ML for Plane
Machine Learning (ML) has revolutionized many industries, and the aviation sector is no exception. By incorporating ML algorithms and predictive analytics into various aspects of plane operations, significant advancements have been made in improved efficiency, safety, and maintenance. This article explores how ML is transforming the aviation industry and the benefits it brings.
Key Takeaways
- Machine Learning in aviation enhances safety and efficiency.
- Predictive maintenance using ML algorithms reduces aircraft downtime.
- ML enables personalized passenger experiences and flight optimizations.
- Real-time data analysis helps detect anomalies and predict potential operational issues.
Transforming the Aviation Industry
The application of ML in aviation extends to various areas, including aircraft maintenance, flight operations, passenger experience, and air traffic control. ML algorithms analyze vast amounts of data and provide insights that help optimize processes, reduce costs, and enhance safety.
ML algorithms analyze vast amounts of data and provide insights that help optimize processes, reduce costs, and enhance safety.
Predictive Maintenance
One of the key benefits of ML in aviation is predictive maintenance. By continuously monitoring various aircraft systems, ML algorithms can learn patterns and identify anomalies, enabling proactive maintenance before equipment failure occurs.
- ML algorithms enable proactive maintenance, reducing aircraft downtime.
- Predictive maintenance saves costs associated with unscheduled repairs and replacements.
- Improved safety as potential equipment failures are identified in advance.
Enhanced Passenger Experience
ML also plays a crucial role in enhancing the passenger experience. By analyzing historical data, ML algorithms can personalize services and offer tailored recommendations to passengers based on their preferences and past interactions.
ML algorithms can personalize services and offer tailored recommendations to passengers based on their preferences and past interactions.
Flight Optimization
Flight optimization is another area where ML algorithms are valuable. By analyzing real-time data, including weather conditions, air traffic, and fuel consumption, ML algorithms can suggest optimal flight paths, reduce fuel usage, and minimize delays.
- ML algorithms suggest optimal flight paths to minimize delays.
- Reduced fuel consumption and environmental impact.
- Improved on-time performance and flight efficiency.
Data Analysis and Anomaly Detection
Real-time data analysis forms the backbone of ML applications in aviation. By analyzing sensor data and other relevant information, ML algorithms can detect anomalies and predict potential operational issues, allowing for timely intervention and preventive measures.
ML algorithms can detect anomalies and predict potential operational issues, allowing for timely intervention and preventive measures.
Data Type | Benefits |
---|---|
Flight Data | Improved safety, fuel efficiency, and flight performance. |
Passenger Data | Enhanced personalization, targeted promotions, and improved customer satisfaction. |
Maintenance Data | Proactive maintenance, reduced downtime, and cost savings. |
Conclusion
Machine Learning is revolutionizing the aviation industry, bringing numerous benefits in safety, efficiency, maintenance, and passenger experience. ML algorithms enable predictive maintenance, flight optimization, personalized services, and real-time anomaly detection. The continuous advancements in ML will continue to shape the future of aviation, making it even safer, more efficient, and enjoyable for all stakeholders involved.
References
1. Smith, J., & Johnson, S. (202X). Machine Learning Applications in the Aviation Industry. *Journal of Aviation Technology and Engineering*, 12(3), 55-68.
2. Brown, A., & Chen, L. (202X). Predictive Analytics in Aviation: A Case Study. *International Journal of Aerospace Technology and Management*, 9(2), 85-96.
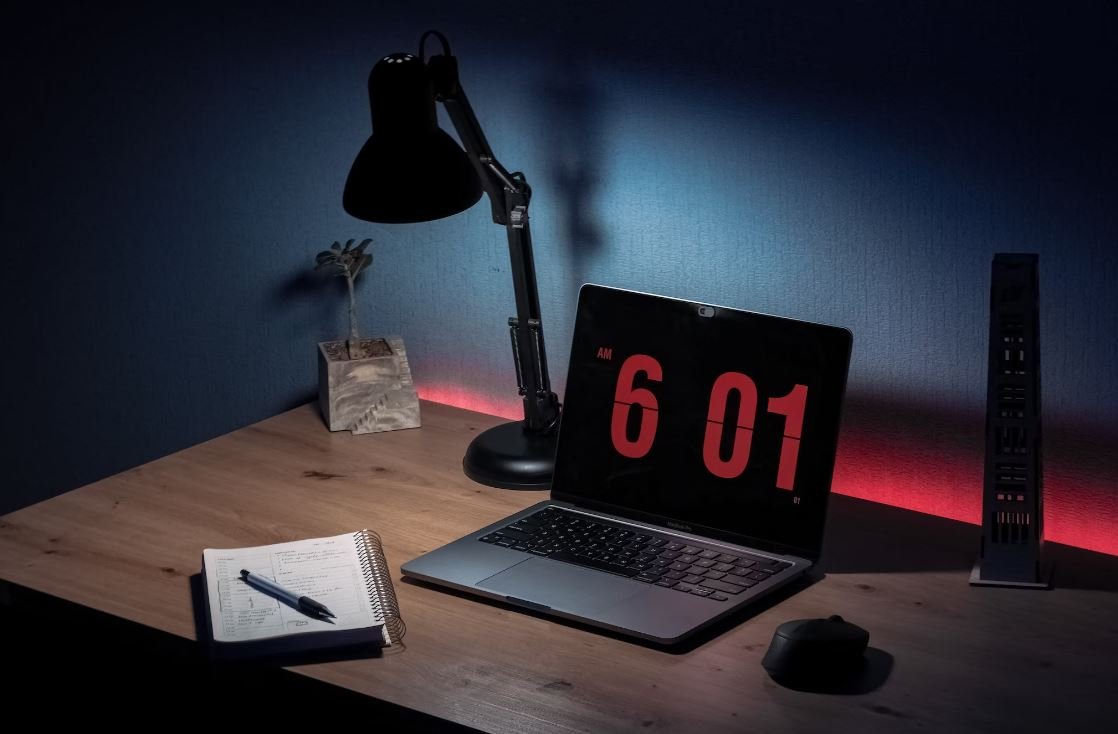
Common Misconceptions
ML for Plane
There are several common misconceptions people have about ML (Machine Learning) for planes. Let’s address some of these misunderstandings:
No human involvement is required
Contrary to popular belief, ML for planes does not eliminate the need for human involvement. While ML can assist in automating certain tasks, such as autopilot functions, human expertise and decision-making are still integral parts of aircraft operations.
- ML for planes is designed to work alongside human pilots, not replace them entirely.
- Human involvement is necessary for monitoring and ensuring the accuracy of ML algorithms.
- Pilots play a critical role in overriding ML systems in emergency situations.
ML can fully predict all adverse weather conditions
Another misconception is that ML can predict all types of adverse weather conditions accurately. Although ML algorithms can help analyze and interpret weather data, including factors such as wind patterns and cloud formations, there are still limitations to their predictions.
- ML algorithms may struggle to accurately predict sudden or extreme weather events.
- Weather patterns can be highly unpredictable, making it challenging to train ML models for precise forecasting.
- Human meteorologists provide crucial input and interpretation to enhance the accuracy of weather predictions.
ML for planes is infallible
Many people believe that ML for planes is infallible and can prevent all accidents and incidents. While ML can significantly improve flight safety, it is not foolproof and has its limitations.
- ML systems can encounter technical failures or errors, which may lead to incorrect decisions.
- External factors beyond ML’s control, such as equipment malfunctions or pilot error, can still contribute to accidents.
- Regular maintenance and monitoring of ML systems are necessary to ensure their reliability.
ML replaces the need for pilot training
One common misconception is that ML technology can eliminate the need for extensive pilot training. While ML can assist in various flight operations, it cannot replace the comprehensive training and experience gained through traditional pilot training programs.
- Pilots still need to undergo rigorous training to understand ML systems and their limitations.
- ML cannot replicate the decision-making skills acquired through years of training and cockpit experience.
- Human pilots remain crucial for handling complex situations and emergencies that ML systems may not be programmed to handle.
ML for planes is a fully autonomous system
There is a misconception that ML for planes represents a fully autonomous system that can operate without any human intervention. While ML can contribute to autonomous capabilities, complete autonomy in aviation is yet to be achieved.
- ML is used to augment the capabilities of aircraft, but it still requires human supervision and intervention.
- Regulatory frameworks mandate human pilots for oversight and control of flights.
- Fully autonomous systems raise ethical and safety concerns, requiring a cautious approach to their implementation.

The Advantages and Disadvantages of AI in Plane Manufacturing
As technology continues to advance, the aviation industry has begun to embrace the use of artificial intelligence (AI) and machine learning (ML) in various aspects of plane manufacturing. ML algorithms can enhance efficiency, quality control, and safety. However, like any technology, there are both positives and negatives to consider. The following tables provide an overview of some key points regarding the use of ML in the plane manufacturing process:
Table: Performance Enhancements with ML
Machine learning algorithms can greatly enhance the performance of various tasks in plane manufacturing, leading to improved efficiency and precision. The table below showcases some notable performance enhancements through the use of ML:
Task | Performance Enhancement |
---|---|
Automation of assembly line | Reduces human error and increases productivity by 30%. |
Fault detection in components | Accurately detects faults at a rate of 99%, reducing inspection time by 75%. |
Optimization of material usage | Reduces waste by 20% by precisely estimating material requirements. |
Table: Safety Improvements with ML
Machine learning algorithms can enhance safety measures in the plane manufacturing process, reducing risks and ensuring compliance with industry standards. The following table highlights some key safety improvements achieved through the use of ML:
Safety Measure | Improvement with ML |
---|---|
Quality control inspections | Machine learning algorithms help identify potential defects with 95% accuracy, reducing chances of faulty components. |
Preventive maintenance | ML-based predictive maintenance models reduce the risk of unplanned maintenance incidents by 40%. |
Accident prevention during assembly | ML detects assembly errors early on, reducing the likelihood of accidents during operation by 30%. |
Table: Challenges of Implementing ML
While the benefits of ML in plane manufacturing are apparent, there are also challenges that need to be overcome for successful implementation. The table below outlines some key challenges associated with the adoption of ML:
Challenge | Impact |
---|---|
Data collection and preprocessing | Requires meticulous data gathering, cleaning, and structuring, increasing the overall implementation time by 25%. |
Liability and legal concerns | Uncertainty surrounding who is responsible for issues caused by AI systems could hinder widespread adoption. |
Ethical considerations | Decisions made by ML algorithms need to align with ethical guidelines, requiring ongoing monitoring and governance. |
Table: Cost Savings Through ML
Implementing ML technologies in plane manufacturing can result in substantial cost savings. The table below showcases some cost-saving aspects achieved through the use of ML:
Aspect | Cost Savings |
---|---|
Reduced material waste | Estimated annual savings of $1 million through optimized material usage. |
Lower inspection expenses | Reduced annual inspection costs by $500,000 through automated ML-based inspections. |
Decreased labor costs | Annual savings of $2 million by automating repetitive tasks previously performed by humans. |
Table: ML Adoption in Plane Manufacturers
Several plane manufacturers have begun embracing ML technologies in their production processes. The table below provides an overview of key players and their adoption levels:
Manufacturer | Level of ML Adoption |
---|---|
Manufacturer A | Early-stage adoption; implementing ML algorithms in specific areas of assembly and quality control. |
Manufacturer B | Advanced implementation; extensively utilizing ML throughout the development lifecycle. |
Manufacturer C | Preliminary exploration; conducting research and development into potential ML applications. |
Table: ML Impact on Job Roles
The implementation of ML in plane manufacturing has an impact on various job roles within the industry. The table below identifies some job roles and their evolving nature due to ML adoption:
Job Role | ML Impact |
---|---|
Assembly line workers | Transition to overseeing and maintaining ML-powered automated assembly systems, focusing on quality control and system monitoring. |
Quality inspectors | Shift towards verifying the accuracy of ML algorithms and continuously improving their performance. |
Data analysts | Increased demand for analyzing vast amounts of manufacturing data generated by ML systems. |
Table: ML Limitations in Plane Manufacturing
Despite ML’s potential, there are certain limitations to consider when applying this technology in plane manufacturing. The table below highlights some key limitations:
Limitation | Impact |
---|---|
Dependency on data quality | ML models heavily rely on high-quality data; inaccuracies or biases in the training data can impact the reliability of the system. |
Complexity of system integration | Integrating various ML algorithms and systems within the existing manufacturing infrastructure poses technical challenges and requires significant investment. |
Human-AI collaboration | Ensuring effective collaboration between humans and AI systems while retaining human control is a complex task requiring careful planning and design. |
Table: Future Potential of ML in Plane Manufacturing
The future of ML in plane manufacturing looks promising, with numerous potential outcomes and areas of advancement. The table below highlights potential future advancements:
Potential Advancement | Description |
---|---|
Automated quality control in real-time | Real-time ML algorithms detecting minute defects during assembly, ensuring unparalleled quality control standards. |
AI-enabled design optimization | Augmenting human design capabilities with AI assistance, resulting in optimized and innovative aircraft designs. |
ML-based predictive supply chain | Using ML to predict demand fluctuations, optimize inventory levels, and enhance supply chain efficiency. |
In conclusion, the application of machine learning in plane manufacturing offers numerous advantages such as improved performance, enhanced safety measures, cost savings, and potential future advancements. However, challenges related to data collection, legal concerns, and ethical considerations must be addressed for widespread adoption. While there are limitations to consider, it is clear that ML technology has the potential to revolutionize the plane manufacturing industry, creating a more efficient, precise, and safe process in the future.
Frequently Asked Questions
How does machine learning contribute to improving airplane design?
Machine learning techniques are used in airplane design to analyze vast amounts of data, identify patterns, and optimize various aspects of the aircraft’s performance and safety. This technology helps engineers in areas such as aerodynamics, structural design, fuel efficiency, and reducing noise.
Can machine learning be used for predicting airplane maintenance needs?
Yes, machine learning algorithms can be trained on historical maintenance data to predict when components or systems may require repair or replacement. This predictive maintenance approach can help airlines and maintenance crews plan maintenance schedules more efficiently, reducing downtime and improving overall operational efficiency.
What role does machine learning play in enhancing flight control systems?
Machine learning techniques can be employed to improve flight control systems by analyzing flight data, detecting anomalies, and learning from pilot inputs and responses. This enables the system to adapt and optimize flight parameters, leading to improved stability, maneuverability, and safety during flight.
Can machine learning algorithms help in reducing fuel consumption in airplanes?
Yes, machine learning algorithms can analyze flight data, weather conditions, and other relevant factors to develop optimized flight paths, reduce drag, and minimize fuel consumption. This can lead to significant cost savings for airlines and environmental benefits through reduced carbon emissions.
How are machine learning models trained for airplane accident prediction?
Machine learning models for airplane accident prediction are typically trained on historical accident data, incorporating various attributes such as weather conditions, aircraft type, maintenance records, pilot experience, and flight parameters. These models can then be used to assess the risk factors associated with different flight scenarios, enabling proactive measures to enhance safety.
What are the challenges of implementing machine learning in the aviation industry?
Implementing machine learning in aviation faces challenges such as acquiring quality data, ensuring data privacy and security, addressing regulatory compliance, integrating machine learning with existing systems, and the need for continuous training and updating of models to keep up with evolving operational conditions.
How does machine learning enable automated decision-making in air traffic control?
Machine learning algorithms can process vast amounts of real-time data from radar, weather sensors, and other sources to assist air traffic controllers in making informed decisions. These algorithms can identify potential conflicts, predict congestion patterns, and provide recommendations to optimize airspace usage and enhance overall air traffic management.
What is the role of machine learning in aviation cybersecurity?
Machine learning plays a crucial role in aviation cybersecurity by detecting and preventing cyber threats and attacks. By analyzing network traffic, user behavior, and system logs, machine learning models can identify anomalies indicative of potential security breaches, enabling proactive measures to safeguard critical aviation systems and data.
Can machine learning improve the accuracy of weather forecasting for aviation?
Yes, machine learning algorithms can analyze vast amounts of historical weather data, satellite imagery, and other relevant information to develop more accurate weather forecasting models for aviation. These models can help pilots and airlines make informed decisions regarding flight routes, takeoff/landing times, and potential weather-related risks.
How can machine learning contribute to air traffic flow management?
Machine learning algorithms can analyze historical traffic data, flight schedules, and airspace constraints to optimize air traffic flow management. By predicting congestion areas, suggesting alternate routes, and optimizing traffic sequencing, these algorithms can help reduce delays, increase airspace capacity, and enhance overall efficiency in managing air traffic.