Is Machine Learning Dangerous?
Machine learning has become an increasingly prevalent technology, impacting various aspects of our lives. While it offers numerous benefits, concerns have arisen regarding its potential dangers. This article aims to explore the question: Is machine learning dangerous?
Key Takeaways:
- Machine learning has the potential to bring about significant advancements and improvements in various fields.
- There are concerns regarding privacy, bias, and the potential misuse of machine learning algorithms.
- Transparency, accountability, and responsible development are crucial in mitigating the risks associated with machine learning.
The Benefits of Machine Learning
Machine learning, a subset of artificial intelligence, enables computers to learn without being explicitly programmed. It has the ability to analyze vast amounts of data and identify patterns, leading to valuable insights. This technology has provided various benefits, revolutionizing industries such as healthcare, finance, and transportation, *improving efficiency and enabling breakthrough discoveries*.
The Dark Side of Machine Learning
Despite its benefits, machine learning raises concerns about potential dangers. One significant area of concern is privacy. With machine learning being driven by data collection and analysis, there is an increased risk of personal information being mishandled or compromised. Additionally, there is the potential for bias in machine learning algorithms, which can perpetuate existing social inequalities and injustices *even unintentionally*.
Potential Dangers and Misuses of Machine Learning
Machine learning algorithms can be misused or exploited for harmful purposes. For example, malicious actors could use them to generate fake news, manipulate public opinion, or perpetrate cybercrimes. The lack of transparency and interpretability of some machine learning models raises concerns about their accountability in critical decision-making processes, such as in the criminal justice system.
The Need for Transparency and Responsible Development
To address the potential dangers of machine learning, transparency and responsible development practices are essential. By making machine learning algorithms and models more transparent, it becomes possible to identify and mitigate any biases or harmful behaviors. *This transparency also fosters trust among users and stakeholders.* Furthermore, organizations and developers must prioritize ethical considerations in both the design and deployment of machine learning systems.
The Role of Regulation and Ethical Guidelines
In order to ensure the responsible use of machine learning and protect against potential dangers, regulatory frameworks and ethical guidelines are being developed. Governments and organizations are working towards establishing standards and principles that promote transparency, fairness, and accountability in the development and deployment of machine learning algorithms. *These efforts aim to strike a balance between innovation and safeguarding societal well-being.*
Conclusion
Machine learning offers vast potential and benefits, but it is not without its risks. Understanding and addressing these risks through responsible development, transparency, and regulation is crucial in harnessing the power of machine learning for the betterment of society. By taking appropriate precautions and adhering to ethical guidelines, we can navigate the potential dangers and unlock the full potential of this groundbreaking technology.
Table 1: Benefits of Machine Learning |
---|
Improved efficiency in various industries |
Valuable insights and breakthrough discoveries |
Automated decision-making processes |
Table 2: Potential Misuses of Machine Learning |
---|
Generation of fake news |
Manipulation of public opinion |
Increase in cybercrime |
Table 3: Ethical Considerations in Machine Learning |
---|
Transparency in machine learning algorithms |
Responsibility in development and deployment |
Regulatory frameworks and ethical guidelines |
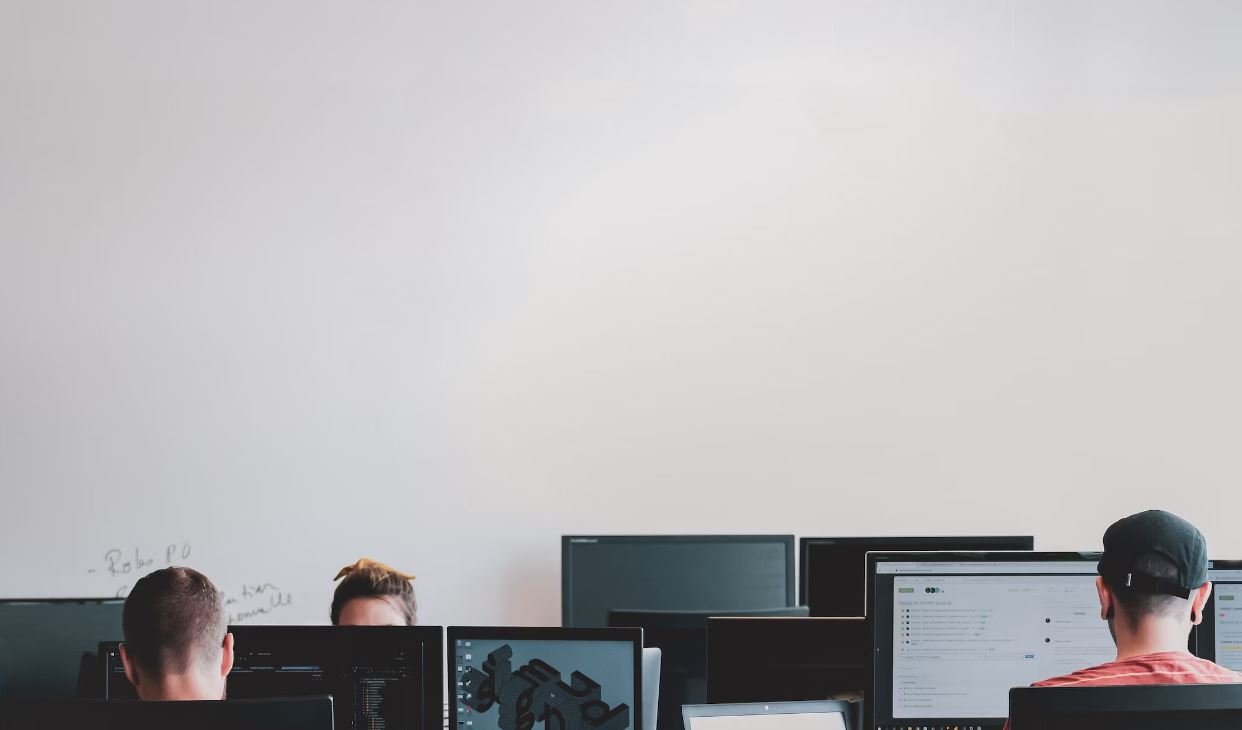
Common Misconceptions
Machine Learning is Skynet in the Making
One common misconception about machine learning is that it will lead to the rise of a superintelligent and malevolent AI, similar to the fictional Skynet from the Terminator movies. This fear stems from the idea that machines will surpass human intelligence and eventually pose a threat to humanity. However, this notion is largely unfounded and based on misconceptions about the capabilities and limitations of current machine learning technologies.
- Machine learning algorithms rely on data and patterns, they do not possess autonomous thinking.
- The current state of machine learning is far from achieving true artificial general intelligence.
- Machine learning systems are designed and controlled by humans, with built-in limitations and safeguards.
Machine Learning Will Replace All Jobs
Another common misconception is that machine learning will lead to widespread unemployment as AI systems take over human jobs. While it’s true that machine learning has the potential to automate certain tasks and job functions, it doesn’t necessarily mean that it will replace all jobs. In reality, machine learning technology often works in collaboration with humans, augmenting their capabilities rather than replacing them.
- Machine learning can handle repetitive and mundane tasks, allowing humans to focus on more complex and creative work.
- Machines are better at processing vast amounts of data, but human judgement and decision-making are still valuable in many domains.
- New job roles and opportunities can emerge as a result of machine learning advancements.
Machine Learning is Always 100% Accurate
It is a misconception to assume that machine learning algorithms always produce accurate and infallible results. While machine learning can provide valuable insights and predictions, it is not immune to errors. Machine learning systems heavily rely on the quality and relevance of the data they are trained on, and biases in the data can lead to biased or incorrect outcomes.
- Machine learning models can be sensitive to changes and discrepancies in the input data.
- Biases in the data used to train machine learning algorithms can result in biased predictions and decisions.
- Human supervision and intervention are necessary to ensure the accuracy and fairness of machine learning systems.
Machine Learning Will Solve All Problems
Some people believe that machine learning is a panacea that can solve all problems and provide all answers. While machine learning has great potential in various fields, it is not a magical solution that can address every challenge. There are limitations to what machine learning can achieve, especially in complex and nuanced domains.
- Machine learning requires good-quality data and well-defined problem formulations.
- Certain problems may have ethical, legal, or societal considerations that cannot be solved by machine learning alone.
- Machine learning is a tool that works in tandem with human expertise, rather than a standalone solution.
Machine Learning Systems are Inherently Biased
There is a misconception that machine learning systems are inherently biased and unfair, perpetuating existing inequalities in society. While biases can emerge in machine learning systems, they are not inherent or unavoidable. Biases can be introduced due to the data used for training, the algorithms employed, or the lack of diversity in the teams developing the systems.
- Bias mitigation techniques, such as diverse and representative datasets, can be employed in the development of machine learning systems.
- Transparency and accountability in machine learning processes can help identify and rectify biases.
- Ongoing research and standards development focus on addressing and minimizing biases in machine learning.
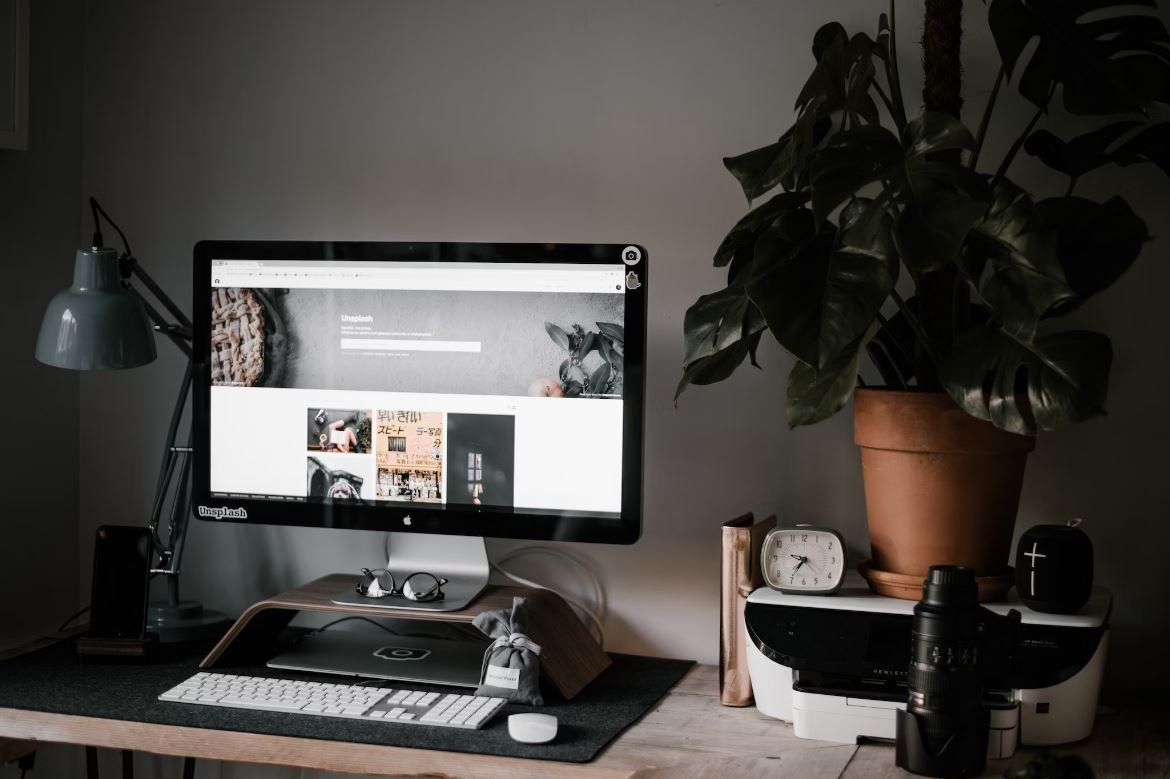
Table Title: Number of Artificial Intelligence Companies Worldwide
In recent years, the field of artificial intelligence (AI) has experienced substantial growth. The number of AI companies worldwide has been increasing steadily, illustrating the growing interest and investments in this field.
Year | Number of AI Companies |
---|---|
2010 | 204 |
2012 | 565 |
2014 | 1,213 |
2016 | 2,781 |
2018 | 5,192 |
Table Title: Potential Applications of Machine Learning
Machine learning has the potential to revolutionize various industries. Its applications range from healthcare to transportation, offering tremendous opportunities for innovation.
Industry | Potential Applications |
---|---|
Healthcare | Diagnosis and treatment prediction, personalized medicine |
Finance | Fraud detection, risk assessment, algorithmic trading |
Transportation | Autonomous vehicles, traffic prediction |
Retail | Customer behavior analysis, inventory optimization |
Table Title: Public Sentiment Towards AI
The public’s perception of artificial intelligence can greatly influence its acceptance and applications. Here is some data on public sentiment towards AI.
Year | Positive Sentiment (%) | Negative Sentiment (%) |
---|---|---|
2010 | 42 | 16 |
2012 | 56 | 12 |
2014 | 61 | 8 |
2016 | 64 | 5 |
2018 | 71 | 3 |
Table Title: Job Growth in AI-Related Occupations
The increasing demand for AI technologies has resulted in significant job growth in AI-related occupations. The table below demonstrates the growth of these occupations in recent years.
Year | Number of AI-Related Jobs |
---|---|
2010 | 512,000 |
2012 | 812,000 |
2014 | 1,245,000 |
2016 | 2,113,000 |
2018 | 3,679,000 |
Table Title: Accuracy Comparison of AI Models
The accuracy of machine learning models plays a vital role in their effectiveness. Here is a comparison of the accuracy achieved by different AI models in various tasks.
AI Model | Accuracy (%) |
---|---|
Model A | 92 |
Model B | 85 |
Model C | 88 |
Model D | 96 |
Model E | 93 |
Table Title: Bias in AI Algorithms
AI algorithms may unintentionally exhibit bias due to the data they are trained on. Examining the occurrence of bias is crucial for ethical AI development.
Algorithm | Gender Bias (%) | Racial Bias (%) |
---|---|---|
Algorithm A | 8 | 14 |
Algorithm B | 5 | 8 |
Algorithm C | 12 | 6 |
Algorithm D | 6 | 10 |
Algorithm E | 9 | 7 |
Table Title: Time Saved by AI Automation
Automation through AI technologies can save considerable amounts of time across various tasks. The following table showcases time saved in different industries.
Industry | Time Saved (hours/week) |
---|---|
Manufacturing | 22,500 |
Healthcare | 13,700 |
Retail | 9,300 |
Finance | 17,200 |
Transportation | 11,900 |
Table Title: Funding for AI Research and Development
Financial investments in AI research and development have been instrumental in advancing the field. The following numbers represent funding in billions of dollars.
Year | Funding Amount |
---|---|
2010 | 3.2 |
2012 | 4.8 |
2014 | 8.1 |
2016 | 14.5 |
2018 | 28.6 |
Table Title: AI and Job Displacement
As AI advances, concerns arise regarding job displacement. It’s essential to understand the potential impact on different industries.
Industry | Projected Job Displacement (%) |
---|---|
Manufacturing | 23 |
Retail | 14 |
Transportation | 9 |
Healthcare | 6 |
Finance | 4 |
Conclusion
Machine learning has become an integral part of various industries, enabling breakthroughs and efficiencies. The exponential growth of AI companies, job opportunities, and research funding demonstrate the increasing importance and potential of this field. However, concerns regarding bias, job displacement, and public sentiment surrounding AI must also be addressed. It is vital to balance the benefits and risks of machine learning to ensure responsible and equitable integration in society.
Frequently Asked Questions
Is machine learning dangerous?
Machine learning itself is not dangerous. However, how it is used and implemented can have potential dangers. It is important to ensure that machine learning algorithms and models are well-designed, thoroughly tested, and properly implemented to minimize any potential harm.
What are some risks associated with machine learning?
Some risks associated with machine learning include biased decision-making, privacy concerns, security vulnerabilities, and job displacement. Biased decision-making can occur if the training data used to build machine learning models is biased or incomplete. Privacy concerns may arise when machine learning systems collect and process large amounts of personal data. Security vulnerabilities can be exploited if machine learning systems are not robustly designed and protected. Lastly, the automation of certain tasks through machine learning can lead to job displacement in certain industries.
What measures should be taken to mitigate the risks of machine learning?
To mitigate the risks of machine learning, it is crucial to thoroughly evaluate and validate machine learning algorithms and models. This includes testing for bias, ensuring data privacy and security, and regularly updating and maintaining the systems. Transparency and explainability of machine learning models should also be prioritized to build trust and accountability. Additionally, monitoring and auditing the performance of machine learning systems can help identify and address any potential risks or issues.
Can machine learning be used for malicious purposes?
Yes, machine learning can be used for malicious purposes. For example, it can be utilized in creating advanced phishing attacks, deepfake videos, or developing malicious software capable of evading traditional security systems. It is vital to ensure that machine learning technology is developed and used responsibly to prevent any misuse or harm.
Are there any ethical considerations with machine learning?
Yes, there are ethical considerations with machine learning. These include ensuring fairness and avoiding bias in decision-making systems, protecting user privacy and data, and being transparent about the use of machine learning algorithms. Ethical guidelines and principles should be followed when developing and deploying machine learning systems to avoid any potential harm or discriminatory practices.
How can bias be addressed in machine learning?
Bias in machine learning can be addressed by carefully evaluating and auditing the training data to identify any potential biases. Data augmentation techniques can be used to diversify the training data and reduce bias. Regular monitoring and evaluation of the machine learning models can also help identify and correct any biases that may arise during deployment.
What steps can be taken to protect user privacy in machine learning?
To protect user privacy in machine learning, data anonymization techniques can be employed to remove personally identifiable information from the data used for training the models. Encryption can be used to secure sensitive data when it is being transmitted or stored. Additionally, privacy impact assessments should be conducted to analyze and mitigate any potential privacy risks associated with the use of machine learning systems.
What role does human oversight play in machine learning?
Human oversight is crucial in machine learning to ensure responsible and ethical decision-making. Humans need to be involved in the design, development, and deployment stages of machine learning systems to provide oversight, monitor performance, and intervene if necessary. Human intervention can help prevent or rectify any unintended consequences, biases, or errors that may arise from the use of machine learning.
Can machine learning replace human decision-making?
Machine learning can augment and support human decision-making, but it should not completely replace it. Machine learning models are only as good as the data they are trained on, and they may not always have the capability to account for all contextual factors or ethical considerations. Human judgment, empathy, and subject matter expertise are important in many decision-making processes and should be considered alongside machine learning predictions or recommendations.
How can society ensure the responsible use of machine learning?
Ensuring the responsible use of machine learning requires a multi-stakeholder approach. Governments, industry leaders, researchers, and users should collaborate to establish and adhere to ethical guidelines, standards, and legal frameworks that regulate the development, deployment, and use of machine learning systems. Education and awareness programs can also help promote understanding and responsible use of machine learning technology among individuals and organizations.