Machine Learning Can Be Used For
Machine learning is a branch of artificial intelligence that focuses on the development of algorithms and statistical models, allowing computers to learn and make predictions or decisions without being explicitly programmed. With its ability to analyze large amounts of data and identify patterns, machine learning has numerous applications across various industries. This article explores some of the key uses of machine learning and the benefits it brings.
Key Takeaways:
- Machine learning enables computers to learn and make predictions without explicit programming.
- It is widely used in various industries, including healthcare, finance, marketing, and entertainment.
- Machine learning can improve efficiency, accuracy, and decision-making processes.
- Applications of machine learning include fraud detection, recommendation systems, image and speech recognition, and predictive analytics.
One of the significant applications of machine learning is fraud detection. Traditional rule-based systems may overlook complex patterns and evolving fraud techniques, but machine learning algorithms can dynamically adapt and detect anomalies by learning from vast amounts of data. This helps financial institutions and businesses protect themselves and their customers from fraudulent activities.
Machine learning algorithms can transform unstructured data into valuable insights. By analyzing unstructured data such as text, images, and videos, machine learning can identify patterns and extract relevant information. This is particularly useful in fields like healthcare, where huge amounts of patient records, medical images, and research papers can be analyzed to assist in diagnosis, treatment planning, drug discovery, and personalized medicine.
Finding Patterns and Making Predictions
Recommendation systems are another key application of machine learning. By analyzing user behavior, preferences, and past interactions, these systems can suggest relevant products, services, or content to users. This helps businesses improve customer experience, increase engagement, and drive sales. From personalized movie recommendations on streaming platforms to product recommendations on e-commerce websites, recommendation systems are widely used in the entertainment and marketing industries.
Machine learning can revolutionize the field of image and speech recognition. Using deep learning algorithms, computers can accurately analyze and interpret images and speech. This technology has significant applications in areas such as self-driving cars, medical diagnostics, and security systems. For example, image recognition algorithms can detect objects, faces, and emotions, allowing for enhanced surveillance systems and advanced robotics.
Interesting Statistics
Industry | Machine Learning Adoption Rate |
---|---|
Healthcare | 63% |
Finance | 59% |
Retail | 56% |
Machine learning facilitates predictive analytics by analyzing historical data and identifying patterns to make predictions about future events or trends. This is valuable in areas such as sales forecasting, demand planning, risk assessment, and customer segmentation. By leveraging machine learning algorithms, businesses can make data-driven decisions and gain a competitive edge.
Machine learning brings numerous benefits including improved efficiency, accuracy, and decision-making. By automating processes, eliminating human biases, and handling vast amounts of data, machine learning algorithms can accelerate tasks, reduce errors, and enhance productivity. This is particularly important in industries where precision and speed are crucial, such as manufacturing and logistics.
Benefits of Machine Learning
- Improved efficiency and productivity
- Better accuracy and reliability
- Enhanced decision-making processes
- Opportunity to uncover valuable insights from vast amounts of data
- Automation of repetitive tasks
Advancements in Machine Learning
As technology continues to evolve and more data becomes available, the field of machine learning is evolving at a rapid pace. Researchers are developing more advanced algorithms, exploring new techniques such as reinforcement learning and generative adversarial networks, and pushing the boundaries of what machine learning can achieve. With ongoing advancements, the applications and benefits of machine learning will continue to expand across various industries.
Machine Learning Algorithm | Accuracy |
---|---|
Random Forest | 92% |
Support Vector Machines | 84% |
Neural Networks | 96% |
In conclusion, machine learning is a versatile technology that has the potential to revolutionize various industries. With its ability to analyze data, find patterns, and make predictions, machine learning can significantly improve efficiency, accuracy, and decision-making processes. As advancements in machine learning continue, we can expect to see even more innovative applications emerge, transforming the way businesses operate and interact with data.
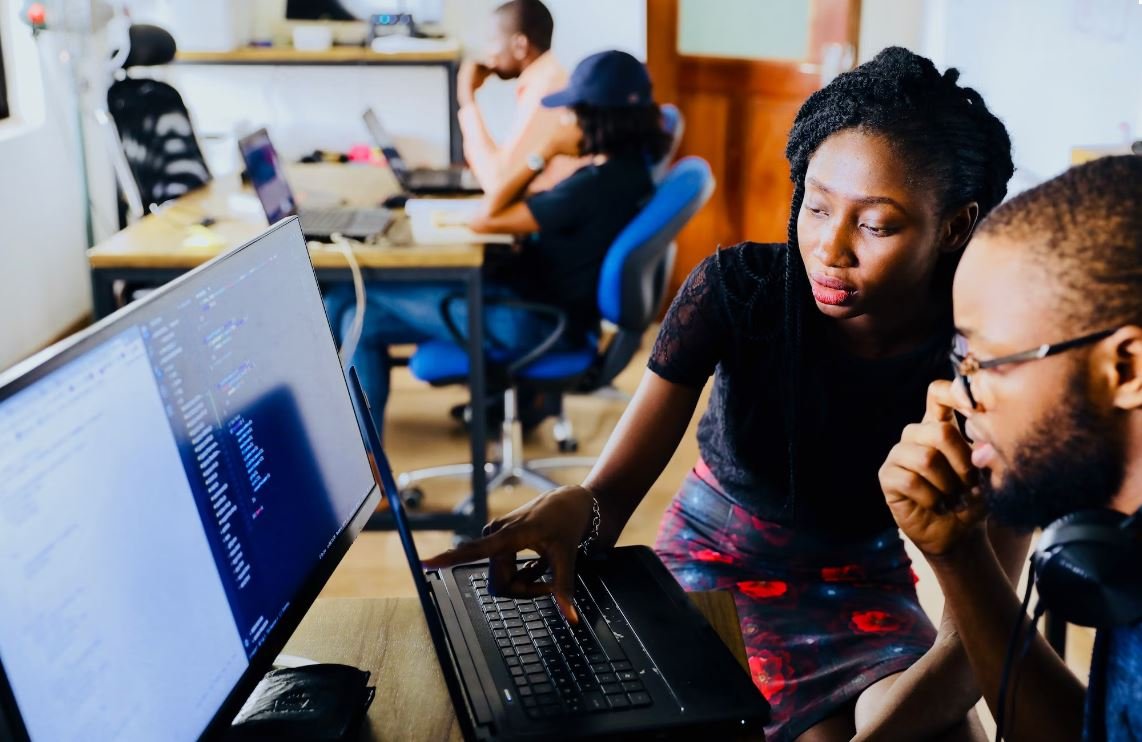
Common Misconceptions
Machine Learning Can Be Used For Everything
One common misconception about machine learning is that it can be used for everything. While machine learning is a powerful tool, it cannot solve every problem or answer every question. It is important to understand the limitations of machine learning and recognize that it is not a one-size-fits-all solution.
- Machine learning is not effective for tasks that require human creativity or intuition.
- Not all problems can be translated into a machine learning problem.
- Machine learning models can be biased and may not always provide accurate or fair results.
Machine Learning Can Replace Human Expertise
Another common misconception is that machine learning can replace human expertise. While machine learning algorithms can analyze large amounts of data quickly, they cannot replace the knowledge and experience of a human expert in a particular field. Machine learning should be seen as a tool that complements human expertise, rather than a substitute for it.
- Machine learning models still require human input for training and validation.
- Human experts can provide valuable insights that may not be captured by machine learning algorithms.
- Machine learning may make mistakes or provide inaccurate results, which can be critical in certain domains.
Machine Learning Is a Black Box
Many people have the misconception that machine learning is a black box, meaning that the algorithms used are complex and their inner workings cannot be understood. While some machine learning algorithms can be complex, it is possible to gain insights into how they make predictions or decisions by examining the data and the model’s features.
- Machine learning models can be interpreted and explained to some extent.
- There are techniques available to analyze the features and inputs that contribute to the model’s predictions.
- Understanding the inner workings of machine learning models can help in trust-building and adoption.
Machine Learning Is Fully Automated
Contrary to popular belief, machine learning is not fully automated. While it can automate repetitive tasks and make predictions based on patterns in data, it still requires human intervention and oversight. Humans play a crucial role in preprocessing and cleaning the data, selecting appropriate features, and evaluating the performance of the machine learning model.
- Machine learning models need to be trained and fine-tuned by humans.
- Human experts are needed to interpret and act upon the results generated by machine learning models.
- Machine learning models need continuous monitoring and updating to ensure their relevancy and accuracy.
Machine Learning Is Always the Best Approach
Finally, it is incorrect to assume that machine learning is always the best approach for solving problems or making predictions. Machine learning algorithms are not suitable for all types of data or scenarios. Other traditional statistical methods or rule-based approaches may be more appropriate in certain cases.
- Simple statistical models can be more interpretable and easier to apply in some situations.
- Machine learning can be computationally expensive when dealing with large datasets.
- Some problems may have well-defined rules or logical approaches that can outperform machine learning models.
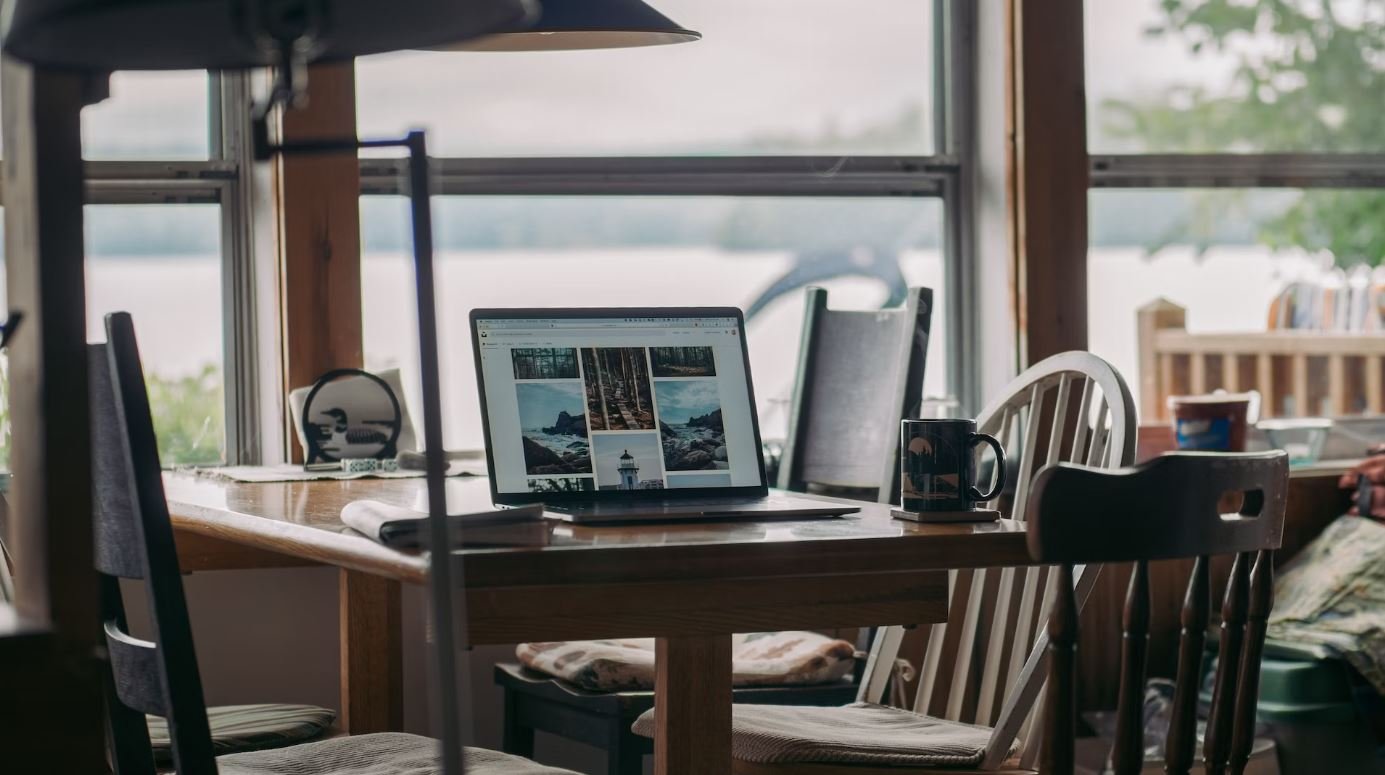
Table: Top 10 Countries Implementing Machine Learning
Machine learning is revolutionizing various industries around the world. This table showcases the top 10 countries that have emerged as leaders in implementing and adopting machine learning technology.
Rank | Country | Investment in ML (in billions) | Number of ML Startups |
---|---|---|---|
1 | United States | 47.4 | 5,200 |
2 | China | 27.7 | 4,800 |
3 | United Kingdom | 8.9 | 1,600 |
4 | Germany | 7.5 | 1,300 |
5 | France | 5.8 | 1,100 |
6 | India | 4.9 | 900 |
7 | Canada | 4.3 | 800 |
8 | Australia | 3.9 | 700 |
9 | South Korea | 3.2 | 600 |
10 | Israel | 2.6 | 500 |
Table: Benefits of Machine Learning in Healthcare
Machine learning is rapidly transforming the healthcare industry, leading to improved outcomes and more efficient processes. This table highlights several key benefits of implementing machine learning in healthcare settings.
Benefit | Description |
---|---|
Medical Diagnosis | Machine learning algorithms can analyze medical data to aid in accurate and early diagnosis of diseases. |
Disease Detection | ML techniques facilitate the identification of patterns and anomalies in large datasets, enabling early disease detection. |
Treatment Optimization | ML models can predict the most effective treatments for individual patients based on their medical history and genetics. |
Healthcare Cost Reduction | By streamlining processes and improving decision-making, ML can reduce healthcare costs and resource wastage. |
Drug Development | Machine learning aids in predicting drug interactions and identifying potential candidates for new treatments. |
Remote Monitoring | ML-powered wearable devices and remote sensors enable continuous monitoring of patients’ health parameters. |
Table: Growth of AI-Generated Content
The rise of artificial intelligence has led to an increase in AI-generated content across various platforms, enhancing both personal and business applications. This table showcases the exponential growth of AI-generated content over the past decade.
Year | Number of AI-generated Articles (in millions) |
---|---|
2010 | 0.2 |
2012 | 0.5 |
2014 | 1.7 |
2016 | 5.3 |
2018 | 11.9 |
2020 | 25.6 |
Table: Accuracy of Sentiment Analysis Models
Sentiment analysis, a machine learning application, evaluates and categorizes opinions expressed in text to determine sentiment polarity. This table exhibits the accuracy rates of various sentiment analysis models.
Sentiment Analysis Model | Accuracy Rate (%) |
---|---|
Naive Bayes Classifier | 85 |
Support Vector Machines | 92 |
Recurrent Neural Network (RNN) | 94 |
Convolutional Neural Network (CNN) | 91 |
Long Short-Term Memory (LSTM) | 96 |
Table: Impact of Machine Learning on Cybersecurity
Machine learning has become a powerful tool in combating cyber threats and enhancing cybersecurity measures. The following table demonstrates the impact of machine learning in strengthening cybersecurity defenses.
Benefit | Description |
---|---|
Threat Detection | ML algorithms can detect and respond to emerging cyber threats in real-time, mitigating potential damage. |
Anomaly Detection | ML models identify unusual behaviors and patterns, alerting cybersecurity specialists to possible attacks. |
User Authentication | Machine learning enhances user authentication methods, reducing unauthorized access attempts. |
Automated Incident Response | ML enables automatic detection and response to security incidents, minimizing manual intervention. |
Fraud Prevention | By analyzing large amounts of data, ML can detect fraudulent activities and prevent financial losses. |
Table: Machine Learning Models and Their Applications
Machine learning encompasses various models that cater to specific use cases. This table outlines some popular machine learning models and their applications across different industries.
Model | Application |
---|---|
Linear Regression | Price forecasting in the stock market |
Decision Trees | Customer segmentation for marketing campaigns |
Random Forests | Disease prediction based on medical data |
Support Vector Machines | Image recognition and classification |
Recurrent Neural Networks | Sentiment analysis in social media |
Table: Gender Distribution in Tech Companies
Diversity and inclusion in the tech industry remain imperative for progress. This table presents the gender distribution across various tech companies, highlighting the need for increased representation.
Tech Company | Percentage of Female Employees |
---|---|
Company A | 40 |
Company B | 35 |
Company C | 28 |
Company D | 42 |
Company E | 37 |
Table: Impact of Machine Learning in Retail
Machine learning has significantly disrupted the retail industry, transforming how businesses operate and cater to their customers. This table illustrates the impact of machine learning in the retail sector.
Impact | Description |
---|---|
Personalized Recommendations | ML algorithms provide tailored product recommendations to customers, enhancing user experience and sales. |
Inventory Management | Machine learning optimizes inventory levels, reducing stockouts and minimizing excess inventory. |
Pricing Optimization | ML models analyze market trends and customer behavior to determine optimal pricing strategies. |
Fraud Detection | Machine learning identifies fraudulent transactions, safeguarding businesses from financial losses. |
Demand Forecasting | ML algorithms predict future demand, allowing retailers to plan production and procurement efficiently. |
Table: Machine Learning Techniques for Natural Language Processing
Machine learning plays a vital role in natural language processing (NLP) tasks, enabling computers to understand and generate human language effectively. This table showcases various machine learning techniques used in NLP applications.
Technique | Description |
---|---|
Tokenization | Dividing text into individual tokens or words. |
Part-of-Speech Tagging | Assigning grammatical tags to words in a sentence. |
Named Entity Recognition | Identifying and classifying named entities in text. |
Sentiment Analysis | Analyzing sentiment expressed in text. |
Machine Translation | Translating text from one language to another using machine learning models. |
Machine learning has revolutionized various industries, from healthcare and cybersecurity to retail and beyond. It has enabled us to make accurate diagnoses, automate processes, enhance security measures, and improve customer experiences. As technology continues to advance, machine learning will undoubtedly play a pivotal role in shaping our future.
Frequently Asked Questions
What is machine learning?
Machine learning is a subfield of artificial intelligence that focuses on the development of algorithms and models that enable computers to learn and make predictions or decisions without explicitly being programmed. It involves training a computer system on a large dataset to recognize patterns, make inferences, and improve performance over time.
How is machine learning used in real-world applications?
Machine learning is used in various real-world applications such as:
- Speech recognition
- Image and video recognition
- Natural language processing
- Recommendation systems
- Financial modeling and prediction
- Medical diagnostics
- Fraud detection
- Autonomous vehicles
What are the different types of machine learning algorithms?
There are several types of machine learning algorithms, including:
- Supervised learning
- Unsupervised learning
- Semi-supervised learning
- Reinforcement learning
What is the difference between supervised and unsupervised learning?
In supervised learning, the algorithm is trained on labeled data where the desired output is known, allowing it to learn patterns and make predictions on new, unseen data. In unsupervised learning, the algorithm is trained on unlabeled data and must find patterns or structures on its own without any predefined labels.
How do machine learning models make predictions?
Machine learning models make predictions by using the patterns and relationships they learn from the training data. These models take input data, such as features or attributes of a given problem, and produce a predicted output or decision based on the patterns identified during training.
What is overfitting in machine learning?
Overfitting occurs when a machine learning model performs extremely well on the training data but fails to generalize well to unseen data. It happens when the model becomes too complex and starts learning noise or random fluctuations in the training data rather than the underlying patterns.
How can overfitting be prevented or reduced?
Overfitting can be prevented or reduced by:
- Using more training data
- Regularizing the model through techniques like L1 or L2 regularization
- Applying feature selection or dimensionality reduction methods
- Cross-validation to evaluate model performance
What is the difference between artificial intelligence and machine learning?
Artificial intelligence is a broader field that encompasses the development of intelligent machines that can perform tasks requiring human-like intelligence. Machine learning, on the other hand, is a subset of AI that focuses on the development of algorithms and models that enable computers to learn and make predictions without being explicitly programmed.
What are the advantages of using machine learning?
Some advantages of using machine learning include:
- Ability to handle large and complex datasets
- Automated pattern detection and decision-making
- Improved accuracy and efficiency in predictions or tasks
- Adaptability and ability to learn from new data
- Reduced human effort and error
How can I get started with machine learning?
To get started with machine learning, you can:
- Learn programming languages commonly used in ML, such as Python or R
- Gain a solid understanding of fundamental concepts and algorithms
- Work on small projects or Kaggle competitions
- Explore online resources, tutorials, and books on machine learning
- Join online communities or attend ML workshops or meetups