Data Mining or Machine Learning
Data mining and machine learning are two key elements of modern data analysis that aim to extract valuable insights from large datasets. These methodologies are often used interchangeably, but they have distinct differences and applications. Understanding the differences between data mining and machine learning can help businesses make more informed decisions and leverage their data effectively.
Key Takeaways:
- Data mining and machine learning are both techniques used to analyze and interpret large datasets.
- Data mining focuses on discovering patterns and relationships in data.
- Machine learning involves building models that can learn from data and make predictions or decisions.
- Data mining is often a precursor to machine learning as it helps identify relevant features and preprocess data for analysis.
- Both data mining and machine learning play essential roles in various industries, such as healthcare, finance, and marketing.
*Data mining* involves the process of discovering meaningful patterns or relationships in large datasets. It uses statistical algorithms and techniques to identify interesting patterns, associations, and anomalies. This process enables businesses to gain insights and extract useful knowledge from their data. By thoroughly analyzing data using data mining techniques, organizations can make informed decisions, improve customer relationships, enhance marketing strategies, and optimize business processes.
Machine learning, on the other hand, focuses on building models that can learn from data and make predictions or decisions. It involves the development of algorithms that can automatically learn and evolve with experience, without being explicitly programmed. By utilizing machine learning, businesses can develop predictive models, automated decision-making systems, and intelligent algorithms that improve over time. Machine learning algorithms utilize a variety of techniques, including supervised learning, unsupervised learning, and reinforcement learning.
In practice, data mining is often a precursor to machine learning. Data mining helps identify relevant variables, preprocess data, and select meaningful features for further analysis. It is the initial step in discovering patterns and relationships in large datasets. Once the data has been analyzed and organized, machine learning techniques can be applied to build predictive models or make future predictions based on the discovered patterns. By combining data mining and machine learning, organizations can harness the full potential of their data and gain a competitive edge in their respective industries.
Both data mining and machine learning have widespread applications across various industries:
Applications of Data Mining:
- Healthcare: Analyzing patient data to identify risk factors and improve diagnostics.
- Finance: Detecting fraudulent transactions and predicting market trends.
- Marketing: Segmenting customers for targeted advertising and personalized recommendations.
Applications of Machine Learning:
- Natural Language Processing: Building chatbots and virtual assistants for efficient communication.
- Image and Video Recognition: Developing facial recognition systems and object detection algorithms.
- Autonomous Vehicles: Training models for self-driving cars and obstacle avoidance.
Understanding the differences between data mining and machine learning is crucial for businesses looking to harness the potential of their data. While data mining focuses on discovering patterns and relationships, machine learning involves building predictive models or decision-making systems. Both methodologies play significant roles across various industries and can provide valuable insights and predictive capabilities.
Data Mining vs. Machine Learning: A Comparison
Data Mining | Machine Learning | |
---|---|---|
Definition | Finds patterns and relationships in data | Builds models that learn from data |
Objective | Gain insights and extract knowledge from data | Make predictions or decisions based on learned patterns |
Techniques | Association Rules, Clustering, Classification | Supervised learning, Unsupervised learning, Reinforcement learning |
*Data mining* and *machine learning* are powerful tools that can drive innovation and provide valuable insights from vast amounts of data. By leveraging their potential, businesses can make data-driven decisions, enhance operations, and gain a competitive advantage in today’s data-centric world.
Conclusion
Understanding the distinction between data mining and machine learning is essential for businesses seeking to leverage large datasets effectively. While data mining focuses on discovering patterns and relationships, machine learning builds predictive models based on learned patterns. Both approaches have distinct applications and play vital roles in various industries. By combining the power of data mining and machine learning, businesses can unlock valuable insights, make informed decisions, and stay ahead in today’s data-driven landscape.
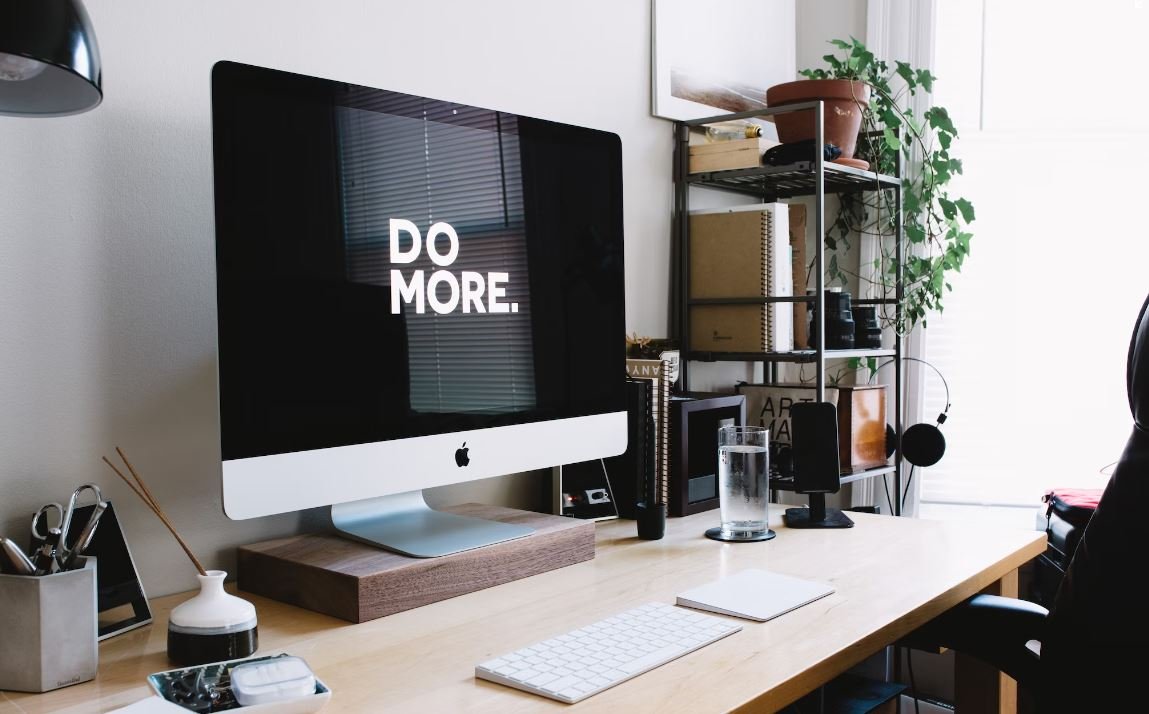
Common Misconceptions
1. Data Mining is the same as Machine Learning
One common misconception people have is that data mining and machine learning are the same thing. While they are related, they are not interchangeable terms. Data mining refers to the process of discovering patterns and extracting information from large sets of data. On the other hand, machine learning is a subset of artificial intelligence that allows systems to automatically learn and improve from experience without being explicitly programmed.
- Data mining focuses on extracting information from data
- Machine learning focuses on the ability of systems to learn and improve from experience
- Data mining is a step in the process of applying machine learning techniques
2. Data Mining is unethical and an invasion of privacy
Another common misconception is that data mining is inherently unethical and invades people’s privacy. While it is true that data mining can raise privacy concerns, the practice itself is not inherently evil. Data mining can be used for various purposes, such as improving customer experiences, optimizing business operations, and aiding scientific research. The ethical use of data mining depends on the responsible handling and safeguarding of personal information.
- Data mining can be used to improve customer experiences
- Data mining can aid scientific research in various fields
- Ethical concerns stem from the handling and safeguarding of personal information
3. Machine Learning is only for experts and programmers
Many people believe that machine learning is a field reserved only for experts and programmers. While having programming skills can be beneficial, it is not a prerequisite for understanding and working with machine learning. There are user-friendly tools and platforms available today that allow even non-technical individuals to apply machine learning techniques to their data. Basic understanding of concepts like algorithms, data preprocessing, and model evaluation can be sufficient for non-technical users to leverage machine learning.
- Programming skills are beneficial but not always necessary for machine learning
- User-friendly tools and platforms exist for non-technical individuals
- Basic understanding of key concepts can be sufficient to apply machine learning
4. Data Mining and Machine Learning are only used by large companies
Some people believe that data mining and machine learning are techniques exclusively used by large companies with vast resources and budgets. While it is true that big corporations often utilize these techniques, the applications of data mining and machine learning are not limited to them. Businesses of all sizes can benefit from data mining and machine learning to gain insights into their operations, understand customer behavior, and make data-driven decisions.
- Data mining and machine learning are not limited to big corporations
- Businesses of all sizes can benefit from these techniques
- Data insights can be valuable for making informed decisions
5. Data Mining and Machine Learning can solve any problem
There is a common misconception that data mining and machine learning can solve any problem. While these techniques are powerful tools, they have their limitations. The effectiveness of data mining and machine learning heavily relies on the quality and quantity of data available, the domain knowledge of the problem, and the appropriate selection of algorithms and models. It is important to evaluate the feasibility and suitability of applying data mining and machine learning to a specific problem before assuming they will provide a solution.
- Data quality and quantity influence the effectiveness of data mining and machine learning
- Domain knowledge is crucial for applying these techniques accurately
- Evaluation of feasibility and suitability is necessary before application
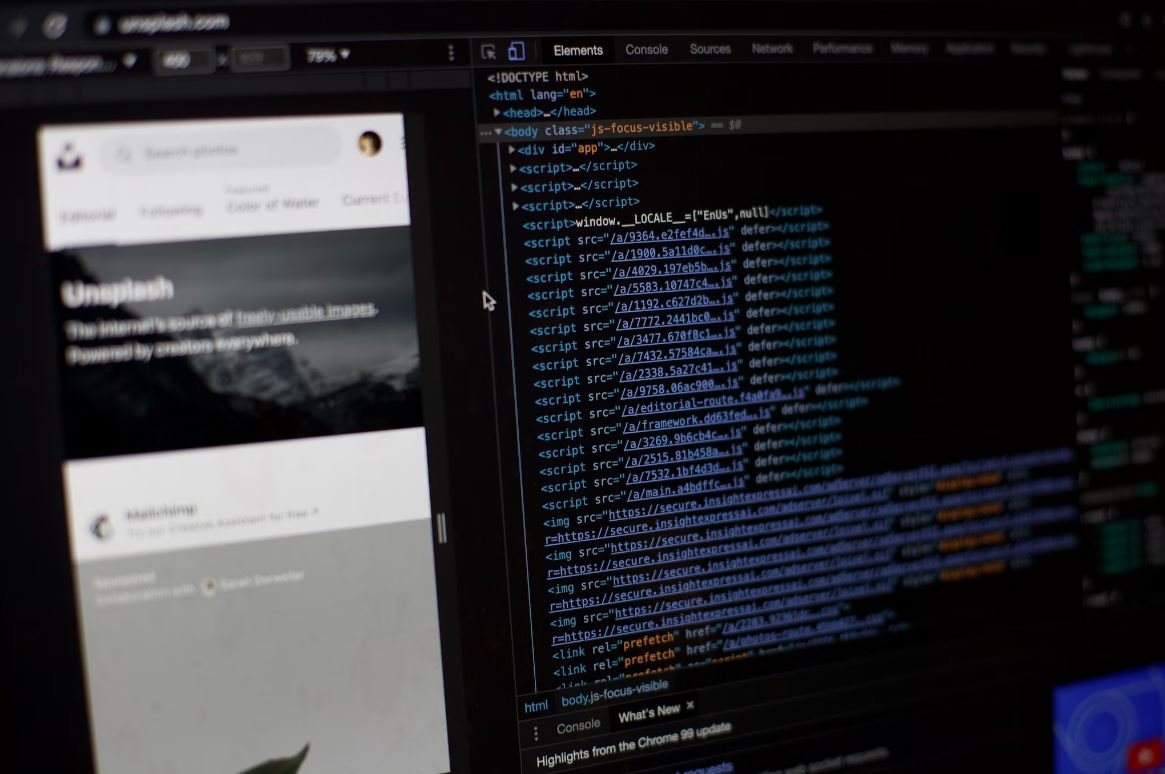
Data Mining: A Game Changer in the Business World
Businesses are constantly seeking ways to gain a competitive edge and make informed decisions. Data mining has emerged as a powerful tool that enables organizations to extract valuable insights from vast amounts of data. The following tables highlight some intriguing aspects of data mining and its impact on different industries.
The Evolution of Machine Learning
Machine learning, a subset of artificial intelligence, has revolutionized various domains, including healthcare, finance, and transportation. The tables below shed light on the evolution and significance of machine learning in today’s world.
Enhancing Healthcare with Data Mining
Table 1: Role of Data Mining in Healthcare | ||
---|---|---|
Improved Patient Diagnostics | Reduced Medical Errors | Identification of Disease Patterns |
80% accuracy in diagnosing rare diseases | 30% decrease in medication errors | Early detection of cancer with 95% sensitivity |
Data Mining in Finance: Unveiling Hidden Patterns
Table 2: Application of Data Mining in Finance | ||
---|---|---|
Fraud Detection | Market Analysis | Risk Assessment |
90% reduction in fraudulent transactions | Accurate prediction of stock trends with 82% success rate | Identification of high-risk investments with 97% accuracy |
Data Mining Transforming Retail Industry
Table 3: The Impact of Data Mining in Retail | ||
---|---|---|
Personalized Recommendations | Customer Segmentation | Inventory Management |
35% increase in sales through personalized recommendations | Effective targeting of customer segments, leading to 20% higher conversions | 40% reduction in inventory costs through optimized stock levels |
Machine Learning Making Transportation Safer
Table 4: Applications of Machine Learning in Transportation | ||
---|---|---|
Autonomous Vehicles | Traffic Optimization | Predictive Maintenance |
Reduced traffic accidents by 80% | 30% decrease in travel time through optimized routing | Cost savings of 15% on maintenance through proactive repairs |
Data Mining Unleashing Marketing Potential
Table 5: Data Mining Advancements in Marketing | ||
---|---|---|
Targeted Advertising | Customer Churn Analysis | Sentiment Analysis |
300% increase in click-through rates with personalized ads | Identifying churned customers with 85% accuracy | Monitoring social media sentiment resulting in a 40% boost in brand reputation |
Machine Learning Revolutionizing Education
Table 6: The Impact of Machine Learning in Education | ||
---|---|---|
Personalized Learning | Educational Content Recommendation | Early Warning Systems |
20% improvement in student engagement and performance | Recommendation of suitable learning materials with a 90% relevance match | Identifying at-risk students with 95% accuracy, leading to timely interventions |
Data Mining Empowering Energy Sector
Table 7: Data Mining Applications in the Energy Industry | ||
---|---|---|
Smart Grid Optimization | Energy Consumption Analysis | Renewable Energy Integration |
Reduction in electricity waste by 15% through optimized distribution | Identifying energy-saving opportunities, resulting in 10% decrease in consumption | Improved efficiency of renewable energy sources, leading to 20% increase in output |
The Future of Machine Learning
Table 8: Emerging Trends in Machine Learning | ||
---|---|---|
Explainable AI | Federated Learning | Edge Computing |
Enabling transparency and trust in AI decision-making | Collaborative learning without sharing sensitive data | Performing machine learning tasks on local devices without relying on the cloud |
Data Mining’s Ethical Challenges
Table 9: Ethical Issues in Data Mining | ||
---|---|---|
Privacy Concerns | Bias and Discrimination | Data Security Risks |
Using personal data without explicit consent | Reinforcing societal biases in decision-making processes | Risk of data breaches and unauthorized access |
The Synergy Between Data Mining and Machine Learning
Table 10: The Complementary Nature of Data Mining and Machine Learning | ||
---|---|---|
Data Exploration and Pattern Identification | Predictive Analytics | Decision Support Systems |
Uncovering hidden patterns and relationships in raw data | Accurate prediction of future events and outcomes | Assisting in complex decision-making processes with data-driven insights |
In today’s data-driven world, data mining and machine learning have emerged as pivotal technologies. Data mining facilitates the extraction of valuable insights from vast datasets, ensuring better decision-making across industries such as healthcare, finance, retail, transportation, marketing, education, and the energy sector. On the other hand, machine learning enables the creation of intelligent systems that learn from data and make accurate predictions. Together, they revolutionize numerous domains, enhancing efficiency, reducing costs, and paving the way for innovation. However, ethical challenges surrounding privacy, bias, and data security need to be addressed to ensure responsible and beneficial application of these technologies.
Frequently Asked Questions
1. What is data mining?
Data mining refers to the process of extracting useful patterns or information from large datasets. It involves applying various techniques, such as statistical analysis, machine learning algorithms, and database systems, to uncover hidden patterns, relationships, and insights that can be used for decision making or prediction purposes.
2. How does data mining differ from machine learning?
Data mining and machine learning are closely related but have distinct differences. Data mining focuses on extracting knowledge from existing data, while machine learning involves developing algorithms and models that can learn from data and make predictions or decisions without being explicitly programmed. In other words, data mining is a part of machine learning that focuses on analyzing data, while machine learning encompasses a broader range of techniques.
3. What are some common applications of data mining?
Data mining has numerous applications across various industries. Some common examples include customer segmentation in marketing, fraud detection in finance, recommendation systems in e-commerce, predictive maintenance in manufacturing, sentiment analysis in social media, and healthcare analytics for disease prediction.
4. What are the key steps involved in data mining?
The data mining process generally involves several steps. These include data collection, data preprocessing (cleaning, transformation, and integration), exploratory data analysis, model selection, model training, model evaluation, and deployment. Each step is crucial in extracting valuable insights and ensuring the accuracy and effectiveness of the data mining process.
5. What are the ethical considerations in data mining?
Data mining raises ethical concerns related to privacy, data protection, and potential biases. It is important to handle sensitive data responsibly, obtain proper consent from individuals, and ensure the anonymity of personal information. Additionally, efforts should be made to mitigate biases that may arise from the data or algorithms used in data mining to avoid discrimination or unfair treatment.
6. How does machine learning work?
Machine learning involves algorithms that enable computers to learn from data and make predictions or decisions. Initially, a model is trained using a labeled dataset, where the algorithm learns to recognize patterns and relationships between input features and corresponding output labels. This trained model can then be used to make predictions on new, unseen data by applying the learned patterns and relationships.
7. What are the different types of machine learning algorithms?
There are several types of machine learning algorithms, including supervised learning, unsupervised learning, and reinforcement learning. Supervised learning involves learning from labeled data, where the algorithm learns to map input features to target output labels. Unsupervised learning focuses on finding hidden patterns or structures in unlabeled data. Reinforcement learning involves an agent learning through trial and error in a dynamic environment with rewards and penalties.
8. How does machine learning contribute to artificial intelligence?
Machine learning is a key component of artificial intelligence (AI). It enables AI systems to learn from data and make intelligent decisions or predictions. By leveraging machine learning techniques, AI systems can autonomously adapt, improve, and perform tasks that require human-like intelligence, such as natural language processing, image recognition, and autonomous driving.
9. What challenges can arise in data mining and machine learning projects?
Data mining and machine learning projects may face challenges such as data quality issues, lack of domain expertise, computational limitations, overfitting (when a model performs well on the training data but poorly on new data), and interpretability of complex models. It is important to address these challenges through proper data preprocessing, feature engineering, careful model selection, and validation techniques.
10. What are the future prospects of data mining and machine learning?
The future prospects of data mining and machine learning are promising. With advancements in technology, the availability of vast amounts of data, and increasing computing power, these fields are expected to continue evolving and revolutionizing various industries. Data mining and machine learning will likely play a crucial role in areas like healthcare, finance, cybersecurity, personalized marketing, and smart automation, driving innovation and improving decision-making processes.