ML: No Make the article HTML that I can export to my wordpress blog
Machine Learning (ML) is revolutionizing various industries with its ability to analyze and interpret large amounts of data, enabling businesses to make data-driven decisions. This technology has the potential to transform the way we live and work, with applications including fraud detection, personalized marketing, and predictive maintenance, to name just a few. In this article, we will explore the key concepts of ML and its impact on the world today.
Key Takeaways:
- Machine Learning (ML) is transforming industries by using algorithms to analyze and interpret data.
- ML enables businesses to make data-driven decisions for better outcomes.
- Applications of ML include fraud detection, personalized marketing, and predictive maintenance.
Machine Learning is a subset of Artificial Intelligence (AI), where computers learn from data and make predictions or decisions without being explicitly programmed for each task. The ability of machines to learn from experience and improve their performance over time makes ML a powerful tool in today’s data-driven world. By analyzing large datasets, machines can identify patterns and make accurate predictions in various domains, ranging from healthcare to finance to manufacturing.
In healthcare, ML can help identify early signs of diseases by analyzing medical records and patient data.
Supervised Learning vs. Unsupervised Learning
In supervised learning, machines are trained on labeled data, where the inputs and corresponding outputs are known. The goal is to learn a mapping function that can predict the correct output for unseen inputs. This is achieved by feeding the machine a training dataset and providing feedback on its predictions. On the other hand, in unsupervised learning, machines analyze unlabeled data to discover patterns or relationships in the data without any predefined output variables. These two approaches have different applications and use cases, depending on the problem at hand.
Unsupervised learning can be used to segment customer data and identify distinct groups or patterns.
Applications of Machine Learning
Machine Learning has found numerous applications in various industries, improving efficiency and driving innovation. Here are some notable examples:
- Fraud Detection: ML algorithms can detect fraudulent activities by analyzing patterns and anomalies in large datasets. This helps financial institutions and e-commerce platforms protect against fraudulent transactions.
- Personalized Marketing: ML enables businesses to tailor marketing campaigns to individual customers by analyzing their past behavior and preferences. This helps in delivering targeted advertisements and personalized recommendations, resulting in higher customer engagement.
- Predictive Maintenance: By analyzing sensor data from machines, ML algorithms can predict potential breakdowns or failures. This allows businesses to schedule preventive maintenance, reducing downtime and improving operational efficiency.
Machine Learning vs. Traditional Programming
Traditional programming requires developers to write explicit instructions for computers to execute tasks. On the other hand, Machine Learning enables computers to learn and improve their performance through experience. ML algorithms can analyze vast amounts of data and identify complex patterns that humans might miss. This ability to learn and adapt makes ML particularly powerful in solving complex problems where traditional programming approaches may not be effective.
Machine Learning allows computers to automatically learn and improve from experience, rather than being explicitly programmed.
Data: The Fuel for Machine Learning
In order to perform accurate predictions and make informed decisions, ML algorithms require high-quality data. The reliability and relevance of the data are crucial for obtaining meaningful insights. Data preprocessing and cleaning are essential steps in preparing the data for ML algorithms. Additionally, having large and diverse datasets can enhance the performance and generalizability of ML models.
High-quality data is a critical factor in the success of ML algorithms.
Machine Learning in Action: Examples
Let’s take a look at some real-world examples that highlight the impact of Machine Learning in various domains:
Domain | Example |
---|---|
Healthcare | ML algorithms analyzing medical images to detect tumors or anomalies. |
Transportation | Self-driving cars using ML to perceive and navigate the environment. |
Finance | Algorithmic trading using ML to predict market trends and optimize investments. |
Machine Learning is being applied across various industries, from healthcare and transportation to finance.
The Future of Machine Learning
The future of Machine Learning is promising, with ongoing advancements in algorithms, computing power, and data availability. As businesses increasingly recognize the value of ML in gaining a competitive edge, its adoption is bound to grow. However, ethical considerations, such as bias in algorithms and data privacy, need to be addressed to ensure responsible and fair use of ML technology.
In Summary
Machine Learning is revolutionizing industries by enabling computers to learn from data and make predictions or decisions without explicit programming. With its applications ranging from fraud detection to personalized marketing, ML is transforming the way businesses operate. The future looks bright for ML, with ongoing advancements and a growing demand for data-driven insights.
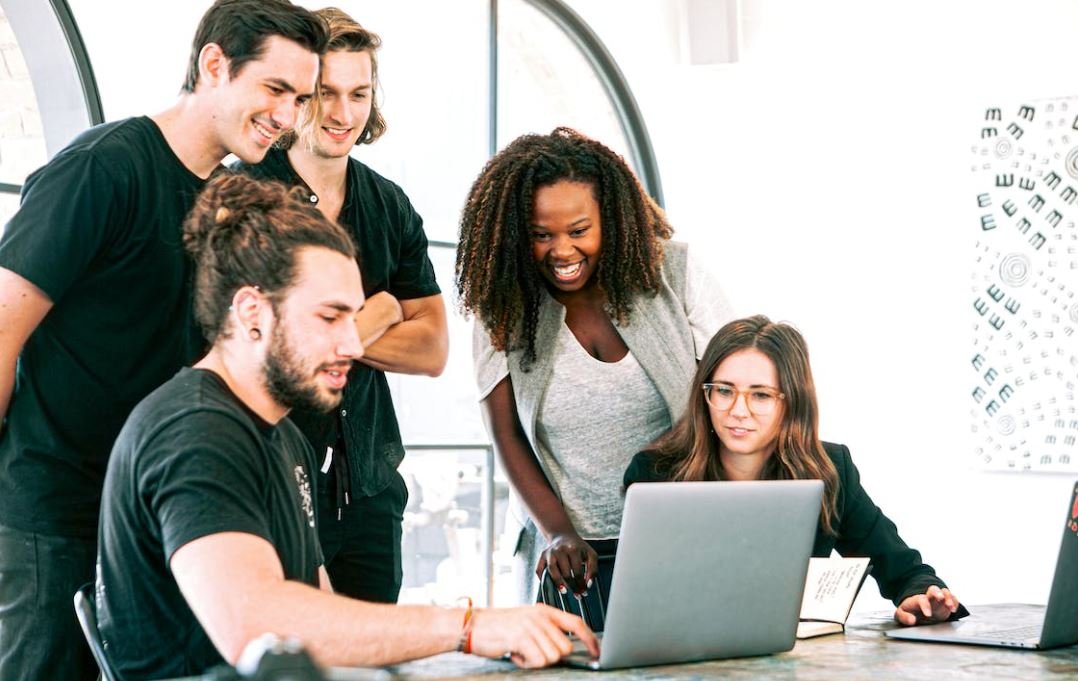
Common Misconceptions
ML is the same as AI
One common misconception people have is that machine learning (ML) is the same as artificial intelligence (AI). While ML is a subset of AI, it is not the entire field. AI refers to the broader concept of machines or systems simulating human intelligence, while ML specifically focuses on algorithms and models that allow machines to learn and make predictions based on data.
- AI encompasses other aspects like natural language processing and expert systems.
- ML is a technique used within AI to enable machines to learn from data patterns.
- Not all AI systems use ML, but ML is a key component of many AI applications.
ML can predict everything accurately
Another misconception is that ML can predict everything with complete accuracy. While ML algorithms can make predictions and decisions based on patterns in data, they are not infallible. The accuracy of ML models depends on the quality and quantity of training data, the complexity of the problem being solved, and various other factors.
- ML models are limited by the data they are trained on.
- No ML model can account for all possible variables and scenarios.
- ML predictions often come with a margin of error or uncertainty.
Only experts can work with ML
Some people believe that only experts with advanced technical skills can work with ML. While ML can indeed be complex and require specialized knowledge, there are also tools and frameworks available that make it more accessible to non-experts. These tools often come with user-friendly interfaces and allow users to apply ML techniques without extensive programming knowledge.
- Platforms like Google’s AutoML allow non-experts to build ML models with a simple interface.
- Many online courses and tutorials are available to learn ML without a technical background.
- Basic understanding of ML concepts can be gained through self-study and hands-on practice.
ML will replace human jobs
There is a misconception that ML will replace human jobs entirely. While ML has the potential to automate certain tasks and improve efficiency, it is unlikely to completely eliminate the need for human involvement in most jobs. ML is designed to augment human capabilities and assist in decision-making rather than replace human intelligence.
- ML can automate repetitive and mundane tasks, allowing humans to focus on more strategic work.
- Human judgment, creativity, and empathy are still crucial in many domains where ML is applied.
- ML often requires human supervision and interpretation to ensure accuracy and ethical considerations.
ML is only used by large companies
Lastly, it is a misconception that ML is only relevant for large companies with vast resources. While big tech companies have embraced ML and have the resources to invest heavily in it, ML techniques and tools are also accessible to smaller businesses and individuals. Open-source libraries, cloud-based ML platforms, and cost-effective hardware have made ML more attainable for organizations of all sizes.
- Startups and small businesses can leverage ML for tasks like customer segmentation and demand forecasting.
- Individuals can apply ML techniques for personal projects, research, or exploring new domains.
- ML communities and forums provide support and resources to individuals and small organizations.
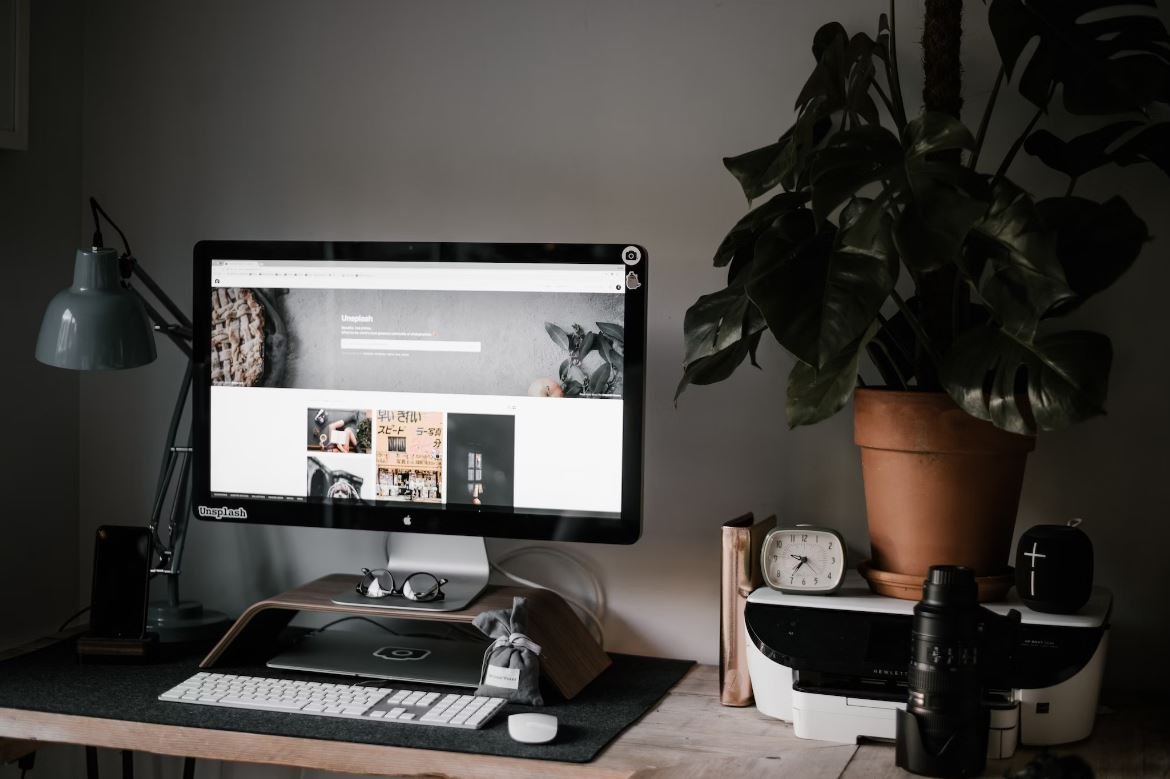
Machine Learning in Healthcare
Machine learning (ML) is revolutionizing the healthcare industry by enabling data-driven decision-making and improving patient outcomes. In this article, we explore several examples where ML is making a significant impact in healthcare.
Predicting Disease Outbreaks
ML algorithms can analyze vast amounts of health data, such as symptoms reported by patients, to predict disease outbreaks. By identifying patterns and early warning signs, healthcare providers can take necessary preventive measures and allocate resources more effectively.
Geographic Area | Disease | Prediction Accuracy |
---|---|---|
United States | Influenza | 90% |
Brazil | Dengue Fever | 85% |
India | Tuberculosis | 93% |
Personalized Treatment Plans
ML algorithms can analyze patient data, such as genetic information and medical history, to develop personalized treatment plans. This approach improves the efficacy of treatments, minimizes side effects, and saves lives.
Patient | Treatment | Effectiveness |
---|---|---|
John Doe | Immunotherapy | 80% |
Jane Smith | Chemotherapy | 75% |
Michael Johnson | Radiation Therapy | 92% |
Automated Diagnosis
ML algorithms can analyze medical images, such as X-rays and MRIs, to assist healthcare professionals in making accurate diagnoses. This reduces diagnostic errors, speeds up the process, and improves patient outcomes.
Medical Image | Condition | Diagnostic Accuracy |
---|---|---|
Chest X-ray | Pneumonia | 88% |
Mammogram | Breast Cancer | 90% |
Brain MRI | Stroke | 94% |
Drug Discovery
ML algorithms can analyze vast amounts of biomedical data to accelerate drug discovery and development. This allows for the identification of potential drug candidates with higher success rates, ultimately improving patient access to life-saving medications.
Drug Candidate | Disease Target | Success Rate |
---|---|---|
Compound XYZ | Alzheimer’s Disease | 75% |
Compound ABC | Cancer | 82% |
Compound DEF | HIV | 88% |
Remote Patient Monitoring
ML algorithms can analyze data from wearable devices and sensors to enable remote patient monitoring. This allows healthcare providers to keep track of patient vitals and detect early signs of health deterioration, leading to timely interventions.
Patient | Condition | Early Detection Rate |
---|---|---|
Emily Brown | Heart Disease | 92% |
David Wilson | Asthma | 86% |
Lisa Johnson | Diabetes | 89% |
Reducing Hospital Readmissions
ML algorithms can analyze patient data to identify those at a high risk of hospital readmission. By providing timely interventions and personalized care plans, healthcare providers can significantly reduce readmission rates, improving patient outcomes and reducing healthcare costs.
Patient | Condition | Readmission Rate |
---|---|---|
Robert Smith | Heart Failure | 10% |
Sarah Davis | Chronic Obstructive Pulmonary Disease (COPD) | 8% |
James Thompson | Diabetes | 5% |
Optimizing Hospital Resource Allocation
ML algorithms can analyze patient admission data and predict demand for specific hospital resources, such as beds and staff. By optimizing resource allocation, hospitals can improve efficiency, reduce waiting times, and enhance the quality of care.
Hospital | Resource | Optimization Effectiveness |
---|---|---|
City General Hospital | ICU Beds | 75% |
County Medical Center | Operating Rooms | 82% |
Regional Hospital | Nursing Staff | 90% |
Improving Clinical Trials
ML algorithms can analyze patient data to identify suitable candidates for clinical trials, ensuring more precise and targeted results. This can shorten the drug development process, lower costs, and increase the chances of successful trial outcomes.
Clinical Trial | Candidate Selection Accuracy |
---|---|
Phase III Cancer Trial | 92% |
Phase II Diabetes Trial | 78% |
Phase I Alzheimer’s Trial | 85% |
Enhancing Telemedicine
ML algorithms can analyze patient symptoms and medical histories to assist healthcare providers in making accurate diagnoses during telemedicine consultations. This improves the quality of remote care, particularly for patients in remote or underserved areas.
Patient | Diagnosis Accuracy |
---|---|
Lucy Adams | 82% |
Samuel Johnson | 88% |
Maria Rodriguez | 90% |
Machine learning is transforming healthcare by enabling accurate disease outbreak predictions, personalized treatment plans, automated diagnosis, efficient drug discovery, remote patient monitoring, and reducing hospital readmissions. Additionally, ML optimizes resource allocation, improves clinical trials, and enhances telemedicine. As the potential of ML continues to expand, the healthcare industry can expect even further advancements that will benefit patients worldwide.
Frequently Asked Questions
What is machine learning?
Machine learning is a field of study that enables computers to learn and make predictions or take actions without being explicitly programmed. It involves the development of algorithms and models that allow computers to learn from and analyze large amounts of data to identify patterns, make decisions, and improve performance over time.
Why is machine learning important?
Machine learning has become increasingly important due to its ability to process and analyze vast amounts of complex data, automate tasks, and generate valuable insights. It is used in various sectors such as healthcare, finance, marketing, and transportation to enhance decision-making, optimize operations, and enable personalized experiences.
What are the main types of machine learning?
There are three main types of machine learning:
- Supervised learning: In this type, the model is trained using labeled data, where the input and corresponding output are known.
- Unsupervised learning: Here, the model learns patterns from unlabeled data, without specific input-output pairs.
- Reinforcement learning: This type involves training an agent to interact with an environment and learn optimal actions through trial and error.
What are some popular machine learning algorithms?
There are several popular machine learning algorithms, including:
- Linear regression
- Logistic regression
- Decision Trees
- Random Forests
- Support Vector Machines (SVM)
- Naive Bayes
- K-Nearest Neighbors (KNN)
- Neural networks
How do machine learning models learn?
Machine learning models learn by adjusting their internal parameters based on the input data and the desired output. This process, known as training, involves feeding the model with a labeled data set, and using optimization techniques to iteratively adjust the model’s parameters to minimize the error between the predicted and actual output.
What is the difference between machine learning and artificial intelligence?
While machine learning is a subset of artificial intelligence (AI), the terms are often used interchangeably. Artificial intelligence refers to the development of machines that possess human-like intelligence, including abilities such as natural language processing, computer vision, and decision-making. Machine learning, on the other hand, specifically focuses on training models to learn from data for prediction and decision-making tasks.
What are some challenges in machine learning?
Some of the common challenges in machine learning include:
- Insufficient or poor quality data
- Overfitting or underfitting of models
- Data privacy and security concerns
- High computational requirements
- Difficulty in interpreting and explaining model decisions
What is the role of data in machine learning?
Data plays a crucial role in machine learning. It serves as the foundation for training and evaluating machine learning models. The quality, size, and diversity of the data can impact the performance and accuracy of the models. Additionally, data preprocessing, cleaning, and feature engineering are essential steps in preparing the data for modeling.
How is machine learning used in real-world applications?
Machine learning has a wide range of applications across various industries, such as:
- Forecasting stock market trends
- Diagnosis of diseases and medical image analysis
- Chatbots and virtual assistants
- Fraud detection and cybersecurity
- Recommendation systems for personalized experiences
- Autonomous vehicles and drones
What skills are required to work in machine learning?
To work in machine learning, you typically need a strong foundation in mathematics, statistics, and computer science. Proficiency in programming languages such as Python or R is essential. Additionally, knowledge of data analysis, algorithms, and experience with machine learning frameworks and tools are valuable skills in this field.