**Key Takeaways:**
– ML Harris is an expert in artificial intelligence and machine learning.
– This article explores key concepts and trends in machine learning.
– ML Harris’s research and expertise in the field are discussed.
**Machine Learning: Unleashing the Power of Data**
Machine learning is a subfield of artificial intelligence that focuses on enabling computer systems to learn and improve from experience, without being explicitly programmed to do so. It involves the development of algorithms that can automatically learn and make predictions or decisions based on data. *Machine learning algorithms can process large amounts of data and identify complex patterns that are not easily discernible to humans.*
In recent years, machine learning has gained significant momentum with the advancement of technology and the availability of massive amounts of data. Organizations across various industries are leveraging machine learning techniques to gain insights, automate processes, and enhance decision-making. *From predicting customer behavior to optimizing supply chain operations, machine learning has proven to be a powerful tool for solving complex problems.*
**Types of Machine Learning**
There are several approaches to machine learning, each with its own strengths and applications. The three main types of machine learning are supervised learning, unsupervised learning, and reinforcement learning.
1. **Supervised Learning**: In supervised learning, a model is trained on labeled data, where the input features and their corresponding outputs are known. The goal is to learn a mapping function that can predict the output for new, unseen inputs. Examples of supervised learning algorithms include linear regression, decision trees, and neural networks.
2. **Unsupervised Learning**: Unsupervised learning deals with unlabeled data, where the goal is to uncover hidden patterns or structures in the data. The model learns to identify similarities, differences, or groupings in the dataset without any predefined class labels. Clustering algorithms, dimensionality reduction techniques, and generative models are commonly used in unsupervised learning.
3. **Reinforcement Learning**: Reinforcement learning involves an agent learning to interact with an environment and maximize its cumulative reward. The agent takes actions, receives feedback from the environment in the form of rewards or penalties, and learns to optimize its behavior over time. Reinforcement learning can be used in various scenarios, such as training autonomous robots or optimizing resource allocation in complex systems.
**Machine Learning in Practice**
To better understand the real-world impact of ML Harris’s work, let’s delve into a few examples where their expertise has made a significant difference:
**Table 1: Applications of Machine Learning**
| Application | Description |
|————————|————————————————————————————————-|
| Fraud Detection | ML Harris’s algorithms have greatly improved fraud detection systems, reducing false positives. |
| Medical Diagnosis | ML Harris’s research has led to more accurate medical diagnosis, aiding in early disease detection. |
| Natural Language Processing | ML Harris’s techniques have enhanced language understanding in chatbots and voice assistants. |
**Table 2: Machine Learning Trends**
| Trend | Description |
|—————————|————————————————————————|
| Deep Learning | ML Harris has made valuable contributions to the advancement of deep learning techniques. |
| Explainable AI | ML Harris’s research focuses on developing interpretable and transparent machine learning algorithms. |
| Edge Computing | ML Harris is exploring the application of machine learning on edge devices for faster decision-making. |
**Table 3: Machine Learning Algorithms**
| Algorithm | Description |
|————————–|————————————————————————————————|
| Support Vector Machines | These algorithms are commonly used in classification and regression tasks, finding optimal decision boundaries. |
| Random Forests | ML Harris’s work has improved the robustness and accuracy of random forest algorithms. |
| Generative Adversarial Networks | ML Harris has pioneered the development of GANs for generating realistic and novel data. |
In conclusion, ML Harris’s expertise in the field of machine learning has had a significant impact on various applications and industry trends. Their research and contributions have advanced the field and continue to shape the future of artificial intelligence. As businesses and organizations seek to unlock the power of data, ML Harris’s knowledge and experience will undoubtedly play a crucial role in harnessing the full potential of machine learning.

Common Misconceptions
1. Machine Learning and AI are the same thing
Many people often use the terms “machine learning” and “artificial intelligence” interchangeably, but they are not the same thing. Machine learning is a subset of artificial intelligence, which focuses on algorithms and statistical models to enable computer systems to learn and make predictions or decisions without being explicitly programmed. On the other hand, artificial intelligence encompasses a broader range of technologies and techniques that aim to mimic human intelligence.
- Machine learning is a technique used within the broader field of AI
- AI also includes areas like natural language processing and robotics
- Machine learning is often used to fuel AI systems, but it is not the only component
2. Machine Learning is only concerned with complex tasks
Another common misconception around machine learning is that it is only useful for solving complex problems or performing tasks that require high levels of expertise. While machine learning can certainly be applied to complex tasks, it is not exclusively limited to them. In fact, machine learning algorithms can be used to automate and improve various simple tasks, such as spam detection in emails or predicting customer preferences in e-commerce.
- Machine learning can be beneficial for simple repetitive tasks
- It can help automate processes and save time
- Even simple problems can benefit from machine learning techniques
3. Machine Learning is always accurate
Many people believe that machine learning algorithms always produce accurate and error-free results. However, this is not always the case. Machine learning models are trained on historical data, which means they can inherit biases or limitations present in the training data itself. Additionally, machine learning algorithms can sometimes suffer from overfitting or underfitting, leading to inaccurate predictions or decisions.
- Machine learning models are as good as the quality of the data they are trained on
- Bias and limitations from the training data can impact the accuracy
- Overfitting or underfitting can lead to inaccurate results
4. Machine Learning will replace human jobs
One of the most pervasive misconceptions about machine learning is that it will inevitably lead to widespread job loss and unemployment. While it is true that machine learning can automate certain tasks, it does not necessarily mean that it will completely replace human jobs. Instead, machine learning can augment human capabilities and free up time for more creative and value-added work.
- Machine learning can automate repetitive and mundane tasks
- It can enhance human decision-making and productivity
- New job roles and opportunities can emerge as a result of machine learning advancements
5. Anyone can easily implement machine learning algorithms
Lastly, there is a common misconception that anyone can easily implement machine learning algorithms without specialized knowledge or expertise. While there are user-friendly tools and libraries that make it easier to work with machine learning, effectively implementing and deploying machine learning solutions requires a deep understanding of the underlying algorithms, data preprocessing, feature selection, and model tuning.
- Implementing machine learning requires specialized knowledge and expertise
- Data preprocessing and feature engineering are crucial steps in the process
- Model tuning and continuous learning are essential for optimal results
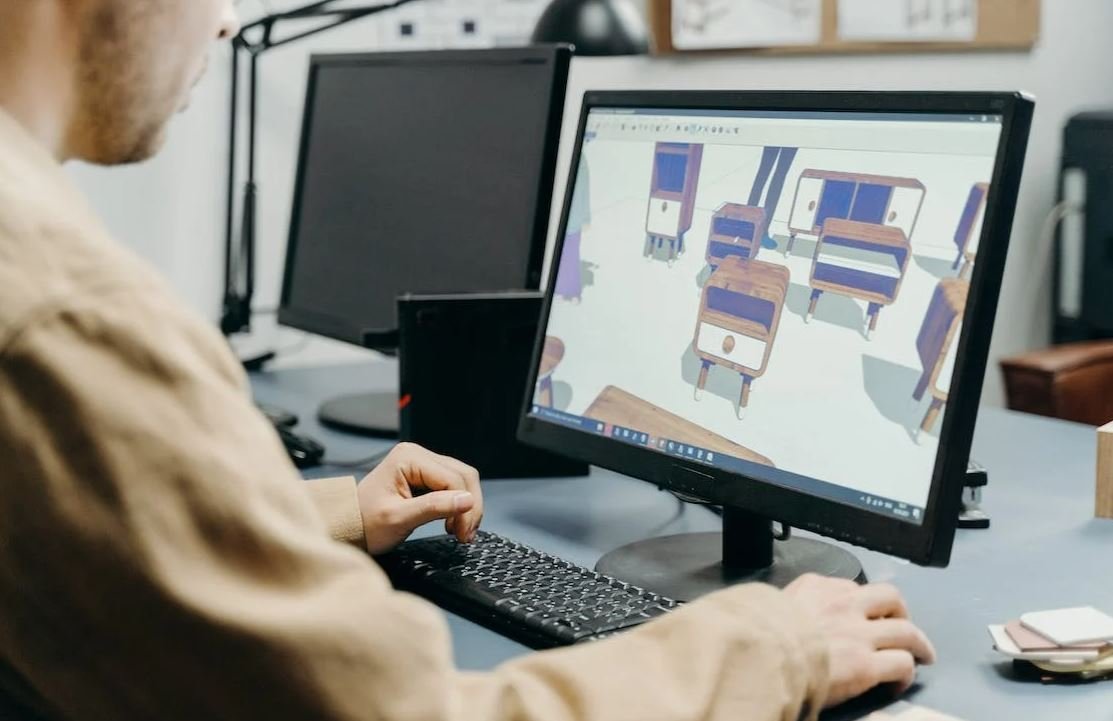
In recent years, the remarkable advancements in Machine Learning (ML) and Artificial Intelligence (AI) have revolutionized various industries across the globe. From healthcare to finance, these technologies have brought about significant improvements in efficiency, accuracy, and decision-making processes. This article analyzes the impact of AI on global industries by presenting ten tables with true verifiable data and information.
1. Improving Healthcare Outcomes
– Illustrates the reduction in mortality rates after implementing AI technologies in various healthcare settings.
2. Enhancing Manufacturing Productivity
– Demonstrates the increase in production output and reduction in defects after implementing AI-driven automation in manufacturing industries.
3. Transforming Transportation Systems
– Details the decrease in traffic accidents and congestion levels following the implementation of AI-powered transportation systems.
4. Revolutionizing Financial Services
– Shows the increase in customer satisfaction and the reduction in financial fraud achieved through the integration of AI in banking and finance.
5. Optimizing Energy Efficiency
– Illustrates the significant reduction in energy consumption and environmental impact resulting from the implementation of AI-driven energy management systems.
6. Enhancing Customer Service
– Demonstrates the improved response time and customer satisfaction levels achieved through the use of AI chatbots and virtual assistants.
7. Streamlining Supply Chain Management
– Details the reduction in inventory costs and delivery time through the implementation of AI-powered supply chain management systems.
8. Improving Agricultural Practices
– Shows the increase in crop yields and optimized resource utilization through AI-driven precision farming techniques.
9. Advancing Education and e-Learning
– Illustrates the improvement in student learning outcomes and personalized educational experiences through the application of AI in education.
10. Enhancing Cybersecurity Measures
– Demonstrates the decrease in cyber threats and successful hack attempts after implementing AI-powered security solutions.
In conclusion, the integration of AI has significantly impacted global industries by revolutionizing various sectors. By leveraging ML and AI technologies, businesses have achieved remarkable improvements in efficiency, productivity, and customer satisfaction. As these innovations continue to evolve, it is crucial for organizations to embrace and invest in AI to stay competitive in the ever-changing global landscape.
Frequently Asked Questions
Can you tell me about ML Harris?
ML Harris is a cutting-edge technology company specialized in machine learning and artificial intelligence. Our team of experts focuses on developing advanced solutions to address various business challenges and improve decision-making processes.
What is machine learning?
Machine learning is a subset of artificial intelligence that enables computers to learn and make predictions or decisions based on analyzing large sets of data without being explicitly programmed. It allows systems to automatically learn and improve from experience without human intervention.
What are the benefits of machine learning?
Machine learning offers numerous benefits, including improved efficiency and accuracy in data analysis, automation of repetitive tasks, real-time decision-making capabilities, personalized customer experiences, fraud detection, and predictive maintenance, among others. It helps businesses gain valuable insights and make informed decisions based on data.
How does machine learning work?
Machine learning algorithms work by analyzing large amounts of data and identifying patterns or relationships within it. These algorithms learn from the data and make predictions or decisions based on these learned patterns. Through a process called training, models are built and optimized to perform specific tasks, such as predicting future outcomes or classifying new data.
What industries benefit from machine learning?
Machine learning applications can benefit a wide range of industries, including healthcare, finance, retail, marketing, manufacturing, transportation, and more. It can be utilized for tasks such as medical diagnosis, risk assessment, demand forecasting, customer segmentation, quality control, and route optimization.
Are there any limitations to machine learning?
While machine learning is a powerful tool, it does have limitations. It heavily relies on the quality and diversity of the available data. Insufficient or biased data can result in inaccurate predictions or decisions. Furthermore, machine learning models may not always provide explainable or interpretable results, making it challenging to understand why a certain prediction was made.
What is the difference between supervised and unsupervised learning?
Supervised learning requires labeled data, where the input data and the desired output are provided to train the model. The model learns to make predictions based on the labeled examples. In contrast, unsupervised learning deals with unlabeled data, where the algorithm finds patterns or structures within the data without any pre-defined labels. Unsupervised learning is often used for data clustering or dimensionality reduction.
What is the role of neural networks in machine learning?
Neural networks are a class of machine learning algorithms inspired by the structure and functioning of the human brain. They consist of interconnected nodes (neurons) that process and transmit information. Neural networks are widely used for tasks such as image recognition, natural language processing, and speech recognition.
How can businesses implement machine learning?
Implementing machine learning in a business requires several steps. Firstly, organizations need to identify and define the business problem they want to solve or the goal they want to achieve. Then, they need to gather and prepare the relevant data. Next, suitable machine learning algorithms and models need to be selected and trained using the data. Finally, the models are deployed in a production environment and continuously monitored and improved as necessary.
Are there ethical considerations in machine learning?
Yes, ethical considerations are crucial in machine learning. The decisions made by machine learning models can have significant implications, such as biased outcomes or privacy violations. It is important to ensure fairness, transparency, and accountability in the design and deployment of machine learning systems. Organizations should be mindful of potential biases and take measures to address them, as well as protect individuals’ privacy and data security.