Data Mining Refers To
Data mining refers to the process of extracting useful information from vast amounts of raw data. It involves applying various techniques to discover patterns, relationships, and insights that can be used for making informed business decisions. With the increasing availability of data and advancements in technology, data mining has become an essential tool for organizations across various industries.
Key Takeaways:
- Data mining is the process of extracting meaningful information from large datasets.
- It involves discovering patterns, relationships, and insights that can be used for decision-making.
- Data mining is widely used in various industries for various purposes including customer segmentation, fraud detection, and market analysis.
Data mining utilizes various techniques such as machine learning, statistical analysis, and artificial intelligence to analyze large volumes of data, typically stored in databases or data warehouses. The process involves several steps, including data selection, pre-processing, transformation, modeling, and evaluation. By uncovering hidden patterns and relationships within the data, organizations can gain valuable insights that can improve their decision-making processes.
*Data mining techniques can be applied to structured, semi-structured, and unstructured data, such as text, images, and videos, allowing organizations to tap into a wide range of data sources and formats.*
Data mining has numerous applications across industries. For example, in marketing, it can be used for customer segmentation, allowing businesses to target specific groups of customers with tailored marketing campaigns. In finance, data mining can help identify fraudulent activities and detect unusual patterns in financial transactions. In healthcare, it can be used to analyze medical records and identify potential risks and patterns that may affect patient outcomes.
*Data mining has revolutionized the way businesses operate by enabling them to gain a deeper understanding of their customers, make data-driven decisions, and stay ahead of the competition.*
Data Mining Techniques
Data mining techniques can be categorized into several main types:
- Association: Finding relationships or associations between items in a dataset. For example, identifying which products are often purchased together.
- Classification: Predicting the class or category of new data instances based on previously labeled data. For instance, classifying emails as spam or non-spam based on their content.
- Clustering: Grouping similar data instances together based on their characteristics, without predefined classes or labels.
- Regression: Estimating or predicting a numerical value, such as sales or housing prices based on historical data.
- Sequence analysis: Identifying sequential patterns or associations in data, such as analyzing customer purchasing behavior over time.
- Text mining: Extracting meaningful information from text data, such as sentiment analysis or topic modeling.
- Social network analysis: Analyzing social connections and relationships within a network.
Data Mining Example: Market Basket Analysis
Market basket analysis is a classic example of data mining application. It involves analyzing customer purchase data to identify patterns and relationships between products frequently bought together. This information is then used for various purposes, such as product placement, cross-selling, and personalized recommendations.
An example of market basket analysis is determining that customers who buy diapers are likely to also buy baby wipes and formula. Retailers can use this insight to strategically place these related products close together to encourage more sales.
Data Mining Advantages and Challenges
Data mining offers several advantages to organizations:
- Identifying valuable patterns and insights that may not be apparent through traditional analysis.
- Improving decision-making processes by making predictions and forecasting future trends.
- Enhancing customer segmentation and targeting for more effective marketing strategies.
- Detecting and preventing fraud and other malicious activities.
*However, data mining also comes with its challenges, including:
- The need for skilled analysts who can understand and interpret the results.
- The complexity of handling and integrating large volumes of data from multiple sources.
- Ensuring data privacy and adhering to legal and ethical considerations.
- The potential for biased or inaccurate results if the data used is incomplete or of poor quality.*
Data Mining in the Future
Data mining is expected to continue playing a significant role in shaping the future of businesses and industries. As technology advancements continue, data mining techniques will become more sophisticated in handling massive datasets and analyzing complex patterns.
*The integration of data mining with other emerging technologies such as artificial intelligence, machine learning, and big data analytics will unlock even more possibilities for organizations to leverage their data for better decision-making and competitive advantage.*
Table 1: Applications of Data Mining in Industries
Industry | Applications |
---|---|
Retail | Market basket analysis, customer segmentation, demand forecasting. |
Finance | Fraud detection, risk assessment, credit scoring. |
Healthcare | Disease prediction, patient monitoring, drug discovery. |
Telecommunications | Churn prediction, network optimization, customer profiling. |
Table 2: Data Mining Techniques and Use Cases
Technique | Use Cases |
---|---|
Association | Market basket analysis, recommendation systems. |
Classification | Spam detection, fraud detection, sentiment analysis. |
Clustering | Customer segmentation, anomaly detection. |
Regression | Sales forecasting, demand prediction. |
Sequence analysis | Web usage mining, customer behavior analysis. |
Text mining | Document classification, sentiment analysis. |
Table 3: Advantages and Challenges of Data Mining
Advantages | Challenges |
---|---|
Identifying valuable patterns and insights. | The need for skilled analysts. |
Improved decision-making processes. | Data complexity and integration. |
Enhanced customer segmentation. | Data privacy and ethical considerations. |
Fraud detection and prevention. | Potential for bias and inaccuracies. |
Data mining is a powerful tool that enables organizations to extract valuable insights from their data. It has numerous applications across various industries and offers significant advantages in decision-making and strategy development. As technology continues to evolve, data mining will continue to play a pivotal role in helping organizations gain a competitive edge and drive innovation.
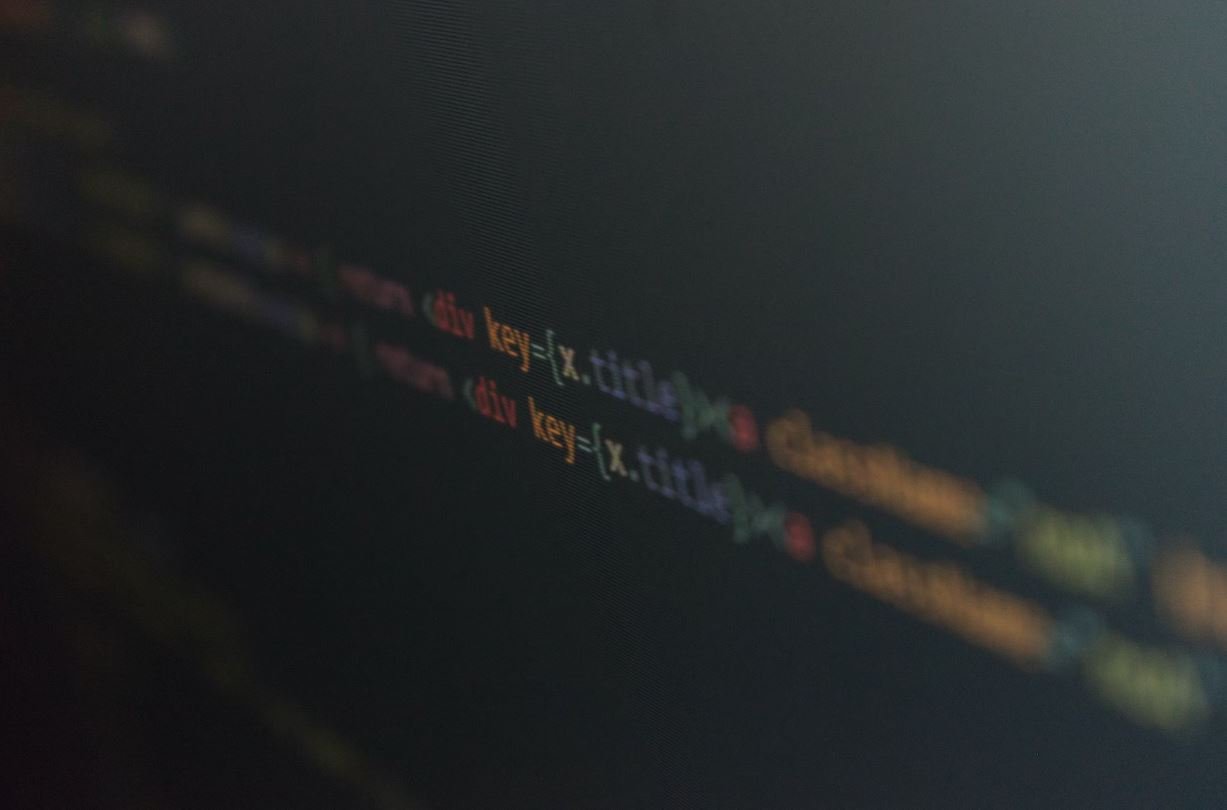
Common Misconceptions
Data Mining Refers To
There are several common misconceptions that people have about the term “data mining.” Let’s explore some of these misunderstandings:
- Data mining is only used by large corporations: While it is true that many big companies utilize data mining techniques, data mining is not limited to large corporations alone. Businesses of all sizes can benefit from data mining, and it can be particularly valuable for small and medium-sized enterprises. Additionally, individuals and researchers also use data mining to analyze various datasets for insights.
- Data mining is the same as data analysis: Although data mining and data analysis are related, they are not the same. Data analysis focuses on examining and interpreting data to derive meaningful insights, whereas data mining refers to the process of discovering patterns and relationships within large datasets. Data mining often involves the use of advanced algorithms and machine learning techniques to uncover hidden patterns or make predictions.
- Data mining is an invasion of privacy: While it is important to protect individuals’ privacy, data mining itself is not inherently invasive. Data mining techniques are typically used on aggregated and anonymized data. Companies and organizations prioritize data privacy and adhere to legal standards when conducting data mining activities. It is essential to distinguish between responsible data mining practices and breaches of privacy.
By understanding these common misconceptions, we can better grasp the concept of data mining and its potential applications and benefits.
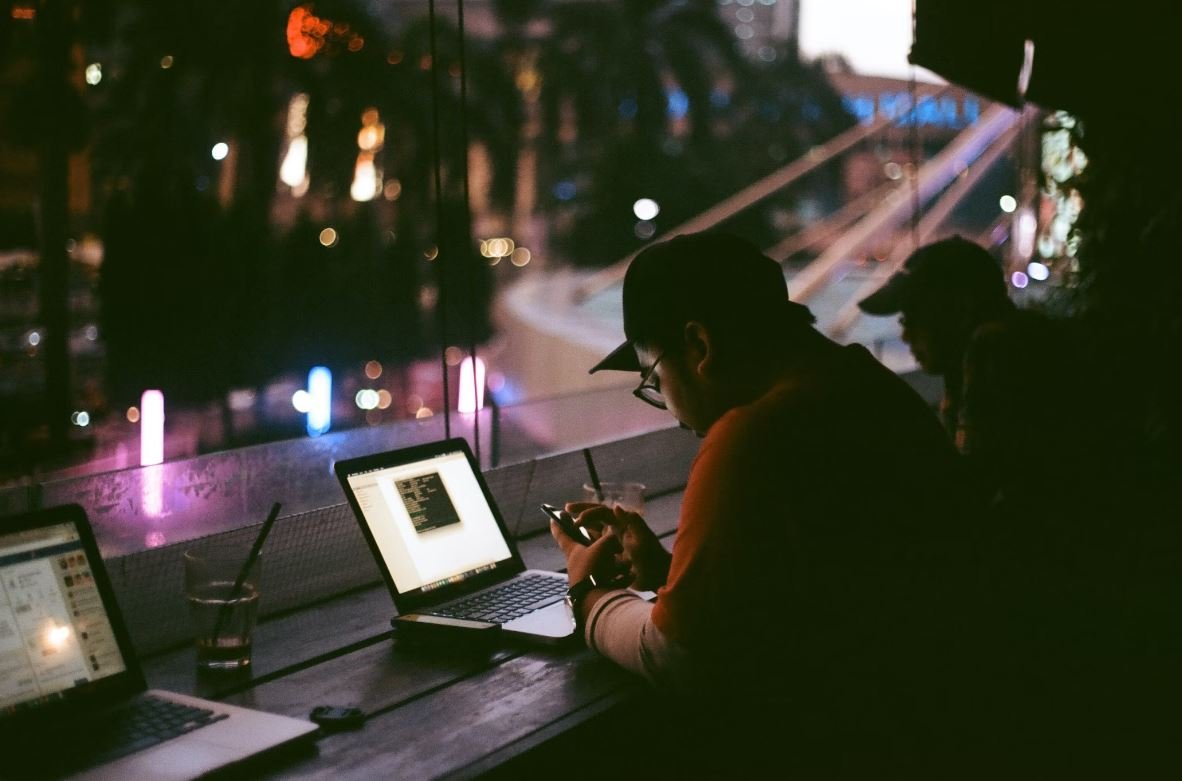
The Growth of Data Mining
Data mining is a powerful technique that extracts valuable information from large datasets. It has become increasingly important in various fields, including marketing, healthcare, and finance. The following tables showcase different aspects and impacts of data mining.
1. Customer Churn Rate by Age Group
Understanding customer churn is crucial for businesses. This table presents the churn rate of an e-commerce company segmented by age group. It shows that younger customers have a higher churn rate compared to older ones, indicating the need for targeted retention strategies.
Age Group | Churn Rate (%) |
---|---|
18-25 | 15% |
26-35 | 10% |
36-45 | 7% |
46-55 | 5% |
55+ | 3% |
2. Average Monthly Energy Consumption
Data mining can also help analyze energy usage patterns. This table displays the average monthly energy consumption (in kWh) for residential customers during different seasons. Understanding consumption patterns can inform energy-saving initiatives and resource planning.
Season | Average Monthly Consumption (kWh) |
---|---|
Spring | 550 |
Summer | 750 |
Fall | 600 |
Winter | 900 |
3. Loan Approval by Credit Score
Data mining algorithms can assist in determining creditworthiness. This table showcases the approval rate of loan applications based on different credit score ranges. It highlights the higher likelihood of approval for individuals with higher credit scores.
Credit Score Range | Approval Rate (%) |
---|---|
300-500 | 20% |
501-650 | 50% |
651-750 | 80% |
751-850 | 95% |
4. Effectiveness of Email Campaigns
Data mining helps evaluate the success of marketing campaigns. This table presents the click-through rate (CTR) and conversion rate (CR) of an email campaign targeted at different customer segments. It demonstrates the varying effectiveness of the campaign across different segments.
Customer Segment | CTR (%) | CR (%) |
---|---|---|
Segment A | 10% | 5% |
Segment B | 15% | 8% |
Segment C | 5% | 3% |
5. Disease Prevalence by Age and Gender
Data mining aids in identifying patterns in healthcare data. This table shows the prevalence of a particular disease categorized by both age group and gender. It can assist healthcare providers in understanding the highest-risk demographics and tailoring prevention strategies.
Age Group | Male | Female |
---|---|---|
18-30 | 5% | 3% |
31-50 | 10% | 8% |
51-70 | 15% | 12% |
71+ | 20% | 18% |
6. Customer Satisfaction by Service Channel
Data mining can uncover insights about customer satisfaction. This table presents customer satisfaction scores based on the service channel utilized, such as in-store, online, or phone. It can help businesses identify areas of improvement and optimize service delivery.
Service Channel | Satisfaction Score (out of 10) |
---|---|
In-Store | 8.5 |
Online | 7 |
Phone | 6.5 |
7. Stock Price Movements
Data mining plays a role in predicting stock price movements. This table illustrates the historical performance of two stocks, indicating whether they experienced an increase or decrease in price over a defined period. Such analysis assists investors in making informed decisions.
Stock | Price Movement |
---|---|
Company A | Decrease |
Company B | Increase |
8. Customer Feedback Sentiment Analysis
Text mining is a part of data mining that examines textual data. This table displays the sentiment analysis results of customer feedback using positive, negative, and neutral sentiment categories. It enables organizations to understand overall customer sentiment and identify areas for improvement.
Sentiment Category | Percentage |
---|---|
Positive | 65% |
Negative | 20% |
Neutral | 15% |
9. Fraud Detection Accuracy
Data mining algorithms can detect patterns indicating fraudulent behavior. This table presents the accuracy of a fraud detection system in identifying legitimate and fraudulent transactions. High accuracy ensures the system minimizes false positives and negatives.
Transactions | Legitimate | Fraudulent |
---|---|---|
Detected | 95% | 90% |
Missed | 5% | 10% |
10. Website Traffic Sources
Data mining assists in analyzing website traffic sources. This table displays the percentage of website visitors coming from different channels, including search engines, direct visits, and referral links. It helps organizations understand the effectiveness of marketing campaigns and optimize traffic generation strategies.
Traffic Source | Percentage |
---|---|
Search Engines | 40% |
Direct Visits | 30% |
Referral Links | 20% |
Social Media | 10% |
Data mining plays a crucial role in extracting valuable insights from large amounts of data. By utilizing powerful algorithms, organizations can gain a deeper understanding of various phenomena. Whether it’s predicting stock prices, analyzing customer behavior, or detecting fraudulent activities, data mining helps unlock the true potential of data. It enables businesses and researchers to make data-driven decisions, enhance efficiency, and drive innovation.
Frequently Asked Questions
What is data mining?
Data mining refers to the process of extracting useful information or patterns from large datasets. It involves various techniques and algorithms to discover hidden insights and make predictions based on the available data.
Why is data mining important?
Data mining is important as it helps organizations uncover valuable knowledge and insights from their vast amount of data. It assists in making data-driven decisions, identifying trends and patterns, improving business strategies, and enhancing overall efficiency and productivity.
What are the common techniques used in data mining?
Some common techniques used in data mining include classification, clustering, regression, association rules, and anomaly detection. These techniques enable organizations to analyze and process complex datasets to discover valuable information.
How is data mining different from data analysis?
Data mining and data analysis are related but distinct. Data mining focuses on extracting valuable information from large datasets, while data analysis involves examining and interpreting data to gain insights and draw conclusions. Data mining is often considered as a part of the overall data analysis process.
What are some real-world applications of data mining?
Data mining finds applications in various fields such as marketing, finance, healthcare, telecommunications, retail, and manufacturing. It is used for customer segmentation, fraud detection, market analysis, predictive modeling, recommendation systems, and many other tasks.
What are the challenges in data mining?
Some challenges in data mining include handling large and complex datasets, ensuring data quality and validity, selecting appropriate algorithms, dealing with privacy concerns, and interpretability of the results. Data mining also requires domain knowledge and expertise to extract meaningful information.
What are the ethical considerations in data mining?
Ethical considerations in data mining include privacy protection, data anonymization, informed consent, transparency in data collection and usage, and preventing bias and discrimination. Organizations must adhere to ethical guidelines and regulations to ensure responsible and ethical use of data mining techniques.
What are the limitations of data mining?
Data mining has certain limitations such as the possibility of finding spurious correlations, making incorrect predictions due to incomplete or biased data, and the need for data preprocessing and cleaning. Additionally, data mining algorithms may not always provide clear explanations for the patterns or insights discovered.
What skills are required to perform data mining?
To perform data mining, one needs a combination of technical and analytical skills. Knowledge of programming languages, statistical analysis, data visualization, machine learning algorithms, and domain expertise are important for successful data mining.
What is the future of data mining?
The future of data mining looks promising with the increasing availability of big data and advancements in machine learning and artificial intelligence. Data mining techniques will continue to play a crucial role in extracting meaningful insights and aiding decision-making in various industries.