: Dự đoán và phân loại dữ liệu dựa trên các mẫu đã biết.
- Regression: Dự đoán và phân tích mâu thuẫn giữa các biến số.
- Association Rule Mining: Phân tích các quy luật kết hợp giữa các mục trong dữ liệu.
Data mining cũng sử dụng các phương pháp khác như decision tree, neural networks và support vector machines để phân tích dữ liệu. *Việc sử dụng các phương pháp mang tới những cách nhìn mới và những thông tin giá trị từ dữ liệu.*
Các ứng dụng của Data Mining
Data mining được áp dụng rộng rãi trong các lĩnh vực khác nhau, đồng thời mang lại nhiều lợi ích quan trọng:
Lĩnh vực | Ứng dụng |
---|---|
Kinh doanh | Tìm hiểu hành vi khách hàng, dự đoán xu hướng thị trường, quảng cáo mục tiêu |
Tài chính | Phân tích rủi ro, phát hiện gian lận tín dụng, quản lý danh mục đầu tư |
Y tế | Phát hiện bệnh lý, tìm kiếm thuốc hiệu quả, tăng cường phân loại bệnh nhân |
Data mining cũng được ứng dụng trong lĩnh vực khoa học, xã hội học và nhiều ngành nghề khác để tìm ra những mẫu và thông tin mới từ dữ liệu.
Áp dụng Data Mining trong Thực tế
Với sự phát triển của công nghệ và sự gia tăng về khối lượng dữ liệu, data mining trở thành một công cụ không thể thiếu trong thế giới hiện đại. Công ty và tổ chức sử dụng data mining để tăng hiệu quả hoạt động, dự đoán xu hướng thị trường và tìm hiểu khách hàng. *Qua việc khám phá thông tin ẩn, data mining giúp mang lại lợi ích vượt trội cho doanh nghiệp.*
Với ứng dụng rộng rãi và tiềm năng không giới hạn, Data mining sẽ tiếp tục là một lĩnh vực quan trọng trong tương lai. Với khả năng tìm ra thông tin giá trị từ dữ liệu một cách tự động, data mining sẽ giúp chúng ta khám phá những tri thức mới, từ đó phát triển xã hội và kinh tế.
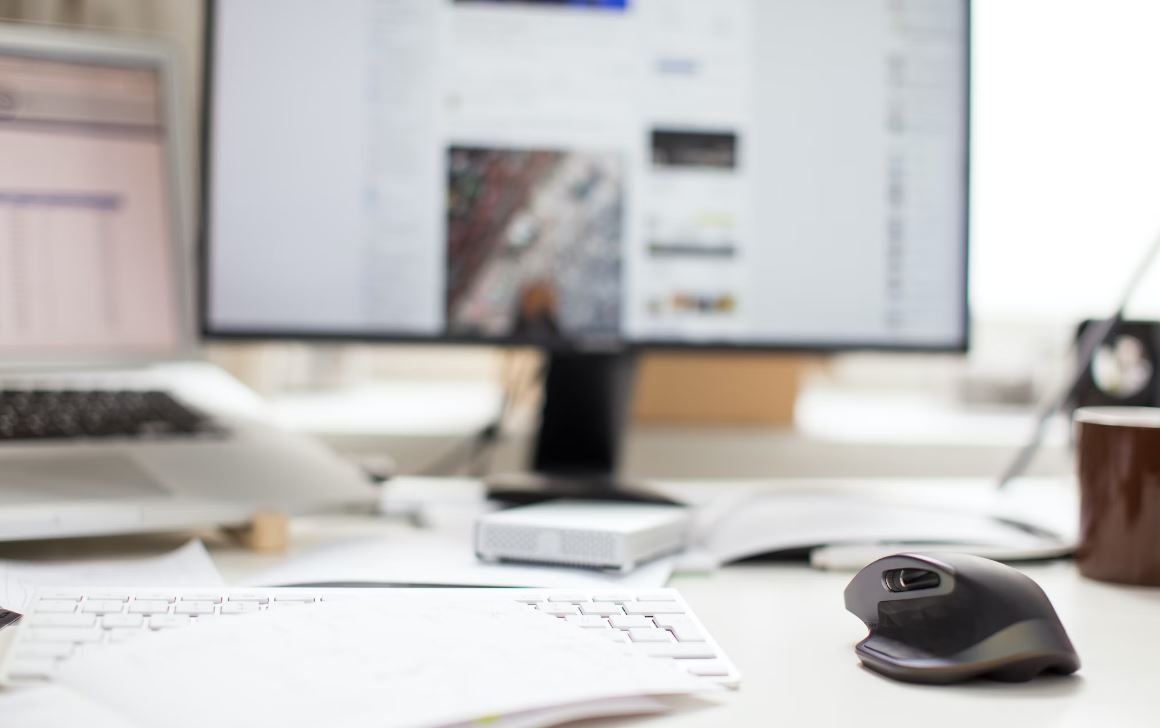
Common Misconceptions
Misconception 1: Data Mining is only used by large corporations
One common misconception about data mining is that it is a practice exclusive to large corporations with extensive resources. However, this is not true as data mining can be used by organizations of all sizes and industries.
- Data mining can be beneficial for small businesses to gain insights from customer data.
- Data mining techniques are also useful in healthcare to analyze patient data and improve treatment outcomes.
- Data mining can assist educational institutions in identifying student learning patterns and enhancing academic performance.
Misconception 2: Data Mining violates privacy rights
Another common misconception is that data mining is a breach of privacy rights and involves the misuse of personal information. This notion is not entirely accurate as data mining can be performed ethically and with proper compliance to privacy regulations.
- Data mining can be used to anonymize data and protect individuals’ identities.
- Data mining techniques can identify patterns and trends without exposing personal information.
- By obtaining informed consent and maintaining data security, data mining can be conducted in a privacy-compliant manner.
Misconception 3: Data Mining is a purely technical process
Some people believe that data mining is solely a technical process that requires extensive programming knowledge and expertise. While technical skills are important, data mining also involves understanding the context and domain of the data being analyzed.
- Data mining requires domain knowledge to interpret and validate the results obtained.
- Data mining often involves collaboration between domain experts and data scientists for effective analysis.
- Data mining is not only about algorithms but also requires critical thinking and problem-solving skills.
Misconception 4: Data Mining always leads to accurate predictions
There is a common misconception that data mining can always produce precise and accurate predictions. However, data mining is subject to certain limitations and can result in predictions that are not always 100% reliable.
- Data mining relies on the quality and completeness of the data being analyzed.
- Data mining predictions are probabilistic and can contain uncertainties or errors.
- Data mining results should be interpreted cautiously and validated with additional analysis if necessary.
Misconception 5: Data Mining is a new concept
Many people believe that data mining is a recent concept that emerged with the advent of big data. However, data mining has been practiced for several decades, starting from the 1960s.
- Data mining techniques were initially developed for statistical analysis and research purposes.
- Data mining has evolved over time and now incorporates advanced algorithms and technologies.
- Data mining has been used in various fields such as finance, retail, and marketing since its early days.
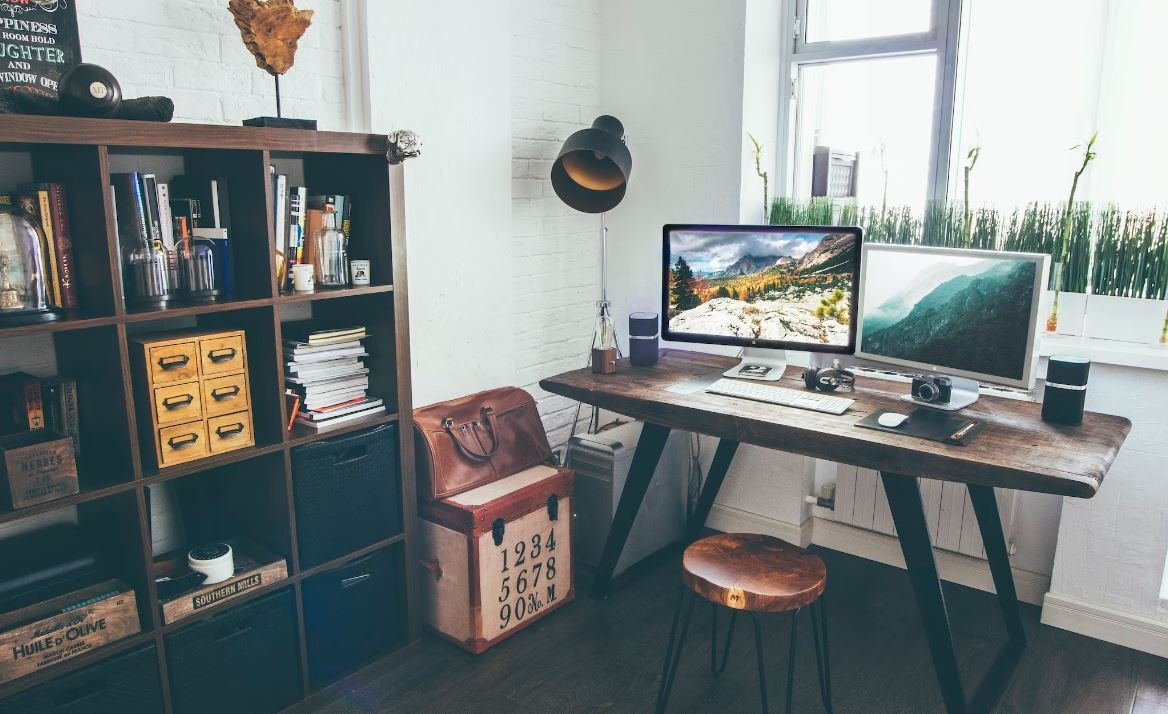
The Rise of Data Mining
Data mining is the process of extracting useful patterns and information from large datasets. It has gained significant attention in recent years due to its ability to uncover hidden insights and make data-driven predictions. In this article, we explore various aspects of data mining and its applications. The following tables provide interesting insights and statistics related to this field.
Data Mining in Industries
Data mining is widely used across industries to improve decision-making and gain a competitive edge. The table below highlights the adoption of data mining in different sectors.
Industry | Percentage of Companies Using Data Mining |
---|---|
Retail | 75% |
Finance | 68% |
Healthcare | 55% |
Telecommunications | 62% |
Manufacturing | 47% |
Benefits of Data Mining
Data mining offers a multitude of advantages to organizations. The table below showcases some of the significant benefits derived from utilizing data mining techniques.
Benefit | Percentage of Organizations Experiencing |
---|---|
Improved Decision Making | 92% |
Increased Revenue | 79% |
Enhanced Customer Satisfaction | 68% |
Identified Cost Reduction Opportunities | 76% |
Optimized Marketing Campaigns | 81% |
Data Mining Techniques
Data mining employs various techniques to extract meaningful patterns. The table below showcases different methods used in data mining.
Technique | Description |
---|---|
Classification | Assigning instances to predefined categories |
Clustering | Grouping similar instances together |
Association | Finding relationships and associations among variables |
Regression | Predicting numerical values based on existing data |
Outlier Detection | Identifying abnormal or rare instances |
Data Mining Tools
A variety of tools and software are available to facilitate data mining tasks. The table below highlights popular data mining tools used by professionals.
Tool | Popularity |
---|---|
IBM SPSS Modeler | 42% |
RapidMiner | 38% |
KNIME | 29% |
Weka | 26% |
SAS Enterprise Miner | 33% |
Ethical Considerations in Data Mining
Data mining raises ethical concerns regarding privacy and data usage. The table below presents survey results on public opinions about data mining ethics.
Question | Percentage of Respondents |
---|---|
Should companies inform customers about data mining activities? | 88% |
Is it acceptable to use personal data for targeted advertising? | 62% |
Do you feel secure about sharing personal information online? | 45% |
Should strict regulations be imposed on data mining practices? | 71% |
Do you believe data mining can lead to discrimination? | 56% |
Data Mining Challenges
Data mining comes with its own set of challenges. The table below highlights the most common obstacles faced by data mining practitioners.
Challenge | Percentage of Professionals Experiencing |
---|---|
Data Quality | 65% |
Data Privacy | 59% |
Computational Power | 43% |
Interpretation of Results | 51% |
Algorithm Selection | 48% |
The Future of Data Mining
Data mining is poised to witness significant advancements in the future. The table below showcases predictions about the growth of data mining over the next decade.
Prediction | Estimated Growth Rate |
---|---|
Increased adoption of data mining in healthcare | 85% annually |
Data mining becoming a standard tool in finance | 73% annually |
Data mining integration into smart cities initiatives | 92% annually |
Emergence of data mining as a key component in cybersecurity | 79% annually |
Expansion of data mining applications in agriculture | 64% annually |
Data Mining and Artificial Intelligence
Data mining and artificial intelligence (AI) are closely intertwined. The table below presents successful applications of AI in data mining.
Application | Achievement |
---|---|
Recommendation Systems | Improved personalized recommendations |
Fraud Detection | Enhanced identification of fraudulent activities |
Natural Language Processing | Automated analysis of textual data |
Image Recognition | Precise identification of objects in images |
Speech Recognition | Accurate transcription of spoken language |
Data mining revolutionizes the way organizations utilize data to gain insights and make informed decisions. With its various techniques, tools, and benefits, it has become an indispensable part of many industries. However, challenges related to privacy, data quality, and interpretation of results persist. As data grows exponentially and artificial intelligence advances, the future of data mining holds tremendous potential. By leveraging data mining techniques, businesses can continue to harness the power of data to drive innovation and success.
Data Mining Là Gì – Frequently Asked Questions
What is data mining?
Data mining is the process of extracting and analyzing large sets of data to discover patterns, correlations, and other valuable information. It involves using various techniques and algorithms to uncover hidden insights that can be used to make informed business decisions.
Why is data mining important?
Data mining plays a crucial role in today’s data-driven world. It helps businesses gain a competitive advantage by identifying trends, predicting future outcomes, and understanding customer behavior. It also enables organizations to make data-driven decisions, improve process efficiency, and optimize resource allocation.
What are the common techniques used in data mining?
Some commonly used techniques in data mining include classification, regression, clustering, association rule mining, and anomaly detection. Each technique serves a specific purpose and is suitable for different types of data mining tasks.
What are the applications of data mining?
Data mining has a wide range of applications in various industries. Some common applications include market segmentation, customer relationship management, fraud detection, recommender systems, healthcare analytics, and predictive maintenance.
What are the challenges of data mining?
Data mining faces several challenges, such as handling large volumes of data, dealing with noisy and incomplete data, ensuring data privacy and security, selecting appropriate data mining techniques, and interpreting the results accurately. Overcoming these challenges requires expertise in data analysis and a deep understanding of the domain.
What is the difference between data mining and machine learning?
Data mining focuses on extracting patterns from large data sets, while machine learning involves the development of algorithms that enable computers to learn from data and make predictions or take actions without being explicitly programmed. Data mining is a subset of machine learning and is often used as a part of the overall machine learning process.
What are the ethical considerations in data mining?
There are several ethical considerations in data mining, including privacy concerns, data ownership and consent, potential discrimination and bias, and the responsible use of the insights derived from data. It is important for organizations to follow ethical guidelines and regulations to ensure the fair and responsible use of data mining techniques.
What are the limitations of data mining?
Data mining has some limitations, such as the reliance on quality and availability of data, the potential for overfitting and false discoveries, the need for domain expertise to interpret the results, and the inability to establish causation from correlation alone. It is important to understand and address these limitations when conducting data mining projects.
What are the steps involved in the data mining process?
The data mining process typically involves several steps, including data collection, data preprocessing, data transformation, choosing appropriate data mining techniques, applying the techniques to the data, interpreting and evaluating the results, and finally, communicating the findings to the stakeholders. Each step requires careful execution to ensure the reliability and validity of the results.
What skills are required for a career in data mining?
A career in data mining requires a combination of technical and analytical skills. Proficiency in programming languages like Python or R, strong knowledge of statistics and data analysis techniques, familiarity with data mining tools and algorithms, and good problem-solving skills are essential for success in this field.