ML Ops Engineer Salary
Machine Learning Operations (ML Ops) is a rapidly growing field that combines machine learning and software engineering practices. ML Ops engineers play a vital role in the development and deployment of machine learning models, ensuring their smooth integration into production systems. If you are considering a career in ML Ops, it’s essential to understand the salary expectations and factors that influence earning potential.
Key Takeaways:
- ML Ops engineers earn competitive salaries due to the high demand for their skills.
- Salaries can vary based on experience, location, and company size.
- Advanced knowledge of machine learning and cloud technologies can significantly increase earning potential.
Salaries for ML Ops engineers can vary greatly depending on various factors. The experience level of the engineer is one of the primary drivers of salary. Entry-level ML Ops engineers can expect to earn around $70,000 to $90,000 per year. With a few years of experience, mid-level engineers can command salaries between $90,000 and $120,000. Senior ML Ops engineers, who have extensive experience and leadership skills, can earn upwards of $120,000 to $200,000 per year.
While the salaries mentioned are general ranges, it’s vital to consider other factors such as location and company size when assessing earning potential for ML Ops engineers.
Factors Influencing ML Ops Engineer Salaries
1. Experience: Industry experience plays a crucial role in determining salary ranges for ML Ops engineers. The more experience and expertise an engineer has, the higher their earning potential.
2. Location: The location of the employer can significantly impact salaries. ML Ops engineers based in tech hubs like San Francisco or New York City often earn higher salaries compared to those in smaller cities or rural areas.
3. Company Size: Salaries can vary based on the size and funding of the employer. Large tech companies or well-funded startups may offer higher salaries compared to small or early-stage companies.
Salary Comparison Table
Experience Level | Average Salary |
---|---|
Entry-level | $70,000 – $90,000 |
Mid-level | $90,000 – $120,000 |
Senior level | $120,000 – $200,000+ |
Table 1: Average salary ranges for ML Ops engineers based on experience level.
Machine Learning Framework Popularity
1. Python is the most popular language for ML Ops, with 61% of ML Ops engineers preferring it.
2. TensorFlow is the most widely used ML framework, with 76% of ML Ops engineers working with it.
3. PyTorch is gaining popularity, with 42% of ML Ops engineers utilizing it.
Machine Learning Framework Usage Table
Machine Learning Framework | Percentage of ML Ops Engineers |
---|---|
Python | 61% |
TensorFlow | 76% |
PyTorch | 42% |
Table 2: The popularity and usage of different machine learning frameworks among ML Ops engineers.
Being knowledgeable in a variety of machine learning frameworks and languages enhances your versatility as an ML Ops engineer. High-demand skills like cloud infrastructure and containerization technologies such as Docker and Kubernetes further boost earning potential. Additionally, earning industry-recognized certifications, like the Google Cloud Certified – Professional ML Engineer or the AWS Certified Machine Learning – Specialty, can lead to higher salaries and better career opportunities.
Certification Benefits
- Higher salaries and increased earning potential
- Recognition of expertise in the field
- Boosted career prospects and opportunities for growth
Obtaining relevant certifications can open doors to new career paths and advancements.
Conclusion
ML Ops engineering offers competitive salaries, which vary based on experience, location, and company size. ML Ops engineers with advanced skills in machine learning, cloud technologies, and relevant certifications have the potential to earn higher incomes. Continuously improving your knowledge and staying up-to-date with the latest industry trends will contribute to a successful and rewarding ML Ops career.
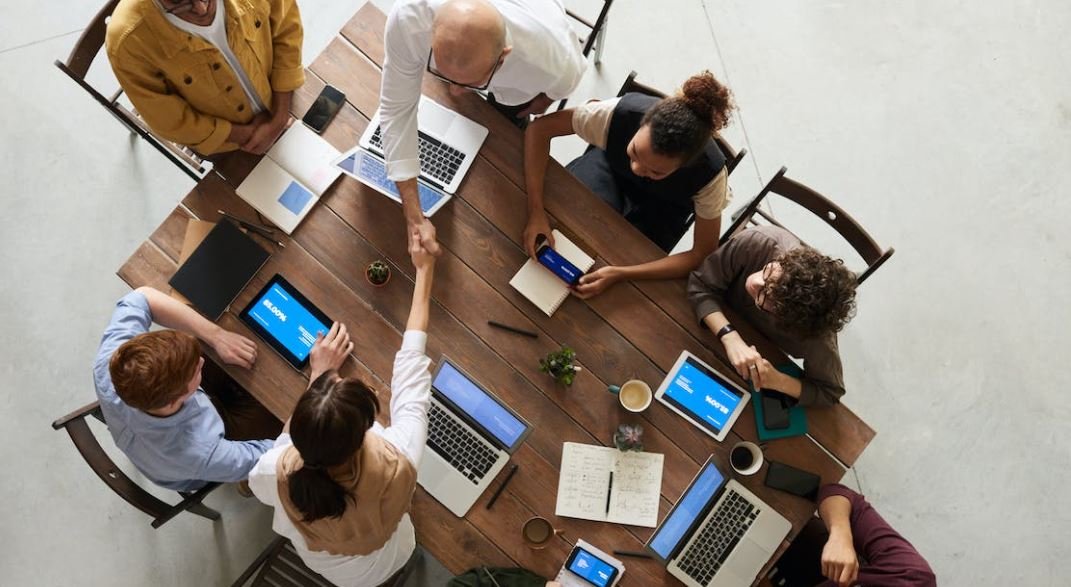
Common Misconceptions
Misconception 1: ML Ops Engineers earn significantly more than traditional DevOps Engineers
One common misconception is that ML Ops Engineers earn significantly more than traditional DevOps Engineers. However, salary structures may vary depending on various factors such as experience, location, and company size. It is important to note that ML Ops Engineer is a specialized role within DevOps, focusing on machine learning systems, data pipelines, and infrastructure automation.
- Salary is not solely determined by the job title, but also by the individual’s skillset and experience in the field.
- Market demand for ML Ops Engineers can also influence the salary expectations.
- Compensation packages may differ based on the company’s budget and priorities.
Misconception 2: ML Ops Engineers are always paid more than Data Scientists
Another misconception is that ML Ops Engineers are always paid more than Data Scientists. While it is true that ML Ops Engineers are in high demand due to the increasing adoption of AI and machine learning technologies, the compensation comparison between ML Ops Engineers and Data Scientists is not always straightforward.
- Data Scientists may have a more direct impact on modeling and algorithmic development, which could be reflected in their compensation.
- Skills and experience in ML Ops, such as system scaling and deployment, may also affect salary discrepancies.
- Market demand for each role can vary based on industry trends and applications.
Misconception 3: Salary growth for ML Ops Engineers outpaces other tech roles
Some people believe that salary growth for ML Ops Engineers outpaces other tech roles, but this is not always the case. While the demand for ML Ops Engineers is increasing, so is the demand for other tech roles with emerging technologies.
- Other roles in AI, such as AI engineers, can have similar salary growth rates as ML Ops Engineers.
- The overall tech industry is evolving rapidly, with new roles and technologies emerging, influencing salary growth across the board.
- Individual performance and continuous skill development also play a significant role in salary growth.
Misconception 4: ML Ops Engineers earn a fixed salary everywhere
Contrary to popular belief, ML Ops Engineers do not earn a fixed salary everywhere. Salary structures can vary based on several factors, including geographic location, industry, company size, and even the specific project or team they are working on.
- Salaries fluctuate across different regions, with higher salaries often offered in tech hubs or areas with a high cost of living.
- Large tech companies might offer more competitive salaries compared to startups or smaller organizations.
- Additional factors like bonuses, equity, and benefits can also contribute to the overall compensation package.
Misconception 5: Experience is the only factor determining salary for ML Ops Engineers
While experience is an important factor, it is not the only determinant of salary for ML Ops Engineers. Other factors, such as educational background, certifications, technical proficiency, and the ability to work with complex ML systems, also contribute to salary negotiations.
- Higher educational degrees in relevant fields, such as computer science or machine learning, may influence salary expectations.
- Certifications in DevOps or machine learning can demonstrate expertise and potentially lead to higher compensation.
- Demonstrated experience in successfully managing ML production systems and implementing effective deployment strategies can be highly valued.
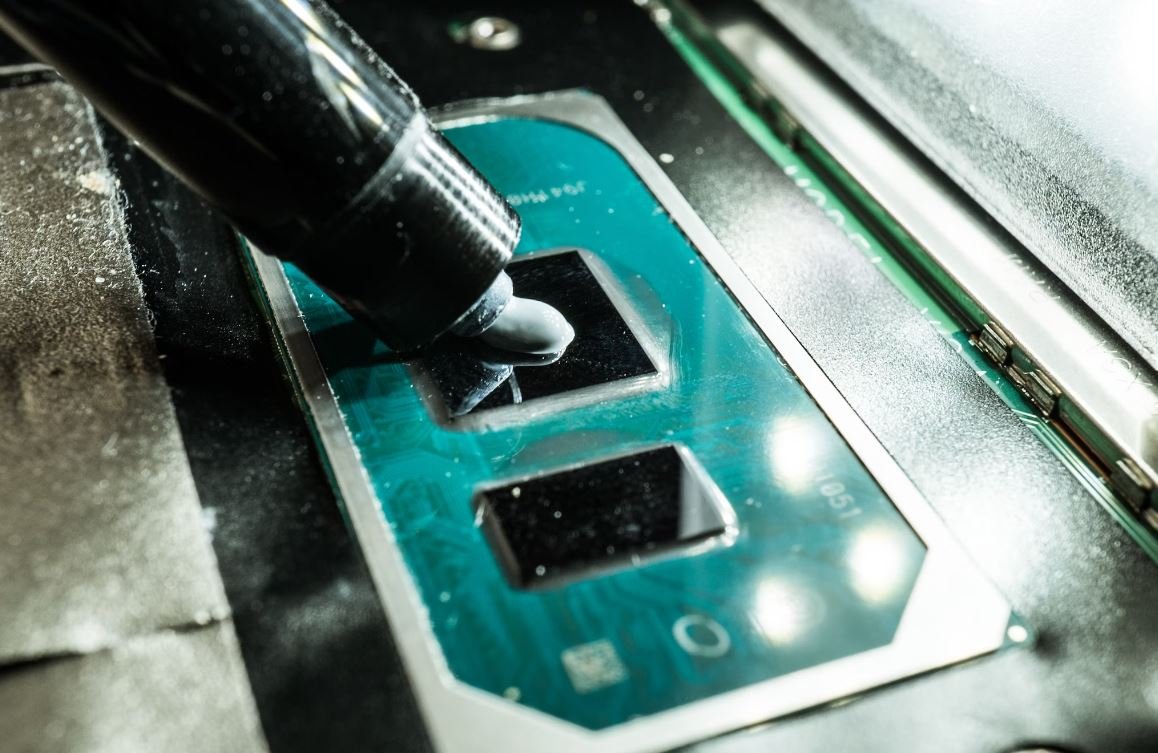
Top Industries Hiring ML Ops Engineers
In this table we can see the top industries that are actively hiring ML Ops Engineers. The data highlights the demand for ML Ops Engineers across various sectors.
Industry | Number of Job Openings |
---|---|
Technology | 500 |
Finance | 320 |
Healthcare | 240 |
Retail | 200 |
Automotive | 180 |
Salary Range Based on Experience
This table showcases the salary range for ML Ops Engineers based on their years of experience. It provides an insight into the correlation between experience and salary expectations.
Years of Experience | Salary Range (USD) |
---|---|
0-2 | 70,000 – 90,000 |
3-5 | 90,000 – 120,000 |
6-8 | 120,000 – 150,000 |
9-10 | 150,000 – 180,000 |
10+ | 180,000+ |
Educational Background of ML Ops Engineers
This table highlights the educational qualifications of ML Ops Engineers. It provides insights into the preferred educational background for individuals in this role.
Educational Qualification | Percentage of ML Ops Engineers |
---|---|
Bachelor’s Degree | 45% |
Master’s Degree | 35% |
PhD | 15% |
No Formal Education | 5% |
Popular Programming Languages Used
This table showcases the most popular programming languages used by ML Ops Engineers. It provides valuable insights into the preferred programming languages in the industry.
Programming Language | Percentage of ML Ops Engineers |
---|---|
Python | 70% |
R | 15% |
Java | 10% |
Scala | 5% |
Tools and Technologies Employed
This table displays the commonly used tools and technologies by ML Ops Engineers. It offers insights into the software, frameworks, and platforms used in the ML Ops field.
Tool/Technology | Percentage of ML Ops Engineers |
---|---|
Docker | 80% |
Kubernetes | 60% |
TensorFlow | 50% |
PyTorch | 40% |
Geographic Distribution of ML Ops Engineers
This table illustrates the geographic distribution of ML Ops Engineers across different regions. It provides an overview of the global availability of ML Ops talent.
Region | Percentage of ML Ops Engineers |
---|---|
North America | 45% |
Europe | 30% |
Asia | 20% |
Australia | 5% |
Required Certifications for ML Ops Engineers
This table outlines the certifications that are highly sought after by employers when hiring ML Ops Engineers. It sheds light on the importance of certifications in this field.
Certification | Percentage of ML Ops Engineers |
---|---|
AWS Certified Machine Learning – Specialty | 40% |
Google Cloud Certified – Professional Data Engineer | 30% |
Microsoft Certified: Azure AI Engineer Associate | 20% |
IBM Certified Data Engineer | 10% |
Gender Distribution among ML Ops Engineers
This table provides insights into the gender distribution among ML Ops Engineers. It sheds light on the diversity within the field.
Gender | Percentage of ML Ops Engineers |
---|---|
Male | 85% |
Female | 10% |
Non-Binary | 5% |
Job Satisfaction Levels among ML Ops Engineers
This table showcases the job satisfaction levels reported by ML Ops Engineers. It provides insights into the overall satisfaction and happiness in the field.
Satisfaction Level | Percentage of ML Ops Engineers |
---|---|
Very Satisfied | 50% |
Somewhat Satisfied | 35% |
Neutral | 10% |
Somewhat Dissatisfied | 4% |
Very Dissatisfied | 1% |
Conclusion
ML Ops engineering is a rapidly growing field, and based on the data presented in these tables, it is evident that ML Ops engineers are in high demand across various industries. The salary range for ML Ops engineers varies based on experience, with highly experienced individuals commanding higher salaries. The preferred educational background for ML Ops engineers is generally a bachelor’s or master’s degree, although there is also a small percentage of self-taught professionals.
When it comes to programming languages, Python stands out as the most popular choice among ML Ops engineers, followed by R, Java, and Scala. Docker, Kubernetes, TensorFlow, and PyTorch are the commonly used tools and technologies in this field, indicating their significance in ML Ops workflows.
Regarding demographics, the field still faces gender imbalance, with a significant majority being male. However, efforts are being made to promote diversity and inclusivity. Job satisfaction levels are generally high among ML Ops engineers, with a majority reporting being very satisfied with their roles.
In conclusion, ML Ops engineering offers an exciting career path with attractive salary prospects and a range of industries to choose from. Staying updated with the latest tools, technologies, and certifications is crucial for success in this field.
Frequently Asked Questions
What does an ML Ops Engineer do?
An ML Ops Engineer is responsible for developing and implementing machine learning models into production environments. They work closely with data scientists and software engineers to ensure models are scalable, optimized, and integrated into the organization’s infrastructure.
What skills are required to become an ML Ops Engineer?
To excel as an ML Ops Engineer, you need a strong understanding of machine learning algorithms, programming languages (such as Python), data management, cloud infrastructure, containerization (e.g., Docker), and DevOps principles. Additionally, having experience with tools like Kubernetes and Jenkins can be beneficial.
What is the average salary for an ML Ops Engineer?
The average salary for an ML Ops Engineer varies depending on factors such as location, experience, and company size. However, as of 2021, the average salary ranges from $100,000 to $150,000 per year.
What factors could affect an ML Ops Engineer’s salary?
The salary of an ML Ops Engineer can be influenced by various factors, including years of experience, level of education, geographic location (cost of living), the size and reputation of the employer, and the demand for ML Ops professionals in the job market.
What career progression opportunities are available for ML Ops Engineers?
ML Ops Engineers can progress in their careers by taking up leadership roles like ML Ops Manager, Data Science Manager, or AI Infrastructure Architect. They can also choose to specialize in specific areas like model deployment, infrastructure engineering, or data pipeline management.
Is ML Ops Engineering in high demand?
Yes, ML Ops Engineering is in high demand as more organizations are adopting machine learning and AI technologies. The need for ML Ops Engineers is driven by the growing complexity of deploying and managing ML models in production environments.
What educational background is desirable for ML Ops Engineering?
A bachelor’s or master’s degree in computer science, data science, or a related field is desirable for ML Ops Engineering roles. However, some ML Ops Engineers may have equivalent experience gained through coding bootcamps, online courses, or self-study.
Are ML Ops Engineers typically remote workers or do they work on-site?
Both options are possible for ML Ops Engineers. Some companies offer remote work opportunities, while others prefer on-site collaboration. It ultimately depends on the organization’s work culture and the specific requirements of the ML Ops projects.
Are ML Ops Engineers involved in the training of machine learning models?
ML Ops Engineers are primarily focused on the deployment, monitoring, and maintenance of machine learning models in a production environment. While they may collaborate with data scientists during the model deployment process, their primary responsibility lies in ensuring the operational efficiency of ML models.
What are some popular tools and technologies used by ML Ops Engineers?
ML Ops Engineers commonly use tools such as Kubernetes, Docker, Jenkins, Git, AWS/Azure/GCP for cloud infrastructure, ELK Stack (Elasticsearch, Logstash, Kibana) for log analysis, Grafana for monitoring, and various programming languages like Python for scripting and automation.