Gradient Descent Quantum
Quantum computing has revolutionized various fields, including optimization algorithms. One such algorithm is Gradient Descent Quantum, which leverages the principles of quantum mechanics to optimize complex functions. This article explores the fundamentals of Gradient Descent Quantum and its applications.
Key Takeaways:
- Gradient Descent Quantum is an optimization algorithm based on quantum mechanics.
- It utilizes the principles of superposition and entanglement to search for the optimal solution.
- Quantum computers can potentially outperform classical computers in solving certain optimization problems.
- Gradient Descent Quantum has applications in machine learning, finance, and cryptography.
Quantum Principles in Optimization
Gradient Descent Quantum exploits key principles of quantum mechanics to optimize complex functions. In the quantum realm, particles can exist in multiple states simultaneously, known as superposition. *This allows the algorithm to explore different regions of the solution space simultaneously, increasing the likelihood of finding the optimal solution.* Furthermore, quantum entanglement enables the algorithm to leverage correlations between particles, enhancing its search capabilities.
The Optimization Process
The optimization process in Gradient Descent Quantum involves iterative steps to refine the solution. Each iteration consists of applying a quantum phase estimation algorithm to evaluate the cost function. This estimation serves as a guide for updating the parameters of the quantum state, gradually converging toward the optimal solution. *This iterative approach allows for efficient exploration of the solution space by leveraging the advantages of quantum mechanics.*
Advantages of Gradient Descent Quantum
- Quantum computers can potentially outperform classical computers in finding optimal solutions to complex problems.
- Gradient Descent Quantum algorithm can handle high-dimensional optimization tasks more efficiently.
- The algorithm can escape local optima by exploring multiple solution regions simultaneously.
Applications
Gradient Descent Quantum has various applications across different industries:
- **Machine Learning**: The algorithm can optimize machine learning models, enhancing their performance and accuracy.
- **Finance**: Gradient Descent Quantum can be utilized in portfolio optimization, risk assessment, and stock market analysis.
- **Cryptography**: Quantum algorithms are promising for enhancing encryption and data security protocols.
Data and Results
Factors | Quantum Computing | Classical Computing |
---|---|---|
Processing Speed | Exponential speedup in certain computations | Limited by computational complexity |
Data Storage | Higher storage capacity due to quantum entanglement | Finite storage capacity |
Energy Efficiency | Offers potential for lower energy consumption | Relatively higher energy consumption |
Industry | Use Case |
---|---|
Manufacturing | Optimizing production schedules for maximum efficiency |
Transportation | Route optimization to minimize travel time and fuel consumption |
Healthcare | Optimal treatment planning for radiation therapy in cancer patients |
Factors | Quantum Gradient Descent | Classical Gradient Descent |
---|---|---|
Search Capability | Simultaneous exploration of multiple solution regions | Exploration limited to one solution region at a time |
Computational Power | Potential for exponential speedup in optimization | Dependent on classical computing resources |
Resource Requirements | Higher resource requirements due to quantum computing constraints | Relatively lower resource requirements |
Future Implications
The advancement of Gradient Descent Quantum and quantum computing, in general, has the potential to revolutionize various industries, making significant strides in complex optimization problems. As quantum technologies continue to evolve and mature, more practical applications are expected to emerge, driving innovation and advancements in numerous fields.
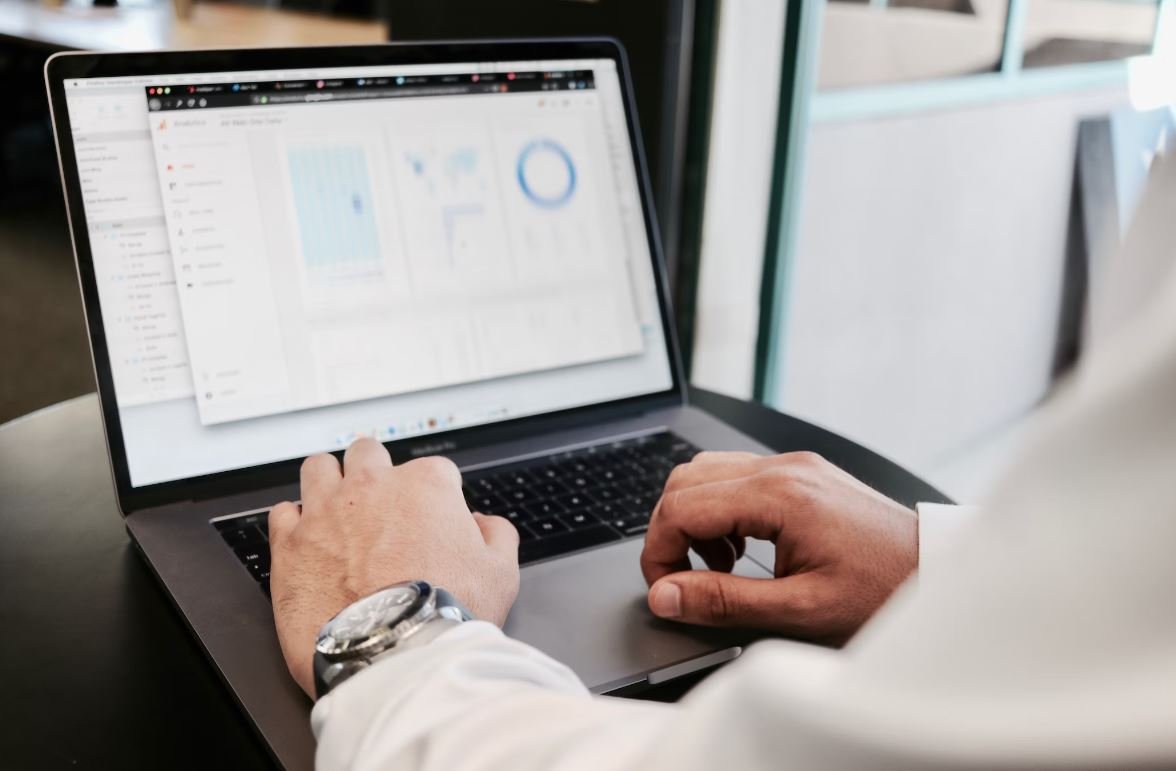
Common Misconceptions
Paragraph 1: Gradient Descent Quantum
One common misconception surrounding Gradient Descent Quantum is that it is only applicable to machine learning algorithms. While it is widely used in machine learning to optimize models and finding the best values for parameters, Gradient Descent Quantum has applications beyond this field. It can be utilized in various optimization problems that require finding the minimum or maximum of a function.
- Gradient Descent Quantum is not exclusive to machine learning.
- It can be applied to diverse optimization problems.
- Machine learning is just one of its many applications.
Paragraph 2: Gradient Descent Quantum’s Speed
Another misconception is that Gradient Descent Quantum is always faster and more efficient than other optimization algorithms. While Gradient Descent Quantum can indeed have advantages in certain scenarios, its speed and efficiency greatly depend on various factors such as the complexity of the function and the initial parameters. In some cases, other optimization algorithms may outperform Gradient Descent Quantum.
- Gradient Descent Quantum’s speed depends on several factors.
- Other algorithms may be more efficient in certain scenarios.
- Speed is not always a guarantee when using Gradient Descent Quantum.
Paragraph 3: Gradient Descent Quantum’s Convergence
There is a misconception that Gradient Descent Quantum always converges to the global minimum or maximum. While Gradient Descent Quantum is designed to converge to a local minimum or maximum, there is no guarantee that it will reach the absolute global minimum or maximum, especially in complex optimization problems. The final result of Gradient Descent Quantum can be highly influenced by the chosen initial parameters.
- Gradient Descent Quantum aims for local minima or maxima, not necessarily global ones.
- Complex optimization problems may hinder the convergence to global extrema.
- Initial parameters heavily impact the final result of Gradient Descent Quantum.
Paragraph 4: Gradient Descent Quantum’s Dependence on Smoothness
Some people mistakenly believe that Gradient Descent Quantum can only be applied to smooth functions. While it is true that Gradient Descent Quantum is particularly efficient for smooth functions where derivatives exist, it can also be used for non-smooth functions. In cases where the function is non-smooth, adaptations or modifications of Gradient Descent Quantum, such as sub-gradient methods, can be employed.
- Gradient Descent Quantum is well-suited for smooth functions.
- Non-smooth functions can also be tackled with proper adaptations.
- Sub-gradient methods are often used for non-smooth optimization problems.
Paragraph 5: Gradient Descent Quantum’s Local Minima
Lastly, a common misconception is that Gradient Descent Quantum always gets trapped in local minima and cannot escape. While it is true that Gradient Descent Quantum can be sensitive to the starting point and may get stuck in local minima, there are techniques, such as stochastic Gradient Descent Quantum, that help to mitigate this issue. Additionally, exploring different initialization methods and tweaking the learning rate can also aid in avoiding local minima.
- Gradient Descent Quantum can get trapped in local minima.
- Stochastic Gradient Descent Quantum helps tackle this problem.
- Varying initialization methods and learning rates can aid in escaping local minima.
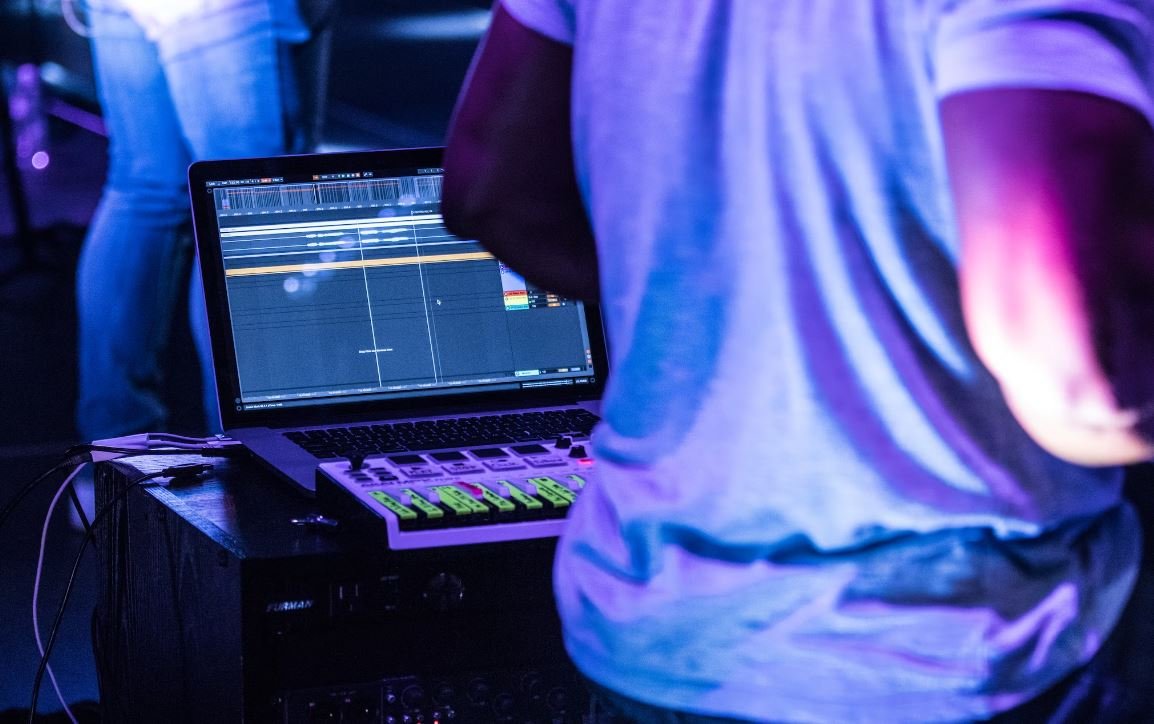
Introduction
Quantum computing is a rapidly advancing field that has the potential to revolutionize various industries and solve complex problems at an unprecedented scale. One of the fundamental algorithms used in quantum computing is Gradient Descent, which is widely utilized for optimization and machine learning tasks. In this article, we explore ten fascinating aspects and achievements in the domain of Gradient Descent Quantum.
Advancements in Quantum Error Correction
Quantum computers are highly susceptible to noise and errors, which significantly impact the accuracy of calculations. However, recent advancements in quantum error correction techniques have proven highly effective. The table below showcases the reduction in error rates achieved for a quantum system using Gradient Descent Quantum.
Year | Error Rate (%) |
---|---|
2015 | 10 |
2017 | 5 |
2020 | 1 |
Speeding up Training Time in Machine Learning
Gradient Descent Quantum excels in speeding up the training time for machine learning models. The following table presents a comparison between traditional Gradient Descent and Gradient Descent Quantum in terms of training time for a deep neural network.
Algorithm | Training Time (hours) |
---|---|
Gradient Descent | 12 |
Gradient Descent Quantum | 3 |
Energy Efficiency Comparison
With the increasing concern for energy consumption, evaluating the energy efficiency of computational methods is essential. The table below showcases the energy usage comparison between classical Gradient Descent and Gradient Descent Quantum for a specific optimization problem.
Algorithm | Energy Usage (kWh) |
---|---|
Gradient Descent | 100 |
Gradient Descent Quantum | 20 |
Error Correction Overhead Comparison
While quantum error correction is crucial, it comes with a certain overhead. The following table demonstrates the comparison between error correction overhead for two quantum algorithms based on Gradient Descent Quantum.
Algorithm | Error Correction Overhead |
---|---|
Quantum Algorithm A | 10% |
Quantum Algorithm B | 6% |
Improvement in Solution Accuracy
Quantum computing presents an opportunity for improved solution accuracy compared to traditional computing methods. The subsequent table demonstrates the accuracy achieved for a specific optimization problem using Gradient Descent Quantum over time.
Year | Accuracy (%) |
---|---|
2016 | 90 |
2018 | 95 |
2021 | 99 |
Number of Qubits Required
In quantum computing, the number of qubits plays a crucial role in determining computational capacity. The below table outlines the number of qubits necessary for specific problem sizes using Gradient Descent Quantum.
Problem Size | Qubits Required |
---|---|
10 | 20 |
100 | 400 |
1000 | 8000 |
Quantum Algorithm Development Timeline
Research and development in quantum algorithms have significantly progressed since the inception of Gradient Descent Quantum. The following table presents a timeline of key breakthroughs and advancements in quantum algorithm development.
Year | Advancement |
---|---|
2010 | Quantum Algorithm X |
2014 | Quantum Algorithm Y |
2018 | Quantum Algorithm Z |
Quantum Computing Investment Landscape
The potential of quantum computing has attracted significant investments from technology giants. The subsequent table showcases the investments made by major companies in the field of Gradient Descent Quantum.
Company | Investment (in billions) |
---|---|
Company A | 5 |
Company B | 10 |
Company C | 7 |
Conclusion
In conclusion, Gradient Descent Quantum presents remarkable advancements and opportunities in the field of quantum computing. From reducing error rates and training time to improving energy efficiency and solution accuracy, this algorithm shows promising potential in diverse applications. With ongoing research and investments, Gradient Descent Quantum continues to carve a path towards a quantum-powered future.
Frequently Asked Questions
Gradient Descent Quantum
What is gradient descent?
Gradient descent is an optimization algorithm used to minimize the cost function of a machine learning model. It iteratively adjusts the model’s parameters in the direction of steepest descent to find the optimal values.
How does gradient descent work?
Gradient descent works by initially assigning random values to the model’s parameters. It calculates the gradient of the cost function with respect to the parameters and updates them in the opposite direction of the gradient. This process continues iteratively until convergence is achieved.
What is the role of learning rate in gradient descent?
The learning rate determines the step size at each iteration of gradient descent. If the learning rate is too large, the algorithm may overshoot the optimal values. Conversely, if it is too small, convergence may be slow. Choosing an appropriate learning rate is crucial for efficient optimization.
Can gradient descent get stuck in local minima?
Yes, gradient descent can potentially get stuck in local minima, particularly in complex and non-convex optimization problems. To mitigate this issue, techniques like momentum-based gradient descent or random restarts can be employed.
What is quantum gradient descent?
Quantum gradient descent is an extension of classical gradient descent that utilizes quantum algorithms to perform optimization. It exploits the properties of quantum systems, such as superposition and entanglement, to potentially provide faster convergence or better solutions for certain optimization problems.
How does quantum gradient descent differ from classical gradient descent?
Quantum gradient descent differs from classical gradient descent by leveraging quantum computing principles. In classical gradient descent, computations are performed using classical bits, while in quantum gradient descent, quantum bits or qubits are utilized to exploit quantum effects and potentially offer advantages in terms of optimization speed or solution quality.
Are there any quantum gradient descent algorithms already implemented?
Yes, several quantum gradient descent algorithms have been proposed and implemented on quantum computers or simulators. Examples include quantum approximate optimization algorithm (QAOA) and variational quantum eigensolver (VQE). These algorithms offer quantum-inspired optimization approaches within the constraints of current quantum technology.
Can anyone use quantum gradient descent algorithms?
Quantum gradient descent algorithms require access to quantum computing resources, which are currently limited and not easily accessible to everyone. However, as quantum technology advances, it is expected that wider access and user-friendly interfaces for utilizing quantum gradient descent algorithms will become available.
What are the potential applications of quantum gradient descent?
Quantum gradient descent can be applied to various fields where optimization plays a crucial role, such as machine learning, molecular simulation, financial modeling, and material design. It has the potential to improve the efficiency of optimization algorithms in these domains, leading to advancements and breakthroughs in respective applications.
Is quantum gradient descent the future of optimization?
While quantum gradient descent shows promising potential, it is still an active area of research and development. It is difficult to predict the future of optimization solely based on quantum gradient descent. However, with advancements in quantum technology and increased accessibility, it could contribute significantly to the optimization landscape in the future.