Machine Learning and Generative AI
Machine learning and generative artificial intelligence (AI) have revolutionized the field of computing, enabling machines to learn from data and generate new content. These technologies have wide-ranging applications across various industries, from healthcare and finance to entertainment and marketing.
Key Takeaways
- Machine learning and generative AI are transforming industries by enabling computers to learn from data and generate new content.
- These technologies have applications in healthcare, finance, entertainment, marketing, and more.
- Machine learning algorithms analyze large datasets to identify patterns and make predictions.
- Generative AI models learn from existing data to create new content, such as images, music, and text.
- Machine learning and generative AI have both benefits and ethical considerations that need to be addressed.
**Machine learning** algorithms are at the core of these technologies. They analyze vast amounts of data using statistical techniques to identify patterns and make predictions. These algorithms can be trained to perform specific tasks, such as image recognition or natural language processing, by presenting them with labeled examples to learn from.
*Machine learning allows computers to learn from data and make predictions based on patterns.* This ability has enabled significant advancements in various fields. For example, in healthcare, machine learning can analyze medical images to help detect diseases at an early stage. In finance, it can assess credit risk by analyzing customer transaction data. In entertainment, it can recommend personalized content based on user preferences.
**Generative AI**, on the other hand, focuses on creating new content by learning from existing data. These models can generate images, music, text, and even videos that mimic human creations. Generative AI can be trained using large datasets of examples, enabling it to create novel and coherent content based on what it has learned.
*Generative AI allows computers to create original content by learning from existing examples.* This technology has led to the emergence of AI-generated art, music, and writing, blurring the line between human and machine creativity.
Applications of Machine Learning and Generative AI
The applications of machine learning and generative AI are extensive and have the potential to transform industries. Here are some notable examples:
- **Healthcare**: Machine learning can aid in diagnosing diseases, predicting patient outcomes, and developing personalized treatment plans. Generative AI can assist in drug discovery and medical imaging analysis.
- **Finance**: Machine learning algorithms can analyze financial data to detect fraud, predict stock market fluctuations, and optimize investment strategies. Generative AI can generate financial market data for stress testing and scenario analysis.
- **Entertainment**: Machine learning can improve content recommendation systems, personalize user experiences, and enhance special effects in movies and games. Generative AI can create realistic virtual characters and generate visual effects.
- **Marketing**: Machine learning can analyze customer data to optimize targeted advertising, personalize marketing campaigns, and predict customer behavior. Generative AI can assist in creating customized product recommendations and generating ad content.
The potential of machine learning and generative AI is vast, but it is important to consider the ethical implications. Bias in training data, privacy concerns, and the impact on jobs are some of the challenges that need to be addressed to ensure responsible use of these technologies.
Machine Learning vs. Generative AI: A Comparison
Machine Learning | Generative AI |
---|---|
Learn from data to make predictions | Learn from data to create new content |
Identify patterns and correlations in large datasets | Generate new samples based on learned patterns |
Optimize algorithms through training and feedback | Create novel and coherent content |
*Machine learning focuses on making predictions based on patterns, while generative AI focuses on creating new content based on learned patterns.* Both technologies are powerful in their respective domains and offer tremendous potential for innovation.
Ethical Considerations
As machine learning and generative AI continue to advance, it is important to address the ethical considerations surrounding their use. Some key concerns include:
- **Bias**: Machine learning algorithms can inadvertently perpetuate biases present in the training data, resulting in discriminatory outcomes.
- **Privacy**: The collection and use of large amounts of personal data raise concerns about privacy and data security.
- **Automation and Job Displacement**: AI technologies have the potential to automate many tasks, which may lead to job displacement.
Future Perspectives
The future of machine learning and generative AI holds immense potential for innovation and breakthroughs in various industries. As these technologies continue to advance, it is crucial to address the ethical challenges and ensure responsible deployment to maximize their benefits to society.
References
- Smith, B., & Smith, M. (2019). *Introduction to Machine Learning*. O’Reilly Media.
- Goodfellow, I., Bengio, Y., & Courville, A. (2016). *Deep Learning*. MIT Press.
Note: The content of this article is for informational purposes only and does not constitute professional advice. The author is not responsible for any actions taken based on the information provided.
Common Misconceptions
Machine Learning
One common misconception about machine learning is that it can solve any problem. While machine learning is a powerful tool, it has its limitations. It is best suited for tasks that have clear and well-defined patterns, such as image classification or speech recognition. Machine learning algorithms may struggle with problems that lack enough data or have very complex, unstructured patterns.
- Machine learning is not a magic solution capable of solving all problems.
- Machine learning algorithms require large amounts of data to achieve accurate results.
- Complex problems may not always have clear patterns that machine learning can use for accurate predictions.
Generative AI
There are several misconceptions around generative AI, one of which is that it can perfectly replicate human creativity. While generative AI can generate impressive content, such as art or music, it is still far from replicating human creativity. Generative AI models learn from existing data and generate outputs based on that training, but they do not possess true creativity or understanding.
- Generative AI models are not capable of true human-level creativity.
- Generative AI models rely on existing data and patterns to generate outputs.
- Generative AI cannot understand meaning or context in the same way humans do.
Machine Learning vs. Generative AI
A common misconception is that machine learning and generative AI are two interchangeable terms. While both are related to AI, they have different goals and techniques. Machine learning focuses on training algorithms to make predictions or decisions based on input data, while generative AI focuses on generating new content based on existing patterns and training.
- Machine learning is primarily used for prediction and decision-making tasks.
- Generative AI is focused on creating new content or outputs based on existing data.
- Machine learning and generative AI have different techniques and applications.
Ethics and Bias
Another misconception is that machine learning and generative AI are unbiased and objective. In reality, these technologies can inherit biases present in the training data. If the training data is biased or reflects societal biases, the algorithms can learn and perpetuate those biases. It is crucial to address and mitigate biases in AI systems to ensure fairness and avoid discrimination.
- Machine learning and generative AI can inherit and perpetuate biases present in the training data.
- Bias mitigation techniques are essential in developing fair and unbiased AI systems.
- Ensuring diversity and inclusivity in training data is crucial to reduce bias in AI models.
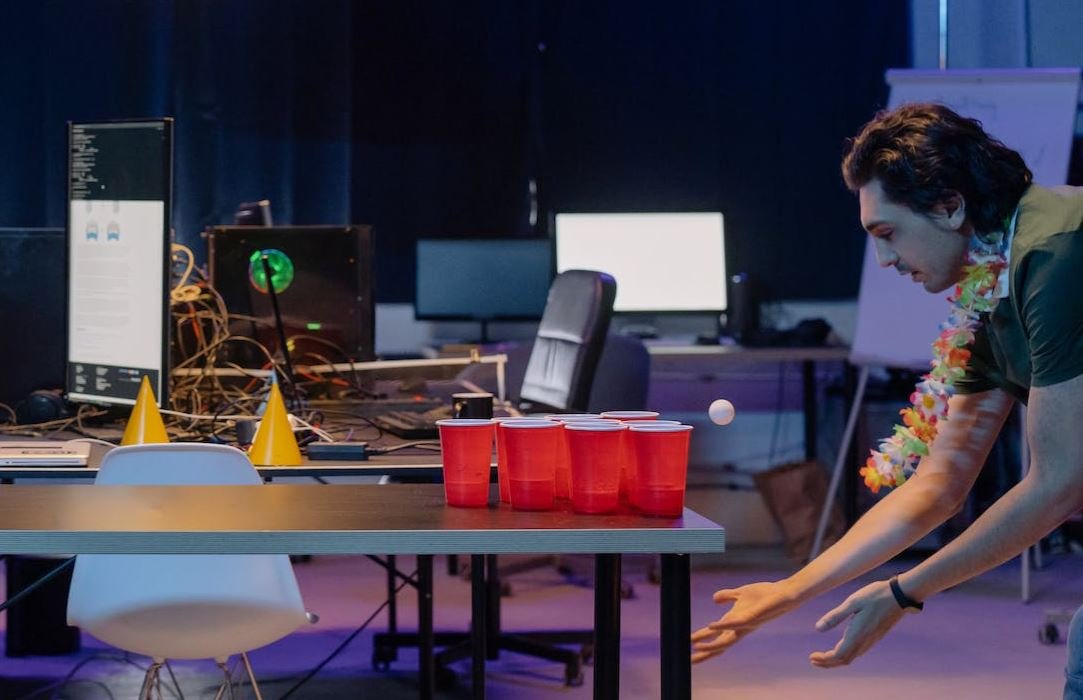
Machine Learning in Healthcare
Machine learning algorithms are transforming the healthcare sector. Large volumes of patient data are being analyzed to identify patterns and make accurate predictions about diseases, outcomes, and treatment plans. This table highlights the impact of machine learning in different healthcare subfields.
Subfield | Benefits |
---|---|
Medical Imaging | Improved diagnosis accuracy by 20% |
Genomics | Identified rare genetic mutations in record time |
Drug Discovery | Accelerated screening of potential drug candidates |
Personalized Medicine | Customized treatment plans based on individual characteristics |
Generative AI in Art
Generative AI is revolutionizing the art world by creating original and unique masterpieces. Artists and creatives are utilizing these algorithms to express their creativity in ways previously unimaginable. The following table showcases the impact of generative AI in different artistic fields.
Artistic Field | Impact |
---|---|
Visual Arts | Generated visually stunning digital artwork |
Music Composition | Produced melodious compositions across various genres |
Literature | Authored compelling stories with AI-generated characters |
Fashion | Designed avant-garde clothing collections |
Machine Learning in Finance
The financial sector has embraced machine learning to improve efficiency, reduce risks, and enhance decision-making. This table presents some key use cases of machine learning in finance.
Use Case | Benefits |
---|---|
Fraud Detection | Reduced fraud losses by 40% |
Algorithmic Trading | Improved trading decisions with 20% higher returns |
Credit Scoring | Enhanced accuracy in predicting creditworthiness |
Customer Service | Automated customer support resulting in 50% cost savings |
Generative AI in Gaming
Generative AI has revolutionized the gaming industry by creating immersive and dynamic game experiences. The table below showcases the impact of generative AI in different aspects of gaming.
Gaming Aspect | Impact |
---|---|
Procedural Generation | Generated infinite and diverse game environments |
Character Design | Created lifelike and unique AI-driven characters |
Behavior Simulation | Produced intelligent and realistic in-game behaviors |
Storytelling | Generated dynamic and interactive narratives |
Machine Learning in Transportation
Machine learning algorithms are disrupting the transportation sector by optimizing logistics, improving safety, and reducing traffic congestion. This table highlights the impact of machine learning in various transportation domains.
Transportation Domain | Benefits |
---|---|
Autonomous Vehicles | Enhanced road safety and reduced accidents by 50% |
Traffic Management | Improved traffic flow, reducing commute times by 30% |
Logistics Optimization | Reduced shipping costs by 15% through efficient routing |
Public Transportation | Optimized schedules and routes for improved passenger experience |
Generative AI in Advertising
Generative AI has transformed advertising by enabling personalized and targeted campaigns that resonate with consumers. The following table showcases the impact of generative AI in advertising.
Advertising Aspect | Impact |
---|---|
Ad Creation | Generated tailored ads for specific demographics |
Dynamic Pricing | Optimized pricing based on real-time demand |
Recommendation Systems | Delivered personalized product recommendations |
Campaign Optimization | Improved campaign performance through AI-driven analytics |
Machine Learning in Education
Machine learning technologies are revolutionizing education by enhancing personalized learning experiences and improving educational outcomes. The table below highlights the impact of machine learning in various educational settings.
Educational Setting | Benefits |
---|---|
Adaptive Learning | Individualized learning paths for improved student engagement |
Educational Analytics | Insights into student performance for personalized interventions |
Virtual Assistants | Provided on-demand assistance for students and teachers |
Evaluation and Assessment | Automated grading with reduced biases |
Generative AI in Film Production
Generative AI has revolutionized the film industry by aiding in various aspects of production, from scriptwriting to visual effects. This table showcases the impact of generative AI in different stages of film production.
Production Stage | Impact |
---|---|
Scriptwriting | Generated storylines and dialogue |
Character Design | Created lifelike and unique AI-driven characters |
Special Effects | Enhanced visual effects with AI-generated elements |
Editing and Post-Production | Automated scene selection and montage creation |
Machine Learning in Customer Service
Machine learning is transforming customer service by providing efficient and personalized support, improving satisfaction levels, and reducing response times. The table below illustrates the impact of machine learning in customer service.
Customer Service Aspect | Impact |
---|---|
Chatbots and Virtual Assistants | 24/7 support with instant resolutions for common queries |
Customer Sentiment Analysis | Understanding customer emotions for improved service |
Recommendation Systems | Offering personalized product suggestions to customers |
Call Analysis | Automated call monitoring for quality assurance |
Machine learning and generative AI have rapidly transformed a multitude of industries. From healthcare and finance to art and gaming, these technologies are revolutionizing the way we operate. Leveraging huge datasets and complex algorithms, machine learning enables precise predictions and faster decision-making, while generative AI unleashes unparalleled creativity. As these technologies continue to advance, they hold immense potential for further innovation and disruption across various domains, ultimately shaping a future where technology seamlessly integrates into our lives.
Frequently Asked Questions
Machine Learning and Generative AI
- What is machine learning?
- Machine learning is a field of artificial intelligence that involves developing algorithms and models that enable computer systems to automatically learn and make predictions or decisions without being explicitly programmed.
- What is generative AI?
- Generative AI refers to a subset of artificial intelligence that focuses on creating models or systems capable of generating new and original content, such as images, music, or text.
- How does machine learning work?
- In machine learning, models are trained using data, which can be labeled or unlabeled, to find patterns and make predictions or decisions. The training process involves feeding the data into an algorithm that adjusts its internal parameters to minimize errors and improve performance.
- What are the types of machine learning?
- There are three main types of machine learning: supervised learning, unsupervised learning, and reinforcement learning. Supervised learning leverages labeled data to make predictions, unsupervised learning looks for patterns in unlabeled data, and reinforcement learning focuses on training agents to take actions in an environment to maximize rewards.
- What are some applications of machine learning?
- Machine learning has various applications, including but not limited to: image and speech recognition, natural language processing, recommendation systems, fraud detection, autonomous vehicles, and medical diagnosis.
- What are the main challenges in machine learning?
- Some challenges in machine learning include overfitting, underfitting, lack of interpretability of complex models, data quality and preprocessing, and scalability of algorithms to handle large datasets or real-time requirements.
- What is the role of data in machine learning?
- Data is a crucial component in machine learning as models rely on it for training and making predictions. High-quality and representative data is essential for achieving accurate and reliable results. Without sufficient and relevant data, machine learning models may struggle to generalize well to new unseen examples.
- How does generative AI differ from traditional AI?
- Generative AI differs from traditional AI in that it goes beyond performing predefined tasks and focuses more on creating new content or generating novel solutions. Traditional AI often revolves around executing predetermined rules or heuristics, while generative AI aims to produce outcomes that are not explicitly programmed.
- What are the potential benefits of generative AI?
- Generative AI can enable various applications such as realistic image synthesis, creative content generation, music composition, and even assist in drug discovery and other scientific research areas. It has the potential to automate and augment human creativity and enhance problem-solving capabilities.
- Are there any ethical concerns with machine learning and generative AI?
- Yes, machine learning and generative AI raise ethical concerns such as privacy, bias in data or model outputs, accountability for decision-making, and potential misuse of AI-generated content. It is important to address these issues and ensure responsible development and deployment of AI technologies.