ML or ML Reddit
Machine Learning (ML) and Reddit—two popular concepts that have gained massive traction in the digital world. But what happens when we combine the two? In this article, we will explore the ML subreddit, also known as r/MachineLearning, and delve into the fascinating discussions, insights, and knowledge shared by its active community of enthusiasts and experts.
Key Takeaways
- ML subreddit (r/MachineLearning) offers a wealth of information on all aspects of machine learning.
- Reddit moderators ensure high-quality content and discussions.
- The subreddit is an invaluable resource for beginners and seasoned ML practitioners.
The ML Subreddit
The ML subreddit, r/MachineLearning, serves as a hub for professionals, researchers, and hobbyists interested in the field of machine learning. With more than 4 million members, this active community shares the latest research papers, innovative algorithms, and practical tips and tricks *to advance the field of ML*.
What to Expect
When you dive into the ML subreddit, be prepared to discover a range of engaging content. From in-depth discussions about the latest breakthroughs in neural networks to tutorials on implementing ML algorithms, this subreddit covers it all. *You might even stumble upon posts revealing unconventional applications of ML, such as predicting the outcome of sporting events based on historical data.*
Community Interaction
One of the remarkable aspects of the ML subreddit is the vibrant community interaction it fosters. Members actively engage in conversations, provide insightful feedback, and share valuable resources with one another. Through comment threads, you’ll find individuals collaborating, debating, and expanding their ML knowledge. *This interactive nature creates an atmosphere of continuous learning and growth.*
Rules and Moderation
The ML subreddit‘s success can be partly attributed to the diligent moderation team that ensures high-quality content and discussions. *From enforcing proper citation practices to encouraging respectful debates, these moderators maintain a positive environment for knowledge exchange.* Additionally, the subreddit has specific guidelines to prevent self-promotion or spammy content, further enhancing the browsing experience for users seeking valuable ML insights.
Finding Answers and Resources
Looking for specific answers or resources? The ML subreddit has you covered. Utilize the search function to find relevant discussions on particular topics, discover curated lists of open-source ML libraries and frameworks, or simply ask the community for help. Whether you are a beginner seeking guidance or an expert searching for the latest research papers, you can rely on the collective expertise of the subreddit’s members *to find the information or resources you need*.
Tables
Table 1 | Table 2 |
---|---|
Data Point 1 | Data Point 2 |
Data Point 3 | Data Point 4 |
Data Point 5 | Data Point 6 |
Continuing Education and Networking
Aside from being a valuable resource for learning, the ML subreddit also serves as a platform for networking. Professionals in the field often connect with one another, share research collaborations, and discuss job opportunities. *By participating in the ML subreddit, you have the chance to expand your professional network and gain exposure to exciting career prospects within the ML community.*
Keeping Up with the Latest
The world of ML is constantly evolving, and staying up to date with the latest advancements is crucial. The ML subreddit provides a convenient solution by curating the most important news, research publications, and conferences in the field. By regularly browsing and engaging with this subreddit, *you can ensure you’re always at the forefront of ML innovation*.
Conclusion
Now that you’re aware of the ML subreddit‘s vibrant community, valuable resources, and interactive nature, it’s time to dive in. Whether you’re a beginner hungry for ML knowledge or an experienced practitioner looking to expand your expertise, the ML subreddit is an excellent online space to explore. *Join the active discussions, learn from experts, and contribute to the ever-evolving world of machine learning.*
Tables
Table 1 | Table 2 |
---|---|
Data Point 1 | Data Point 2 |
Data Point 3 | Data Point 4 |
Data Point 5 | Data Point 6 |
Tables
Table 1 | Table 2 |
---|---|
Data Point 1 | Data Point 2 |
Data Point 3 | Data Point 4 |
Data Point 5 | Data Point 6 |
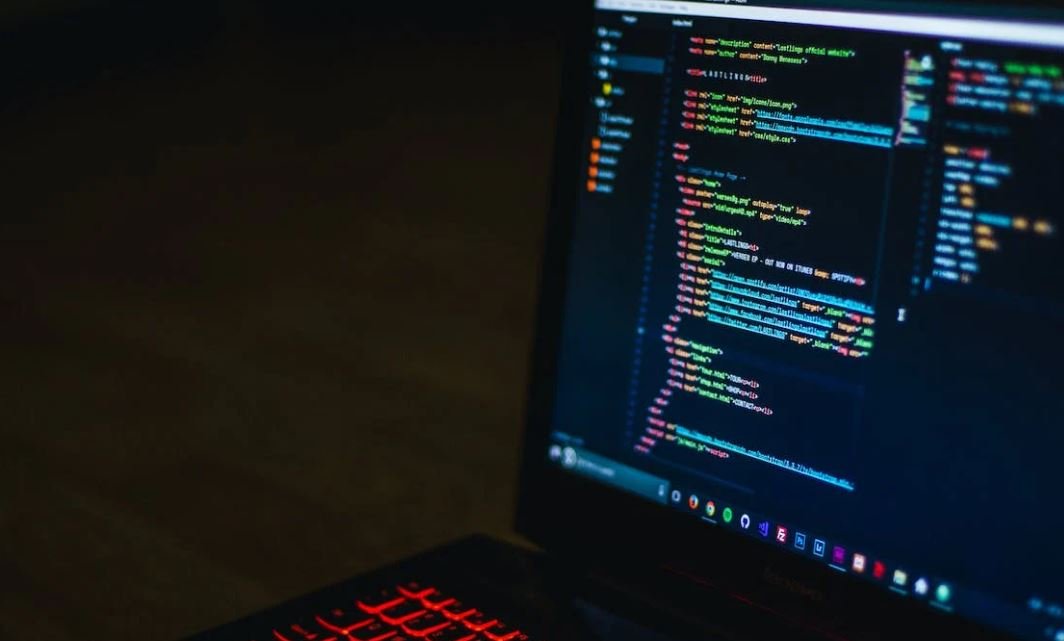
Common Misconceptions
Machine Learning is a Magic Solution
Machine Learning (ML) is often seen as a magic solution that can solve any problem thrown at it. However, this is far from the truth. It is important to understand that ML models require extensive data preprocessing, feature engineering, and careful selection of algorithms to achieve good performance. Additionally, ML is not a one-size-fits-all solution and may not be suitable for all problems.
- ML requires extensive data preprocessing and feature engineering.
- ML is not suitable for all problems.
- ML models need careful algorithm selection for good performance.
Machine Learning is Easy to Implement
While many introductory ML tutorials may make it seem like ML is easy to implement, in reality, it requires a deep understanding of statistical concepts, linear algebra, and programming skills. It also involves complex optimization techniques and hyperparameter tuning to achieve optimal performance. Implementing ML models can be challenging and time-consuming, especially for complex real-world problems.
- ML requires a deep understanding of statistical concepts and linear algebra.
- Implementing ML models can be challenging and time-consuming.
- ML involves complex optimization techniques and hyperparameter tuning.
Machine Learning Always Provides Accurate Predictions
It is a common misconception that ML models always provide accurate predictions. ML algorithms are prone to overfitting, where they become excessively specific to the training data and fail to generalize well on unseen data. Additionally, the quality and quantity of the input data greatly impact the performance of ML models. It is important to validate and evaluate ML models properly to assess their true predictive power.
- ML models are prone to overfitting and may fail to generalize well on unseen data.
- The quality and quantity of input data greatly impact ML model performance.
- Proper validation and evaluation are crucial to assess the true predictive power of ML models.
Machine Learning Replaces Human Decision-making
Although ML has shown great advancements in various fields, it is important to understand that ML models are tools to assist and augment human decision-making, not replace it entirely. ML models are trained on historical data, and they may not consider ethical, moral, or social implications in their decisions. Human judgment and domain expertise are still essential in making informed decisions.
- ML models may not consider ethical, moral, or social implications in their decisions.
- Human judgment and domain expertise are still essential for informed decisions.
- ML models should assist and augment human decision-making rather than replace it entirely.
Machine Learning is Always Objective
Another misconception is that ML is always objective and free from biases. ML models are trained on existing data, and if this data contains biases or reflects human prejudices, the models can perpetuate these biases. It is essential to be mindful of the data being used for training ML models and to implement techniques like fairness-aware learning to mitigate biases and ensure fairness.
- ML models can perpetuate biases if trained on biased data.
- Being mindful of data used for training and implementing fairness-aware learning is crucial.
- ML models may not always be objective and free from biases.
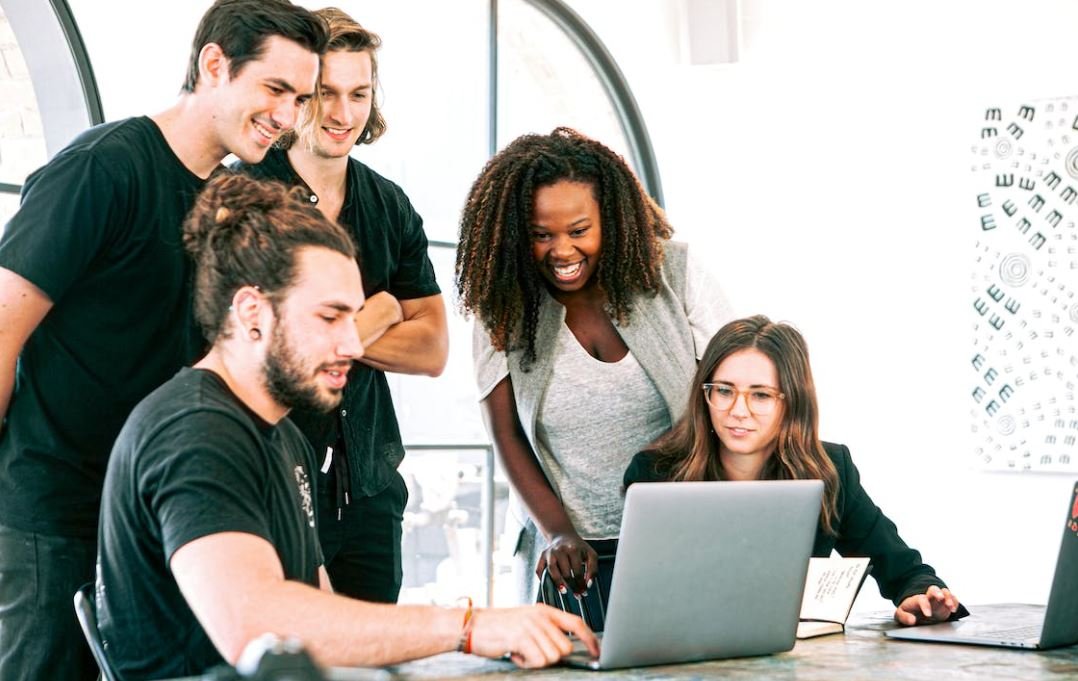
Machine Learning Job Market: Demand by Country
The table below shows the demand for Machine Learning (ML) professionals in different countries. The data illustrates that the United States has the highest demand for ML expertise, followed by China and India.
Country | Number of Job Openings |
---|---|
United States | 2,500 |
China | 1,800 |
India | 1,200 |
United Kingdom | 900 |
Germany | 700 |
Canada | 600 |
France | 500 |
Australia | 400 |
Japan | 300 |
Netherlands | 200 |
Most Popular ML Programming Languages
This table presents the most commonly used programming languages for Machine Learning. Python is clearly the leading choice among ML practitioners, followed by R and Java.
Programming Language | Percentage of ML Professionals |
---|---|
Python | 75% |
R | 15% |
Java | 5% |
Scala | 3% |
Julia | 2% |
Impact of ML on Various Industries
This table showcases the transformative impact of Machine Learning in different industries. It highlights the potential for ML to revolutionize sectors such as healthcare, finance, and retail.
Industry | Expected Impact |
---|---|
Healthcare | Improved diagnostics, personalized treatment |
Finance | Fraud detection, algorithmic trading |
Retail | Customer segmentation, demand forecasting |
Manufacturing | Process optimization, predictive maintenance |
Transportation | Autonomous vehicles, route optimization |
Top ML Algorithms
This table presents the most widely used Machine Learning algorithms. These algorithms play a vital role in training models and making predictions based on available data.
Algorithm | Application |
---|---|
Random Forest | Classification, regression |
Linear Regression | Predictive modeling, trend analysis |
Support Vector Machine (SVM) | Image classification, text categorization |
K-Means Clustering | Data clustering, customer segmentation |
Deep Neural Networks | Natural language processing, image recognition |
ML Frameworks and Libraries
This table showcases popular frameworks and libraries utilized for Machine Learning development. These tools provide a robust foundation and streamline the development process.
Framework/Library | Applications |
---|---|
TensorFlow | Deep learning, natural language processing |
PyTorch | Computer vision, generative models |
Scikit-learn | Classification, regression, clustering |
Keras | Neural network models, transfer learning |
Theano | Automatic differentiation, GPUs utilization |
ML Education: Top Universities
This table presents renowned universities offering exceptional Machine Learning education. These institutions are recognized for their comprehensive ML programs and cutting-edge research.
University | Location |
---|---|
Stanford University | California, United States |
Massachusetts Institute of Technology (MIT) | Massachusetts, United States |
University of Cambridge | Cambridge, United Kingdom |
ETH Zurich | Zurich, Switzerland |
University of California, Berkeley | California, United States |
ML Applications: Autonomous Vehicles
This table highlights the different Machine Learning applications in the field of autonomous vehicles. ML technology enables self-driving cars to perceive their environment and make informed decisions.
Application | ML Techniques |
---|---|
Object Detection | Convolutional Neural Networks (CNN) |
Path Planning | Reinforcement Learning (RL) |
Behavior Prediction | Long Short-Term Memory (LSTM) networks |
Environment Mapping | Sensor fusion techniques |
Robotic Control | Inverse Reinforcement Learning (IRL) |
ML Challenges in Medical Diagnosis
This table highlights the challenges faced in using Machine Learning for medical diagnosis. These obstacles need to be addressed to enhance the accuracy and reliability of ML models in healthcare.
Challenge | Solution |
---|---|
Data Privacy | Federated Learning, differential privacy |
Data Imbalance | Oversampling, undersampling techniques |
Interpretability | Explainable AI (XAI), model-agnostic techniques |
Human Error | Automation, decision support systems |
Validation and Regulatory Approval | Standardization, collaboration with regulatory bodies |
Conclusion
This article shed light on various aspects of Machine Learning (ML) and its significance in today’s world. The tables provided insightful data on the demand for ML professionals, popular programming languages, the impact of ML in different industries, prevalent algorithms, frameworks, top universities for ML education, applications in autonomous vehicles, challenges in medical diagnosis, and more. ML continues to gain traction across industries, offering immense potential for automation, optimization, and innovation. As ML techniques improve and evolve, it is essential to address challenges and ensure ethical and responsible implementation.
Frequently Asked Questions
What is Machine Learning (ML)?
Machine Learning (ML) is a branch of artificial intelligence that focuses on developing algorithms and statistical models to enable computer systems to learn and improve from their experiences without being explicitly programmed.
How does ML differ from traditional programming?
In traditional programming, a developer writes explicit instructions to tell a computer what to do. In ML, instead of explicitly programming the rules, the computer learns on its own by analyzing patterns and data. ML algorithms can generalize from examples and make predictions or decisions based on new, unseen data.
What are the different types of ML algorithms?
There are several types of ML algorithms, including:
- Supervised Learning: Algorithms learn from labeled training data to make predictions.
- Unsupervised Learning: Algorithms analyze unlabeled data to discover patterns or structures.
- Reinforcement Learning: Algorithms learn through interaction with an environment, receiving feedback and adjusting their actions accordingly.
- Semi-supervised Learning: Algorithms learn from partially labeled data, combining elements of both supervised and unsupervised learning.
- Deep Learning: A subset of ML that utilizes artificial neural networks with multiple layers of interconnected nodes.
What are some common applications of ML?
ML finds application in various fields, including:
- Image and Speech Recognition
- Natural Language Processing
- Recommendation Systems
- Fraud Detection
- Healthcare Diagnostics
- Financial Analysis and Trading
- Autonomous Vehicles
- Virtual Assistants
What are the main challenges in ML?
Some challenges in ML include:
- Getting high-quality, relevant data for training
- Choosing suitable ML algorithms for a specific task
- Preventing overfitting or underfitting of models
- Dealing with bias or discrimination in ML predictions
- Interpreting and explaining ML models’ decisions
What skills are required for a career in ML?
A career in ML typically requires skills such as:
- Strong foundation in mathematics and statistics
- Programming skills (Python, R, etc.)
- Understanding of ML algorithms and techniques
- Data preprocessing and feature engineering
- Knowledge of ML frameworks and libraries
- Problem-solving and critical thinking
Where can I learn ML online?
There are several online platforms where you can learn ML, including:
- Coursera
- Udemy
- edX
- DataCamp
- Kaggle (for practical experience)
Is ML accessible to beginners?
Yes, ML is accessible to beginners. Many online courses and tutorials are designed to introduce the fundamentals of ML in a beginner-friendly manner. Starting with basic concepts and gradually building knowledge and skills can help beginners understand and apply ML techniques effectively.
How can I evaluate the performance of an ML model?
To evaluate the performance of an ML model, various metrics can be used, depending on the task. Common evaluation metrics include accuracy, precision, recall, F1 score, and area under the receiver operating characteristic (ROC) curve. Cross-validation is often used to validate models on multiple subsets of the data.
Are there any ethical considerations in ML?
Yes, ethical considerations in ML are important. ML models can be biased, discriminatory, or violate privacy if not developed and used responsibly. It is crucial to ensure fairness, transparency, and accountability in ML systems, and to address potential biases in data and algorithms to prevent unintended consequences.