Data Mining Technology
Data mining technology is a vital tool in today’s digital age, allowing businesses and organizations to extract meaningful insights from large sets of data. With the ability to analyze and interpret vast amounts of information, data mining technology has revolutionized various industries and paved the way for data-driven decision making.
Key Takeaways
- Data mining technology enables businesses to extract valuable insights from large datasets.
- It helps uncover patterns, trends, and correlations that drive informed decision-making.
- Data mining technology has transformed industries such as marketing, finance, healthcare, and more.
Data mining involves the use of algorithms and statistical models to discover patterns and extract knowledge from vast amounts of data. **By leveraging advanced computational power** and sophisticated algorithms, organizations gain the ability to analyze various types of data, including structured, unstructured, and semi-structured data, to uncover valuable information hidden beneath the surface. *This technology allows businesses to unlock hidden insights that may have otherwise gone unnoticed.*
The Processes of Data Mining
Data mining technology encompasses several fundamental processes:
- Data collection: Gathering relevant data from various sources such as databases, data warehouses, and web resources.
- Data preprocessing: Cleaning, transforming, and preparing the data for analysis.
- Data modeling: Utilizing algorithms and statistical models to identify patterns and relationships within the data.
- Interpretation and evaluation: Analyzing the results of the data mining process to draw meaningful conclusions and make informed decisions.
Through data mining technology, organizations can uncover valuable insights that can be utilized in various ways. For example, businesses can utilize data mining to **identify consumer behavior patterns**, enabling more targeted marketing and personalized advertising campaigns. *This technology can unlock hidden opportunities for businesses to enhance customer engagement and drive revenue growth.* Furthermore, financial institutions can leverage data mining to **detect fraudulent activities**, helping minimize risks and prevent monetary losses.
Data Mining Examples
Let’s explore some real-world examples of how data mining technology has transformed different industries:
Industry | Data Mining Application |
---|---|
Healthcare | Identifying disease patterns and predicting patient outcomes |
Retail | Segmenting customers for targeted marketing campaigns |
Manufacturing | Optimizing production processes and supply chain management |
Data mining technology has proven to be an invaluable asset in today’s data-rich world. With the ability to turn vast amounts of data into actionable insights, organizations gain a competitive edge in decision-making and problem-solving. By unlocking patterns and relationships buried within the data, businesses can make more informed decisions, improve operational efficiency, and accelerate growth.
Ultimately, data mining technology empowers organizations to harness the power of data for strategic advantage and business success. By adopting data mining techniques, businesses can unlock valuable insights that drive innovation, optimize processes, and gain a deeper understanding of their customers and markets they operate in. Embracing data mining technology is no longer a luxury; it has become a necessity for businesses that strive to thrive in the data-driven era.
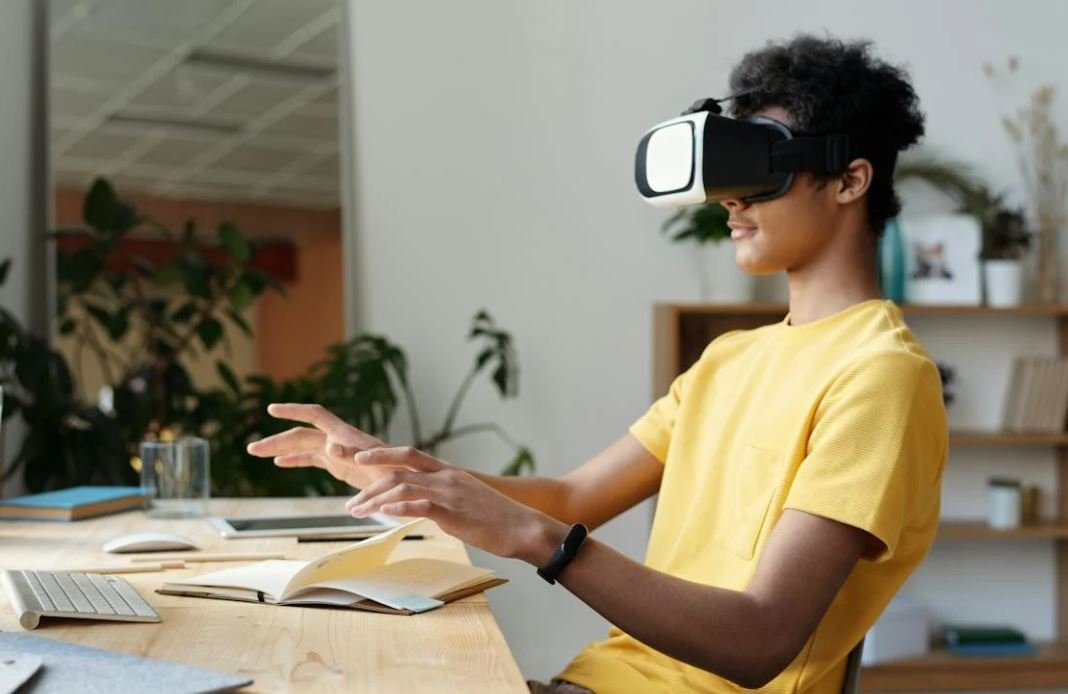
Data Mining Technology
Common Misconceptions
There are several common misconceptions that people often have about data mining technology. It is important to clarify these misunderstandings to better understand the capabilities and limitations of this powerful tool.
- Data mining is the same as data warehousing.
- Data mining is only useful for large organizations.
- Data mining violates privacy.
One common misconception surrounding data mining is that it is the same as data warehousing. While the two are related, they have distinct differences. Data warehousing refers to the process of storing data from various sources in a central location for easy analysis and reporting. On the other hand, data mining refers to the extraction of useful patterns and insights from the data stored in the warehouse.
- Data warehousing stores data, while data mining analyzes it.
- Data mining relies on the data collected in data warehouses.
- Data mining is a subset of data warehousing activities.
Another misconception is that data mining is only beneficial for large organizations. While it is true that data mining can be especially advantageous for organizations with vast amounts of data, small and medium-sized businesses can also benefit from its use. Data mining allows businesses of all sizes to gain valuable insights from their data, identify trends, predict customer behavior, and make data-driven decisions.
- Data mining is beneficial for organizations of all sizes.
- Data mining can help small and medium-sized businesses improve their operations.
- Data mining is scalable and adaptable to the needs of organizations.
A common misconception surrounding data mining is that it violates privacy. While it is true that data mining involves analyzing large amounts of data, it does not necessarily mean that individual privacy is compromised. Data mining techniques focus on discovering patterns and trends in aggregated data, rather than identifying individuals. Furthermore, organizations implementing data mining technologies must adhere to stringent privacy regulations and ethical guidelines to protect individuals’ personal information.
- Data mining focuses on patterns, not individual identification.
- Data mining techniques prioritize privacy and adhere to regulations.
- Data mining can be used responsibly while protecting individual privacy.
In conclusion, understanding the common misconceptions surrounding data mining technology is crucial for its effective utilization. It is not synonymous with data warehousing, can benefit organizations of all sizes, and can be implemented in a privacy-conscious manner. By dispelling these misconceptions, businesses can harness the power of data mining to drive their decision-making processes and gain a competitive edge in today’s data-driven world.
- Dispelling misconceptions enables effective utilization of data mining.
- Data mining empowers decision-making and promotes competitiveness.
- Data mining is an essential tool in today’s data-driven world.
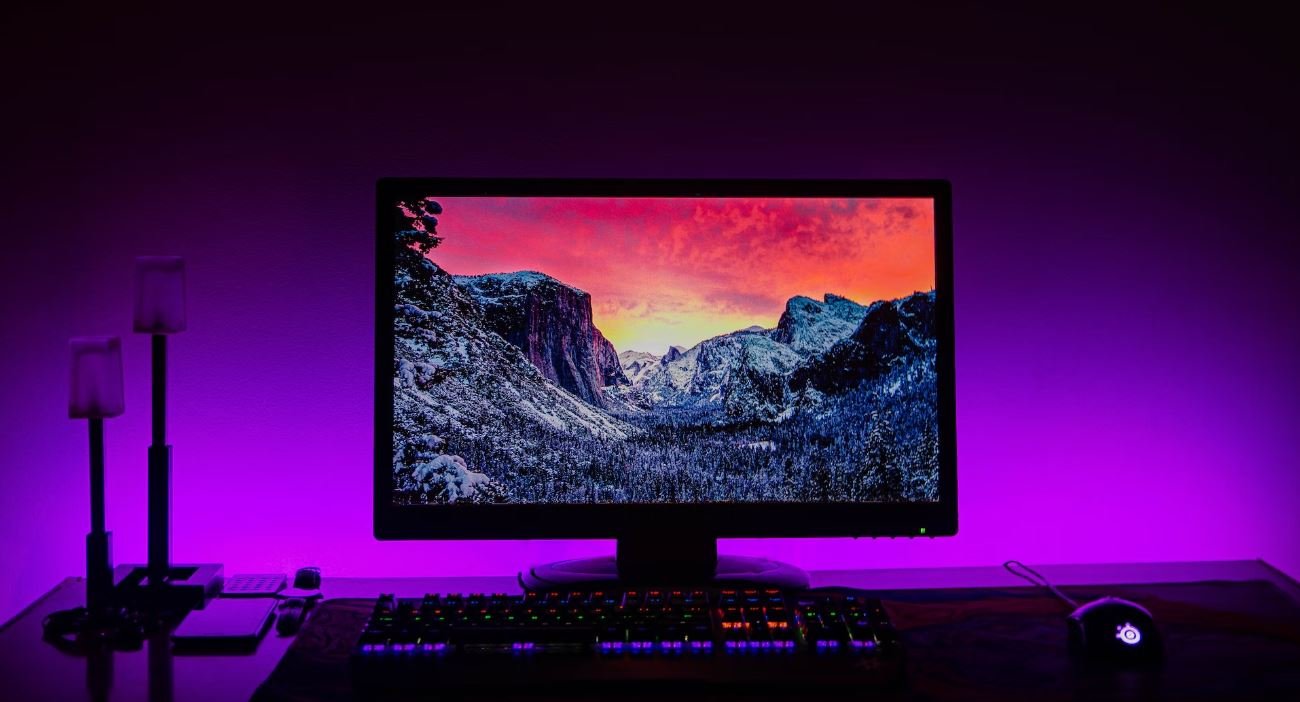
Data Mining Technology Enhancing Business Insights
Data mining technology is revolutionizing the way businesses gather, analyze, and utilize data to gain valuable insights. By examining large datasets, patterns and trends can be identified, enabling companies to make informed decisions and improve operational efficiency. The following tables highlight various aspects of data mining technology and its impact on different industries.
Retail Industry: Customer Segmentation
Customer segmentation is crucial for retailers to identify target markets and tailor their strategies accordingly. This table showcases the distribution of customer segments based on buying behavior, demographics, and preferences.
| Segment | Buying Behavior | Demographics | Preferences |
|———–|—————–|———————-|———————|
| Premium | Frequent | High-income earners | Luxury goods |
| Value | Occasional | Middle-income earners| Discounts |
| Trendy | Frequent | Young adults | Fashion-forward |
| Bargain | Frequent | Budget-conscious | Clearance items |
Finance Sector: Fraud Detection
Data mining technology plays a crucial role in identifying fraudulent activities in the finance sector. This table represents various factors that aid in fraud detection, such as transaction patterns, user behavior, and anomaly detection.
| Risk Factor | Transaction Patterns | User Behavior | Anomaly Detection |
|———————|—————————|———————-|———————|
| Unusual withdrawal | Rapid deposit fluctuations| Multiple logins | Suspicious IPs |
| Abnormal activity | Out-of-pattern purchases | Unexpected account access| Unusual location |
Healthcare Industry: Disease Prediction
Data mining technology can aid in predicting diseases and improving diagnosis accuracy. This table demonstrates several indicators utilized for disease prediction, including symptoms, medical history, and genetic predispositions.
| Disease | Symptoms | Medical History | Genetic Markers |
|———–|—————–|———————-|———————|
| Diabetes | Frequent urination| Family history | Genetic variations |
| Cancer | Unexplained weight loss | Previous treatments | Mutations |
| Asthma | Wheezing | Allergies | Genetic predisposition |
| Hypertension | Headaches | High blood pressure | Genetic mutations |
Education Sector: Student Performance
Data mining technology enables educators to analyze student performance and implement personalized learning strategies. This table exhibits factors impacting student performance, including attendance, hours of study, and socio-economic background.
| Factor | Attendance | Study Hours | Socio-Economic Background |
|————–|——————-|———————|—————————-|
| High Performers | Regular attendance | Extensive study | Affluent |
| Average Performers | Occasional absences | Moderate study | Middle-class |
| Low Performers | Irregular attendance | Minimal study | Disadvantaged |
Marketing: Customer Retention
Data mining technology helps marketing teams forecast customer churn and implement effective retention strategies. The table below identifies factors influencing customer retention, such as average order value, customer satisfaction ratings, and engagement levels.
| Factor | Average Order Value | Satisfaction Ratings | Engagement Levels |
|———————|———————–|————————|——————-|
| Retained Customers | Higher than average | Positive | Active participation |
| At-Risk Customers | Slight decrease | Neutral | Decreased |
| Churned Customers | Significant decrease| Negative | Inactive participation |
Transportation Industry: Route Optimization
Data mining technology aids in optimizing transportation routes and reducing travel time. This table presents various factors considered for route optimization, including traffic congestion, distance, and delivery time.
| Route | Traffic Congestion | Distance (in km) | Estimated Delivery Time |
|————–|———————–|———————-|——————————-|
| Route A | High congestion | 50 | 2 hours |
| Route B | Moderate congestion | 40 | 1.5 hours |
| Route C | Low congestion | 45 | 1.75 hours |
Insurance Sector: Risk Assessment
Data mining technology helps insurance companies assess risks and set appropriate premiums. This table illustrates factors considered for risk assessment, including age, previous claims history, and the type of insured assets.
| Risk Factor | Age Range | Claims History | Insured Assets |
|———————|———————-|——————-|——————|
| High Risk | 18-25 years | Multiple Claims | Luxury vehicles |
| Moderate Risk | 26-40 years | Few Claims | Residential property |
| Low Risk | 41-60 years | No Claims | Health insurance |
Telecommunications: Network Performance
Data mining technology assists telecommunication companies in analyzing network performance and resolving connectivity issues. The table below showcases factors influencing network performance, including signal strength, average dropped calls, and data speed.
| Factor | Signal Strength | Dropped Calls (per day) | Data Speed (Mbps) |
|———————|———————|—————————–|—————–|
| Excellent Performance| Strong signal | None | >100 |
| Average Performance | Moderate signal | Few | 50-100 |
| Poor Performance | Weak signal | Frequent | <50 |
Energy Sector: Consumption Analysis
Data mining technology allows the energy sector to analyze consumption patterns and develop energy-saving strategies. This table outlines factors influencing energy consumption, such as usage fluctuations, appliance efficiency, and weather conditions.
| Factor | Usage Fluctuations | Appliance Efficiency | Weather Conditions |
|——————|———————-|————————|———————–|
| High Consumption | Sudden spikes | Lower efficiency | Extreme temperatures |
| Average Consumption | Moderate fluctuations | Average efficiency | Mild temperatures |
| Low Consumption | Consistent | High efficiency | Moderate temperatures |
Conclusion
Data mining technology has become an invaluable tool in various industries, resulting in improved decision-making, enhanced efficiency, and accurate predictions. By effectively harnessing data’s power, businesses can gain a competitive edge and meet customer needs more effectively. The power of data mining technology continues to drive innovation and shape the future of industries worldwide.
Frequently Asked Questions
Questions about Data Mining Technology
What is data mining technology?
Data mining technology is a process that extracts meaningful patterns and information from large datasets. It involves using various algorithms and techniques to uncover hidden relationships, trends, and insights within the data.