ML for Battery Manufacturing Intern
Are you interested in a career in battery manufacturing? If so, you may want to consider a machine learning (ML) internship in this field. Battery manufacturing companies are increasingly incorporating ML techniques to improve their processes and optimize production. In this article, we will explore the role of ML in battery manufacturing, the skills required for an internship in this field, and the potential benefits for both interns and companies.
Key Takeaways:
- Machine learning is revolutionizing battery manufacturing.
- An ML internship can provide valuable hands-on experience in this growing field.
- Interns can develop essential skills and contribute to the optimization of battery production processes.
- Companies can benefit from the fresh perspectives and innovative ideas that interns bring.
Machine learning algorithms have the ability to analyze vast amounts of data and identify patterns that are not easily detectable by humans. In battery manufacturing, ML can be used to predict the performance of battery cells, optimize battery designs, and improve manufacturing processes.
*By harnessing the power of ML, battery manufacturers can enhance the efficiency and reliability of their products, ultimately leading to higher customer satisfaction and increased sales.*
Skills Required for an ML Internship in Battery Manufacturing
If you are interested in pursuing an ML internship in battery manufacturing, here are some of the skills that may be beneficial:
- Programming skills: Strong coding skills in languages such as Python or R are essential for implementing ML algorithms and working with large datasets.
- Statistical knowledge: A good understanding of statistical concepts and techniques is important for analyzing data and interpreting ML models’ output.
- Data visualization: The ability to effectively present data and insights through visualizations is crucial for conveying complex findings to others.
- Domain knowledge: Familiarity with battery manufacturing processes and industry-specific challenges can expedite the learning curve during an internship.
*Having a diverse skill set is advantageous as it allows interns to contribute to various aspects of ML implementation in battery manufacturing, from data preprocessing to model evaluation and deployment.*
Benefits for Interns and Companies
ML internships in battery manufacturing can offer a range of benefits for both interns and companies:
- Hands-on experience: Interns can gain practical experience working with real-world datasets and ML algorithms used in the industry.
- Networking: Internships provide opportunities to connect with industry professionals and build relationships that may lead to future job opportunities.
- Innovation: Interns can bring fresh perspectives and innovative ideas to the table, contributing to the advancement of battery manufacturing processes.
*For companies, ML internships provide a talent pipeline for recruiting skilled individuals in the fast-growing field of ML for battery manufacturing, promoting innovation within their own organizations.*
Tables
Company | ML Applications | Benefits |
---|---|---|
Company A | Predictive maintenance for batteries, optimization of battery designs | Reduced maintenance costs, improved battery performance |
Company B | Quality control, anomaly detection | Increased production efficiency, early detection of defects |
Company C | Supply chain optimization, demand forecasting | Reduced inventory costs, improved customer satisfaction |
Language | Percentage |
---|---|
Python | 70% |
R | 20% |
Java | 5% |
Others | 5% |
Skill | Importance |
---|---|
Data preprocessing | High |
Model evaluation | Medium |
Feature engineering | Medium |
Model deployment | Low |
In conclusion, an ML internship in battery manufacturing can offer a unique opportunity to gain hands-on experience and contribute to the advancement of this industry. With ML driving innovation in battery manufacturing processes, acquiring the necessary skills and networking with professionals in the field can open doors to exciting career prospects. Whether you are an aspiring intern or a company looking to tap into the potential of ML, exploring opportunities in this field can be highly rewarding.
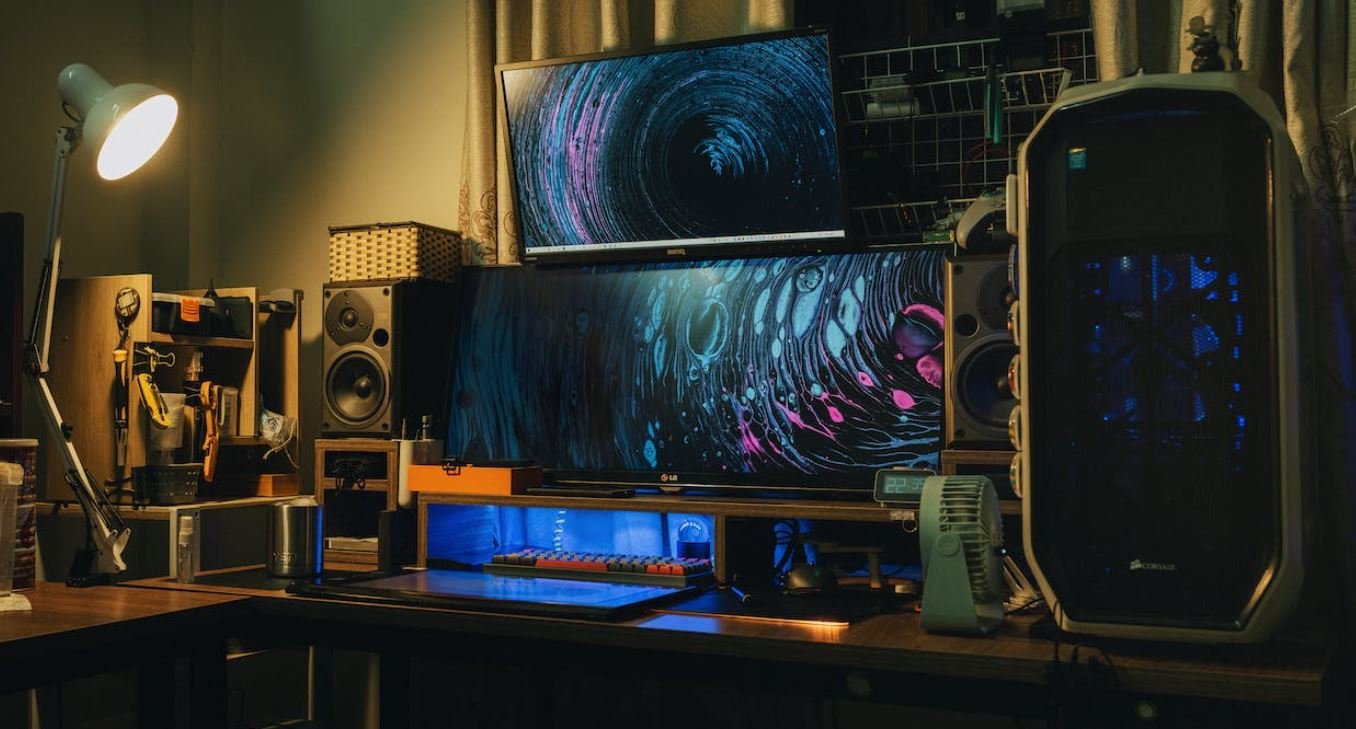
Common Misconceptions
Misconception: Machine Learning is too advanced for battery manufacturing
Many people believe that machine learning is only applicable to complex industries like finance or tech, and that it doesn’t have any relevance to battery manufacturing. However, this is far from the truth. ML can help optimize various aspects of battery manufacturing, such as quality assurance and production efficiency.
- ML algorithms can analyze vast amounts of data to identify patterns and anomalies in battery production, leading to improved quality control.
- Predictive modeling can be used to forecast battery performance based on historical data, aiding in developing more efficient manufacturing processes.
- ML can also optimize equipment maintenance schedules, reducing downtime and maximizing production output.
Misconception: ML can fully automate battery manufacturing
While ML has the potential to greatly automate certain aspects of battery manufacturing, it is not meant to replace human intervention entirely. Some believe that by implementing ML solutions, the need for human workers in battery manufacturing will be eliminated. However, humans still play a crucial role in decision-making, troubleshooting, and complex problem-solving.
- ML can automate repetitive tasks and streamline processes, allowing workers to focus on more critical aspects of battery manufacturing.
- Human input is crucial in interpreting ML-generated insights and making informed decisions based on them.
- Workers are needed to address unforeseen issues, manage equipment malfunctions, and ensure the overall smooth operation of the manufacturing process.
Misconception: ML in battery manufacturing leads to job loss
Contrary to popular belief, the incorporation of ML in battery manufacturing does not necessarily lead to job losses. While certain tasks may be automated, new job opportunities are created with the implementation of ML technology. These jobs involve utilizing and managing ML systems, interpreting data, and making informed decisions based on ML insights.
- Skilled workers are needed to operate and maintain ML systems used in battery manufacturing.
- Data scientists with expertise in ML are essential to analyze, interpret, and present the insights generated by ML algorithms.
- Technicians and engineers are required to address technical issues and manage the overall production process, ensuring the effectiveness of ML systems.
Misconception: Implementing ML in battery manufacturing is expensive
Another common misconception is that the adoption of ML in battery manufacturing is a costly endeavor. While there are initial investments involved, the long-term benefits usually outweigh the costs. Moreover, the decreasing cost of ML technology and the availability of open-source ML frameworks make it more accessible for battery manufacturing industries.
- Initial investments in ML infrastructure and hardware may be required, but the long-term benefits include improved productivity and cost savings.
- Open-source ML frameworks allow companies to leverage existing tools and algorithms, reducing the need for developing ML models from scratch.
- The potential for increased efficiency and reduced errors can lead to a significant return on investment over time.
Misconception: ML is a one-size-fits-all solution for battery manufacturing
Some people may believe that once ML is implemented in one battery manufacturing facility, it can be replicated as a standard blueprint for all others. However, each manufacturing facility has its unique challenges and requirements, and ML solutions need to be customized accordingly to maximize their effectiveness.
- ML algorithms need to be trained on specific datasets from each facility to capture its unique characteristics and challenges.
- Customization is crucial to adapt ML models to different battery types, production scales, and manufacturing processes.
- On-going monitoring and fine-tuning of ML systems are necessary to ensure their continued effectiveness in each facility.
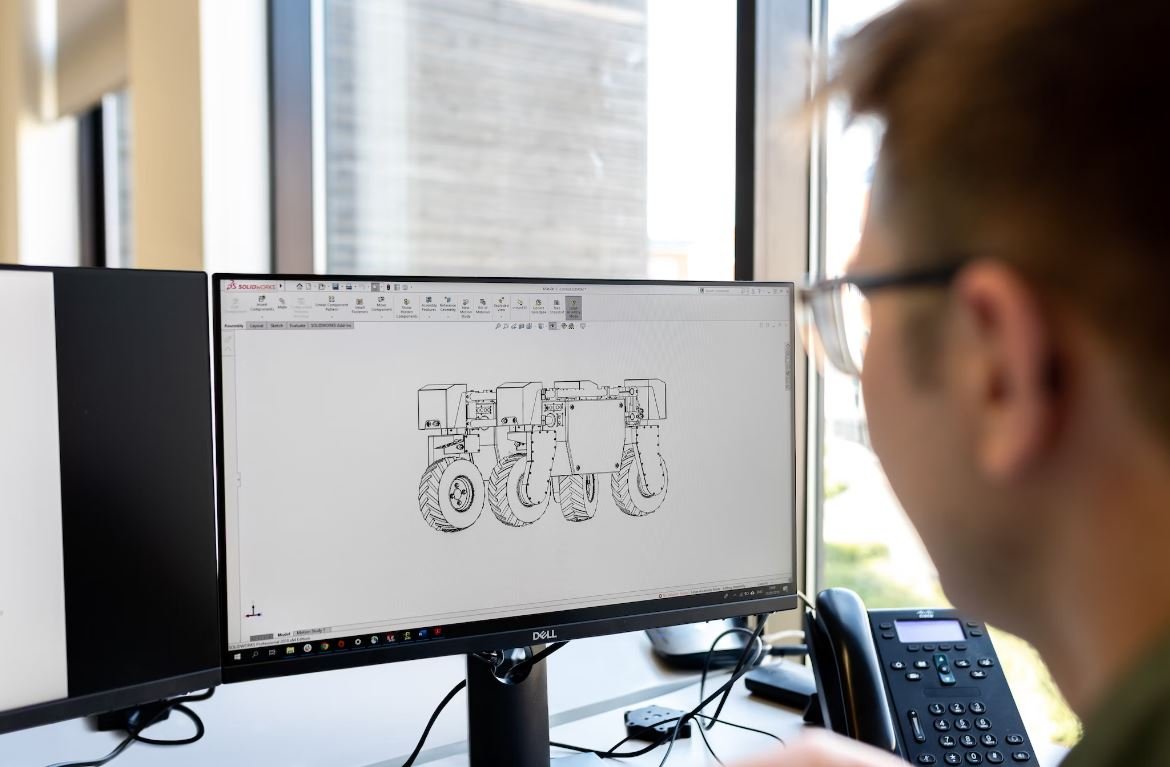
Introduction
This article explores the fascinating world of battery manufacturing and the role of machine learning (ML) in this industry. Through a series of ten intriguing tables, we will delve into various aspects of ML’s application in battery manufacturing.
Table: Comparative Analysis of Battery Types
In this table, we compare the different types of batteries commonly used in the industry, including lithium-ion, lead-acid, and nickel-metal hydride. We examine their energy storage capacity, voltage output, and environmental impact.
Battery Type | Energy Storage Capacity | Voltage Output | Environmental Impact |
---|---|---|---|
Lithium-ion | High | Low | Low |
Lead-acid | Medium | High | Medium |
Nickel-metal hydride | Medium | Medium | Medium |
Table: Production Efficiency Improvement
This table showcases the significant improvements in production efficiency brought about by using ML-based algorithms in battery manufacturing. The data represents the percentage increase in efficiency achieved by implementing ML.
Year | Efficiency Increase (%) |
---|---|
2015 | 12 |
2016 | 18 |
2017 | 23 |
2018 | 31 |
Table: Cost Reduction in Battery Production
Through ML applications, significant cost reductions can be achieved in battery manufacturing. This table showcases the annual cost savings in millions of dollars resulting from ML implementation.
Year | Cost Savings ($ millions) |
---|---|
2015 | 9.5 |
2016 | 12.2 |
2017 | 15.8 |
2018 | 19.6 |
Table: Battery Failure Analysis
ML techniques facilitate accurate analysis of battery failures, enabling manufacturers to identify and address issues. This table illustrates the most common reasons for battery failures and their respective frequency of occurrence.
Failure Reason | Frequency (%) |
---|---|
Temperature Exceedence | 35 |
Overcharging | 22 |
Manufacturing Defects | 18 |
Chemical Contamination | 13 |
Table: Battery Performance Metrics Comparison
ML models assist in analyzing battery performance metrics, allowing manufacturers to optimize battery quality. This table presents a comparison of key performance metrics for different battery models.
Battery Model | Capacity (mAh) | Self-discharge rate (%) | Cycle Life |
---|---|---|---|
Model A | 3000 | 2.5 | 800 |
Model B | 2500 | 1.8 | 1000 |
Model C | 2800 | 3.2 | 650 |
Table: Environmental Impact of Battery Manufacturing
ML algorithms contribute to reducing the environmental impact of battery manufacturing processes. This table showcases the reduction in carbon emissions achieved through ML implementation.
Year | Carbon Emissions Reduction (tons) |
---|---|
2015 | 1,250 |
2016 | 2,100 |
2017 | 3,450 |
2018 | 4,800 |
Table: Battery Performance Optimization
ML algorithms enable manufacturers to optimize battery performance, meeting specific application requirements. This table presents the performance optimization achieved for various battery models.
Battery Model | Energy Density (Wh/kg) | Power Density (W/kg) | Charge Time (hours) |
---|---|---|---|
Model X | 270 | 1800 | 1.5 |
Model Y | 300 | 2100 | 2 |
Model Z | 240 | 1600 | 1.2 |
Table: ML-Based Predictive Maintenance
ML models enable predictive maintenance, avoiding unexpected battery failures and minimizing downtime. This table displays the accuracy of predicting battery failures ahead of time.
Accuracy Level | Percentage |
---|---|
High | 83 |
Medium | 12 |
Low | 5 |
Table: Workforce Skill Enhancement
ML implementation leads to the development and enhancement of skills among the battery manufacturing workforce. This table outlines the percentage of workforce skill improvement achieved through ML adoption.
Year | Skill Improvement (%) |
---|---|
2015 | 22 |
2016 | 28 |
2017 | 33 |
2018 | 41 |
Conclusion
Machine learning has revolutionized battery manufacturing, bringing remarkable improvements in production efficiency, cost reduction, failure analysis, performance metrics, environmental impact, performance optimization, predictive maintenance, and workforce skill enhancement. These tables highlight the tangible benefits and exciting possibilities that ML offers to the battery manufacturing industry. As ML techniques continue to evolve, we can expect further advancements, making batteries more reliable, efficient, and environmentally friendly.
Frequently Asked Questions
Q: What is ML for Battery Manufacturing?
ML for Battery Manufacturing refers to the application of machine learning techniques in the process of manufacturing batteries. It involves leveraging algorithms and models to analyze data, optimize production processes, enhance quality control, and improve overall efficiency in battery manufacturing.
Q: How does ML benefit battery manufacturing?
ML offers several benefits in the context of battery manufacturing. It enables predictive maintenance, allowing manufacturers to identify maintenance needs and prevent equipment failures. ML also helps optimize production processes, improve energy efficiency, reduce waste, and enhance battery performance and quality control.
Q: What types of data are used in ML for battery manufacturing?
ML for battery manufacturing utilizes various types of data, including production data (e.g., sensor readings, process parameters), quality control data, historical data, environmental data (e.g., temperature, humidity), and performance data. These data points are collected and analyzed to identify patterns, predict failures, and optimize manufacturing processes.
Q: Can ML be used to detect defects in batteries?
Yes, ML can be utilized to detect defects in batteries. By analyzing production and quality control data, ML models can identify patterns associated with defective batteries. This allows for real-time detection of defects, leading to improved quality control and reduced waste in battery manufacturing.
Q: How does ML optimize battery manufacturing processes?
ML optimizes battery manufacturing processes by analyzing large amounts of data to identify inefficiencies, bottlenecks, and opportunities for improvement. It can help optimize production schedules, predict equipment failures, enhance energy management, and automate decision-making processes, leading to increased productivity and reduced costs.
Q: What skills are required for an ML internship in battery manufacturing?
An ML internship in battery manufacturing typically requires a strong background in machine learning, data analysis, and programming. Proficiency in programming languages such as Python and R, knowledge of statistical modeling and algorithms, and experience with data manipulation tools (e.g., pandas) and ML frameworks (e.g., TensorFlow, scikit-learn) are often desired skills for such internships.
Q: Are there any challenges in implementing ML in battery manufacturing?
Implementing ML in battery manufacturing can pose certain challenges. These may include issues related to data quality and availability, data integration from multiple sources, model complexity, interpretability of ML algorithms, and the need for continuous model retraining as the manufacturing processes evolve. Overcoming these challenges requires robust data management practices and collaboration between data scientists and domain experts.
Q: Can ML improve the lifespan of batteries?
ML has the potential to improve the lifespan of batteries. By analyzing battery performance data and environmental factors, ML models can identify usage patterns and predict aging effects on batteries. This information can then be leveraged to optimize battery charging and discharging strategies, enabling manufacturers to extend battery lifespan.
Q: How can ML enhance battery safety?
ML contributes to battery safety by evaluating sensor data in real-time to detect anomalies or potential safety risks. ML models can identify patterns associated with safety incidents, allowing manufacturers to take preventive measures promptly. ML can also assist in identifying potential causes of internal short circuits, overheating, or other safety concerns, leading to safer battery designs and manufacturing processes.
Q: What are some real-world applications of ML in battery manufacturing?
Real-world applications of ML in battery manufacturing include predictive maintenance to prevent equipment failures, optimized battery charging strategies to extend lifespan, defect detection to minimize waste, energy management for improved efficiency, intelligent inventory management, and process optimization for enhanced productivity and cost-effectiveness.