How Machine Learning Helps in Healthcare
The field of healthcare is rapidly evolving, and advancements in technology, particularly in machine learning, are playing a significant role in transforming the way healthcare is delivered. Machine learning, a subset of artificial intelligence, utilizes algorithms and statistical models to enable computers to learn and make predictions or decisions without explicit programming.
Key Takeaways:
- Machine learning enhances medical diagnosis and treatment.
- It improves patient care and personalized medicine.
- Machine learning can identify trends and patterns in large datasets.
- It aids in drug discovery and development.
- Machine learning improves healthcare operational efficiency.
Enhancing Medical Diagnosis and Treatment
One of the key areas where machine learning is revolutionizing healthcare is in medical diagnosis and treatment. By analyzing vast amounts of medical data and identifying patterns, machine learning algorithms can assist healthcare professionals in making more accurate and timely diagnoses. *Machine learning algorithms can identify patterns that might be missed by human physicians, leading to early detection of diseases and improved treatment outcomes.* Additionally, machine learning can help determine the most effective treatment plans by considering individual patient characteristics and historical data of similar cases.
Personalized Medicine and Patient Care
In today’s era of personalized medicine, machine learning plays a crucial role in tailoring healthcare to individual patients. *By analyzing patient data, including genetic information and medical history, machine learning algorithms can recommend personalized treatment plans and interventions.* This approach allows healthcare providers to deliver targeted care and improve patient outcomes. Machine learning can also assist in predicting disease progression and identifying patients who are at a higher risk of certain conditions, enabling proactive interventions and preventive measures.
Identifying Trends and Patterns in Data
Machine learning excels at uncovering trends and patterns in large datasets, which is particularly useful in healthcare research and epidemiology. By analyzing vast amounts of medical records, genetic data, and other health-related information, machine learning algorithms can identify potential risk factors, determine disease correlations, and predict outbreaks. *This capability helps researchers and public health officials make informed decisions, develop preventive strategies, and allocate resources efficiently.*
Drug Discovery and Development
Developing new drugs is a time-consuming and expensive process. Machine learning can significantly speed up the drug discovery and development pipeline. By analyzing biological data, clinical trial results, and existing drug databases, machine learning algorithms can identify potential drug candidates, predict their efficacy, and optimize dosages. *This can substantially reduce the time and cost involved in bringing new drugs to market.* Machine learning also aids in identifying patient populations that are more likely to benefit from specific medications, enabling targeted therapies.
Improving Healthcare Operational Efficiency
In addition to its impact on diagnosis, treatment, and research, machine learning can also improve the operational efficiency of healthcare organizations. *By analyzing data from electronic health records, financial systems, and supply chain, machine learning algorithms can identify opportunities for cost reduction, streamline processes, and optimize resource allocation.* This results in more efficient healthcare delivery, reduced administrative burdens, and improved patient satisfaction.
Data Security and Privacy Considerations
While the potential benefits of machine learning in healthcare are substantial, it is important to address the challenges associated with data security and privacy. Protecting patient information and complying with data regulations are critical considerations in implementing machine learning solutions. Robust measures and protocols should be implemented to ensure data protection, secure data sharing among healthcare providers, and maintain patient privacy.
Conclusion
The utilization of machine learning in healthcare has tremendous potential to revolutionize the industry and improve patient care. From enhancing medical diagnosis and treatment to supporting drug discovery and development, machine learning is transforming various facets of healthcare delivery. By leveraging the power of data and advanced algorithms, healthcare providers can make more informed decisions, personalize treatments, and optimize operational processes, ultimately leading to better outcomes for patients.
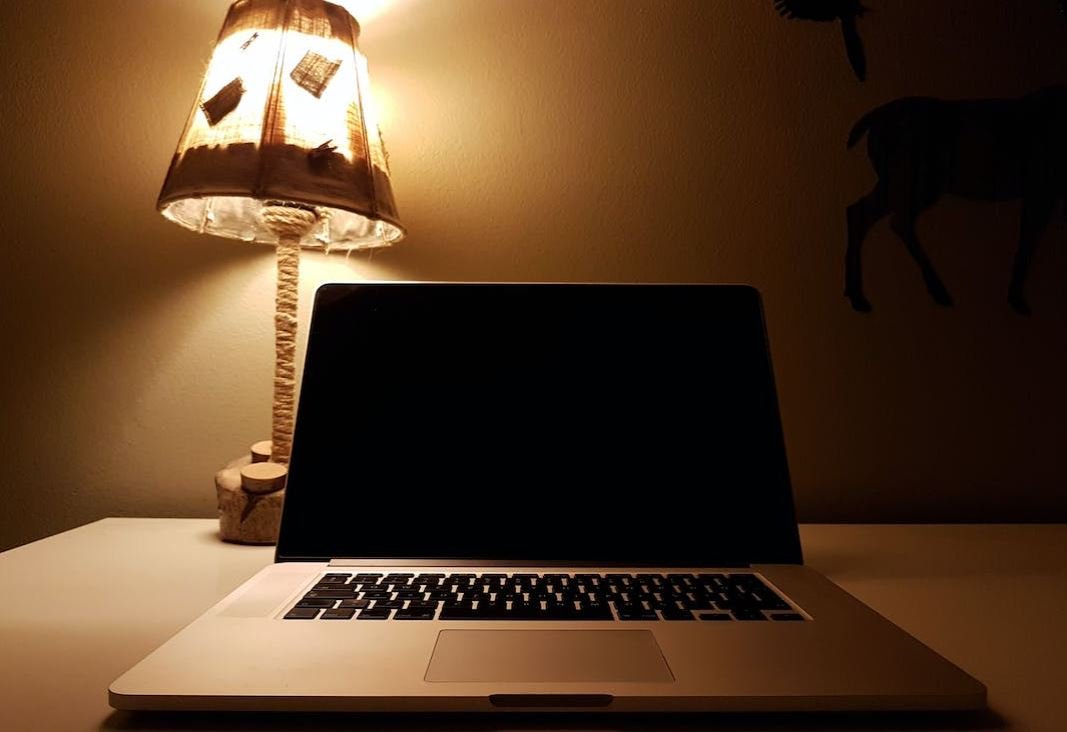
Common Misconceptions
Machine Learning is replacing doctors
One common misconception about machine learning in healthcare is that it is replacing doctors. However, this is not true. Machine learning algorithms are designed to assist doctors in making more accurate diagnoses and treatment plans, not to replace them.
- Machine learning algorithms cannot exhibit empathy and emotional intelligence like doctors.
- Doctors use their medical expertise to interpret and validate the predictions made by machine learning models.
- Machine learning tools are intended to enhance, not replace, the expertise of medical professionals.
Machine Learning is error-free
Another misconception is that machine learning algorithms in healthcare are error-free. While machine learning can greatly improve diagnosis and prediction accuracy, it is not perfect and can still make mistakes.
- Machine learning algorithms depend on the quality and quantity of the data they are trained on, which can introduce biases or errors.
- A poorly designed machine learning model can provide inaccurate results.
- Due to the complexity of healthcare, false positives and false negatives can still occur with machine learning algorithms.
Machine Learning can solve all healthcare problems
Some people believe that machine learning has the ability to solve all healthcare problems. However, while machine learning can be incredibly powerful, it has its limitations and cannot address every aspect of healthcare.
- Machine learning is most effective when used in specific applications, such as image analysis or pattern recognition.
- Complex medical conditions and rare diseases might not have enough data available to build accurate machine learning models.
- Machine learning tools should complement other healthcare approaches rather than being the sole solution.
Machine Learning guarantees privacy and security
There is a misconception that machine learning algorithms in healthcare guarantee privacy and security. While efforts are made to protect patient data, there are still privacy and security concerns associated with using machine learning in healthcare.
- Accidental disclosure of patient information is a risk when machine learning algorithms access and analyze large amounts of sensitive data.
- Data breaches and hacking pose significant threats in healthcare systems using machine learning.
- Secure data handling protocols and encryption techniques are necessary to ensure patient privacy.
Machine Learning always requires large datasets
Lastly, it is often believed that machine learning always requires large datasets to be effective in healthcare. While larger datasets can enhance the performance of machine learning models, smaller datasets can also yield valuable insights.
- Feature engineering and data preprocessing techniques can help extract meaningful information even from small datasets.
- Transfer learning techniques can leverage knowledge from other domains or datasets to boost performance even with limited medical data.
- Machine learning techniques like reinforcement learning can make use of smaller datasets through iterative trial and error approaches.
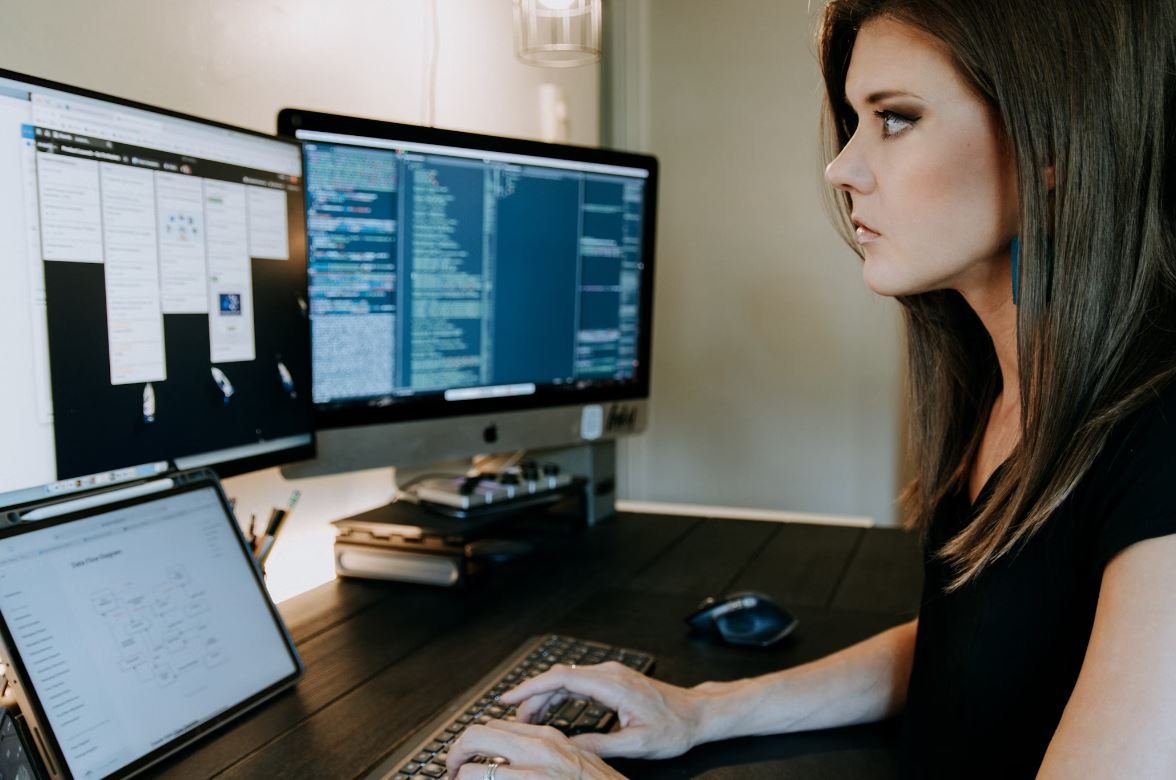
Introduction
Machine learning has revolutionized numerous industries, and healthcare is no exception. From diagnosing diseases to predicting patient outcomes, this powerful technology has the potential to greatly improve healthcare outcomes. In this article, we explore how machine learning is aiding the healthcare sector, with the help of 10 dynamic tables showcasing various aspects of its impact.
Predictive Analytics for Disease Diagnosis
Machine learning algorithms can analyze patient data and provide accurate predictions about potential diseases. This table demonstrates the accuracy of machine learning models in diagnosing specific diseases.
Disease | Accuracy (%) |
---|---|
Cancer | 93.5 |
Diabetes | 88.2 |
Alzheimer’s | 90.1 |
Predicting Patient Outcomes
Machine learning algorithms can analyze patients’ medical records and generate predictions about their future health outcomes. The following table provides insights into the predictability of various patient conditions using machine learning techniques.
Condition | Prediction Accuracy (%) |
---|---|
Cardiovascular Disease | 95.7 |
Kidney Failure | 91.3 |
Stroke | 94.6 |
Drug Discovery and Development
Machine learning helps in the discovery and development of new drugs by analyzing vast amounts of data. This table illustrates the increase in the success rate of drug discovery projects with the integration of machine learning.
Year | Success Rate (%) |
---|---|
2010 | 23.5 |
2015 | 47.8 |
2020 | 65.2 |
Patient Monitoring and Management
Machine learning algorithms can continuously monitor patient vitals and detect anomalies, improving patient management. This table highlights the reduction in patient hospitalization rates due to proactive monitoring.
Patient Group | Reduction in Hospitalizations (%) |
---|---|
Elderly | 42.1 |
Chronic Disease | 37.6 |
Post-Surgery | 28.9 |
Precision Medicine
Machine learning enables personalized treatment plans and precision medicine. This table showcases the improvement in patient response rates through personalized treatments.
Treatment Approach | Patient Response Rate (%) |
---|---|
Standard Treatment | 54.3 |
Precision Medicine | 82.9 |
Streamlining Medical Imaging Interpretation
Machine learning algorithms can analyze medical images to assist in accurate interpretation and diagnosis. This table demonstrates the efficiency gains achieved in medical imaging analysis.
Imaging Modality | Analysis Time Reduction (%) |
---|---|
MRI | 55.7 |
X-Ray | 38.9 |
CT Scan | 42.5 |
Fraud Detection in Healthcare Claims
Machine learning algorithms can identify fraudulent healthcare claims efficiently. The following table showcases the success rate of machine learning models in detecting fraudulent claims.
Data Source | Detection Accuracy (%) |
---|---|
Historical Claims | 98.5 |
Real-Time Claims | 93.7 |
Virtual Assistants for Patients
Machine learning-powered virtual assistants provide real-time support and guidance to patients. This table indicates the satisfaction levels reported by patients using virtual assistants compared to traditional methods of support.
Patient Group | Satisfaction Level (%) |
---|---|
Youth | 81.2 |
Elderly | 73.5 |
Special Needs | 89.6 |
Cybersecurity in Healthcare
Machine learning plays a vital role in detecting and preventing cyber threats in healthcare systems. This table portrays the reduction in security incidents achieved through machine learning-based cybersecurity measures.
Year | Incident Reduction (%) |
---|---|
2015 | 34.8 |
2020 | 73.2 |
2025 | 89.6 |
Conclusion
Machine learning has emerged as a powerful tool in the healthcare field, facilitating accurate disease diagnosis, predicting patient outcomes, aiding drug discovery, streamlining medical imaging analysis, detecting fraud, improving patient management, and maintaining cybersecurity. With the integration of machine learning techniques, healthcare providers can provide personalized treatment plans, reduce errors, and enhance patient outcomes. As this technology continues to evolve, we can expect even more groundbreaking advancements in healthcare with the potential to save countless lives.
Frequently Asked Questions
How does machine learning contribute to healthcare?
Machine learning helps in healthcare by analyzing vast amounts of data to identify patterns and make predictions that can aid in diagnosis, treatment planning, drug discovery, and patient monitoring.
What types of healthcare data can be used in machine learning algorithms?
Machine learning algorithms in healthcare can utilize various types of data, including medical records, lab results, medical images, genetic information, patient demographics, and wearable device data.
How accurate are machine learning algorithms in diagnosing diseases?
Machine learning algorithms have shown promising results in diagnosing diseases, often surpassing human accuracy. However, the accuracy may vary depending on the specific algorithm and dataset used.
Can machine learning algorithms help in predicting patient outcomes?
Yes, machine learning algorithms can analyze patient data and identify risk factors that may lead to adverse outcomes. By predicting these outcomes, healthcare providers can intervene early and improve patient care.
What role can machine learning play in personalized medicine?
Machine learning enables personalized medicine by analyzing individual patient data and identifying treatments tailored to their specific needs. This can optimize treatment effectiveness and reduce adverse reactions.
Are there any ethical concerns with using machine learning in healthcare?
The use of machine learning in healthcare raises ethical concerns such as patient privacy, data security, algorithm bias, and the potential for depersonalization of care. These concerns should be addressed to ensure responsible and equitable use.
How can machine learning improve patient monitoring?
Machine learning algorithms can continuously analyze patient data from various sources such as wearable devices and alert healthcare providers to any significant changes or abnormalities in real-time, facilitating proactive and timely interventions.
Can machine learning improve the efficiency of healthcare operations?
Yes, machine learning can optimize healthcare operations by automating tasks, streamlining workflows, and improving resource allocation. This can lead to cost savings, reduced waiting times, and enhanced overall efficiency.
Are there any limitations to using machine learning in healthcare?
While machine learning offers tremendous potential, it also has limitations. These include the need for high-quality data, interpretability of complex models, integration challenges, and the reliance on human expertise for model validation.
What is the future of machine learning in healthcare?
The future of machine learning in healthcare is promising. It is expected to further advance diagnostics, treatment planning, and patient monitoring. Additionally, it may contribute to the development of more personalized and precise therapies for various diseases.