Machine Learning as a Service (MLaaS)
With the rapid advancement of technology, machine learning has become an integral part of various industries. Machine Learning as a Service (MLaaS) has emerged as a convenient solution for businesses to access and utilize machine learning capabilities without having to invest in expensive hardware or infrastructure. MLaaS providers offer a range of pre-trained models and tools that can be easily integrated into existing systems, enabling businesses to leverage the power of machine learning in their operations.
Key Takeaways:
- MLaaS allows businesses to access and utilize machine learning capabilities without significant upfront costs.
- Providers offer a variety of pre-trained models and tools for easy integration.
- Data security and privacy should be considered when using MLaaS.
- MLaaS offers scalability and flexibility for businesses to meet their evolving needs.
How Does MLaaS Work?
MLaaS provides users with a platform to access and utilize machine learning algorithms, models and tools. *This enables businesses to easily incorporate machine learning into their operations, even without in-house expertise.* MLaaS platforms typically offer APIs (Application Programming Interfaces) that allow developers to integrate machine learning capabilities into their applications or systems. The models and algorithms provided by MLaaS are usually pre-trained on large datasets, making them ready to use without the need for extensive training and fine-tuning.
The Benefits of MLaaS
MLaaS offers several benefits for businesses:
- Cost-effectiveness: By utilizing MLaaS, businesses avoid the need for expensive infrastructure and hardware, reducing upfront costs.
- Accessibility: MLaaS platforms provide pre-trained models and tools that are readily available, enabling businesses to quickly implement machine learning capabilities.
- Scalability: MLaaS allows businesses to scale their machine learning operations as per their needs, without the need for additional hardware or resources.
- Flexibility: MLaaS platforms offer a range of algorithms and models, allowing businesses to choose the most suitable ones for their specific use cases.
- Time-saving: MLaaS eliminates the need for extensive training and fine-tuning of models, reducing the time required to integrate machine learning into workflows.
Important Considerations for MLaaS
While MLaaS brings numerous advantages, there are important considerations to keep in mind:
- Data Security: Businesses must ensure the vendor’s data security measures align with their requirements and industry standards.
- Data Privacy: Confidential or sensitive data must be adequately protected to avoid breaches or mishandling.
- Data Ownership: It is crucial to understand the ownership and rights related to the data used in MLaaS platforms.
- Performance: The performance of MLaaS models should be thoroughly evaluated to ensure they meet the required standards.
- Integration: Compatibility with existing systems and ease of integration is essential for a seamless implementation of MLaaS.
- Support and Documentation: Access to reliable support and comprehensive documentation from the MLaaS provider helps in troubleshooting and maximizing usage.
Comparison of Leading MLaaS Providers
Provider | Key Features | Pricing Model |
---|---|---|
Google Cloud ML Engine | Large-scale machine learning models, distributed training, and prediction. | Pay-As-You-Go or monthly packages based on usage. |
Amazon SageMaker | Managed environment for training, building, and deploying machine learning models. | Pay-As-You-Go or discounted pricing based on usage. |
Popular Use Cases for MLaaS
MLaaS can be applied to various domains and use cases, including:
- Fraud detection and prevention
- Recommendation systems
- Natural Language Processing (NLP)
- Image and video analysis
- Customer sentiment analysis
- Anomaly detection
MLaaS vs. Traditional Machine Learning
MLaaS differs from traditional machine learning in several ways:
- MLaaS eliminates the need for significant hardware and infrastructure investments, making it more cost-effective for businesses.
- Traditional machine learning requires in-house expertise for model development and maintenance, while MLaaS platforms offer pre-trained models and tools.
- MLaaS provides scalability and flexibility to meet the changing needs of businesses, allowing for quicker implementation.
- Traditional machine learning often requires extensive training and fine-tuning of models, whereas MLaaS models are typically pre-trained and ready to use.
Conclusion
Machine Learning as a Service (MLaaS) offers businesses of all sizes a convenient and cost-effective way to leverage the power of machine learning. By providing pre-trained models and tools, MLaaS platforms enable businesses to quickly integrate machine learning capabilities into their operations without significant upfront investments. However, careful attention must be paid to data security, privacy, and other considerations when opting for MLaaS. With a range of leading providers and numerous use cases, MLaaS continues to revolutionize industries and drive innovation forward.

Common Misconceptions
1. Machine Learning as a Service (MLaaS) is only for tech experts
One common misconception about MLaaS is that it is a complex technology that only experts in the field of technology can understand and use. However, MLaaS platforms are designed to be user-friendly, allowing users with little to no coding experience to easily access and utilize machine learning capabilities.
- MLaaS platforms provide intuitive user interfaces for easy interaction.
- Users can access pre-built machine learning models without the need to build their own.
- Many MLaaS platforms offer comprehensive documentation and tutorials to guide users through the process.
2. MLaaS replaces the need for data scientists
Another misconception is that MLaaS completely replaces the need for data scientists. While MLaaS platforms offer automated machine learning capabilities, they do not eliminate the need for human data scientists who can analyze and interpret the results.
- Data scientists are still necessary for understanding the context of the data and making informed decisions.
- MLaaS platforms can assist data scientists by automating certain parts of the machine learning process.
- Data scientists can leverage MLaaS to quickly iterate and experiment with different algorithms and models.
3. MLaaS provides 100% accurate predictions
MLaaS platforms are sometimes perceived as infallible prediction machines that produce 100% accurate results. However, like any technology, machine learning models have limitations and can make mistakes.
- MLaaS platforms have their own limitations, such as relying on the quality and quantity of available data.
- Predictions can be affected by biases in the data used for training the models.
- Users must evaluate and validate the predictions made by MLaaS platforms to ensure their accuracy and reliability.
4. MLaaS is only beneficial for large enterprises
Some people mistakenly believe that MLaaS is only beneficial for large enterprises with extensive resources. However, MLaaS can be advantageous for organizations of all sizes, including startups and small businesses.
- MLaaS eliminates the need for significant upfront infrastructure investments, making it cost-effective for small businesses.
- MLaaS offers scalability, allowing organizations to easily adjust their machine learning capabilities as their needs evolve.
- Small businesses can leverage MLaaS to gain insights from their data and make data-driven decisions without needing in-house data science expertise.
5. MLaaS always leads to job loss
One misconception is that the adoption of MLaaS will inevitably lead to job losses, particularly in the field of data science. While MLaaS can automate certain aspects of machine learning, it often complements and enhances human expertise rather than replacing it.
- Data scientists can focus on higher-value tasks such as model selection, performance evaluation, and addressing complex problems.
- The need for data scientists and ML experts will continue to grow as MLaaS adoption increases, as their expertise is crucial for interpreting and refining the machine learning models.
- MLaaS can free up time for data scientists to engage in more strategic work and drive innovation within organizations.
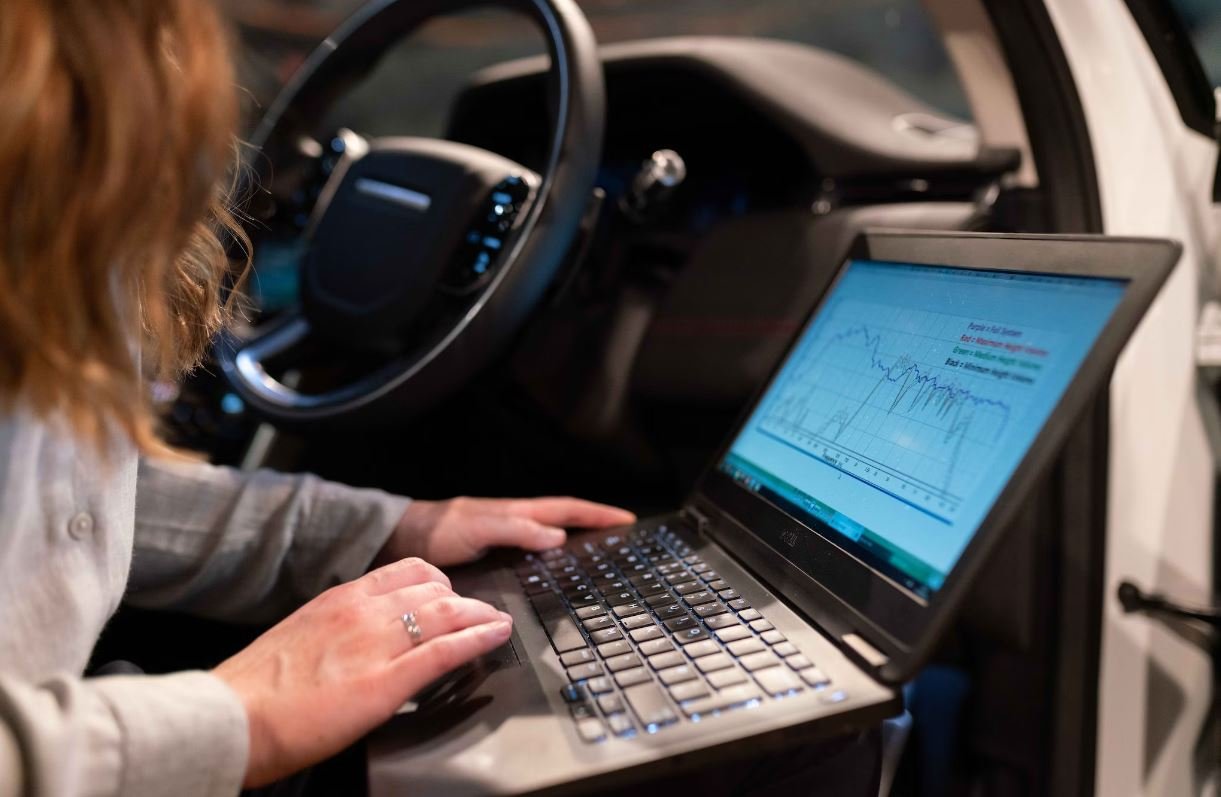
Table 1: Leading Providers of MLaaS
Machine Learning as a Service (MLaaS) has gained significant popularity in recent years, enabling businesses to access and utilize advanced machine learning algorithms without the need for extensive in-house resources. This table highlights some of the leading providers in the MLaaS market and the unique features they offer:
Provider | Key Features | Usage Cost (per month) | Availability |
---|---|---|---|
Amazon Web Services (AWS) | AWS SageMaker, scalable infrastructure, wide range of ML models | $10,000 | Global |
Microsoft Azure | Azure Machine Learning, automated model selection, data labeling | $7,500 | Global |
Google Cloud Platform (GCP) | Cloud AutoML, TensorFlow integration, large-scale data processing | $8,500 | Global |
IBM Watson | Watson Studio, deep learning frameworks, natural language processing | $9,000 | Global |
Table 2: Industries Leveraging MLaaS
Machine Learning as a Service finds applicability across various industries, empowering organizations to optimize their operations, enhance decision-making processes, and unlock valuable insights. This table showcases some industries utilizing MLaaS:
Industry | MLaaS Application | Benefits |
---|---|---|
Healthcare | Medical image analysis, disease prediction models | Improved diagnosis accuracy, personalized treatment plans |
Retail | Customer segmentation, demand forecasting | Enhanced customer experience, optimized inventory management |
Finance | Fraud detection, investment analysis | Reduced financial risks, enhanced portfolio management |
Manufacturing | Quality control, predictive maintenance | Improved product quality, minimized downtime and maintenance costs |
Table 3: Popular Machine Learning Algorithms
Machine Learning as a Service platforms offer a wide range of powerful algorithms that can be swiftly deployed to solve complex problems. Here are some commonly used algorithms:
Algorithm | Application | Advantages |
---|---|---|
Linear Regression | Price prediction, trend analysis | Interpretability, simplicity |
Random Forest | Classification, outlier detection | Robustness, handling non-linear relationships |
Support Vector Machines (SVM) | Text classification, image recognition | Effective in high-dimensional spaces, resilience to overfitting |
Deep Learning (Neural Networks) | Natural language processing, computer vision | Complex pattern recognition, great performance with big data |
Table 4: Comparison of MLaaS and On-Premises Deployment
Choosing between MLaaS and on-premises deployment is a critical decision for organizations. This table presents a comparison of the two approaches:
Aspect | MLaaS | On-Premises Deployment |
---|---|---|
Initial Cost | Low, pay-as-you-go pricing | High due to infrastructure setup and maintenance |
Scalability | Highly scalable, supports growing demands | Limited scalability, requires investment in new infrastructure |
Expertise Required | Minimal, MLaaS providers handle complexities | Extensive ML expertise needed for development and maintenance |
Flexibility | Offers a range of pre-built models and APIs | Allows customization of algorithms based on specific needs |
Table 5: Success Stories of MLaaS Implementation
Real-world success stories demonstrate the immense value MLaaS brings to businesses. Below are a few notable examples:
Company | Application | Outcome |
---|---|---|
Netflix | Recommendation system | Improved user engagement and personalized content suggestions |
Uber | Dynamic pricing | Optimized pricing strategies leading to increased revenue |
Coca-Cola | Supply chain optimization | Reduced costs by identifying optimal transportation routes |
SpaceX | Launch payload trajectory prediction | Enhanced mission success rate and improved fuel efficiency |
Table 6: Factors Influencing MLaaS Adoption
The adoption of MLaaS is influenced by various factors, both internal and external to organizations. This table highlights some key factors:
Factor | Impact |
---|---|
Data Privacy Regulations | Strong regulations demand secure cloud-based solutions |
Cost Considerations | Organizations evaluate the value MLaaS offers in comparison to costs |
Skill Gap | Limited in-house ML expertise drives adoption of MLaaS |
Time to Market | MLaaS enables greater speed and agility in deploying ML models |
Table 7: MLaaS Security Features
Ensuring the security and privacy of data is a primary concern for organizations leveraging MLaaS. The following table outlines some security features provided by MLaaS platforms:
Provider | Data Encryption | Access Controls | Secure APIs |
---|---|---|---|
Amazon Web Services (AWS) | At-rest and in-transit encryption | Identity and access management, resource policies | Throttling, authorization mechanisms |
Microsoft Azure | Encrypted storage, secure communication channels | Role-based access control, Azure Active Directory integration | API management, Azure Key Vault |
Google Cloud Platform (GCP) | Encryption at-rest, in-transit, and in-use | Identity and access management, VPC service controls | API authentication, threat detection and response |
IBM Watson | Data encryption in transit and at rest | Fine-grained access controls, multi-factor authentication | Secure API endpoints, security information and event management (SIEM) |
Table 8: Pros and Cons of MLaaS
As with any technology, Machine Learning as a Service comes with its advantages and drawbacks. Here is a balanced view of the pros and cons:
Pros | Cons |
---|---|
Low initial cost and pay-as-you-go pricing | Data privacy and security concerns in cloud-based solutions |
Rapid deployment and scalability | Reliance on third-party providers for critical services |
Reduced need for extensive in-house ML expertise | Limitations in customization and algorithm flexibility |
Access to cutting-edge ML algorithms and models | Potential vendor lock-in and dependency |
Table 9: MLaaS Pricing Comparison
Pricing models vary across MLaaS providers, and organizations consider several factors while evaluating costs. This table provides a comparison:
Provider | Subscription Pricing | Additional Charges |
---|---|---|
Amazon Web Services (AWS) | Monthly subscription based on usage tier | Data transfer, additional storage |
Microsoft Azure | Free tiers, pay-as-you-go, and enterprise plans | Compute and storage resources, bandwidth |
Google Cloud Platform (GCP) | Usage-based pricing with tiered rates | Ingress and egress network traffic, persistent disk usage |
IBM Watson | Flexible pricing options catering to enterprise needs | Data storage, API requests |
Table 10: MLaaS Market Forecast
The adoption of MLaaS is projected to experience substantial growth in the coming years. The following table presents the estimated market value for MLaaS:
Year | Estimated Market Value (USD Billion) |
---|---|
2022 | 17.5 |
2025 | 53.2 |
2030 | 118.7 |
Machine Learning as a Service (MLaaS) has revolutionized the way businesses leverage machine learning algorithms, providing cost-effective access to advanced models and algorithms. This article explored leading MLaaS providers, industries benefiting from MLaaS, popular algorithms, deployment comparisons, success stories, influencing factors, security features, pros and cons, pricing comparisons, and market forecasts. With the rapid growth of MLaaS solutions and their potential to transform industries, organizations are empowered to unlock valuable insights, enhance decision-making, and gain a competitive edge in today’s data-driven era.
Frequently Asked Questions
Machine Learning as a Service (MLaaS)
Q: What is Machine Learning as a Service (MLaaS)?
A: MLaaS is a cloud-based solution that enables users to utilize machine learning algorithms and models without having to build or maintain the infrastructure required for training and inference.
Q: What are the benefits of using MLaaS?
A: Using MLaaS allows businesses to leverage the power of machine learning without the need for extensive knowledge in the field. It reduces the time and cost required for development, deployment, and scaling of machine learning models. Additionally, MLaaS platforms typically provide pre-trained models that can be customized to specific needs.
Q: How does MLaaS work?
A: MLaaS providers offer an infrastructure where users can upload their data and access machine learning capabilities through APIs or web interfaces. The data is processed and analyzed using machine learning algorithms that generate predictions or insights. The results can then be used for various purposes such as decision-making, automation, or improving user experiences.
Q: What types of applications can benefit from MLaaS?
A: MLaaS can be beneficial in various domains including but not limited to e-commerce, finance, healthcare, recommendation systems, fraud detection, natural language processing, and computer vision. Its applications range from personalized product recommendations to medical diagnosis and autonomous driving.
Q: Are there any limitations in using MLaaS?
A: While MLaaS offers great benefits, it also has some limitations. For instance, the performance of the models may be affected by the quality and quantity of the provided data. Additionally, relying solely on pre-trained models may lack the specificity and customization required for certain use cases. Integrating MLaaS with existing systems and ensuring data privacy and security are also important considerations.
Q: What are the popular MLaaS platforms?
A: Some popular MLaaS platforms include Amazon SageMaker, Google Cloud Machine Learning Engine, Microsoft Azure Machine Learning, IBM Watson, and BigML. These platforms provide a range of features and services to cater to different user needs.
Q: Is MLaaS suitable for small businesses?
A: Yes, MLaaS can be a viable option for small businesses as it eliminates the need for large upfront investments in infrastructure and expertise. It allows small businesses to leverage the power of machine learning and gain competitive advantage by utilizing advanced technologies without significant financial burden.
Q: How can I get started with MLaaS?
A: To get started with MLaaS, you can explore different MLaaS providers and choose a platform that suits your requirements. Familiarize yourself with their documentation and APIs, and start experimenting with sample datasets and pre-trained models. As you gain proficiency, you can then progress to training your own models and integrating MLaaS into your existing systems.
Q: What should I consider when selecting an MLaaS provider?
A: When selecting an MLaaS provider, consider factors such as the platform’s ease of use, supported programming languages, availability of pre-built models, scalability, cost, integration capabilities, and compatibility with your existing infrastructure. Additionally, ensure that the provider offers reliable technical support, security measures, and data privacy policies that align with your organization’s requirements.
Q: Can I migrate my existing machine learning models to an MLaaS platform?
A: Yes, in most cases, you can migrate your existing machine learning models to an MLaaS platform. However, the process may involve some modifications or adjustments to adapt to the platform’s APIs and requirements. It is recommended to consult the platform’s documentation or seek assistance from their support team to ensure a smooth transition.