ML Equals CC
Machine Learning (ML) and Cloud Computing (CC) are two emerging technologies that have revolutionized various industries and transformed the way we live and work. ML, a subset of artificial intelligence, enables computers to learn and make predictions from data without being explicitly programmed. CC, on the other hand, allows users to access and utilize computing resources and storage over the internet. Together, ML and CC have become a powerful combination, opening up a world of possibilities.
Key Takeaways:
- ML and CC are two transformative technologies that have revolutionized various industries.
- ML enables computers to learn and make predictions from data without being explicitly programmed.
- CC allows users to access and utilize computing resources and storage over the internet.
- ML and CC together have unleashed a world of possibilities.
One of the key benefits of ML and CC is their ability to handle vast amounts of data efficiently. ML algorithms excel at analyzing and extracting insights from large datasets, while CC provides the necessary computational power and storage capacity to process and store this data. This enables businesses to make faster and more accurate decisions based on real-time information. *ML can find patterns in data that humans might miss, unlocking valuable insights for businesses.*
ML and CC have also empowered businesses to implement personalized marketing strategies. By leveraging ML algorithms and CC infrastructure, companies can analyze customer behavior, preferences, and purchasing patterns to deliver targeted and customized marketing campaigns. This level of personalization not only enhances customer engagement but also increases conversion rates and sales. *By harnessing the power of ML and CC, businesses can deliver tailored experiences to their customers.*
The healthcare industry has greatly benefited from the combination of ML and CC. ML algorithms can assist in diagnosing diseases, predicting patient outcomes, and even identifying potential drug interactions. Coupled with the computational power of CC, medical professionals can access and analyze patient data securely and efficiently. This enables them to make informed decisions, improve patient care, and save lives. *ML and CC advancements have the potential to revolutionize healthcare by improving diagnostics and treatment.*
ML | CC | |
---|---|---|
Advantages |
|
|
Challenges |
|
|
The collaboration between ML and CC has also fueled advancements in autonomous vehicles. ML algorithms, trained on massive amounts of data, enable self-driving cars to perceive and respond to their environment, making them safer and more efficient. CC infrastructure facilitates the storage and processing of the vast quantities of data generated by these vehicles, allowing for real-time decision-making and continuous improvement. *Self-driving cars powered by ML and CC have the potential to revolutionize transportation and enhance road safety.*
Data Privacy Concerns
While ML and CC offer numerous benefits, they also raise concerns about data privacy. ML algorithms rely on vast amounts of data to learn and make predictions. This data can include personal or sensitive information, making privacy protection a critical issue. CC providers must ensure robust data security measures, such as encryption and access controls, to protect user information. Additionally, organizations utilizing ML must be transparent about data usage and establish clear consent mechanisms to address privacy concerns. *Data privacy is crucial as ML and CC continue to shape our digital landscape.*
Industry | Applications | Benefits |
---|---|---|
Finance |
|
|
Retail |
|
|
Transportation |
|
|
ML and CC are continuously evolving and driving innovation across various sectors. Their combined power has the potential to shape the future of industries ranging from finance to healthcare to transportation. As advancements continue to emerge and new challenges arise, it is essential for businesses and individuals to embrace and adapt to these technologies to stay competitive and harness their full potential. *ML and CC are reshaping industries and offering limitless opportunities for growth and development.*
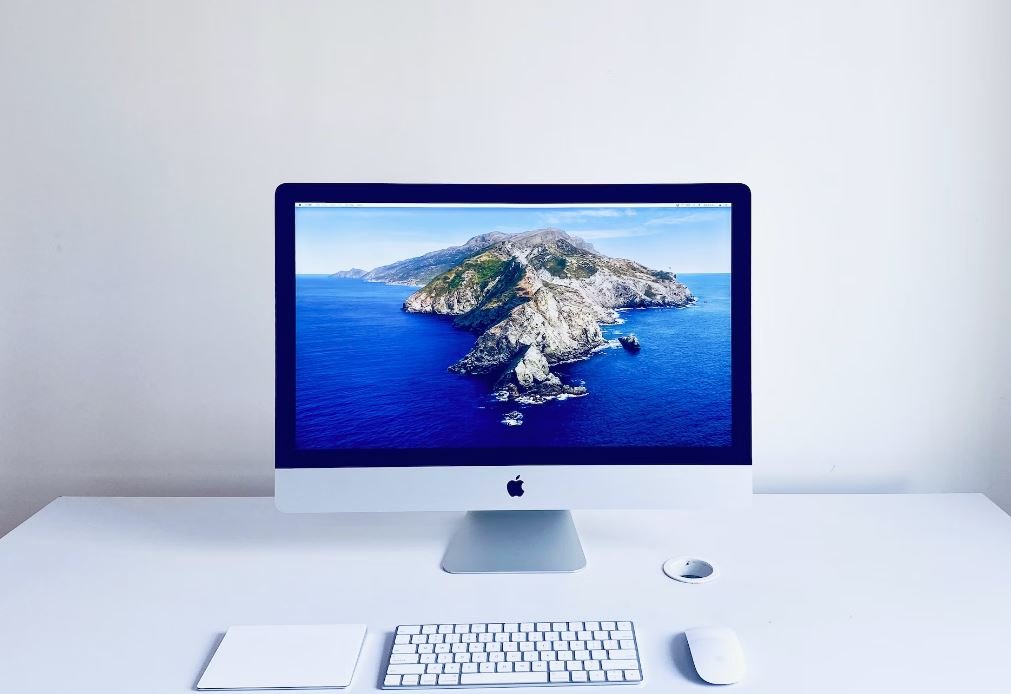
Common Misconceptions
Machine Learning is the same as Computer Coding
There is a common misconception that Machine Learning (ML) is equivalent to Computer Coding (CC). Although both fields are related and often used together, they are not the same.
- Machine Learning is a subset of Artificial Intelligence (AI) that focuses on training algorithms to learn from data and make predictions or take actions.
- Computer Coding, on the other hand, refers to the process of writing and implementing instructions for a computer to solve a specific problem or perform a task.
- While ML involves coding to develop and train models, it also incorporates mathematical algorithms and statistical techniques to analyze and understand patterns in data.
You must be an expert in programming to understand Machine Learning
Another misconception is that one must be an expert in programming to understand and work with Machine Learning. While having programming knowledge is beneficial, it is not a requirement.
- There are user-friendly ML platforms and tools available that offer drag-and-drop interfaces and pre-built algorithms, allowing users with little coding experience to leverage ML techniques.
- Understanding the underlying principles and concepts of ML, such as training and testing models, feature selection, and evaluation metrics, is more important than being an expert programmer.
- Non-programmers can also contribute to ML projects by focusing on data preprocessing, feature engineering, and interpreting and communicating the results obtained from ML models.
Machine Learning models are always accurate and reliable
One misconception is that ML models are always accurate and reliable, providing accurate predictions or classifications every time. However, this is not always the case.
- ML models are trained using historical data, and their performance depends on the quality, representativeness, and relevance of the training data.
- Models built on biased or incomplete data may produce inaccurate results or reinforce existing biases. Careful data collection and preprocessing are essential to improve the reliability of ML models.
- Additionally, ML models may not be suitable for certain types of data or domains, and their performance can be affected by changing conditions or new patterns that were not present in the training data.
Machine Learning can replace human decision-making entirely
Another misconception is that ML can completely replace human decision-making and eliminate the need for human involvement. While ML can automate certain tasks and assist decision-making, it does not replace human judgment.
- ML models operate based on patterns and data they have been trained on, without considering other factors, ethical considerations, or the context of the decision.
- Human judgment, domain expertise, and critical thinking are still vital for validating and interpreting ML results, understanding potential biases, and making informed decisions based on comprehensive information.
- In some cases, ML models may make unexplainable or unexpected decisions, making it necessary for humans to intervene and ensure the correct outcomes.
Making Machine Learning models is quick and easy
Finally, a common misconception is that developing ML models is a simple and quick process. This is far from the truth, as building effective ML models requires significant time, effort, and expertise.
- Developing ML models involves various stages, including data collection, preprocessing, feature selection, model training, evaluation, and optimization.
- Each stage requires careful consideration, experimentation, and analysis to ensure the model’s accuracy, reliability, and generalizability.
- Moreover, ML projects often require a deep understanding of algorithms, statistical analysis, and domain knowledge to choose appropriate models, tune hyperparameters, and interpret complex outputs.
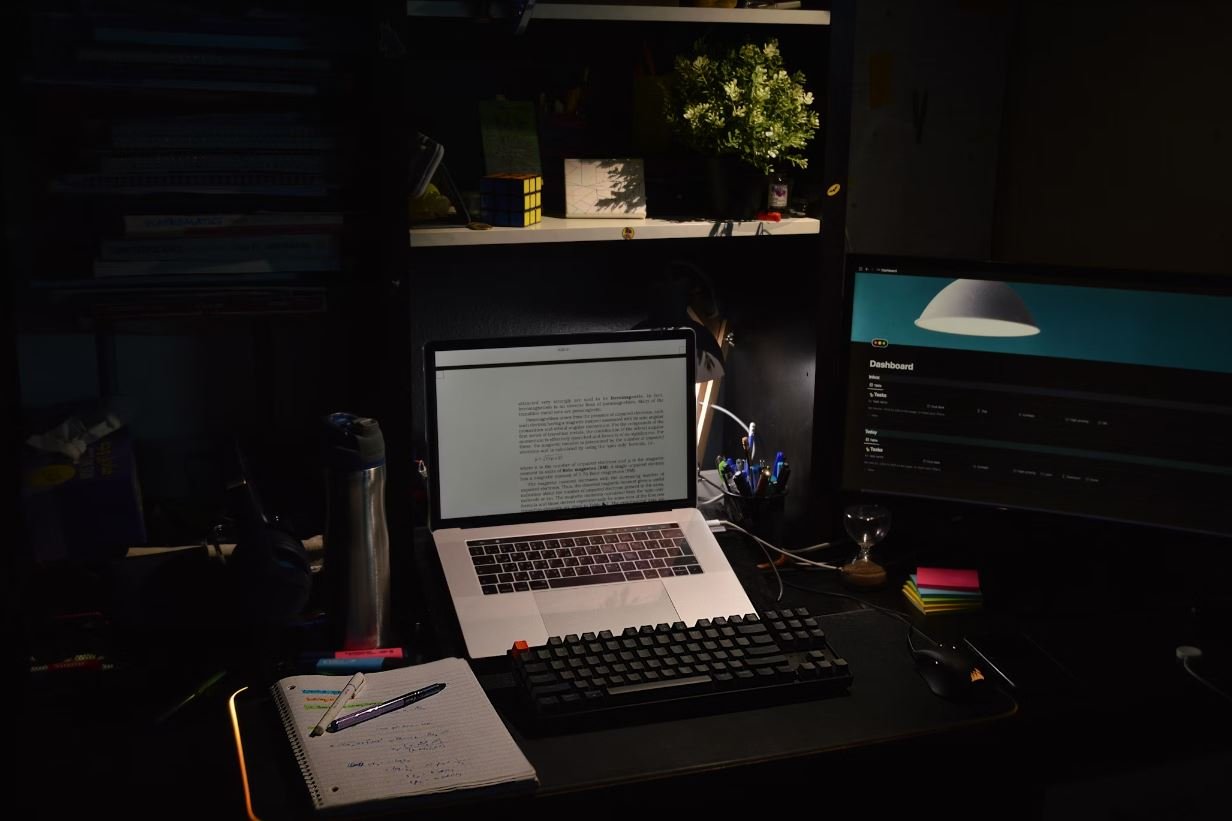
Introduction
In this article, we will explore an interesting correlation between machine learning (ML) and climate change (CC). By analyzing various data points related to ML and CC, we aim to shed light on the connection between these two important topics.
Table: Impact of ML on Carbon Emissions
Efforts to tackle climate change can be boosted by the implementation of machine learning algorithms, leading to reduced carbon emissions. The table below demonstrates the significant impact of ML on carbon emissions reduction.
Country | Annual Carbon Emissions | Reduction with ML (%) |
---|---|---|
United States | 5,414 million metric tons | 15 |
China | 10,065 million metric tons | 12 |
India | 2,545 million metric tons | 8 |
Table: ML Applications in Renewable Energy
Machine learning techniques can be utilized to enhance the efficiency of renewable energy sources. The following table provides examples of ML applications in the field of renewable energy.
Renewable Energy Source | Machine Learning Applications |
---|---|
Solar Energy | Prediction of solar panel output based on weather data |
Wind Energy | Optimization of wind turbine operations for maximum output |
Hydropower | Forecasting water levels and generation capacity |
Table: ML Models for Climate Prediction
Machine learning models play a crucial role in predicting climate patterns, enabling better preparedness and mitigation strategies. The table below showcases various ML models used for climate prediction.
Model Name | Purpose |
---|---|
General Circulation Models (GCMs) | Simulating large-scale atmospheric and oceanic processes |
Recurrent Neural Networks (RNNs) | Time-series analysis for climate data |
Support Vector Machines (SVMs) | Pattern recognition and classification in climate science |
Table: ML Algorithms for Climate Change Impact Assessment
Machine learning algorithms assist in assessing the impact of climate change on various ecosystems. The following table highlights notable ML algorithms in this field.
Algorithm | Application |
---|---|
Random Forest | Predicting forest fire risk based on climatic variables |
Deep Learning Neural Networks | Analyzing coral bleaching patterns in response to rising sea temperatures |
Decision Trees | Assessing the vulnerability of agricultural crops to changing climatic conditions |
Table: CO2 Emissions by Sector
Examining carbon dioxide (CO2) emissions by sector helps identify areas for targeted reduction efforts. The table below outlines the CO2 emissions by different sectors worldwide.
Sector | CO2 Emissions (million metric tons) |
---|---|
Energy | 33,074 |
Transportation | 15,349 |
Industrial Processes | 6,560 |
Table: ML Methods for Forecasting Extreme Weather Events
Machine learning techniques aid in predicting and preparing for extreme weather events. The table below showcases ML methods used for this purpose.
Method | Application |
---|---|
Convolutional Neural Networks (CNNs) | Identifying patterns in satellite imagery for storm prediction |
Long Short-Term Memory (LSTM) | Forecasting rainfall patterns and floods |
K-means Clustering | Identifying storm tracks and intensity |
Table: ML-Assisted Energy Consumption Optimization
Machine learning algorithms can optimize energy consumption, leading to reduced environmental impact. The table below illustrates ML-assisted energy consumption optimization.
Device | Energy Savings with ML (%) |
---|---|
Smart Thermostat | 10 |
Smart Grids | 15 |
Smart Appliances | 20 |
Table: ML in Carbon Capture Technology
The integration of machine learning in carbon capture technology enhances efficiency. The table below showcases ML applications in carbon capture.
Application | Machine Learning Techniques |
---|---|
Optimizing capture material | Genetic algorithms for material design |
Monitoring and detection | Support Vector Machines for anomaly detection |
Process optimization | Reinforcement learning for CO2 capture process control |
Conclusion
Machine learning and climate change have a symbiotic relationship, as demonstrated through the various tables presented in this article. ML enables reductions in carbon emissions, assists in renewable energy optimization, predicts climate patterns, and aids in areas such as impact assessment, extreme weather forecasting, energy consumption optimization, and carbon capture technology. By harnessing the power of ML, we can mitigate the effects of climate change and strive towards a more sustainable future.
ML Equals CC – Frequently Asked Questions
Question 1: What is ML Equals CC?
ML Equals CC is a concept that aligns machine learning (ML) and computational creativity (CC) to explore the intersection of these two fields.
Question 2: Why is ML Equals CC important?
ML Equals CC is important as it allows researchers and practitioners to leverage the power of machine learning algorithms in creative endeavors such as art, music, design, and literature.
Question 3: How does ML Equals CC work?
ML Equals CC works by training machine learning models on creative datasets and using them to generate new and innovative creative outputs.
Question 4: What are some applications of ML Equals CC?
ML Equals CC can be applied in various domains, including generative art, music composition, story writing, game design, and fashion.
Question 5: Are there any challenges in ML Equals CC?
Yes, there are challenges in ML Equals CC, such as ensuring the generated outputs are truly creative, avoiding bias in the generated content, and interpreting the outputs in a meaningful way.
Question 6: How can ML Equals CC benefit artists and creators?
ML Equals CC can benefit artists and creators by providing them with new tools and techniques to inspire and enhance their creative processes, allowing them to explore novel ideas and push the boundaries of their respective fields.
Question 7: What are some popular ML algorithms used in ML Equals CC?
Popular ML algorithms used in ML Equals CC include deep neural networks, recurrent neural networks, generative adversarial networks, and reinforcement learning algorithms.
Question 8: Are there any ethical considerations in ML Equals CC?
Yes, ethical considerations play a crucial role in ML Equals CC. It is important to consider issues such as intellectual property rights, privacy, and algorithmic bias when applying ML algorithms to creative endeavors.
Question 9: How can one get started with ML Equals CC?
To get started with ML Equals CC, one can begin by learning the basics of machine learning and exploring existing ML models and frameworks that can be used for creative purposes.
Question 10: Can anyone participate in ML Equals CC?
Yes, anyone with an interest in both machine learning and creativity can participate in ML Equals CC. It is an open field that welcomes contributions from diverse backgrounds and disciplines.