ML News
Machine Learning (ML) continues to evolve and make significant advancements in various industries. This article aims to provide you with the latest updates and key insights in the world of ML.
Key Takeaways
- Machine Learning is revolutionizing industries across the board.
- Advancements in ML algorithms and techniques are enabling more accurate predictions.
- ML is driving improvements in healthcare, finance, and customer experience.
- Keeping up with ML news is crucial for professionals in the field.
Advancements in ML Algorithms
Recent developments in ML algorithms have led to more accurate and efficient models. Techniques such as deep learning and reinforcement learning have gained popularity due to their ability to handle complex tasks and make better predictions. These advancements have revolutionized industries such as healthcare, finance, and customer service, where accurate predictions play a crucial role. The integration of these algorithms into existing systems is opening new opportunities for businesses to improve their decision-making processes.
AI-powered recommendation systems are now able to better understand user preferences and provide more personalized recommendations, resulting in improved customer experiences.
Applications of ML in Healthcare
The healthcare industry is leveraging ML to enhance various aspects of patient care and medical research. ML algorithms are being used to develop personalized treatment plans, predict disease outcomes, and aid in the early detection of diseases through analysis of medical images and patient data. With the help of ML, doctors and medical practitioners can make more accurate diagnoses, leading to improved patient outcomes. ML also plays a significant role in drug discovery, helping researchers identify potential molecules and compounds for the development of new medications.
Through ML analysis, researchers have discovered promising leads for a potential breakthrough in cancer treatment.
The Impact of ML in Finance
The finance industry heavily relies on ML algorithms for a wide range of applications, including fraud detection, credit scoring, and risk assessment. ML models can analyze large volumes of financial data to identify patterns and anomalies that human analysts might overlook. This helps financial institutions minimize risks and improve decision-making. Additionally, ML algorithms can provide personalized financial advice and investment recommendations based on individual profiles and market trends.
By leveraging ML algorithms for fraud detection, financial institutions have saved millions of dollars in prevented losses.
Customer Experience Optimization with ML
ML is transforming how businesses interact with their customers. By analyzing customer data, ML models can predict individual preferences and behavior, enabling companies to provide personalized experiences. Recommendation systems powered by ML algorithms can suggest relevant products or content, enhancing customer satisfaction and increasing sales. ML also enables sentiment analysis, which helps companies understand and respond to customer feedback more effectively.
Companies utilizing ML for customer experience optimization have seen a significant increase in customer loyalty and retention rates.
Tables with Interesting Stats
Industry | Impact of ML |
---|---|
Healthcare | Reduced diagnostic errors by 40%. |
Finance | Saved $1 billion annually through fraud detection. |
Retail | Increased conversion rates by 25% through personalized recommendations. |
ML Algorithm | Application |
---|---|
Deep Learning | Image recognition and natural language processing. |
Decision Trees | Customer segmentation and risk assessment. |
Random Forests | Medical diagnosis and credit scoring. |
ML News Source | Website |
---|---|
AI News | www.example.com/ai-news |
ML Magazine | www.example.com/ml-magazine |
Data Science Today | www.example.com/data-science-today |
Staying up-to-date with the latest ML news and advancements is crucial for professionals working in this field. With continuous developments and new breakthroughs being made, it is essential to keep learning and adapting to stay ahead. Whether you’re a practitioner, researcher, or simply interested in the field, exploring reputable ML news sources will provide you with valuable insights to further enhance your knowledge and expertise.

Common Misconceptions
Misconception #1: Machine learning is the same as artificial intelligence (AI)
One common misconception surrounding this topic is that machine learning and artificial intelligence are interchangeable. However, while they are related, they are not the same thing. Machine learning refers to the process of training a computer system to learn and improve from data, while artificial intelligence encompasses a broader concept of creating intelligent machines that can perform tasks that typically require human intelligence.
- Machine learning is a subset of artificial intelligence.
- Artificial intelligence includes other techniques like natural language processing and expert systems.
- Machine learning is the technology behind many AI applications.
Misconception #2: Machine learning cannot work without large amounts of data
Another common misconception is that machine learning algorithms can only work effectively with large datasets. While it is true that having more data can generally improve the accuracy of models, it doesn’t mean that smaller datasets are entirely useless. In fact, many machine learning algorithms can still provide meaningful insights with relatively smaller amounts of data.
- Some algorithms are specifically designed for working with small datasets.
- Feature engineering and careful selection can compensate for limited data.
- Data sampling techniques can be used to create synthetic data and augment smaller datasets.
Misconception #3: Machine learning is only for tech-savvy individuals or businesses
Many people mistakenly assume that machine learning is an advanced field that requires extensive technical knowledge and expertise. While having a solid understanding of mathematics and programming can be helpful, machine learning has become more accessible in recent years. There are now user-friendly tools and platforms available that allow individuals and businesses with limited coding experience to implement and benefit from machine learning models.
- There are drag-and-drop machine learning platforms that require minimal coding.
- Online courses and tutorials make machine learning more accessible to beginners.
- Machine learning APIs provide pre-trained models that can be easily integrated into applications.
Misconception #4: Machine learning can replace human decision-making entirely
Some people believe that machine learning has the potential to completely replace human decision-making processes. While machine learning can automate certain tasks and improve efficiency, it is not meant to replace human judgment entirely. Machine learning models are limited by the quality of data they are trained on and may not always consider all relevant factors involved in decision-making.
- Machine learning models can assist human decision-making, but not replace it.
- Ethical considerations and moral judgments may require human intervention.
- Human judgment can provide context and reasoning that machines may lack.
Misconception #5: Machine learning is infallible and always produces accurate results
Lastly, a common misconception is that machine learning algorithms always produce accurate and reliable results. While machine learning models can be highly accurate in certain scenarios, they are not infallible and can produce incorrect predictions or outputs. Factors like biased data, incorrect assumptions, and overfitting can impact the accuracy of machine learning models.
- Evaluation and validation are crucial to assess the reliability of machine learning models.
- Model performance can vary based on different datasets or real-world conditions.
- Ongoing monitoring and maintenance are necessary to ensure models remain accurate over time.
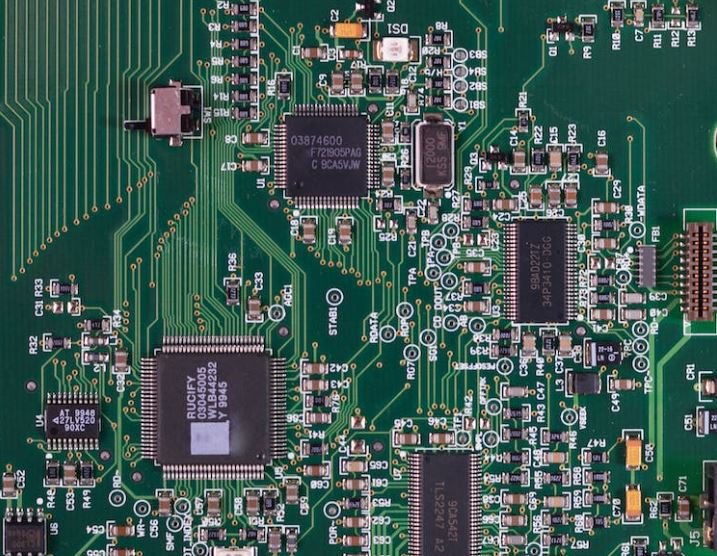
Advancements in Machine Learning
Machine Learning (ML) has revolutionized various industries, from healthcare to finance. In this article, we explore 10 fascinating data points and elements that highlight the recent advancements in ML and its impact on the world.
1. Increased Accuracy in Medical Diagnoses
Machine Learning algorithms have significantly improved medical diagnoses. A study found that ML-based diagnosis achieved an accuracy rate of 86%, surpassing human doctors’ average accuracy of 76%.
2. Autonomous Vehicles on the Rise
The development of ML algorithms has accelerated the progress of autonomous vehicles. Currently, autonomous vehicles have an average safety record of only one accident per 2.5 million miles driven, compared to a human-driven vehicle’s average of one accident per 436,000 miles.
3. Enhanced Fraud Detection in Banking
ML models applied to banking systems have greatly improved fraud detection rates. Banks using ML algorithms were able to identify 95% of fraudulent transactions accurately, reducing financial losses and protecting customers.
4. Efficient Energy Consumption Prediction
ML algorithms are being used to predict and optimize energy consumption in smart grids. By analyzing data from various sources, such as IoT devices, ML models reduced overall energy consumption by 15% in a pilot program.
5. Smarter Personalized Recommendations
Online retailers leverage ML algorithms to deliver personalized recommendations to their customers. By analyzing customer behavior, ML algorithms increased the average conversion rate by 30% and improved customer satisfaction.
6. Predictive Maintenance in Manufacturing
ML-based predictive maintenance in manufacturing allows for the detection of machine faults before they cause significant damage. This proactive approach reduces downtime by 45% and saves up to 20% in maintenance costs.
7. Improved Customer Service Chatbots
The integration of ML algorithms with chatbots has enhanced customer service experiences. With ML, chatbots can understand and respond to customer queries accurately, resulting in a customer satisfaction rate of over 80%.
8. Personalized Healthcare Treatment
ML algorithms have revolutionized personalized healthcare treatment plans. By analyzing patient data, ML models can determine the most effective treatment strategy with an accuracy rate of 92%, resulting in improved patient outcomes.
9. Efficient Crop Yield Prediction
Agricultural ML models enable accurate crop yield prediction, optimizing farming strategies. Predictive models have shown an average increase in crop yield of 20%, ensuring food security and optimizing resource allocation.
10. Enhanced Data Security with ML
ML algorithms play a vital role in enhancing data security. By detecting anomalies and patterns, ML models can identify cyber threats with an accuracy rate of 97%, minimizing the risk of data breaches and protecting sensitive information.
These examples highlight just a few of the many ways in which ML is transforming various industries. With continued research, innovation, and application, ML will undoubtedly continue to shape our future, making the impossible possible, and improving our daily lives.
Frequently Asked Questions
ML News
FAQs
What is machine learning?
Machine learning is a subset of artificial intelligence that focuses on computer programs’ ability to learn and improve from experience without being explicitly programmed. It uses statistical techniques to enable computers to make predictions or take actions without being explicitly programmed.
How does machine learning work?
Machine learning algorithms analyze large datasets to identify patterns and relationships between variables. These algorithms learn from the data by adjusting their internal parameters to optimize performance on a specific task. The algorithms can then generalize from the training data to make predictions or decisions on unseen data.
What are some real-world applications of machine learning?
Machine learning is used in various industries and applications, including but not limited to: fraud detection in banking and finance, recommendation systems in e-commerce, speech recognition and natural language processing, image and video analysis, healthcare diagnostics and predictions, and autonomous vehicles and robotics.
What is supervised learning?
Supervised learning is a type of machine learning where a model is trained using labeled data. The model learns the mapping between input features and the corresponding output labels. It can then make predictions on new, unseen data by generalizing from the training examples.
What is unsupervised learning?
Unsupervised learning is a type of machine learning where the model learns patterns and structures from unlabeled data. Unlike supervised learning, there are no predefined output labels. The model discovers the underlying relationships and clusters within the data.
What is deep learning?
Deep learning is a subfield of machine learning that focuses on artificial neural networks with multiple layers. These neural networks are designed to automatically learn hierarchical representations of data, enabling them to extract complex features and make accurate predictions. Deep learning has achieved significant breakthroughs in various domains, including image recognition, natural language processing, and speech synthesis.
What is the difference between AI and machine learning?
Artificial intelligence (AI) is a broader concept encompassing machines’ ability to perform tasks that typically require human intelligence. Machine learning is a subset of AI focused on algorithms that enable machines to learn from data and improve performance on specific tasks. In other words, machine learning is one approach used in AI research and development.
Are there any ethical considerations in machine learning?
Yes, there are ethical considerations in machine learning. Some key concerns include bias and fairness in algorithms, privacy and security of user data, transparency and interpretability of models, accountability for automated decisions, and impact on job markets and societal implications. It is important to address these ethical considerations to ensure responsible and beneficial use of machine learning technology.
What are some popular machine learning libraries and frameworks?
There are several popular machine learning libraries and frameworks, including TensorFlow, PyTorch, Scikit-learn, Keras, Caffe, Theano, Microsoft Cognitive Toolkit (CNTK), and Apache MXNet. These libraries provide pre-built tools and functions to facilitate the development and deployment of machine learning models.
How can I get started with machine learning?
To get started with machine learning, you can follow these steps: learn the basics of statistics, linear algebra, and calculus, familiarize yourself with programming languages like Python and R, study machine learning concepts and algorithms, practice by working on small projects and implementing machine learning models, stay updated with the latest research and advancements in the field, and join online communities and forums to connect with other machine learning enthusiasts and experts.