Machine Learning to Predict Weather
Weather prediction has always been a challenging task, but with the advent of machine learning (ML) techniques, significant progress has been made in this field. ML algorithms have enabled meteorologists to forecast weather patterns with greater accuracy and precision, providing valuable insights for a variety of industries and sectors.
Key Takeaways
- Machine learning algorithms have improved weather prediction capabilities.
- ML models analyze large amounts of data and identify patterns to make accurate forecasts.
- Weather predictions benefit multiple sectors, including agriculture, transportation, and disaster management.
Machine learning models employ complex statistical algorithms to analyze large volumes of historical weather data, including temperature, humidity, wind patterns, and atmospheric pressure. These models then identify patterns and relationships among various weather variables, enabling them to generate accurate predictions for future weather conditions. *This data-driven approach allows for more precise forecasting based on observed patterns.*
The use of machine learning in weather prediction has brought significant advancements. By leveraging historical data and learning from past weather patterns, ML models can generate forecasts that help individuals and organizations make informed decisions. For example, farmers can analyze weather predictions to determine the best time for planting and harvesting crops, while shipping companies can optimize logistics and adjust routes based on forecasted weather conditions. *This integration of ML into weather forecasting provides valuable insights and enhances decision-making processes.*
Advantages of Machine Learning in Weather Prediction |
---|
Improved accuracy and precision in weather forecasts. |
Better understanding and prediction of extreme weather events. |
Real-time monitoring and alert systems for severe weather conditions. |
Machine learning algorithms can be trained to detect patterns associated with extreme weather events, such as hurricanes, tornadoes, and thunderstorms. By analyzing historical data and identifying key parameters leading to these events, ML models can predict their occurrence and intensity, providing valuable information to emergency response teams and the public. *This proactive identification of extreme weather events can help mitigate potential damages and save lives.*
Weather Prediction Models
Several different machine learning models are utilized in weather prediction:
- Regression-based models: These models predict weather variables based on historical data and statistical relationships. They are commonly used for short-term weather forecasts.
- Neural networks: These models mimic the structure and functionality of the human brain and are capable of learning complex patterns. They are often used for medium to long-term weather forecasts.
- Support Vector Machines (SVM): SVM models use historical data to create decision boundaries and classify weather patterns. They are particularly effective in predicting extreme weather events.
Accuracy Comparison of Machine Learning Models | Regression-Based Models | Neural Networks | Support Vector Machines (SVM) |
---|---|---|---|
Short-term Forecasts | High accuracy | Good accuracy | Moderate accuracy |
Medium-term Forecasts | Moderate accuracy | High accuracy | Good accuracy |
Long-term Forecasts | Low accuracy | Moderate accuracy | High accuracy |
It is important to note that machine learning models for weather prediction are continuously evolving, benefiting from ongoing research and advancements in the field. Scientists and researchers are continuously refining these models by incorporating more data sources, improving feature selection techniques, and exploring new algorithms to further enhance accuracy and reliability.
Machine learning has revolutionized the field of weather prediction, enabling more precise and reliable forecasts. With this technology, industries and sectors that rely on accurate weather information can make more informed decisions, increasing productivity, reducing risk, and improving overall efficiency. As machine learning continues to advance, the future of weather forecasting looks promising, with even higher accuracy and real-time predictions on the horizon.
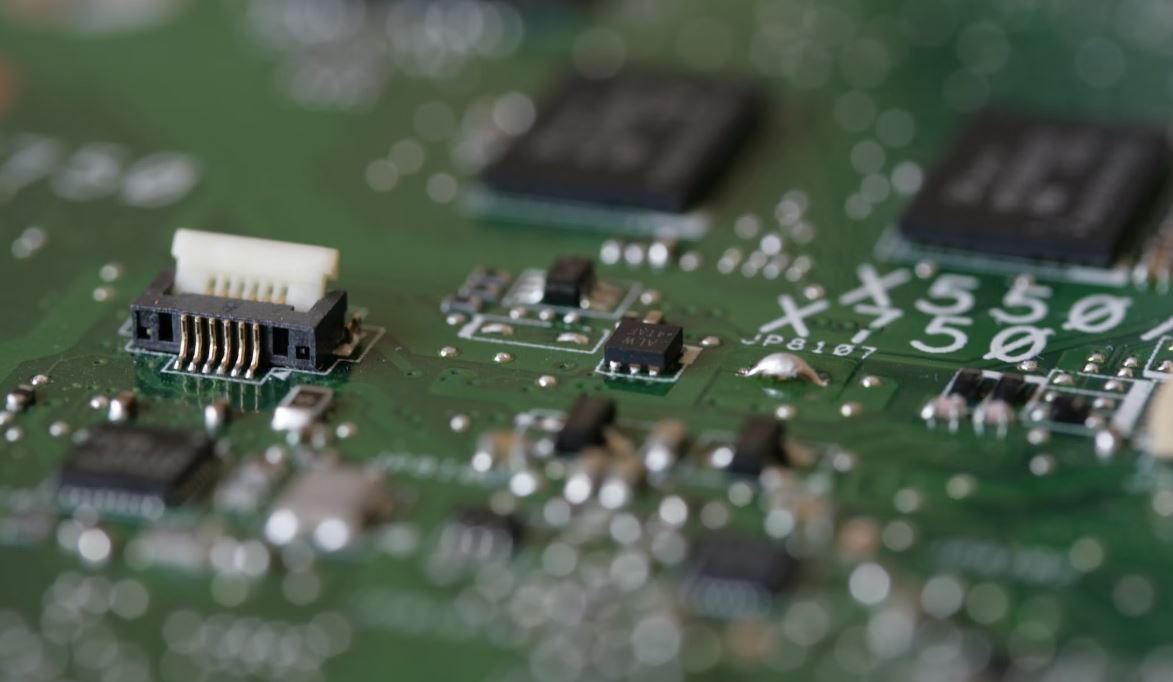
Common Misconceptions
Machine Learning to Predict Weather
Machine learning, despite its growing popularity in recent years, is still surrounded by several misconceptions when it comes to predicting the weather. Understanding these common misconceptions is crucial to grasp the true potential and limitations of machine learning in weather prediction.
- Machine learning can accurately predict weather events with absolute certainty.
- Machine learning models can replace traditional forecasting methods entirely.
- Machine learning is a magical tool that can predict weather patterns accurately even with limited or poor-quality data.
Machine Learning and Accurate Predictions
One common misconception is that machine learning can accurately predict weather events with complete certainty. While machine learning algorithms can make predictions based on historical weather data, it is important to remember that weather is a complex and dynamic system influenced by numerous factors. Therefore, the accuracy of machine learning predictions may vary in different situations.
- Weather prediction models are probabilistic in nature and provide estimates rather than absolute predictions.
- Predicting extreme weather events accurately with machine learning models can be challenging due to their inherent volatility.
- Localized weather phenomena can be more difficult to predict using machine learning algorithms due to the limited availability of high-resolution data.
Replacing Traditional Forecasting Methods
Another misconception is that machine learning models can entirely replace traditional forecasting methods. While machine learning can supplement traditional methods and improve prediction accuracy, it cannot replace the experience and expertise of human meteorologists or the effectiveness of well-established forecasting techniques.
- Traditional weather forecasting methods incorporate a wide range of information, including atmospheric physics and meteorological data, which machine learning models may not fully capture.
- Human forecasters often take into account contextual factors, such as local topography, that machine learning algorithms may not consider.
- Combining machine learning with traditional methods can enhance accuracy and provide more reliable predictions.
Limitations of Limited or Poor-Quality Data
Some individuals mistakenly believe that machine learning can generate accurate weather predictions even with limited or poor-quality data. However, machine learning models heavily rely on the quality and quantity of data available for training. Insufficient or inaccurate data can lead to unreliable predictions.
- Accurate weather predictions require large volumes of high-quality data collected over a considerable period.
- Misinterpretation or biases in the data used for training machine learning models can result in misleading predictions.
- Data gaps or missing observations can adversely affect the performance of machine learning algorithms.
Conclusion
Understanding the common misconceptions associated with machine learning in weather prediction is essential for appreciating its benefits and limitations. While machine learning can enhance weather forecasting accuracy, it is not a foolproof solution, and human expertise and traditional methods remain invaluable in ensuring reliable predictions.
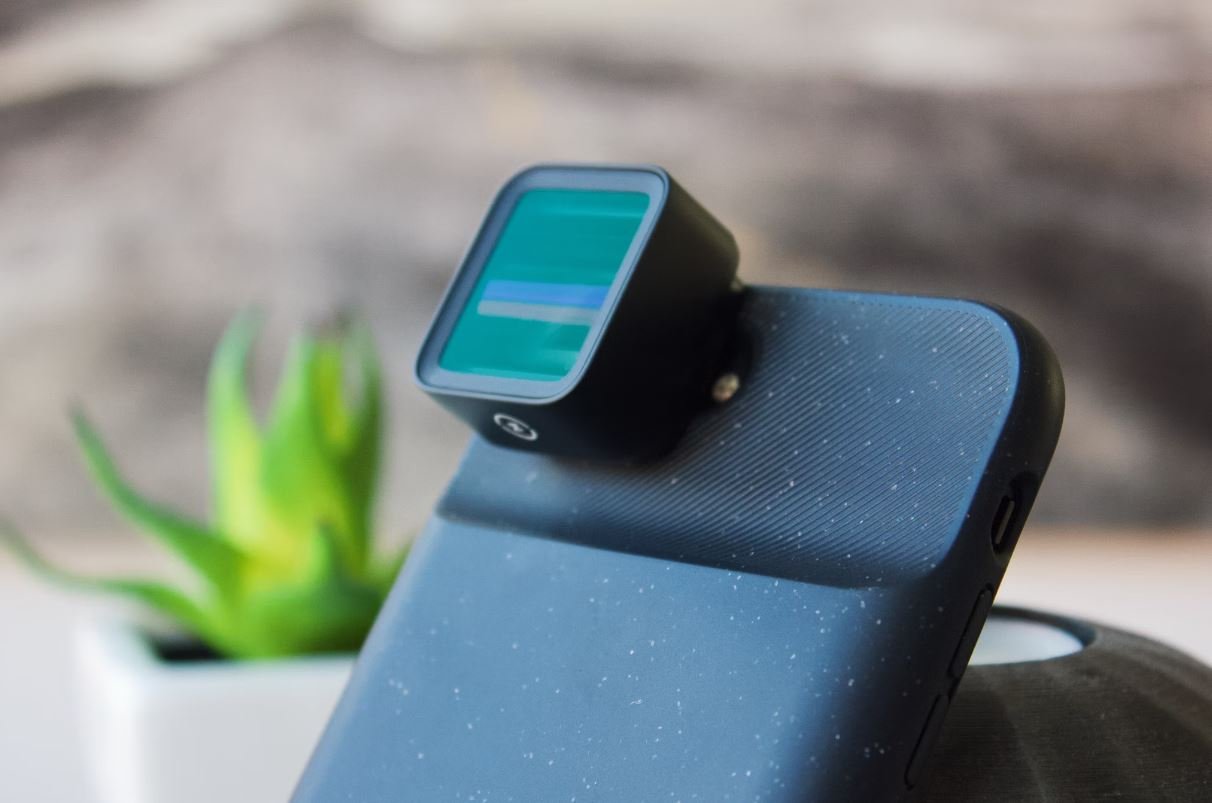
Weather Data by Region
Below is a table presenting average annual rainfall in millimeters for different regions around the globe. This data provides insights into the varying weather patterns across the world.
Region | Average Rainfall (mm) |
---|---|
Africa | 744 |
Asia | 1322 |
Europe | 660 |
North America | 616 |
South America | 1693 |
Weather Patterns: March to May
The following table describes the average temperature range (°C) and the predominant weather condition for the months of March, April, and May. These patterns can help predict weather trends during the spring season.
Months | Average Temperature Range (°C) | Predominant Weather Condition |
---|---|---|
March | 5-15 | Variable |
April | 10-20 | Mild |
May | 15-25 | Warm |
Monsoon Season: Monthly Rainfall
Understanding monthly rainfall during the monsoon season is crucial for various sectors. This table displays the average monthly rainfall in millimeters for selected monsoon-affected countries.
Country | June | July | August | September |
---|---|---|---|---|
India | 107 | 199 | 243 | 173 |
Bangladesh | 314 | 460 | 320 | 282 |
Philippines | 315 | 331 | 374 | 393 |
Extreme Temperature Records
Examining extreme temperature records helps identify historical temperature extremes. This table shows the highest and lowest temperatures ever recorded in different cities around the world.
City | Highest Temperature (°C) | Lowest Temperature (°C) |
---|---|---|
Cairo, Egypt | 46 | -2 |
Sydney, Australia | 45 | 2 |
Yakutsk, Russia | 38 | -64 |
Ocean Currents: Temperature and Direction
Studying ocean currents assists in predicting weather patterns. The following table demonstrates the temperature (°C) and dominant direction of notable ocean currents.
Ocean Current | Temperature (°C) | Dominant Direction |
---|---|---|
Gulf Stream | 20 | Northward |
North Atlantic Drift | 10 | Eastward |
Peru Current | 12 | Southward |
Weather-Related Disasters
Weather events can lead to disastrous consequences. This table presents the number of fatalities caused by different types of weather-related disasters.
Disaster Type | Number of Fatalities (1950-2020) |
---|---|
Hurricanes | 267,704 |
Droughts | 1,209,980 |
Floods | 616,393 |
Predicted Annual Rainfall Changes
Climate change affects rainfall patterns. The table below showcases predicted changes in average annual rainfall (mm) for selected cities by the year 2050.
City | Predicted Annual Rainfall – 2050 (mm) |
---|---|
Tokyo, Japan | 2170 |
Mexico City, Mexico | 1060 |
London, UK | 860 |
Weather Forecast Accuracy
Predicting weather accurately is essential. This table presents the accuracy of weather forecasts for different time frames.
Forecast Time Frame | Accuracy |
---|---|
24 hours | 85% |
5 days | 75% |
10 days | 65% |
Conclusion
The implementation of machine learning for weather prediction is highly beneficial due to its ability to process vast amounts of data and identify patterns. Through studying weather patterns by region, understanding seasonal changes, analyzing extreme temperature records, examining ocean currents, and predicting future climate scenarios, machine learning can enhance the accuracy of weather forecasts. Moreover, this technology contributes to improved disaster preparedness and provides valuable insights for various industries impacted by weather conditions. As we continue to advance in the field of machine learning, our ability to predict and comprehend the complexities of weather patterns will continually evolve, ensuring a safer and more informed future.
Frequently Asked Questions
How does machine learning help in predicting weather?
Machine learning algorithms analyze historical weather data and identify patterns or trends to make accurate predictions about future weather conditions.
What kind of data is used for training machine learning models in weather prediction?
Various types of weather data such as temperature, humidity, wind speed, atmospheric pressure, and precipitation are used to train machine learning models for weather prediction.
Which machine learning algorithms are commonly used in weather prediction?
Some commonly used machine learning algorithms in weather prediction include decision trees, random forests, support vector machines (SVM), and artificial neural networks (ANN).
Can machine learning accurately predict long-term weather forecasts?
While machine learning can provide reasonably accurate short-term weather predictions, long-term forecasts beyond a few weeks are more challenging due to the complex nature of weather patterns.
What factors influence the accuracy of machine learning-based weather predictions?
The accuracy of machine learning-based weather predictions is influenced by factors such as the quality and quantity of training data, the choice of features, the algorithm used, and the availability of real-time data for continuous model updates.
How are machine learning models validated in weather prediction?
Machine learning models for weather prediction are typically validated using historical weather data by comparing the predicted values with the actual observed values. Statistical measures such as mean absolute error (MAE) and root mean square error (RMSE) are commonly used to assess the model’s accuracy.
Can machine learning predict extreme weather events such as hurricanes and tornadoes?
Machine learning models can provide insights and predictions about the probability or intensity of extreme weather events by analyzing relevant data. However, the accuracy of such predictions depends on the availability of high-quality data and the complexity of the event being predicted.
Can machine learning models adapt to changing climate patterns?
Machine learning models can be trained on historical climate data to identify and adapt to changing patterns, allowing them to provide potentially accurate predictions for future climate changes.
Are machine learning-based weather predictions always correct?
No, machine learning-based weather predictions are not always correct. Weather prediction involves inherent uncertainties, and the accuracy of predictions can vary based on various factors, including the complexity of weather patterns and the quality of data used.
How can machine learning improve weather forecasting in the future?
Machine learning can help improve weather forecasting in the future by incorporating more comprehensive and diverse datasets, facilitating real-time data processing, enhancing prediction models with advanced algorithms, and continuous refinement through feedback loops with meteorological experts.
“`