Data Mining Success Stories
Data mining, also known as knowledge discovery in databases, is the process of extracting valuable information and patterns from large sets of data. It involves using a variety of techniques and algorithms to find hidden relationships and insights that can drive business decisions. In recent years, data mining has become increasingly popular and has led to some remarkable success stories. This article will explore a few of these success stories and highlight the key takeaways from each.
Key Takeaways
- Data mining is the process of extracting valuable information and patterns from large datasets.
- Success stories in data mining demonstrate the potential impact and value it can bring to businesses.
- Techniques and algorithms used in data mining can uncover hidden relationships and insights.
- Data mining has applications in various industries, including finance, healthcare, and marketing.
- Data mining success stories highlight the importance of data quality and domain knowledge.
**One notable data mining success story** is the case of Netflix. By analyzing their vast collection of user data, Netflix was able to develop a powerful recommendation engine that suggests personalized content to its users. *This algorithm-driven approach has greatly improved user experience and contributed to the company’s success.*
Data mining has also been instrumental in the field of healthcare. **Researchers at the University of Pennsylvania** used data mining techniques to predict patient readmissions. Through a combination of patient demographic data, medical history, and clinical variables, they were able to identify high-risk patients and develop interventions to reduce readmissions. *This has led to improved patient outcomes and cost savings for healthcare providers.*
Success Story | Industry | Impact |
---|---|---|
Netflix recommendation engine | Entertainment | Improved user experience |
Patient readmission prediction | Healthcare | Reduced readmissions and cost savings |
In the finance industry, **credit card companies** have successfully applied data mining techniques to detect fraudulent activities. By analyzing patterns and anomalies in transaction data, these companies can identify suspicious transactions and prevent fraud in real-time. *This has not only minimized financial losses for customers but also increased trust in the industry’s security measures.*
Data mining has also revolutionized targeted marketing efforts. **Amazon**, for example, uses data mining to analyze customer behavior and preferences. By understanding their customers’ purchasing patterns and interests, Amazon can provide personalized product recommendations and optimize their marketing campaigns. *This has resulted in increased sales and customer satisfaction.*
Success Story | Industry | Impact |
---|---|---|
Credit card fraud detection | Finance | Minimized financial losses and increased trust |
Targeted marketing by Amazon | Retail | Increased sales and customer satisfaction |
**In conclusion**, data mining has proven to be incredibly valuable across various industries. From entertainment to healthcare, finance to retail, businesses have leveraged data mining techniques to gain invaluable insights and achieve significant improvements. By harnessing the power of data, organizations can enhance decision-making, optimize operations, and ultimately drive success.
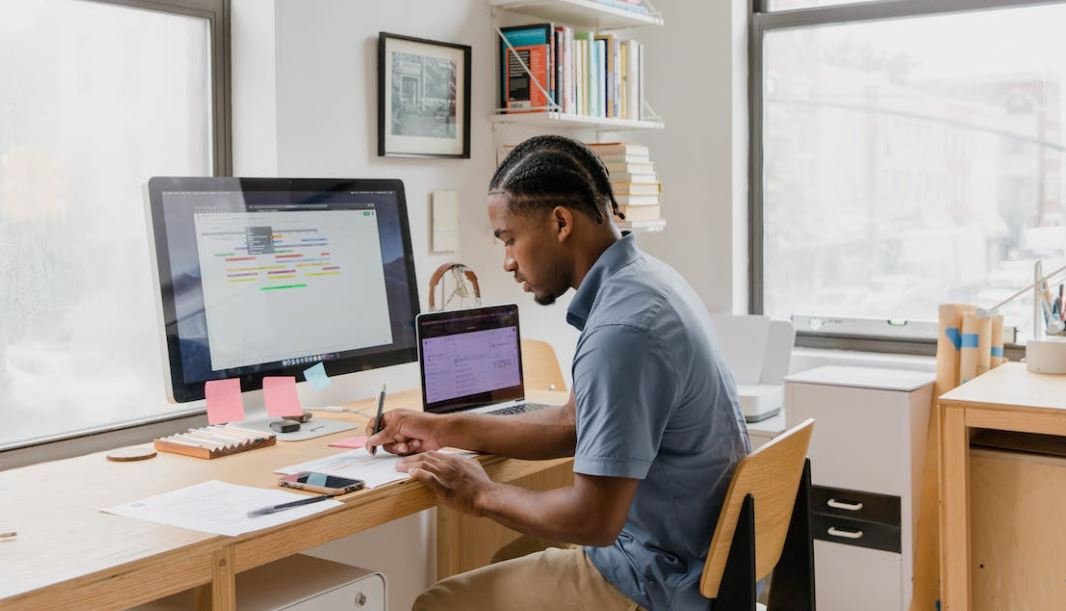
Common Misconceptions
1. Data mining is a magical solution that guarantees success
One common misconception about data mining is that it is a magical solution that guarantees success. However, data mining is just a tool that helps companies uncover patterns, correlations, and insights from large sets of data. It requires careful analysis and interpretation to generate meaningful results.
- Data mining is not a quick fix solution
- Data mining requires expertise in data analysis
- Data mining success is not guaranteed
2. Data mining allows companies to predict the future with certainty
Another misconception is that data mining allows companies to predict the future with certainty. While data mining can help identify trends and patterns, it cannot predict future events with 100% accuracy. Factors such as external influences and unforeseen circumstances can impact future outcomes.
- Data mining provides insights, not certainties
- Data mining cannot account for unforeseen events
- Data mining is just one factor in making predictions
3. Data mining is only useful for large companies
Some people believe that data mining is only useful for large companies with vast amounts of data. However, data mining techniques can be applied by businesses of all sizes. Even small companies can benefit from analyzing their customer data and making data-driven decisions.
- Data mining can be applied by businesses of all sizes
- Data mining can help small companies gain a competitive edge
- Data mining is scalable to fit the needs of different organizations
4. Data mining compromises privacy and security
Privacy and security concerns often arise when discussing data mining. While there have been instances of misuse of personal data, data mining techniques can be implemented with proper privacy and security measures in place. It is important for businesses to adhere to ethical guidelines and protect customer information.
- Data mining can be carried out with privacy and security measures
- Data mining can help identify and mitigate privacy risks
- Data mining can be used responsibly and ethically
5. Data mining can replace human decision-making
Lastly, some people believe that data mining can replace human decision-making entirely. However, data mining should be seen as a tool to support decision-making, not replace it. Human judgement, creativity, and experience are still crucial for understanding the context and implications of the data.
- Data mining complements human decision-making
- Data mining helps humans make informed decisions
- Data mining supports decision-making but does not replace it
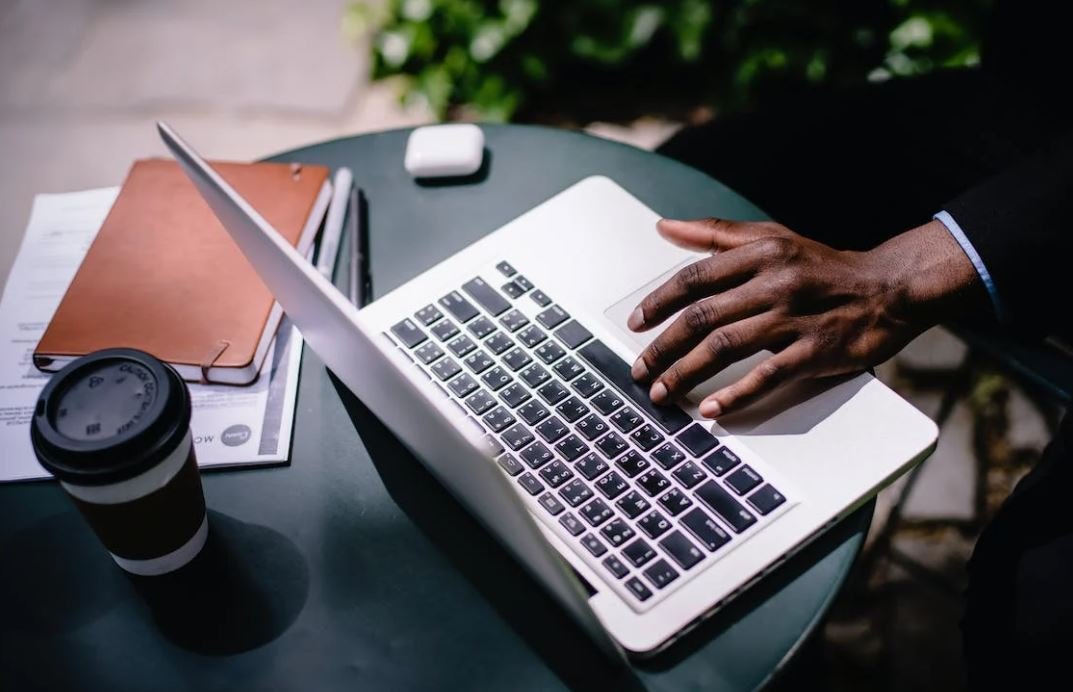
Data Mining Success Stories
Data mining, the process of discovering patterns and extracting valuable information from large datasets, has been instrumental in driving innovation and improving decision-making across various industries. From healthcare to finance, data mining has unearthed valuable insights that have led to remarkable achievements. This article highlights ten fascinating data mining success stories that demonstrate the power and potential of this transformative practice.
Unraveling Genetic Mysteries
By applying data mining techniques to genetic data, scientists have been able to uncover crucial relationships between genes and diseases. In a study analyzing genomic data from thousands of individuals, researchers identified a genetic variant associated with a rare autoimmune disorder. This breakthrough discovery paves the way for the development of targeted treatments and personalized medicine.
Road Traffic Prediction
Data mining has revolutionized transportation management by enabling accurate road traffic predictions. By analyzing historical traffic data and external factors such as weather and events, transportation authorities can optimize traffic flow, reduce congestion, and enhance road safety. This proactive approach has resulted in significant time and cost savings for commuters and increased overall transportation efficiency.
Predictive Maintenance in Manufacturing
Data mining has proven vital in predictive maintenance, helping manufacturers anticipate equipment failures before they occur. By monitoring sensor data and analyzing patterns, machine learning algorithms can detect early warning signs of potential breakdowns. Manufacturers can then schedule maintenance activities at optimal times, minimizing downtime, and reducing costs associated with emergency repairs.
Customer Behavior Analysis
Through data mining, businesses gain comprehensive insights into customer behavior, enabling them to improve marketing strategies and enhance customer experiences. By analyzing purchase histories and demographic data, companies can identify patterns and preferences, personalize recommendations, and tailor marketing campaigns to specific segments. This targeted approach boosts customer satisfaction, retention rates, and ultimately, the bottom line.
Fraud Detection
Financial institutions rely on data mining algorithms to detect and prevent fraudulent activities. By analyzing transactional data and identifying anomalous patterns, machine learning models can flag suspicious activities such as credit card fraud or identity theft. This proactive approach protects both financial institutions and customers, saving billions of dollars annually.
Improving Crop Yields
Data mining has played a pivotal role in agriculture by helping farmers optimize crop yields. By analyzing historical weather data, soil conditions, and crop performance, predictive models can identify optimal planting times, fertilizer application rates, and irrigation schedules. These insights enable farmers to make informed decisions, maximize productivity, and minimize environmental impact.
Enhancing Healthcare Outcomes
Data mining has empowered healthcare providers to improve patient outcomes and deliver more personalized care. By analyzing electronic health records, patient demographics, and clinical data, machine learning algorithms can predict disease progression, identify at-risk patients, and optimize treatment plans. This data-driven approach has led to improved diagnoses, decreased hospital readmissions, and ultimately, saved lives.
Optimizing Supply Chain Management
Data mining facilitates more efficient supply chain management by uncovering valuable insights from vast amounts of data. By analyzing historical sales data, customer demand patterns, and logistics information, companies can optimize inventory levels, streamline distribution, and anticipate market fluctuations. These optimizations result in reduced costs, improved customer service, and enhanced overall supply chain performance.
Crime Prevention and Law Enforcement
Data mining has revolutionized crime prevention and law enforcement strategies. By applying advanced analytics to law enforcement data, police departments can identify crime hotspots, forecast crime trends, and allocate resources accordingly. This data-driven approach aids in proactively preventing crime, enhancing public safety, and improving overall community well-being.
Conclusion
Data mining has emerged as a powerful tool for extracting knowledge and valuable insights from large datasets, fueling innovation and transforming industries. From unraveling genetic mysteries to optimizing supply chain management, the ten success stories highlighted here demonstrate the immense potential of data mining in improving decision-making, increasing efficiency, and enhancing outcomes across diverse fields. As technology continues to advance, the applications of data mining will only expand, unlocking new possibilities and shaping an even more data-driven future.
Frequently Asked Questions
Question 1
What is data mining?
Data mining is the process of extracting useful information and patterns from large datasets. It involves discovering hidden patterns, relationships, and insights that can be used for decision-making purposes.
Question 2
How does data mining contribute to business success?
Data mining helps businesses gain valuable insights into their customers, markets, and operations. By analyzing large amounts of data, businesses can identify patterns and trends, make informed decisions, improve strategic planning, optimize marketing campaigns, detect fraud, and enhance customer experiences.
Question 3
Can you provide examples of successful data mining applications?
Certain successful data mining applications include personalized product recommendations by e-commerce platforms, predictive maintenance in manufacturing industries, credit scoring in the finance sector, targeted marketing campaigns by retailers, and fraud detection systems in the banking industry.
Question 4
What are the key steps involved in data mining?
The key steps in data mining include data collection, data cleaning and preprocessing, exploratory data analysis, model selection, model evaluation, and interpretation of results. Each step plays a crucial role in the overall success of data mining projects.
Question 5
How can organizations ensure the success of their data mining projects?
To ensure the success of data mining projects, organizations should have clear objectives and well-defined project scopes. They should also invest in high-quality data, employ skilled data analysts and domain experts, use appropriate data mining techniques, and continuously evaluate and iterate their models based on feedback and changing business needs.
Question 6
What are the challenges associated with data mining?
Some common challenges in data mining include data quality issues, data privacy concerns, selecting the right algorithms and models, handling large volumes of data, dealing with missing or incomplete data, and ensuring the ethical use of data.
Question 7
What are the ethical considerations in data mining?
Ethical considerations in data mining involve ensuring data privacy, obtaining informed consent from individuals, avoiding discrimination or bias in data analysis, and using the mined data responsibly and in accordance with applicable laws and regulations.
Question 8
How is data mining different from data analytics?
Data mining focuses on extracting patterns and insights from large datasets. It involves discovering hidden relationships and making predictions. On the other hand, data analytics encompasses a broader range of activities, including data mining, statistical analysis, data visualization, and deriving actionable insights from data.
Question 9
What are the limitations of data mining?
Data mining has certain limitations such as the need for high-quality data, requirement of expertise in data analysis, potential for false discoveries or overfitting, inability to handle unstructured or qualitative data, and the challenge of interpreting complex models.
Question 10
How can I get started with data mining?
To get started with data mining, you can begin by learning the fundamentals of data analysis, statistics, and machine learning. Familiarize yourself with popular data mining tools and programming languages like Python or R. Practicing on small datasets and participating in online courses or tutorials can also help you gain practical experience and build a foundation in data mining.