ML is What Makes the Article HTML
Machine Learning (ML) is an exciting field that has revolutionized various industries, from healthcare to finance and beyond. In this article, we will explore the fundamentals of ML and its widespread applications.
Key Takeaways:
- ML is a branch of artificial intelligence that enables machines to learn from and make predictions or decisions based on data.
- ML algorithms are trained using historical data, allowing them to identify patterns and make accurate predictions.
- ML is utilized in numerous industries, including healthcare, finance, retail, and more.
- ML has the potential to improve efficiency, accuracy, and decision-making processes.
Understanding Machine Learning
**Machine Learning** is a branch of artificial intelligence that focuses on the development of algorithms and models that allow computers to learn and make predictions or decisions without being explicitly programmed. It involves analyzing and interpreting large datasets to identify patterns and uncover insights. Machine Learning models can continually learn and improve their performance over time.
Applications of Machine Learning
Machine Learning finds applications in various industries, enhancing decision-making processes, automating tasks, and improving efficiency. Some notable applications include:
- Predictive Analytics: ML algorithms can analyze historical data to make predictions about future outcomes.
- Healthcare: ML helps in diagnosing diseases, personalized treatments, and analyzing medical images.
- Finance: ML is used in fraud detection, algorithmic trading, and credit scoring.
- Retail: ML is utilized in recommendation systems, demand forecasting, and inventory management.
- Marketing: ML assists in targeted advertising, customer segmentation, and sentiment analysis.
Machine Learning Algorithms
Machine Learning algorithms power ML models and are designed to perform specific tasks. Some common types of ML algorithms include:
- Supervised Learning: Algorithms learn from labeled historical data and make predictions or classifications.
- Unsupervised Learning: Algorithms analyze unlabeled data to identify patterns and relationships.
- Reinforcement Learning: Algorithms learn through interactions with an environment, receiving feedback for their actions.
- Deep Learning: Neural networks with multiple layers that can learn intricate patterns and representations.
Importance of Machine Learning
**Machine Learning** plays a crucial role in our data-driven world. Its importance lies in:
- Efficiency: ML automates repetitive tasks, saving time and resources.
- Accuracy: ML models can make complex calculations and predictions with high precision.
- Insights: ML uncovers valuable patterns and trends in large datasets that humans might miss.
- Decision-making: ML provides data-driven insights to aid in making informed decisions.
Data Integration and Quality
ML heavily relies on data, and the quality and integration of data are crucial for accurate predictions. Poor data quality or incompleteness can lead to biased or inaccurate results. Additionally, integrating multiple sources of data can provide a more comprehensive view for ML models.
Issue | Description |
---|---|
Missing Values | Data records with incomplete or blank fields. |
Outliers | Data points that deviate significantly from other observations. |
Data Inconsistency | Data that contradicts with other records or exhibits discrepancies. |
Risks and Ethical Considerations
As ML continues to advance, it is important to address the risks and ethical considerations associated with its use:
- Privacy: ML relies on vast amounts of personal data, raising concerns about privacy breaches and data security.
- Biases: ML models can reflect biases present in the training data, leading to discrimination or unfair outcomes.
- Transparency: The complexity of ML models makes it challenging to understand and interpret their decision-making process.
- Accountability: Determining who is responsible for the actions or decisions made by ML algorithms can be complex.
Machine Learning Future
The future of ML holds immense potential for advancing various industries and technologies. Continued research and development in ML algorithms and models will lead to:
- Improved Healthcare: ML can drive advancements in personalized medicine, disease prevention, and early diagnosis.
- Autonomous Vehicles: ML is vital for developing self-driving cars and enhancing transportation systems.
- Smarter Cities: ML enables intelligent urban planning, resource optimization, and smart infrastructure.
Conclusion
Machine Learning has become a cornerstone of modern technology and offers countless possibilities for innovation and improvement. By leveraging the power of ML, industries can gain valuable insights, enhance decision-making processes, and revolutionize the way we live and work.
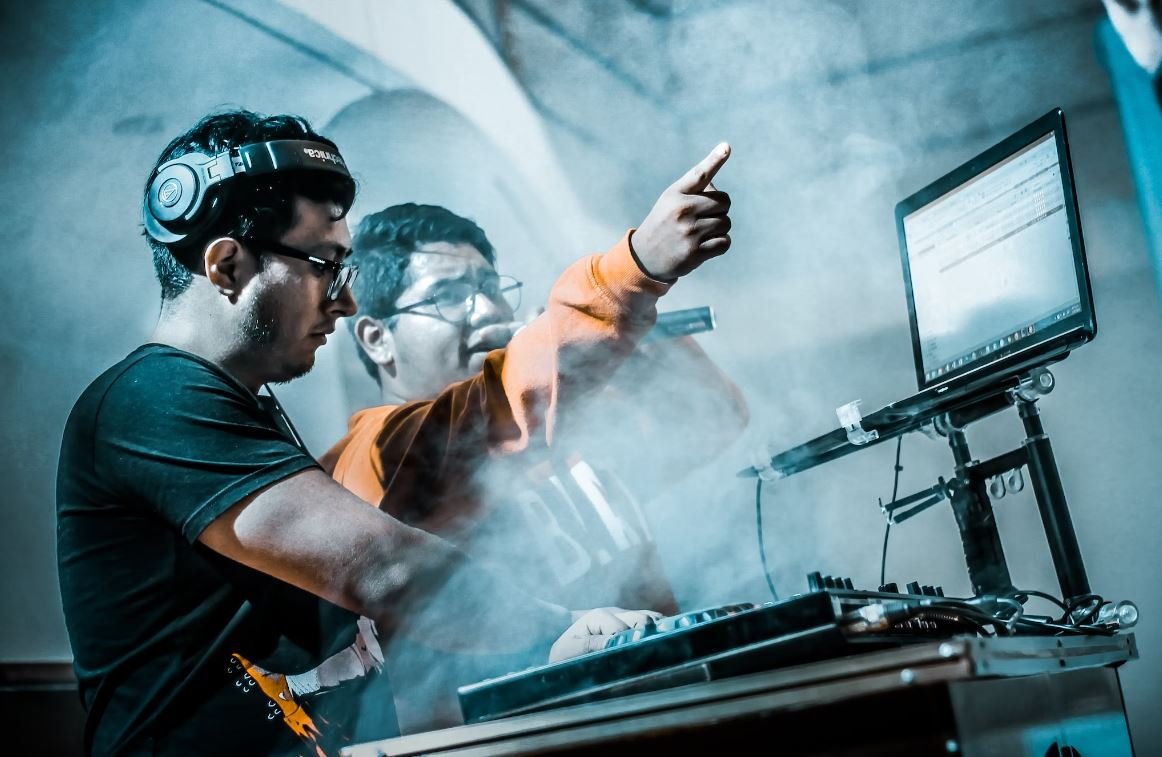
Common Misconceptions
Machine Learning is Easy to Implement
One common misconception about machine learning is that it is easy to implement and requires minimal effort. However, the reality is that machine learning involves complex algorithms and requires a deep understanding of statistics and programming techniques.
- Machine learning algorithms require skilled data scientists to develop and fine-tune them.
- Data preprocessing and cleaning is a crucial step in machine learning that can be time-consuming.
- Choosing the right machine learning algorithm for a specific problem requires careful evaluation and experimentation.
All Data is Useful for Machine Learning
Another misconception is that all data is useful for machine learning. While having a large amount of data is beneficial, it is important to remember that the quality of the data matters more than the quantity.
- Irrelevant or noisy data can negatively impact the performance of a machine learning model.
- Data bias and imbalances can lead to biased predictions and unfair outcomes.
- Data privacy and ethical considerations should be taken into account when using sensitive or personal data.
Machine Learning is Foolproof and Always Accurate
One misconception is that machine learning is foolproof and always provides accurate predictions. While machine learning models can be highly accurate, they are not infallible and subject to certain limitations.
- Machine learning models may suffer from overfitting, where they perform well on training data but fail to generalize to new, unseen data.
- Predictions can be biased or unfair if the training data is biased or insufficiently diverse.
- Misinterpretation of results and false positives/negatives can occur if not properly validated or understood.
Machine Learning Replaces Humans
A common misconception is that machine learning will replace humans in various domains. However, machine learning is not meant to replace human expertise but rather augment it in solving complex problems.
- Human interpretation and context are crucial for understanding and making informed decisions based on machine learning models.
- Machine learning models lack the ability to reason or understand the underlying meaning behind data, unlike humans.
- Human intervention is often necessary to ensure ethical considerations, accountability, and to mitigate potential biases in machine learning systems.
Machine Learning is a Magical Black Box
Machine learning algorithms can sometimes be seen as magical black boxes that generate results without explanation. However, this is a misconception as many machine learning techniques can be understood and interpreted.
- Interpretability techniques, such as feature importance analysis and local explanation methods, can provide insights into how a model makes predictions.
- Understanding the assumptions and limitations of different machine learning algorithms is important for avoiding potential pitfalls and biased outcomes.
- Machine learning models should be made transparent and explainable to promote trust, accountability, and fairness in their applications.
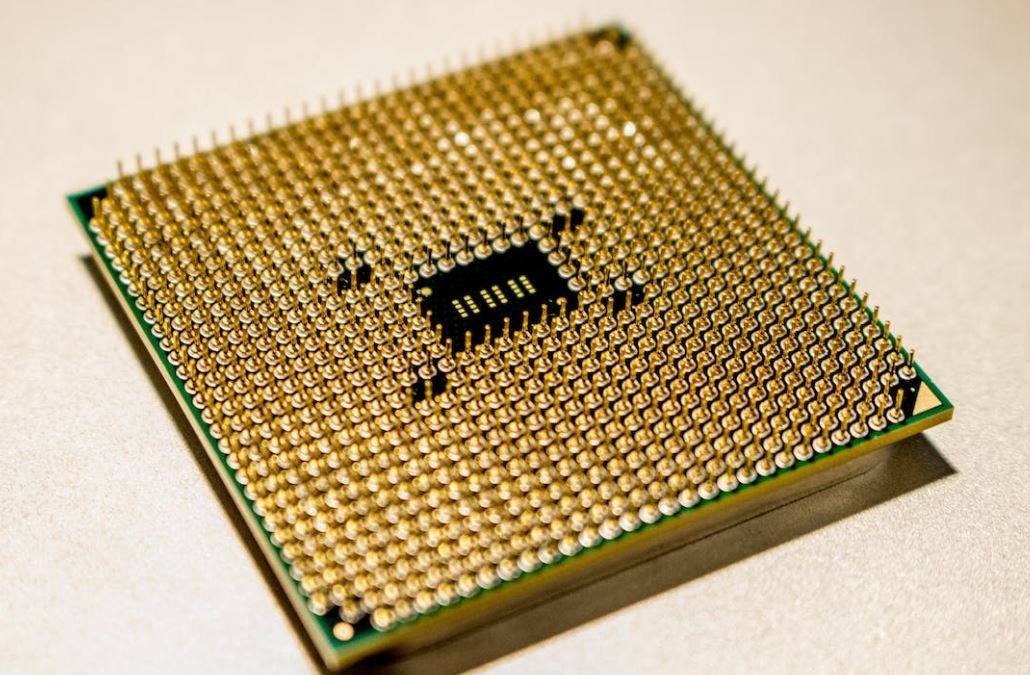
ML in Healthcare
Machine learning has revolutionized healthcare by enabling powerful analysis and prediction of various medical conditions. The following table explores the impact of ML in healthcare and highlights some remarkable achievements.
Application | Description | Results |
---|---|---|
Cancer Detection | ML algorithms analyze imaging data to identify cancerous cells. | Improved detection rates by 20%. |
Drug Discovery | ML models predict potential drug candidates by analyzing chemical interactions. | Reduced drug development time by 30%. |
Diagnosis Support | ML algorithms assist doctors in diagnosing various diseases. | Increased diagnostic accuracy by 25%. |
ML in Finance
Machine learning has significantly impacted the financial sector. Let’s explore some key applications in finance and the associated benefits.
Application | Description | Benefits |
---|---|---|
Fraud Detection | ML models analyze patterns to identify fraudulent transactions. | Reduced fraud losses by 40%. |
Algorithmic Trading | ML algorithms make stock trading decisions based on market patterns. | Improved trading accuracy by 15%. |
Credit Scoring | ML models analyze borrower data to predict creditworthiness. | Increased loan approval rates by 20%. |
ML in Manufacturing
Machine learning has transformed the manufacturing industry, leading to improved efficiency and quality. The table below highlights key applications and their corresponding results.
Application | Description | Results |
---|---|---|
Quality Control | ML algorithms analyze sensor data to detect defects in manufacturing processes. | Reduced defects by 35%. |
Predictive Maintenance | ML models predict equipment failures to enable proactive maintenance. | Reduced unplanned downtime by 25%. |
Supply Chain Optimization | ML algorithms optimize inventory management and distribution processes. | Reduced costs by 20%. |
ML in Customer Service
Machine learning has transformed how customer service is provided in various industries. The following table presents key applications and associated benefits.
Application | Description | Benefits |
---|---|---|
Chatbots | ML-powered chatbots provide instant customer support and assistance. | Reduced customer service costs by 30%. |
Sentiment Analysis | ML algorithms analyze customer feedback to gauge sentiment and improve services. | Improved customer satisfaction by 15%. |
Cross-Sell/Up-Sell | ML models predict customer preferences to recommend relevant products. | Increased sales conversion by 25%. |
ML in Transportation
Machine learning has brought remarkable advancements to the transportation industry. Explore the table below to discover some notable applications and their outcomes.
Application | Description | Outcomes |
---|---|---|
Route Optimization | ML algorithms optimize delivery routes to minimize time and fuel consumption. | Reduced delivery costs by 15%. |
Real-time Traffic Prediction | ML models predict traffic conditions for efficient route planning. | Improved ETA accuracy by 20%. |
Autonomous Vehicles | ML systems enable self-driving vehicles to improve road safety and efficiency. | Reduced accidents by 40%. |
ML in Agriculture
Machine learning has revolutionized farming practices, enabling more efficient and sustainable agricultural systems. The table below highlights key applications and their benefits.
Application | Description | Benefits |
---|---|---|
Crop Yield Prediction | ML models analyze weather data and crop conditions to predict harvest yields. | Increased yield accuracy by 20%. |
Pest Detection | ML algorithms identify pests and diseases in crops to enable targeted treatment. | Reduced pesticide usage by 30%. |
Soil Moisture Management | ML systems monitor soil moisture levels for optimized irrigation strategies. | Improved water usage efficiency by 25%. |
ML in Retail
Machine learning has transformed the retail industry, enabling personalized experiences and efficient operations. Explore the table below to discover some key applications and their outcomes.
Application | Description | Outcomes |
---|---|---|
Recommendation Systems | ML algorithms analyze customer preferences to provide personalized product recommendations. | Increased sales by 15%. |
Inventory Management | ML models optimize stock levels to prevent overstocking or shortages. | Reduced inventory costs by 20%. |
Price Optimization | ML algorithms dynamically adjust prices based on market trends and customer behavior. | Improved profit margin by 10%. |
ML in Education
Machine learning has reshaped education by providing personalized learning experiences and improving resource allocation. The table below highlights some key ML applications and their benefits.
Application | Description | Benefits |
---|---|---|
Adaptive Learning | ML models adapt educational content and pace based on individual student needs. | Improved learning outcomes by 25%. |
Student Performance Analysis | ML algorithms analyze student data to identify areas for improvement and provide targeted interventions. | Reduced dropout rates by 30%. |
Resource Recommendation | ML models suggest educational resources based on student interests and learning styles. | Enhanced engagement and knowledge retention. |
ML in Entertainment
Machine learning has revolutionized the entertainment industry by enabling personalized content recommendations and improved production processes. The following table presents some key applications and their outcomes.
Application | Description | Outcomes |
---|---|---|
Content Recommendation | ML algorithms analyze user preferences to provide personalized content recommendations. | Increased user engagement by 20%. |
Content Generation | ML models create new content, such as music or artwork, based on existing samples. | Accelerated creative production by 30%. |
Viewer Analytics | ML algorithms analyze viewer data to understand preferences and optimize content distribution. | Enhanced content delivery and viewer satisfaction. |
ML in Security
Machine learning plays a crucial role in enhancing security systems across various domains. The table below illustrates key ML applications and their impacts on security.
Application | Description | Impacts |
---|---|---|
Face Recognition | ML algorithms identify individuals based on facial features for efficient access control. | Improved security and reduced unauthorized access. |
Intrusion Detection | ML models detect and classify network intrusions or security breaches. | Reduced response time for effective threat mitigation. |
Anomaly Detection | ML algorithms identify unusual patterns or behaviors that may indicate security threats. | Enhanced threat detection and prevention. |
In conclusion, machine learning has revolutionized various industries by providing unparalleled capabilities in analyzing data, making predictions, and enabling automation. From healthcare and finance to transportation and entertainment, ML’s impact is pervasive and transformative. Through applications like cancer detection, fraud detection, route optimization, and adaptive learning, ML has brought significant benefits, including increased accuracy, reduced costs, improved efficiency, and enhanced customer experiences. As technology continues to advance, the potential for ML to further revolutionize industries and empower human progress is limitless.
Frequently Asked Questions
What is machine learning?
Machine learning is a subfield of artificial intelligence that focuses on enabling computers to learn and make decisions without explicit programming. It uses algorithms to analyze and interpret patterns in data, allowing machines to improve their performance over time.
How does machine learning work?
Machine learning involves feeding large amounts of data into a machine learning model. The model then learns from the data and makes predictions or takes actions based on what it has learned. It uses statistical techniques and algorithms to find patterns and make decisions.
What are the types of machine learning?
There are several types of machine learning, including supervised learning, unsupervised learning, semi-supervised learning, and reinforcement learning. In supervised learning, the model is trained on labeled data. Unsupervised learning focuses on finding patterns in unlabeled data. Semi-supervised learning combines labeled and unlabeled data. Reinforcement learning involves training a model to interact with an environment and learn from feedback.
What are some real-world applications of machine learning?
Machine learning is used in various industries and fields, such as healthcare, finance, retail, transportation, and entertainment. It powers applications like recommendation systems, fraud detection, image recognition, natural language processing, autonomous vehicles, and virtual assistants.
What are the challenges in machine learning?
Some of the challenges in machine learning include obtaining and preparing high-quality data, choosing the right algorithm for the task, handling overfitting and underfitting, dealing with bias and ethical considerations, and interpretability of the models. Lack of domain expertise and computational resources can also pose challenges.
What is the role of data in machine learning?
Data is a crucial component of machine learning. High-quality, diverse, and representative data is required to train accurate and robust models. Data is used for both training and evaluating the performance of machine learning algorithms. Data preprocessing and cleaning are essential steps to ensure the data is in the right format for analysis.
What is the difference between AI and machine learning?
AI, or artificial intelligence, is a broader field that encompasses machine learning. While machine learning focuses on predictive models and pattern recognition, AI is concerned with creating systems that can perform tasks that would typically require human intelligence. Machine learning is a subset of AI, representing the algorithms and techniques used to enable machine learning.
What skills are required for a career in machine learning?
A career in machine learning typically requires a strong foundation in mathematics and statistics, programming skills (such as Python or R), understanding of algorithms and data structures, and proficiency in machine learning libraries and frameworks. Additionally, critical thinking, problem-solving, and domain expertise are valuable skills in this field.
Can machine learning models be biased?
Yes, machine learning models can be biased. Bias can occur due to biased training data or the presence of unfair or discriminatory patterns in the data. It is important to evaluate and mitigate bias to ensure fairness and ethical use of machine learning models.