Data Mining Lift
Data mining lift is a key performance metric used in data analysis projects to evaluate the effectiveness of a predictive model or the impact of a certain variable on outcomes. It measures the ratio of predicted positive results to the expected positive results.
Key Takeaways
- Data mining lift is a performance metric in data analysis.
- It quantifies the effectiveness of a predictive model.
- Lift measures the ratio of predicted positive results to expected positive results.
In data mining, models are developed to predict an outcome or identify patterns in vast datasets. These models could be used in various domains, including marketing, finance, and healthcare. **The lift metric** helps in assessing the model’s accuracy and determining if it provides any significant improvement compared to a random selection or a baseline model.
**One interesting aspect** of data mining lift is that it allows businesses to identify target segments for marketing campaigns, improving their accuracy and efficiency. By understanding the lift associated with certain customer attributes or behaviors, marketers can tailor their offers to highly receptive audiences, thus increasing conversion rates and maximizing the return on investment.
Calculating Data Mining Lift
The lift is calculated by dividing the response rate of a targeted model by the response rate of a baseline model. It is often represented as a multiple of the baseline response rate. The formula for calculating the lift is as follows:
Response Rate | Lift Calculation |
---|---|
20% | 1.5x |
40% | 3x |
60% | 4.5x |
80% | 6x |
100% | 7.5x |
*An interesting insight* from the calculations is that the higher the response rate, the greater the lift value. This means that targeting specific groups with a higher likelihood of positive outcomes can significantly improve the overall performance of a model or campaign.
Interpreting Data Mining Lift
A lift value less than 1 suggests that the model’s predictions are worse than random chance, while a lift value greater than 1 indicates improvement compared to the baseline model. **It’s important to note** that lift values significantly greater than 1 imply strong predictive power.
Lift Range | Interpretation |
---|---|
0 – 1 | Weak predictive power |
1 – 3 | Moderate predictive power |
3 – 5 | Strong predictive power |
5+ | Very strong predictive power |
**Another interesting finding** is that lift values above 5 are considered to have very strong predictive power, indicating a substantial impact of the variables on the outcome. Organizations can leverage this knowledge to focus on influential factors and make data-driven decisions.
Applying Data Mining Lift
Data mining lift can guide decision-making in various business scenarios, including:
- Marketing campaigns: Identifying target segments with higher lift values to enhance campaign effectiveness.
- Risk assessment: Evaluating the impact of certain variables on the likelihood of specific risks occurring.
- Customer retention: Understanding the lift of churn prediction models to prioritize retention efforts.
By leveraging the power of data mining lift, organizations can optimize their strategies and allocate resources more efficiently.
As data mining techniques continue to evolve, understanding lift and its applications becomes increasingly crucial for effective decision-making and maximizing business outcomes. So, next time you delve into data analysis, keep data mining lift in mind as a powerful metric to evaluate the predictive strength of your models and make informed decisions.
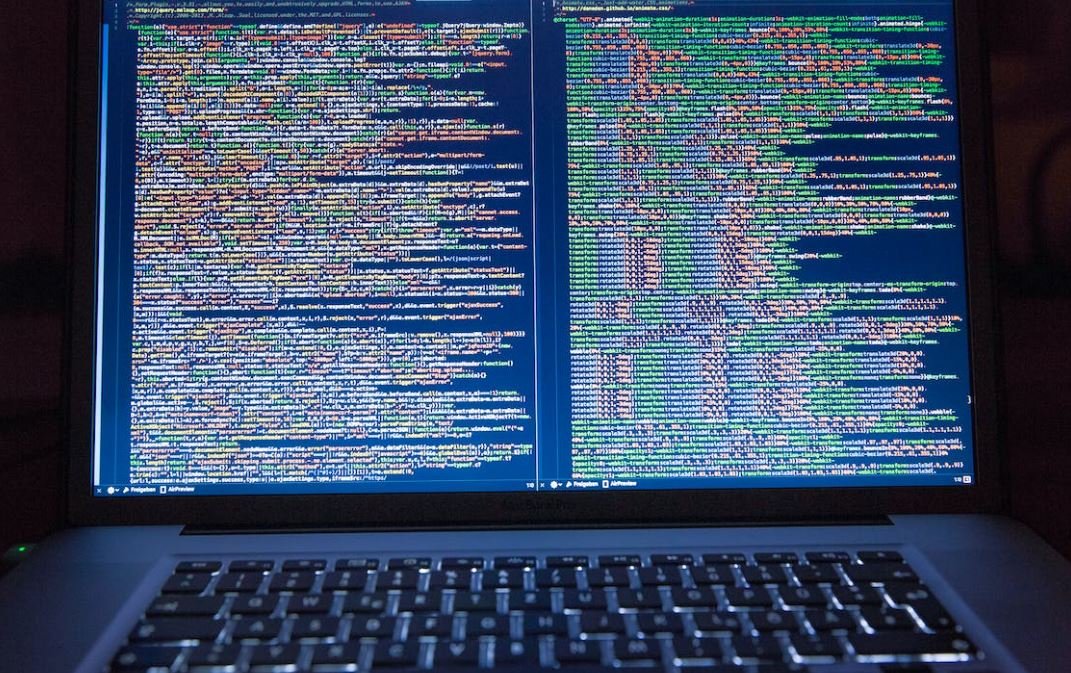
Common Misconceptions
Misconception: Data mining is only about gathering information
One common misconception about data mining is that it is solely concerned with gathering information. However, data mining goes beyond just collecting data. It involves analyzing and interpreting the collected data to find valuable patterns, trends, and insights.
- Data mining involves extracting meaningful insights from data
- Data mining requires advanced analytics techniques
- Effective data mining helps in making data-driven decisions
Misconception: Data mining is all about finding the “holy grail”
Another misconception is that data mining always leads to finding a groundbreaking discovery or a “holy grail” insight. While data mining can certainly uncover valuable and actionable insights, it does not guarantee a revolutionary finding in every instance.
- Data mining identifies patterns that may lead to insights
- Data mining helps uncover hidden relationships in data
- Not all data mining efforts lead to extraordinary discoveries
Misconception: Data mining is a single-step process
Some people mistakenly believe that data mining is a single-step process. However, data mining involves several steps and requires careful planning, data preparation, model building, and evaluation to yield accurate and reliable results.
- Data mining entails preprocessing and cleaning the data
- Data mining involves selecting appropriate modeling techniques
- Data mining requires proper evaluation and validation of results
Misconception: Data mining is only applicable to large datasets
An incorrect assumption is that data mining is only applicable to large datasets. In reality, data mining techniques can be applied to datasets of various sizes, including small and medium-sized datasets, to uncover meaningful insights.
- Data mining can be employed on datasets of any size
- Data mining can extract valuable insights from smaller datasets too
- Data mining offers benefits to organizations with diverse data sizes
Misconception: Data mining is synonymous with invasion of privacy
One major misconception surrounding data mining is that it is synonymous with invasion of privacy. While it is true that data mining can involve analyzing large amounts of personal data, responsible data mining practices prioritize privacy and adhere to ethical guidelines.
- Data mining can be performed while respecting privacy laws
- Data mining should be conducted with anonymized or aggregated data
- Data mining respects the privacy and rights of data subjects
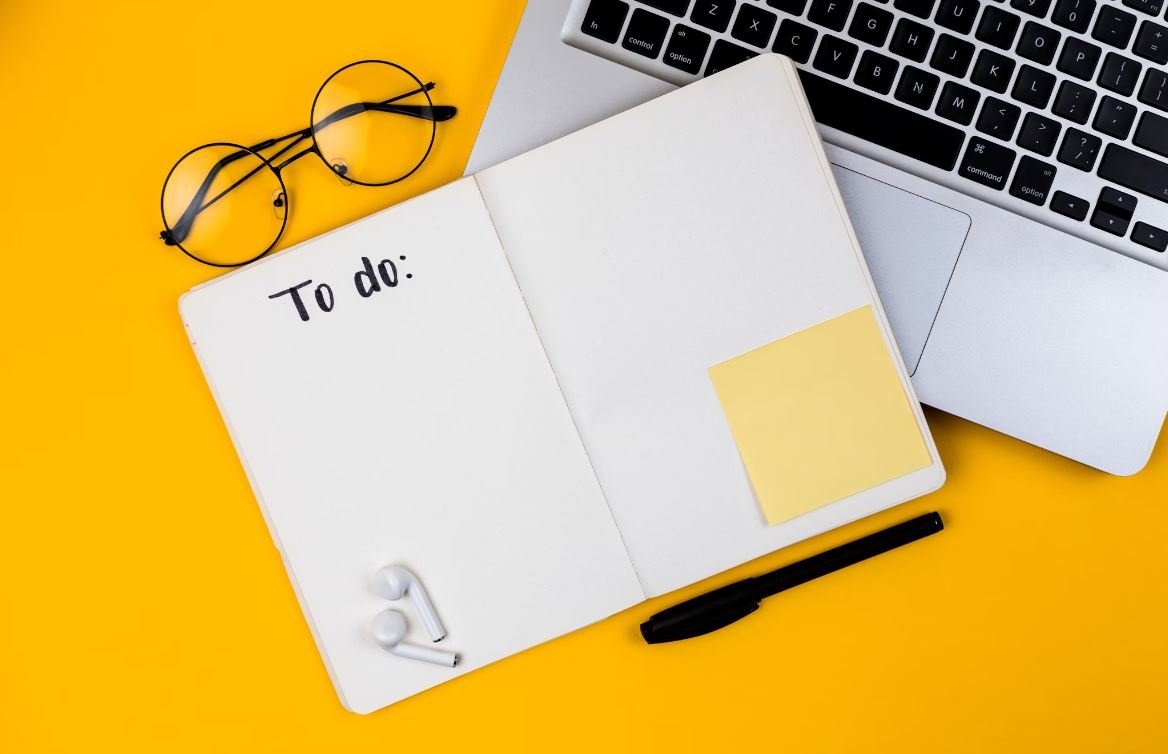
Data Mining Lift Analysis
Data mining lift analysis is a valuable technique used in various industries to identify patterns and relationships within data. It helps businesses make informed decisions by revealing insights that may not be apparent through traditional analysis. The following tables provide interesting data and information related to data mining lift and its applications.
Customer Purchase History
This table showcases the purchase history of customers in a retail store. The data includes customer names, their total purchases, and their most frequently bought items. It demonstrates how data mining lift analysis can uncover actionable insights to optimize marketing campaigns and improve customer satisfaction.
Insurance Claim Fraud Detection
In the insurance industry, the detection of fraudulent claims is crucial to prevent unnecessary losses. This table presents information on various claim attributes such as claim amount, claim type, and fraudulent indicators. Employing data mining lift analysis in this context allows insurers to identify suspicious patterns and uncover potential fraudsters.
Online User Behavior
Understanding the behavior of online users is essential for e-commerce businesses to enhance their user experience. This table represents user interactions on a website, including page views, click-through rates, and user demographics. By utilizing data mining lift analysis, businesses can personalize recommendations and advertisements to improve customer satisfaction and boost sales.
Loan Default Prediction
In the banking sector, predicting loan defaults helps lenders assess the creditworthiness of borrowers. This table exhibits several features such as income, employment status, and credit score, which are correlated with loan repayment defaults. Data mining lift analysis assists banks in identifying high-risk loan applicants and designing risk mitigation strategies.
Stock Market Prediction
Table showing the historical price movements of stocks, along with factors such as market sentiment, economic indicators, and news sentiment. By applying data mining lift analysis, investors can discover patterns and trends that can aid in predicting future stock price movements, enabling them to make informed investment decisions.
Churn Analysis
Customer churn is a concern for businesses across various industries. This table displays customer attributes, usage patterns, and churn indicators. Data mining lift analysis enables businesses to identify factors contributing to churn, allowing them to implement targeted retention strategies and minimize customer loss.
Social Media Sentiment Analysis
In the era of social media, understanding public sentiment is crucial for businesses to manage their brand reputation effectively. This table showcases sentiment scores associated with brand mentions on various social media platforms. Employing data mining lift analysis allows companies to gain valuable insights into public opinion, enabling them to tailor their strategies accordingly.
Weather Data Analysis
Table presenting historical weather data, including temperature, humidity, and precipitation levels. Applying data mining lift analysis to this type of data can reveal correlations between weather conditions and various outcomes, such as sales volumes, energy consumption, or transportation delays.
Website Conversion Rate Optimization
This table illustrates website conversion rate data, including different variations of landing pages, conversion rates, and associated factors such as user demographics. By utilizing data mining lift analysis, businesses can identify the most effective webpage layout, content, and design elements to optimize conversion rates and maximize customer acquisition.
Healthcare Diagnosis Accuracy
Accurate diagnosis is critical in the healthcare industry. This table showcases patient medical records, symptoms, and corresponding diagnoses made by physicians. Employing data mining lift analysis in healthcare datasets can help identify patterns indicative of specific diseases, contributing to accurate and timely diagnoses.
From customer purchase history and insurance fraud detection to stock market prediction and healthcare diagnosis accuracy, data mining lift analysis offers valuable insights across numerous domains. Employing this technique equips businesses with the ability to make data-driven decisions, optimize operations, and gain a competitive edge in the modern era.
Data Mining Lift – Frequently Asked Questions
What is data mining?
Data mining is the process of discovering patterns and useful information from large datasets. It involves extracting and analyzing data to uncover hidden patterns, relationships, and insights that can be used for decision-making and predicting future trends.
Why is data mining important?
Data mining plays a crucial role in various industries as it helps organizations gain a competitive advantage. It enables businesses to make data-driven decisions, identify trends and patterns, detect anomalies, and improve overall efficiency and effectiveness.
What are the main steps in the data mining process?
The main steps in the data mining process include data collection, data cleaning, data transformation, data modeling, data evaluation, and deployment. Each step involves various techniques and algorithms to extract meaningful insights from the data.
What are the key techniques used in data mining?
Some of the key techniques used in data mining are classification, clustering, association rule mining, regression analysis, and anomaly detection. These techniques allow for the extraction of valuable information from complex datasets.
What are the benefits of data mining?
Data mining offers several benefits, including improved decision-making, increased revenue, cost reduction, enhanced customer satisfaction, fraud detection, and risk assessment. It helps organizations gain insights that can lead to better business strategies and processes.
What are some challenges in data mining?
There are several challenges in data mining, such as data quality issues, scalability problems, privacy concerns, selecting appropriate algorithms, and interpretability of results. Overcoming these challenges requires expertise in data analysis and knowledge of domain-specific requirements.
What industries benefit from data mining?
Data mining has applications in various industries, including retail, healthcare, finance, telecommunications, marketing, and manufacturing. These industries can leverage data mining techniques to optimize operations, improve customer satisfaction, and drive business growth.
What types of data can be used in data mining?
Data mining can be applied to different types of data, such as structured data (e.g., databases), unstructured data (e.g., text documents), semi-structured data (e.g., XML files), and multimedia data (e.g., images, videos). The ability to analyze diverse datasets enhances the potential insights.
What ethical considerations are important in data mining?
Ethical considerations in data mining include ensuring data privacy and security, obtaining informed consent for data usage, avoiding discrimination and bias in decision-making, being transparent about data collection and usage practices, and complying with relevant regulations and laws.
What are the future trends in data mining?
Some future trends in data mining include the integration of artificial intelligence and machine learning techniques, increased focus on real-time data analysis, advancements in big data analytics, and the development of more efficient and scalable algorithms for handling large datasets.