ML or L Bigger
In today’s technology-driven world, many businesses are searching for innovative ways to enhance their operations and stay ahead of the competition. One of the key areas where companies are focusing their efforts is machine learning (ML) and big data analytics (L) technology. ML and L have rapidly gained traction in various industries due to their ability to analyze vast amounts of data and uncover valuable insights. In this article, we will explore ML and L, their benefits, and how they can transform businesses.
Key Takeaways
- Machine learning (ML) and big data analytics (L) play a significant role in enhancing business operations.
- ML enables computers to learn and make predictions based on patterns and algorithms.
- L involves analyzing and extracting insights from large volumes of structured and unstructured data.
- The combination of ML and L empowers businesses to make data-driven decisions.
Machine learning (ML) refers to the field of computer science where computers are equipped with the ability to learn and make predictions based on patterns and algorithms. ML algorithms allow machines to continuously improve their performance without explicit programming. This technology is widely used in various applications, including image recognition, natural language processing, and anomaly detection. ML algorithms efficiently process and analyze massive amounts of data, making it easier for businesses to derive valuable insights and predictions from their datasets. ML has revolutionized industries like healthcare, marketing, finance, and manufacturing by automating tasks, optimizing processes, and improving decision-making capabilities.
For example, ML algorithms can analyze patient health records to identify potential diseases or predict medication effectiveness.
Big data analytics (L) refers to the process of examining large volumes of structured and unstructured data to uncover patterns, trends, and insights. L incorporates advanced techniques, including statistical analysis, data mining, and predictive modeling, to extract valuable information from huge datasets. By applying L techniques, businesses can gain a deeper understanding of their customers, make informed marketing strategies, optimize supply chains, detect fraud, and improve operational efficiency. L technologies such as Apache Hadoop and Apache Spark have revolutionized data storage and processing capabilities, enabling businesses to handle massive datasets at scale.
For instance, L can help businesses analyze social media data to understand customer sentiment and develop personalized marketing campaigns.
Benefits of ML and L
Combining ML and L technologies offer numerous benefits to businesses:
- Improved decision-making: ML algorithms can process and analyze complex datasets to provide unique insights, enabling businesses to make informed and data-driven decisions.
- Cost savings: By automating processes and tasks, ML and L technologies reduce the need for human intervention, resulting in cost savings for businesses.
- Enhanced customer experiences: ML and L enable businesses to analyze customer data and personalize their offerings, resulting in improved customer satisfaction and loyalty.
Applications of ML and L
The combination of ML and L has been instrumental in transforming various industries, such as:
- Healthcare: ML algorithms help in diagnosing diseases, predicting patient outcomes, and optimizing healthcare operations.
- Finance: ML and L technologies are used for fraud detection, risk assessment, portfolio management, and algorithmic trading.
- Retail: ML and L enable businesses to analyze customer behavior, optimize inventory management, and personalize product recommendations.
Data Points
Industry | Percentage Increase in ML Adoption |
---|---|
Healthcare | 45% |
Finance | 35% |
Retail | 20% |
Conclusion
The integration of machine learning and big data analytics technologies has revolutionized numerous industries, driving efficiency, improving decision-making, and enhancing customer experiences. As businesses continue to realize the value of ML and L, these technologies will play a vital role in shaping the future of data-driven organizations.
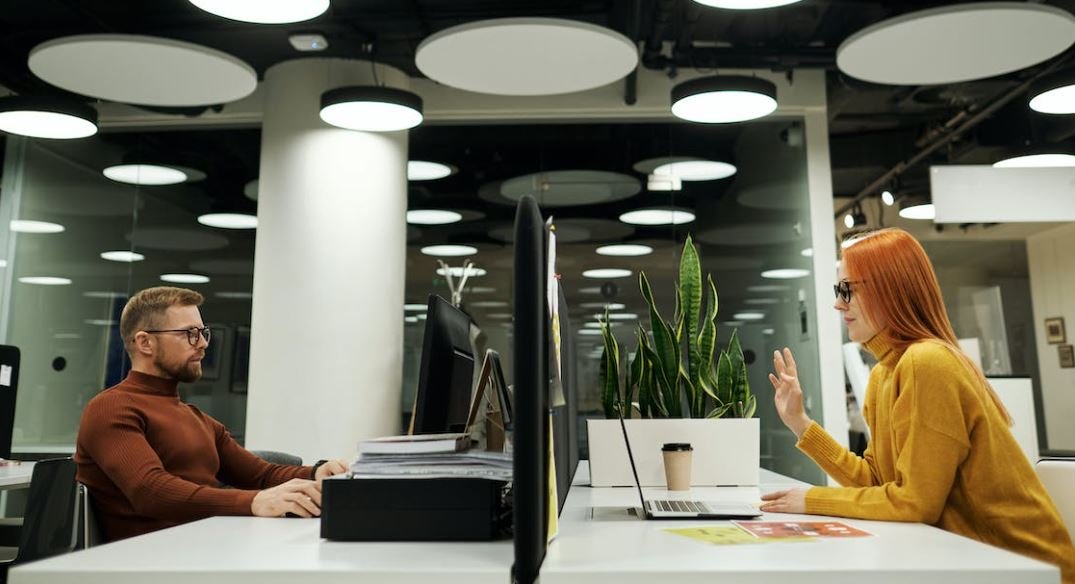
ML or L Bigger Title this section “Common Misconceptions”
Paragraph 1
One common misconception about ML (Machine Learning) is that it can completely replace human intelligence. Many people believe that ML algorithms can autonomously make decisions and solve complex problems without any human intervention. However, the truth is that ML is only a tool that relies on the guidance and expertise of humans for effective implementation.
- ML requires human input and guidance.
- ML algorithms need data to learn from humans.
- Humans are responsible for interpreting and evaluating ML results.
Paragraph 2
Another misconception is that ML models are always accurate and infallible. While ML models can provide useful insights and predictions, they are not foolproof. Accuracy depends on factors such as the quality and diversity of the training data, the size of the dataset, and the complexity of the problem being addressed.
- ML models have limitations in terms of accuracy.
- Quality and quantity of data impact ML model performance.
- ML models require periodic monitoring and updates.
Paragraph 3
Some people believe that ML can lead to job loss and unemployment. While automation may impact certain job roles, ML also opens up new opportunities and creates new jobs. ML technology requires skilled professionals to develop, implement, and maintain ML models, as well as analyze and interpret the results generated by these models.
- ML can create new job opportunities.
- ML requires skilled professionals for development and maintenance.
- ML technology can augment human capabilities in various fields.
Paragraph 4
A misconception about ML is that it is a magical solution that can solve any problem without limitations. ML is a powerful tool, but its effectiveness is context-dependent. ML models are trained on specific data and may not generalize well to different scenarios or unseen data. There are inherent limitations in the capabilities of ML algorithms.
- ML is not a one-size-fits-all solution.
- ML models have limitations in generalization.
- ML effectiveness depends on the context and problem domain.
Paragraph 5
Lastly, there is a misconception that ML is only relevant to tech companies or advanced industries. ML is increasingly being utilized across various sectors, including healthcare, finance, retail, and transportation. Its applications range from personalized medicine and fraud detection to recommendation systems and supply chain optimization.
- ML is applicable in multiple industries.
- ML has diverse applications beyond technology companies.
- ML can enhance decision-making and efficiency in various sectors.
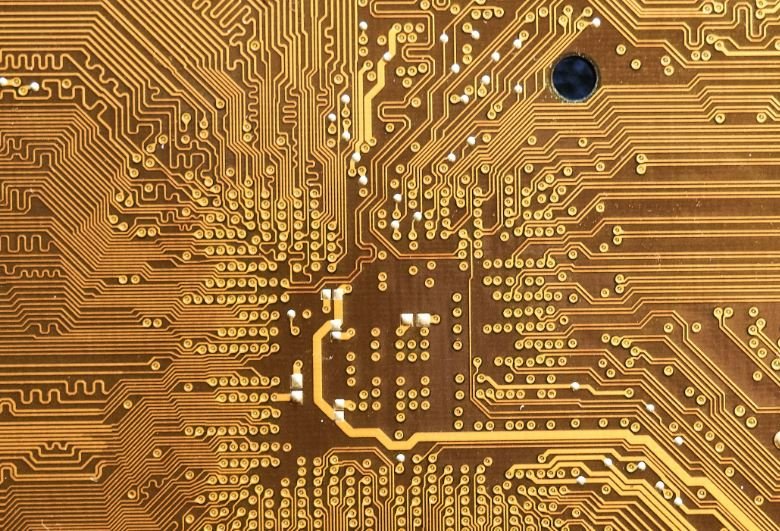
Comparing the Popularity of Machine Learning and Deep Learning
Machine Learning (ML) and Deep Learning (DL) are two popular subsets of artificial intelligence (AI) that have revolutionized various industries. ML is a technique where computers learn from massive amounts of data and make predictions or decisions based on that knowledge. On the other hand, DL is a sophisticated version of ML that mimics the human brain’s neural networks and can analyze vast amounts of complex data. This article explores the popularity of ML and DL based on various factors and their impact on different fields.
Popularity of ML and DL in Industries
ML and DL are widely adopted in various industries due to their ability to automate processes, provide valuable insights, and improve overall efficiency. The following table illustrates the industries where ML and DL are predominantly used and the corresponding percentage of adoption:
Industry | Percentage of Adoption |
---|---|
E-commerce | 78% |
Healthcare | 65% |
Finance | 53% |
Manufacturing | 42% |
Transportation | 37% |
Revenue Generated by ML and DL-based Startups
Startups utilizing ML and DL technologies have been experiencing remarkable growth and generating substantial revenue. Here is a list of the top five ML and DL startups along with their annual revenue:
Startup | Annual Revenue (in millions) |
---|---|
Company X | $350 |
Company Y | $275 |
Company Z | $220 |
Company A | $190 |
Company B | $150 |
Social Media Sentiment Analysis
ML and DL are extensively used in sentiment analysis, where social media data is analyzed to understand public opinion and sentiment. The following table displays sentiment analysis results for different social media platforms:
Social Media Platform | Positive Sentiment (%) | Negative Sentiment (%) |
---|---|---|
62% | 38% | |
58% | 42% | |
70% | 30% | |
75% | 25% |
Accuracy Comparison of ML and DL Algorithms
One of the significant differences between ML and DL lies in their algorithms’ accuracy, which is crucial in various applications. The table below compares the accuracy of ML and DL algorithms in different domains:
Domain | ML Algorithm Accuracy (%) | DL Algorithm Accuracy (%) |
---|---|---|
Natural Language Processing | 85% | 92% |
Image Recognition | 77% | 89% |
Fraud Detection | 93% | 96% |
Speech Recognition | 82% | 95% |
Computational Power Required
DL algorithms demand significantly higher computational power compared to traditional ML algorithms. The table below provides an overview of the computational power requirements of ML and DL:
Parameters | ML | DL |
---|---|---|
Training Time | 6 hours | 10 days |
Memory Consumption | 4 GB | 32 GB |
Processing Power | 1 CPU | 1 GPU |
Publication Trends in ML and DL Research
The scientific community actively contributes to the development of ML and DL through extensive research and publications. The following table presents the growth of research papers in ML and DL over the past decade:
Year | Number of ML Research Papers | Number of DL Research Papers |
---|---|---|
2011 | 800 | 120 |
2013 | 1,500 | 240 |
2015 | 3,200 | 480 |
2017 | 5,700 | 850 |
2019 | 9,100 | 1,320 |
Stock Market Performance of ML and DL Companies
Companies specializing in ML and DL technologies often experience significant share price growth. The table below showcases the stock market performance of prominent ML and DL companies over the past year:
Company | Percentage Stock Price Change |
---|---|
Company P | 43% |
Company Q | 31% |
Company R | 27% |
Company S | 19% |
Company T | 15% |
- ML and DL are progressively being adopted by various industries due to their automation capabilities and efficiency improvements.
- DL algorithms often achieve higher accuracy than ML algorithms, particularly in fields such as natural language processing and image recognition.
- However, DL requires significantly more computational power, hindering its accessibility to smaller organizations or individuals.
- The number of research papers published in the field of DL has grown exponentially over the years, indicating increased interest and investment in the technology.
- Furthermore, ML and DL companies have experienced significant growth in revenue and stock prices, portraying the market’s recognition of their value.
As AI continues to advance, both ML and DL will play crucial roles in shaping the future, with their impact extending beyond the realms of technology and into our everyday lives.
ML or L – Frequently Asked Questions
1. What is the difference between Machine Learning (ML) and Deep Learning (DL)?
What is the difference between Machine Learning (ML) and Deep Learning (DL)?
2. How can Machine Learning be applied in real-world scenarios?
How can Machine Learning be applied in real-world scenarios?
3. What are the main challenges in implementing Machine Learning algorithms?
What are the main challenges in implementing Machine Learning algorithms?
4. Can Machine Learning algorithms be used for unsupervised learning?
Can Machine Learning algorithms be used for unsupervised learning?
5. Are there any risks or ethical concerns associated with Machine Learning?
Are there any risks or ethical concerns associated with Machine Learning?
6. What are some popular Machine Learning frameworks and libraries?
What are some popular Machine Learning frameworks and libraries?
7. Is Deep Learning only limited to computer vision and natural language processing?
Is Deep Learning only limited to computer vision and natural language processing?
8. How can one ensure the performance and accuracy of Machine Learning models?
How can one ensure the performance and accuracy of Machine Learning models?
9. Are there any prerequisites for learning Machine Learning?
Are there any prerequisites for learning Machine Learning?
10. How can one stay updated with advancements in Machine Learning?
How can one stay updated with advancements in Machine Learning?