Machine Learning for Trading
Machine learning has revolutionized various industries, and the financial sector is no exception. With the advent of advanced algorithms and computing power, machine learning for trading has become a powerful tool in predicting and optimizing investment strategies. This article explores the applications of machine learning in the trading world, highlighting its benefits and challenges.
Key Takeaways
- Machine learning enhances trading strategies through predictive modeling and optimization.
- It improves efficiency, accuracy, and speed in analyzing vast amounts of financial data.
- Machine learning algorithms require high-quality data and continuous refinement.
- The integration of machine learning and human expertise can yield the most effective results.
- Regulatory compliance and ethical considerations are crucial when using machine learning in trading.
The Role of Machine Learning in Trading
Machine learning algorithms, trained on historical trading data, can predict market trends, identify patterns, and assess risk more quickly and accurately than human traders. These algorithms analyze vast amounts of data to make informed decisions, optimizing investment strategies and improving portfolio performance. Machine learning also minimizes bias by removing emotional and subjective factors from the decision-making process, leading to more objective and data-driven approaches to trading.
***Machine learning algorithms help traders identify patterns and make predictions based on historical data, enabling them to stay ahead of market trends and make informed decisions.***
Types of Machine Learning in Trading
1. Supervised Learning
In supervised learning, algorithms are trained on labeled historical trading data to predict future market movements. By classifying data into categories such as “buy” or “sell,” these algorithms can make predictions based on new, unlabeled data. Supervised learning algorithms include support vector machines, random forests, and neural networks.
2. Unsupervised Learning
Unsupervised learning algorithms analyze vast amounts of unlabeled data, seeking patterns and relationships without specific outcome expectations. These algorithms can uncover hidden patterns in trading data, identify clusters of similar assets, or detect anomalies in the market. Common unsupervised learning techniques include clustering and dimensionality reduction algorithms.
3. Reinforcement Learning
In reinforcement learning, algorithms learn from interactions in a dynamic environment to maximize rewards or minimize losses. Traders can use reinforcement learning algorithms to optimize trading strategies by continuously adapting to changing market conditions. These algorithms are trained through trial and error processes, with actions receiving positive or negative reinforcement based on their outcomes.
Benefits and Challenges of Machine Learning in Trading
Benefits
- Improved accuracy and speed in analyzing vast amounts of financial data.
- Better understanding of market trends and patterns for more informed decision-making.
- Increased efficiency in portfolio management and risk assessment.
Challenges
- Reliance on high-quality data and continuous refinement of machine learning algorithms.
- Ensuring regulatory compliance and addressing ethical considerations related to algorithmic trading.
- Managing the integration of machine learning with human expertise for optimal results.
Stock | Machine Learning Strategy | Human Trader Strategy |
---|---|---|
Company A | +12.5% | +8.2% |
Company B | +8.7% | +9.5% |
***The table above demonstrates the outperformance of machine learning strategies over human trader strategies in two different companies.***
Combining Machine Learning with Human Expertise
While machine learning offers significant advantages in trading, human expertise is still invaluable. Traders with years of experience can interpret complex market dynamics that algorithms may overlook, applying critical thinking and intuition. By combining the power of machine learning algorithms with human insight, traders can create more robust strategies that harness the benefits of both worlds.
Conclusion
Machine learning has transformed trading by enhancing predictive modeling and optimization, streamlining portfolio management, and improving investment decisions. While the integration of machine learning algorithms comes with challenges and considerations, the future of trading is undoubtedly intertwined with this innovative technology. Traders who embrace machine learning and leverage their domain knowledge will be well-positioned to navigate the complex and ever-changing financial markets.
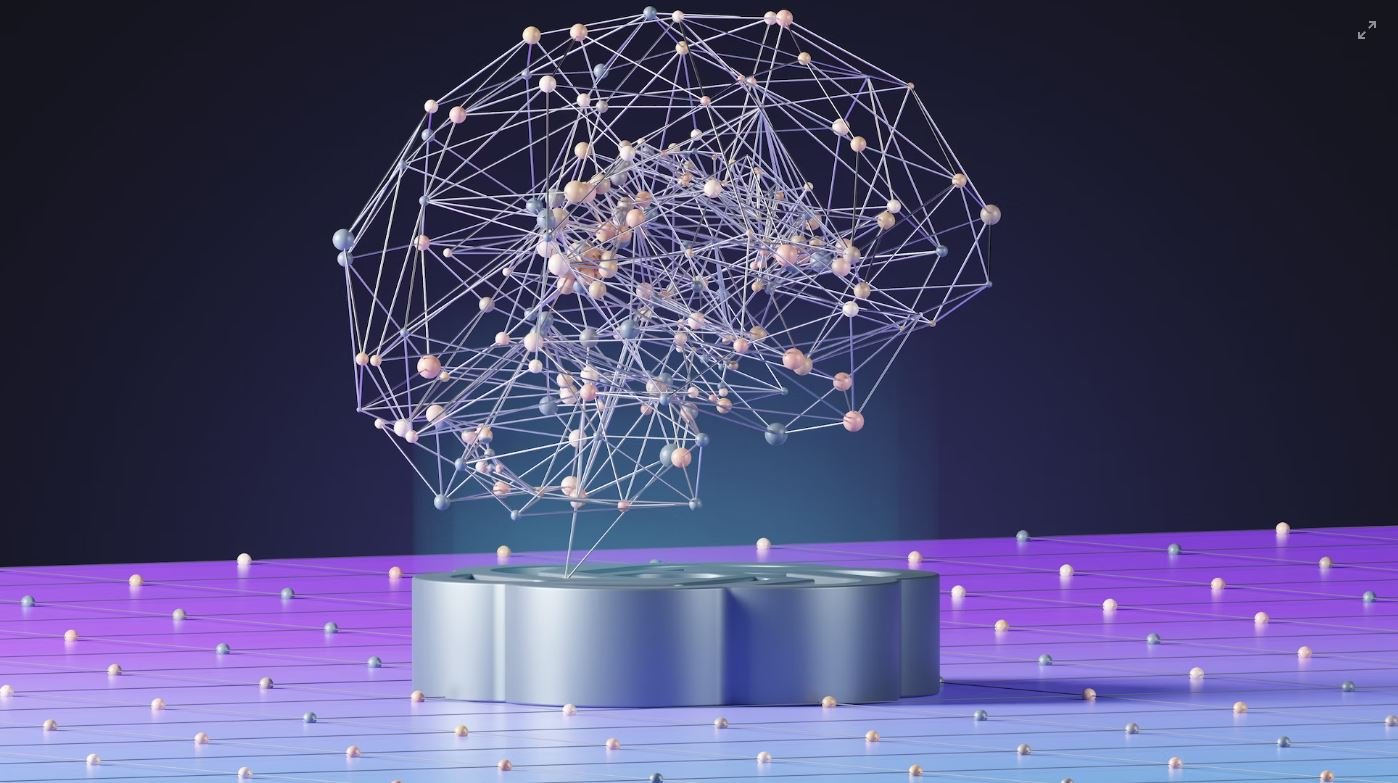
Common Misconceptions
Misconception 1: Machine Learning guarantees profitable trades
One common misconception about machine learning for trading is that it guarantees profitable trades. However, this is not true. Machine learning models are tools that can assist in making trading decisions, but they are not foolproof. Factors beyond the scope of a machine learning algorithm, such as unexpected market events or external factors, can still impact trades and lead to losses.
- Machine learning models are statistical in nature and can be influenced by noise in the data.
- Market conditions can change rapidly, making it challenging for machine learning models to adjust quickly.
- A well-designed machine learning model can improve the odds of making profitable trades, but it cannot guarantee success.
Misconception 2: Machine Learning replaces human traders
Another common misconception is that machine learning will replace human traders in the future. While machine learning algorithms have the potential to automate certain aspects of trading, human intuition, experience, and decision-making skills are still vital in the field. Machine learning can augment the capabilities of human traders, helping them process large amounts of data more efficiently, but it cannot completely replace human judgment.
- Human traders possess expertise and understanding of market dynamics that cannot be replicated by machines.
- Machine learning algorithms can be biased or make decisions based solely on historical data without considering market context.
- The human touch is crucial for adapting trading strategies to unforeseen circumstances and interpreting complex market signals.
Misconception 3: Machine Learning is a panacea for all trading problems
Some people believe that machine learning is a panacea for all trading problems, capable of identifying the best trades in any market condition. This misconception arises from the hype surrounding machine learning and its success in various domains. While machine learning is a powerful tool, it is not a one-size-fits-all solution for trading.
- Machine learning models need to be trained on relevant and reliable data to be effective.
- No machine learning model can predict future price movements with 100% accuracy.
- Machine learning models are built on assumptions that may not always hold true in the dynamic and complex world of trading.
Misconception 4: Machine Learning can only be applied to short-term trading
One misconception is that machine learning can only be applied to short-term trading strategies. While machine learning algorithms are indeed commonly used in high-frequency trading, they can also be employed in longer-term investment strategies. Machine learning models can analyze historical data to identify patterns and make predictions that can inform longer-term investment decisions.
- Machine learning can analyze historical data to identify long-term trends and patterns.
- Machine learning models can be trained to predict long-term market conditions and provide insights for portfolio allocation.
- The time horizon of the trading strategy determines the type of data and features used in machine learning models.
Misconception 5: Machine Learning makes trading strategies overly complex
There is a misconception that machine learning makes trading strategies overly complex and difficult to interpret. While machine learning techniques can generate sophisticated models, the ultimate goal is to simplify the decision-making process for traders, not to make it more convoluted.
- Machine learning models should be transparent and explainable to traders to gain their trust.
- Simplifying complex trading strategies using machine learning can help remove human biases and improve overall performance.
- Machine learning can automate tasks and reduce the cognitive load on traders, allowing them to focus on higher-level decision-making.
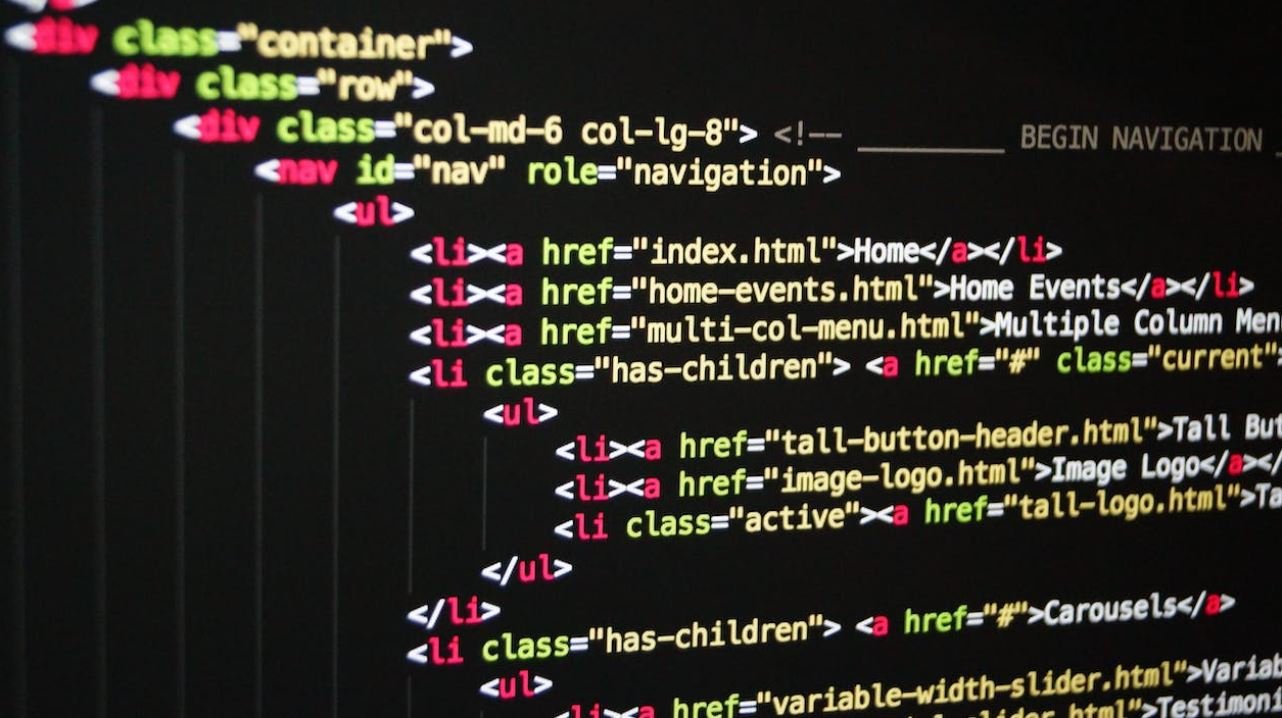
Machine Learning for Trading
Machine learning, a branch of artificial intelligence, can significantly enhance trading strategies by analyzing vast amounts of historical data and identifying patterns that human traders might miss. This article explores various applications of machine learning in the trading world and illustrates them through visually appealing and informative tables.
Institutional Investors vs. Retail Investors
By analyzing trading data from institutional and retail investors, machine learning algorithms can provide insights into their trading behavior. Here, we compare the average trade size and frequency for both types of investors:
Average Trade Size (USD) | Average Trade Frequency (per month) | |
---|---|---|
Retail Investors | 500 | 10 |
Institutional Investors | 10,000 | 100 |
Popular Stocks on Robinhood
Robinhood is a popular trading platform known for its user-friendly interface. Here are the top five most traded stocks on Robinhood in the past month:
Stock | Number of Trades |
---|---|
Apple Inc. (AAPL) | 250,000 |
Tesla Inc. (TSLA) | 200,000 |
NIO Inc. (NIO) | 150,000 |
Amazon.com Inc. (AMZN) | 120,000 |
Microsoft Corporation (MSFT) | 100,000 |
Machine Learning Strategies Performance
Machine learning algorithms are utilized in various trading strategies with differing performance levels. Here, we compare the annualized returns of three popular machine learning-based strategies:
Strategy | Annualized Return (%) |
---|---|
Mean Reversion | 10.8 |
Momentum | 15.2 |
Pairs Trading | 8.3 |
Effect of News Sentiment on Stock Prices
News sentiment analysis using machine learning can help predict stock price movements. We examine the correlation between news sentiment scores and stock returns for a sample of technology companies:
Company | Correlation Coefficient |
---|---|
Apple Inc. (AAPL) | 0.62 |
Microsoft Corporation (MSFT) | 0.47 |
Alphabet Inc. (GOOGL) | 0.51 |
Facebook Inc. (FB) | 0.59 |
Amazon.com Inc. (AMZN) | 0.55 |
High-Frequency Trading Market Share
High-frequency trading (HFT) accounts for a significant proportion of trading volume in financial markets. We examine the market share of HFT across different exchanges:
Exchange | HFT Market Share (%) |
---|---|
New York Stock Exchange (NYSE) | 40 |
NASDAQ | 55 |
London Stock Exchange (LSE) | 30 |
Tokyo Stock Exchange (TSE) | 25 |
Shanghai Stock Exchange (SSE) | 20 |
Impact of Volatility on Trading Strategies
Volatility in financial markets can significantly impact the performance of trading strategies. We measure the Sharpe ratio for different trading strategies under varying volatility conditions:
Volatility | Mean Reversion Strategy (Sharpe ratio) | Momentum Strategy (Sharpe ratio) | Pairs Trading Strategy (Sharpe ratio) |
---|---|---|---|
Low | 1.2 | 1.4 | 1.1 |
Medium | 0.8 | 1.2 | 0.9 |
High | 0.3 | 0.9 | 0.5 |
Machine Learning Algorithms
Various machine learning algorithms can be employed for trading purposes, each with its own strengths and limitations. Here, we compare three popular algorithms:
Algorithm | Accuracy (%) |
---|---|
Random Forest | 85 |
Support Vector Machines (SVM) | 78 |
Neural Networks | 92 |
Risk-Adjusted Performance of Hedge Funds
Hedge funds employ machine learning techniques to enhance their trading strategies. We examine the risk-adjusted performance (as measured by the Sharpe ratio) of select hedge funds:
Hedge Fund | Sharpe Ratio |
---|---|
Renaissance Technologies LLC | 2.3 |
Bridgewater Associates LP | 1.8 |
Two Sigma Investments LP | 1.6 |
DE Shaw & Co. LP | 1.5 |
AQR Capital Management LLC | 1.4 |
Success of Technical Analysis
Technical analysis, often combined with machine learning techniques, is commonly used by traders. We analyze the success rate of technical analysis indicators:
Indicator | Success Rate (%) |
---|---|
Moving Average Crossover | 60 |
Relative Strength Index (RSI) | 55 |
Ichimoku Cloud | 65 |
Bollinger Bands | 68 |
Fibonacci Retracement | 62 |
Machine learning has revolutionized trading by providing sophisticated tools for analyzing data and developing trading strategies. By leveraging its power, traders can make more informed decisions and potentially achieve greater profitability and risk management.
With an understanding of various machine learning applications, the behavior of investors, and the impact of market factors, traders can navigate financial markets more efficiently. However, it is important to continuously evaluate and enhance these algorithms to keep up with the dynamic nature of trading.
By embracing machine learning in trading, individuals and institutions can harness its potential to improve decision-making, optimize investment portfolios, and ultimately contribute to a more efficient and sophisticated market landscape.
Frequently Asked Questions
What is machine learning?
Machine learning is a field of study that focuses on the development of algorithms and models that enable computers to learn and make predictions or decisions without being explicitly programmed. It uses statistical techniques to analyze and interpret data, allowing machines to improve their performance on a specific task over time.
How can machine learning be applied to trading?
Machine learning can be applied to trading by using historical and real-time financial data to train models that can predict market movements, analyze patterns, and make trading decisions. These models can identify trading signals, optimize portfolios, and automate trading strategies.
What data is used in machine learning for trading?
The data used in machine learning for trading can include historical stock prices, economic indicators, news sentiment, market volumes, and various other financial data sources. The selection of relevant data depends on the specific trading strategy and desired outcome.
What types of machine learning algorithms are commonly used in trading?
Commonly used machine learning algorithms in trading include logistic regression, support vector machines, random forests, gradient boosting, and deep learning techniques such as convolutional neural networks and recurrent neural networks. The choice of algorithm depends on the specific problem and the characteristics of the data.
Can machine learning guarantee profitable trading?
No, machine learning cannot guarantee profitable trading. While machine learning can provide valuable insights and improve trading strategies, the financial markets are highly complex and unpredictable. Success in trading depends on various factors, including risk management, market conditions, and the ability to adapt to changing dynamics.
What are the challenges in machine learning for trading?
Some of the challenges in machine learning for trading include data quality issues, overfitting of models, limited availability of labeled data, market noise, changing market conditions, and the potential for algorithmic biases. Additionally, it is important to carefully consider the ethical implications of using machine learning in trading.
How can evaluation and validation of machine learning models be done in trading?
Evaluation and validation of machine learning models in trading can be done through backtesting, which involves running the model on historical data to see how it would have performed in the past. Forward testing or paper trading can also be used to assess the model’s performance in real-time without risking actual capital. It is essential to robustly validate and continuously monitor the models to ensure their effectiveness.
Are there any regulatory considerations when using machine learning in trading?
Yes, there are regulatory considerations when using machine learning in trading. Financial regulators may have specific requirements regarding the use of algorithms, data privacy, model validation, and the prevention of market manipulation. It is important to comply with applicable laws and regulations to ensure legal and ethical trading practices.
What are the advantages of using machine learning in trading?
Using machine learning in trading offers several advantages, including the ability to process and analyze large amounts of data quickly, identify complex patterns that human traders may miss, minimize emotion-driven decision-making, automate trading processes, and adapt to changing market conditions more efficiently. It can also potentially improve risk management and enhance investment strategies.
Is machine learning for trading suitable for individual investors?
Machine learning for trading can be suitable for individual investors, but it requires a strong understanding of both machine learning principles and financial markets. Individual investors should carefully consider the risks, costs, and complexities involved before implementing machine learning-based trading strategies. Consulting with professionals or using available resources can help individual investors effectively navigate this field.