ML in a Quart
Machine learning (ML) is revolutionizing the way businesses operate and make decisions. With the power of ML algorithms, companies can analyze vast amounts of data to uncover valuable insights and predictions. ML has become an indispensable tool in various industries, ranging from healthcare and finance to marketing and agriculture. In this article, we explore the key benefits and applications of ML, showcasing how it can transform businesses to drive success in an increasingly data-driven world.
Key Takeaways
- Machine learning enables businesses to analyze large datasets and extract valuable insights.
- ML is used across various industries, including healthcare, finance, marketing, and agriculture.
- ML algorithms can be applied to tasks such as customer segmentation, fraud detection, and predictive analytics.
- Implementing ML requires skilled professionals and data infrastructure.
- Continuous learning and adaptation are essential for successful ML implementation.
**ML algorithms** are designed to learn from data and improve their performance over time. These algorithms are based on statistical models and computational techniques that allow machines to automatically learn patterns and make predictions or decisions without explicit programming. *With ML, businesses can discover valuable insights hidden within vast datasets, empowering data-driven decision-making.* The scalability and efficiency of ML algorithms make them suitable for handling varied and complex tasks across different industries.
Applications of ML in Business
The use of ML in business spans across many sectors and applications. Let’s explore some key areas where ML provides significant benefits:
- **Customer Segmentation:** ML algorithms can analyze customer data to classify them into distinct groups based on their preferences, behavior, and demographics. This segmentation enables companies to tailor products and marketing strategies to specific customer segments, ultimately improving customer satisfaction and profitability.
- **Fraud Detection:** By analyzing patterns and anomalies in large datasets, ML algorithms can detect fraudulent activities and transactions with higher accuracy compared to traditional rule-based systems. ML-powered fraud detection systems help businesses mitigate risks and protect their financial resources.
- **Predictive Analytics:** ML models can analyze historical data to make predictions and forecasts about future events or trends. This enables businesses to anticipate customer behavior, market trends, demand patterns, and optimize their operations accordingly. Predictive analytics helps companies make informed decisions and gain a competitive edge in their industries.
*The ability of ML algorithms to process vast amounts of data and identify patterns that may not be immediately apparent to humans is what sets them apart.* Machine learning algorithms can uncover complex relationships and dependencies that can impact business performance. By leveraging the power of ML, companies can gain valuable insights and make data-driven decisions, leading to improved performance and increased efficiency.
Benefits and Challenges of Implementing ML
While the benefits of ML are apparent, implementing ML solutions comes with its own set of challenges. Let’s consider both the benefits and challenges:
Benefits of ML Implementation | Challenges of ML Implementation |
---|---|
|
|
*Finding skilled professionals who can develop and implement ML algorithms is crucial for successful ML adoption.* Building the necessary data infrastructure, including data storage and processing capabilities, is also essential. Moreover, businesses must address data privacy concerns and ensure compliance with relevant regulations. Finally, the ever-evolving nature of data and business dynamics requires continuous learning and adaptation for ML models to remain effective over time.
Conclusion
ML has become an indispensable tool for businesses, enabling them to unlock valuable insights and make data-driven decisions. The implementation of ML offers numerous benefits, ranging from improved decision-making to enhanced efficiency and productivity. However, it is essential to address the challenges associated with ML implementation, including the need for skilled professionals, robust data infrastructure, and ongoing learning and adaptation. Embracing ML can truly transform businesses and give them a competitive edge in the rapidly changing business landscape.
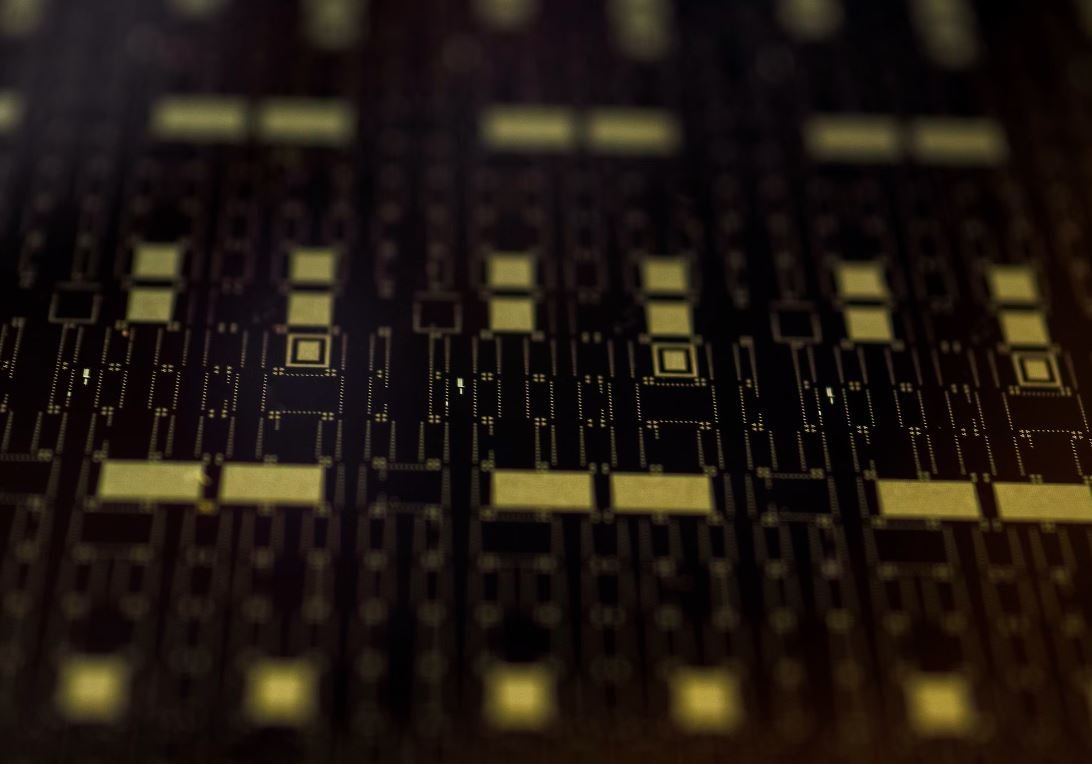
Common Misconceptions
Misconception 1: ML in a Quart means full automation
One common misconception people have about ML in a Quart is that it means full automation. While machine learning can improve and automate certain tasks, it does not completely replace human involvement. An ML algorithm still requires human guidance and supervision to ensure accurate and ethical results.
- ML algorithms require human input and guidance.
- Human supervision ensures accuracy and ethics in ML applications.
- ML in a Quart is a tool to enhance human productivity, not replace it.
Misconception 2: ML in a Quart guarantees accurate predictions
Another misconception is that ML algorithms in a Quart can provide guaranteed accurate predictions. While machine learning can make predictions based on large datasets and patterns, there is always a margin of error. The accuracy of ML predictions depends on various factors such as the quality of data, complexity of the problem, and the algorithm itself.
- ML predictions have a margin of error.
- The accuracy of ML predictions depends on data quality and complexity of the problem.
- ML algorithms can provide insights and probabilities rather than definite outcomes.
Misconception 3: ML in a Quart is only for large organizations
Some people believe that ML in a Quart is only beneficial for large organizations with massive datasets. However, ML algorithms can be beneficial for businesses of all sizes. Small and medium-sized enterprises (SMEs) can leverage ML to automate processes, analyze customer behavior, and make data-driven decisions, leading to improved efficiency and competitiveness.
- ML benefits all organizations, irrespective of their size.
- SMEs can use ML to enhance efficiency and competitiveness.
- ML algorithms are scalable and adaptable to different business needs.
Misconception 4: ML in a Quart is only for tech-savvy individuals
Some people assume that ML in a Quart is only accessible and relevant to individuals with technical knowledge and expertise. However, with the availability of user-friendly ML tools and platforms, anyone can benefit from ML applications. Non-technical individuals can use pre-built ML models or collaborate with ML experts to leverage the power of machine learning.
- You don’t need to be tech-savvy to benefit from ML in a Quart.
- User-friendly ML tools and platforms are available for non-technical individuals.
- Collaboration with ML experts can help individuals leverage ML efficiently.
Misconception 5: ML in a Quart is a threat to job security
There is a misconception that ML in a Quart will lead to job losses due to automation. While ML can automate certain tasks, it also creates opportunities for new jobs and roles. ML can augment human skills, allowing individuals to focus on higher-value tasks such as analysis, decision-making, and creativity. It is more about job transformation than job elimination.
- ML in a Quart can create new job opportunities.
- ML can augment human skills and enhance productivity.
- Job roles may transform with the integration of ML, but not necessarily disappear.
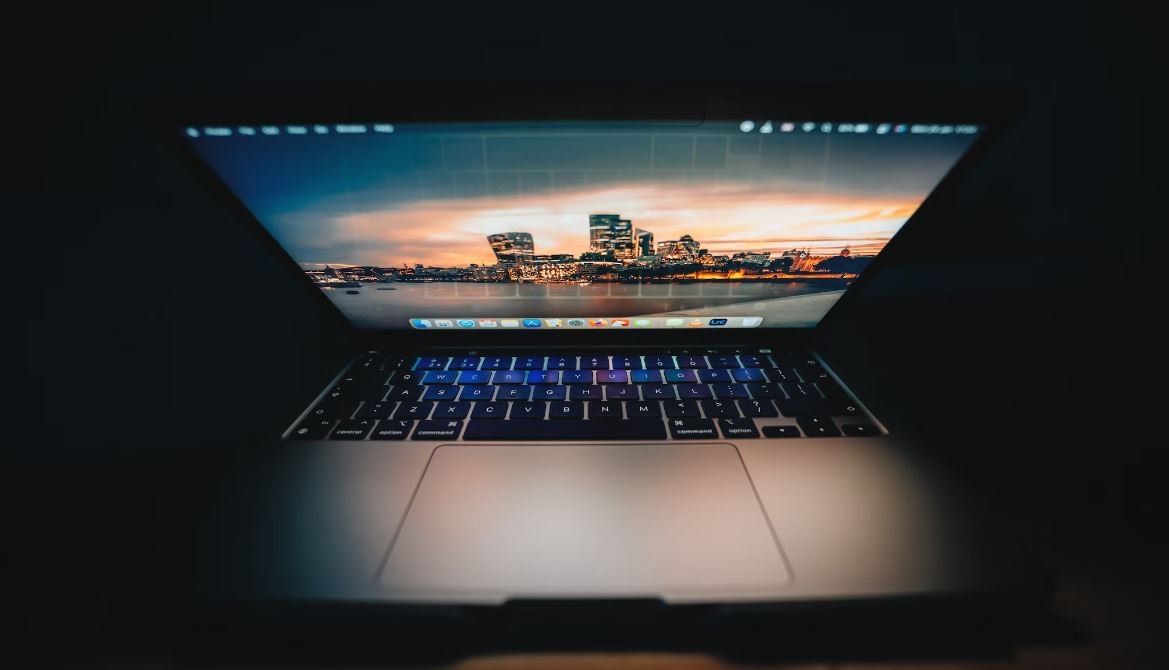
ML in a Quart
In recent years, Machine Learning (ML) has emerged as a powerful tool that has revolutionized various industries. The ability of ML algorithms to learn from data and make accurate predictions has unlocked new possibilities in sectors such as healthcare, finance, and entertainment. This article explores the applications and impact of ML, showcasing ten fascinating examples of real-world implementations.
The Rise of ML in Medicine
Machine Learning techniques have significantly influenced the field of medicine by improving diagnostic accuracy, reducing treatment costs, and enhancing patient outcomes. The following table illustrates ten remarkable ML applications in medicine:
Application | Description | Benefits |
---|---|---|
Radiology Image Analysis | Automated detection of abnormalities in medical images | Quicker diagnosis, reduced human error |
Cancer Diagnosis | Prediction of cancer presence and subtype | Early detection, better treatment planning |
Drug Discovery | Identifying potential new drug candidates | Accelerated drug development, lower costs |
Clinical Decision Support | Assisting doctors in making treatment recommendations | Improved care accuracy, reduced misdiagnosis |
Genomics | Interpreting vast amounts of genetic data | Personalized medicine, targeted therapies |
Health Monitoring | Continuous monitoring of vital signs and disease indicators | Timely intervention, early warning signs |
Electronic Health Records | Efficient management and analysis of patient data | Improved record keeping, better patient care coordination |
Robot-Assisted Surgery | Enhancing surgical precision and minimizing complications | Reduced surgery time, enhanced outcomes |
Healthcare Fraud Detection | Identifying fraudulent activities in insurance claims | Cost savings, improved resource allocation |
Mental Health Diagnosis | Recognition of mental health disorders from patient records | Early intervention, improved treatment planning |
ML Applications in Finance
The finance industry has been swiftly adopting ML techniques to analyze vast amounts of data, increase efficiency, and make more accurate predictions. Here, we present ten intriguing implementations of ML in finance:
Application | Description | Benefits |
---|---|---|
Stock Market Prediction | Forecasting stock prices based on historical data | Informed investment decisions, reduced risk |
Credit Risk Assessment | Evaluating borrower’s creditworthiness | Reduced default rates, improved loan assessment |
Fraud Detection | Identifying fraudulent transactions and activities | Minimized financial losses, enhanced security |
Algorithmic Trading | Automated buying and selling of financial instruments | Increased trade execution speed, improved strategies |
Customer Segmentation | Grouping customers based on behavior and preferences | Targeted marketing campaigns, improved customer experience |
Loan Approval | Predicting loan approval likelihood | Faster loan processing, reduced human bias |
Market Sentiment Analysis | Analyzing social media trends for stock market prediction | Improved decision-making, timely responses |
Insurance Underwriting | Evaluating risk and pricing insurance policies | Accurate pricing, improved profitability |
Portfolio Management | Optimizing asset allocation and portfolio performance | Maximized returns, reduced risks |
Predictive Pricing | Predicting price fluctuations in financial markets | Improved pricing strategies, reduced losses |
ML Transforms Entertainment
The entertainment industry has embraced ML to enhance user experiences, recommend content, and create innovative experiences. Below are ten captivating ML applications within the entertainment sector:
Application | Description | Benefits |
---|---|---|
Recommendation Systems | Personalized content recommendations for users | Improved user engagement, increased content consumption |
Speech and Image Recognition | Enabling automatic transcription and content tagging | Easier content annotation, enhanced search capabilities |
Character Animation | Creating realistic movements for animated characters | Enhanced visual effects, immersive experiences |
Sentiment Analysis | Understanding audience reception through social media data | User feedback analysis, improved content marketing |
Movie Genre Classification | Automated classification of movies into genres | Targeted recommendations, improved search filters |
Music Composition | Generating original music using ML algorithms | Innovative content creation, reduced production time |
Ad Placement Optimization | Optimizing ad placement and content targeting | Higher ad performance, increased revenue |
Content Moderation | Automatic detection and removal of inappropriate content | Enhanced user safety, improved content quality |
Game AI | Development of intelligent AI opponents | Enhanced gaming experience, increased challenge |
Real-time Video Analytics | Automatic analysis of video content for live events | Enhanced live streaming experience, instant insights |
Machine Learning has become a driving force behind innovations in multiple industries, ranging from medicine to finance and entertainment. With its ability to process massive amounts of data and make intelligent predictions, ML has opened doors to new solutions and transformed the way we live and work. As the technology continues to advance, we can expect even more remarkable applications in the future, pushing the boundaries of what is possible.
Frequently Asked Questions
What is ML in a Quart?
ML in a Quart is a term used to refer to running machine learning models using Flask, a micro web framework for Python, specifically inside a Quart Flask application environment. This combination allows users to deploy and serve their machine learning models as web applications.
What are the benefits of ML in a Quart?
ML in a Quart offers several benefits, including:
- Integration of machine learning models within a Flask-based web framework.
- Scalability and flexibility provided by Quart’s asynchronous capabilities.
- Ability to easily build and deploy machine learning-based web applications.
- Access to a wide range of Flask extensions for enhanced functionality.
- Efficient utilization of available computational resources.
<
How does ML in a Quart work?
ML in a Quart works by creating a Flask application using the Quart library and incorporating the machine learning model(s) into the application’s routes. When a request is made to the Flask application, the appropriate route handles it by executing the corresponding machine learning code and returning the result as a response.
Can any machine learning model be used with ML in a Quart?
Yes, ML in a Quart supports various machine learning models. Quart being built on top of Flask provides flexibility, allowing users to integrate any machine learning model that can be run within a Python environment. Models using frameworks such as TensorFlow, PyTorch, scikit-learn, or even custom implementations can be utilized with ML in a Quart.
Do I need to have prior experience with Flask to use ML in a Quart?
Having prior experience with Flask is beneficial, but not mandatory. Familiarity with Flask would enable users to make the most of its features and extensions. However, the Quart documentation provides comprehensive guidelines to help users start building ML-based applications even if they are new to Flask.
Are there any limitations to consider when using ML in a Quart?
While ML in a Quart offers great flexibility and scalability, there are a few limitations to consider:
- Increased complexity compared to simpler Flask applications, especially for beginners.
- Potential overhead in terms of computational resources required for asynchronous processing.
- Possibility of challenges in debugging due to the asynchronous nature of Quart.
Can ML in a Quart be deployed on cloud platforms?
Yes, ML in a Quart applications can be deployed on various cloud platforms that support Flask applications, such as Heroku, Google Cloud Platform, Amazon Web Services, or Azure. The deployment process for ML in a Quart on these platforms is similar to deploying a regular Flask application.
Is ML in a Quart suitable for real-time prediction scenarios?
Yes, ML in a Quart can be utilized for real-time prediction scenarios. Quart’s asynchronous capabilities allow for efficient handling of multiple requests, enabling real-time prediction with low latency. However, the actual suitability depends on the specific machine learning model and the computational requirements associated with it.
Can ML in a Quart handle large datasets?
ML in a Quart can handle large datasets, but the performance and scalability depend on various factors. These include the available computational resources, the efficiency of the machine learning model, and the optimizations applied to handle large datasets. It is advisable to consider model size, memory requirements, and the specific use case when working with large datasets.
Is it possible to deploy multiple machine learning models with ML in a Quart?
Yes, ML in a Quart allows for the deployment of multiple machine learning models within a single Flask application. Users can define different routes for each model or utilize a single route that handles multiple models based on different requests or parameters. The choice depends on the specific application requirements and desired functionality.