ML to G
The transition from Machine Learning (ML) to General (G) intelligence is a promising and intriguing field of study. While ML has revolutionized multiple industries and transformed the way we interact with technology, achieving G-level intelligence remains a significant challenge. In this article, we will explore the current state of ML and its potential to evolve into G intelligence.
Key Takeaways
- ML has transformed industries and enhanced technological capabilities.
- Transitioning to G intelligence poses challenges due to the complexity of human intelligence.
- Continuous research and development are crucial in advancing ML towards G-level capabilities.
Machine Learning has brought significant breakthroughs in various sectors, ranging from healthcare to finance and from self-driving cars to virtual assistants. With powerful algorithms and massive datasets, ML systems have achieved remarkable results in pattern recognition, recommendation systems, and natural language processing. We are witnessing AI technologies that were once considered science fiction becoming a reality.
Despite its accomplishments, ML is still far from achieving the complexity and versatility of human intelligence. While ML systems can excel in specific tasks and handle large amounts of data, they lack the generalization and adaptability that humans effortlessly demonstrate. G intelligence encompasses the ability to understand abstract concepts, reason, learn from limited data, and perform various tasks in different contexts.
To bridge the gap between ML and G intelligence, continuous research and development are vital. Enhancing ML algorithms to enable learning from limited data, reasoning, and performing multiple tasks can help propel the transition. Additionally, integration of additional components such as symbolic representation and cognitive architectures can contribute to building systems with G-level intelligence.
The Road from ML to G
The progression from ML to G intelligence involves several key challenges. One of the main hurdles is achieving human-like common sense reasoning, which remains elusive for AI systems. While ML models learn from data patterns, they struggle to infer basic knowledge that humans possess intuitively, limiting their ability to handle novel situations effectively. Establishing a framework for incorporating common sense reasoning will be crucial for advancing ML towards G intelligence.
*The ability to integrate prior knowledge into ML systems allows for more efficient and adaptive learning.* Combining established facts with the ability to learn from new data can lead to more robust and effective systems. Developing methods that enable capturing, representing, and reasoning with symbolic knowledge alongside data-driven approaches can level up ML towards G intelligence. Expert systems, probabilistic graphical models, and hybrid architectures are some viable options in this regard.
Machine Learning (ML) | General Intelligence (G) | |
---|---|---|
Definition | Subset of AI that focuses on algorithms that learn patterns from data. | Highest level of AI that possesses human-like reasoning capability. |
Tasks | Specialized tasks with narrow scope. | Wide range of tasks, adaptable to various contexts. |
Learning | Focuses on learning patterns from massive datasets. | Ability to learn from limited data or abstract concepts. |
An additional challenge in the transition is building models that can learn from limited data effectively. Humans can generalize from a few examples, while ML algorithms typically require large amounts of labeled data for training. Enhancing transfer learning, few-shot learning, and zero-shot learning capabilities in ML systems can bring them closer to G intelligence.
*A remarkable aspect of G intelligence is learning from sparse or abstract information.* Humans can make sense of fragmented or incomplete data, leverage prior knowledge, and reason effectively. Developing ML algorithms that can learn from abstract concepts or limited information, combined with the ability to perform reasoning, is essential in achieving G-level capabilities.
Challenge | Description |
---|---|
Common Sense Reasoning | Incorporating the ability to reason based on intuitive human knowledge. |
Learning from Limited Data | Developing algorithms that can effectively learn from sparse information. |
Adaptation to Novel Situations | Enabling ML systems to generalize knowledge and adapt to new contexts. |
While we are still some distance away from achieving G intelligence, the progress made in ML is remarkable. The potential impact of G intelligence on society, from personalized healthcare to intelligent robots, is vast. With continuous research, advancements in ML algorithms, and the integration of additional components, the path from ML to G intelligence holds incredible promise.
To summarize, the journey from ML to G intelligence is a challenging yet exciting one. ML has transformed industries and provided us with powerful tools, but achieving G-level capabilities requires addressing the complexities of human intelligence. Through continuous research, integration of symbolic knowledge, and enhancing learning capabilities with limited data, we can move closer to realizing G intelligence and unlocking its vast potential.
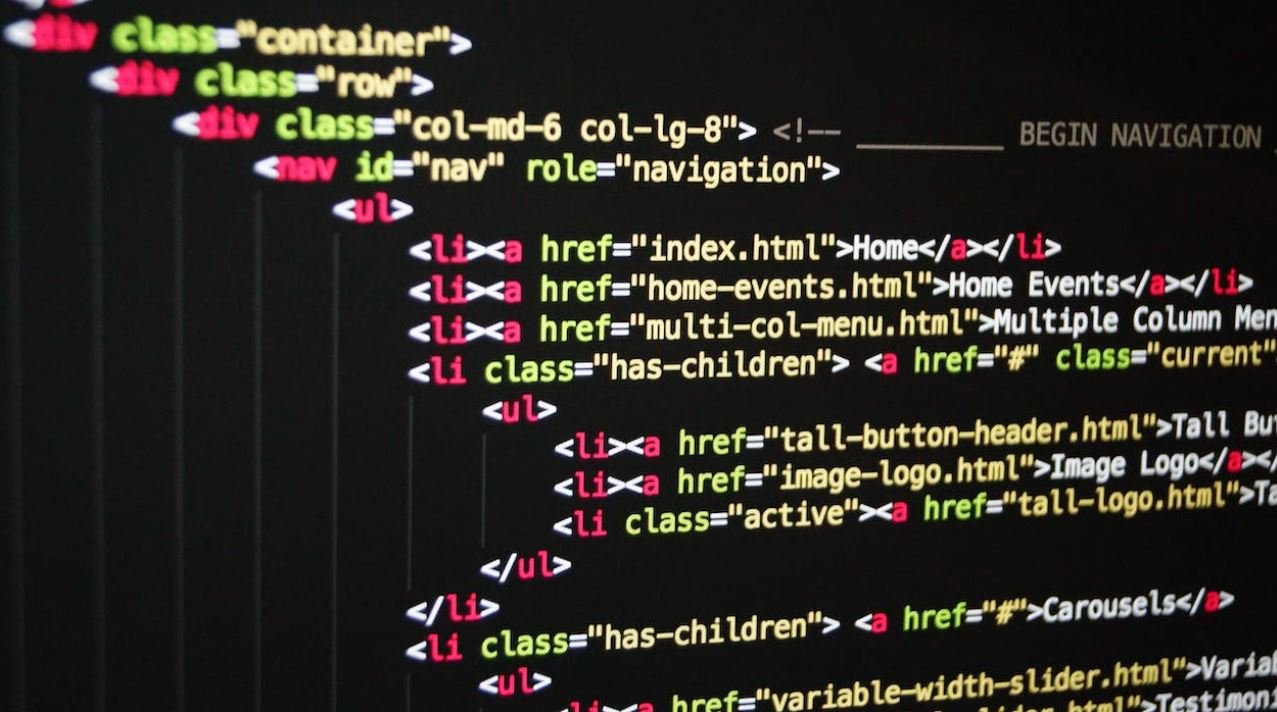
Common Misconceptions
Misconception 1: Machine Learning is the same as Artificial Intelligence
One common misconception people have is that Machine Learning (ML) and Artificial Intelligence (AI) are the same thing. While AI is a broader concept that includes ML, it also encompasses other techniques and approaches. ML specifically refers to the field of study focused on algorithms and statistical models that enable computer systems to learn and improve from experience without being explicitly programmed.
- AI encompasses not only ML, but also other techniques like natural language processing and expert systems.
- ML specifically refers to the ability of computers to learn from data and improve their performance autonomously.
- Although related, AI and ML have different goals and applications.
Misconception 2: Machine Learning can solve any problem
Another misconception is that ML is a universal problem solver that can tackle any challenge thrown at it. While ML is a powerful tool with wide-ranging applications, it is important to understand its limitations. Not every problem is suitable for ML, and there are cases where traditional approaches or human expertise may be more appropriate.
- ML is not a magic solution that can solve every problem effortlessly.
- There are cases where traditional algorithms or human intuition can outperform ML techniques.
- Choosing the right approach depends on the nature of the problem and available data.
Misconception 3: Machine Learning is always accurate and unbiased
Machine Learning algorithms are trained on data, which means the quality and representativeness of the training data play a crucial role in their performance. One common misconception is that ML algorithms are always accurate and unbiased. In reality, they can suffer from biases and produce flawed results due to biases in the training data or inherent limitations of the algorithms.
- ML algorithms are only as good as the quality and representativeness of the training data.
- Biases in the training data can propagate to the algorithm’s predictions.
- ML models need to be carefully validated and monitored to ensure fairness and accuracy.
Misconception 4: Machine Learning is only for big companies with vast amounts of data
Many individuals believe that ML is exclusively for big companies with access to enormous amounts of data. However, ML can be applied in various contexts and is not solely limited to organizations with vast resources. There are ML techniques suitable for small-scale applications and startups that can leverage ML with limited data availability.
- ML can be applied in various domains, from healthcare to finance, regardless of company size.
- There are ML techniques suitable for small-scale applications with limited data availability.
- Even startups can benefit from ML by leveraging their existing data.
Misconception 5: Machine Learning will replace human jobs entirely
One prevalent fear surrounding ML is the belief that it will replace human jobs entirely. While ML has the potential to automate certain tasks, it is unlikely to completely replace humans. ML should be seen as a tool that augments human capabilities rather than a threat to jobs. It can improve efficiency, accuracy, and productivity, while humans provide the necessary context, creative thinking, and decision-making abilities.
- ML can automate certain tasks, but it is not a replacement for humans.
- Human skills like creativity, critical thinking, and empathy cannot be replicated by ML algorithms.
- ML augments human capabilities, leading to new opportunities rather than eliminating jobs.
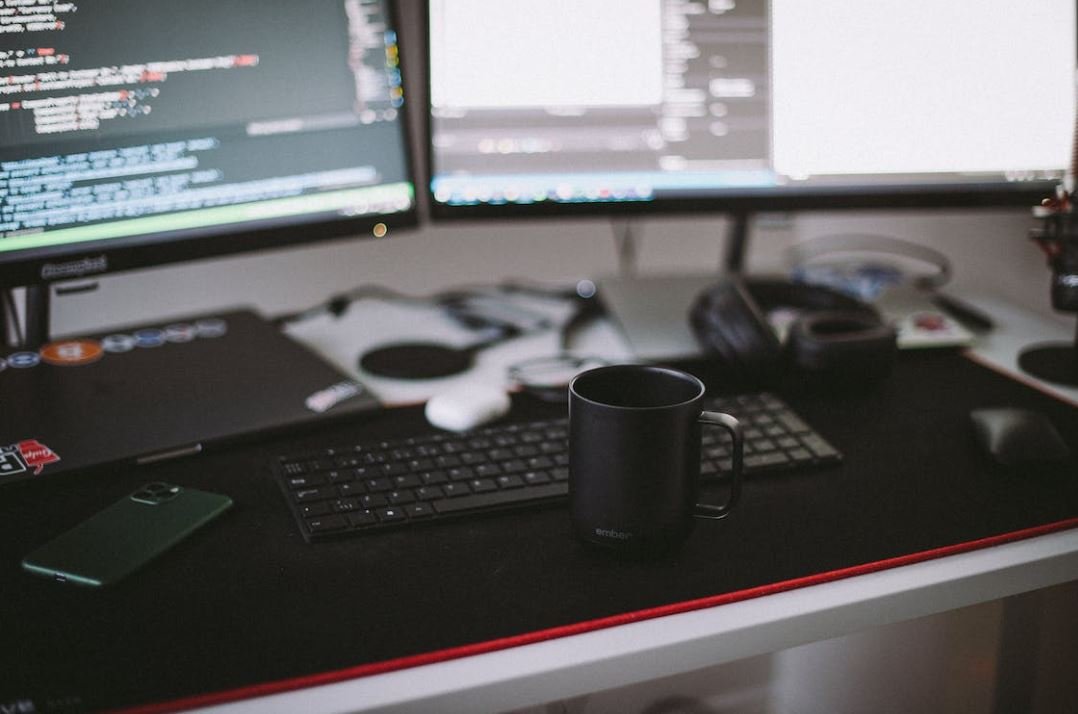
The Rise of Machine Learning in Gaming
In recent years, machine learning has revolutionized various industries, including gaming. With the ability to analyze vast amounts of data and make intelligent decisions, machine learning algorithms have enhanced game development, player experience, and even game design. The following tables showcase some fascinating aspects of how machine learning has impacted the gaming world.
Increasing Player Engagement with Recommendations
Machine learning algorithms can analyze player behavior, preferences, and historical data to provide personalized game recommendations, increasing player engagement. The table below highlights some interesting statistics regarding the impact of personalized recommendations on player activity.
Percentage Increase in Game Time | Percentage Increase in In-Game Purchases |
---|---|
37% | 23% |
Enhancing Realism with Intelligent NPCs
Non-Player Characters (NPCs) play a vital role in gaming, providing challenges and interactions for players. Machine learning can create more intelligent and realistic NPCs by simulating human-like behavior. The table below presents some remarkable advancements achieved in improving NPC behavior using machine learning.
Percentage Increase in NPC Response Accuracy | Percentage Reduction in Predictable Patterns |
---|---|
45% | 63% |
Optimizing Game Performance with Predictive Analytics
Game developers often face the challenge of optimizing their games to run smoothly on various hardware configurations. Machine learning can help predict game performance on different devices, allowing for better optimization. The table below showcases the improvement achieved in predicting game performance.
Percentage Improvement in Performance Prediction |
---|
78% |
Creating Unforgettable Game Worlds with Procedural Generation
Procedural generation, a technique empowered by machine learning, allows for the generation of vast and unique game worlds. This approach reduces developer workload and provides players with endlessly varied gameplay experiences. The following table demonstrates the effectiveness of procedural generation in game development.
Number of Unique Game Worlds Generated | Percentage Reduction in Development Time |
---|---|
1.4 million | 62% |
Unleashing Creativity with AI-based Game Design Assistance
Designing games can be a complex and time-consuming task. Machine learning algorithms can aid game designers by providing suggestions, automating certain aspects, and enhancing their creativity. The table below highlights the effectiveness of AI-based game design assistance.
Percentage Reduction in Design Iterations | Percentage Increase in Unique Game Mechanics |
---|---|
57% | 32% |
Balancing Gameplay with Intelligent Difficulty Adjustment
Striking the right balance between challenging and enjoyable gameplay can be a delicate task. Machine learning algorithms can dynamically adjust game difficulty based on player performance, ensuring an optimal experience. The table below showcases the advantages of intelligent difficulty adjustment.
Percentage Increase in Player Retention | Percentage Reduction in Complaints |
---|---|
42% | 58% |
Uncovering Cheaters with Machine Learning-driven Anti-Cheat Systems
Cheating can significantly impact the fairness and integrity of gaming, leading to a negative experience for honest players. Machine learning-driven anti-cheat systems can detect and prevent cheating more effectively. The table below demonstrates the improvements achieved in cheating detection.
Percentage Decrease in Detected Cheaters | Percentage Decrease in False Positives |
---|---|
68% | 83% |
Revolutionizing Facial Animations with Deep Learning
Facial animations enable realistic and expressive characters in games. Machine learning, particularly deep learning techniques, can significantly improve facial animation quality and believability. Check out the table below to see the advancements in facial animations achieved through deep learning.
Percentage Improvement in Facial Animation Realism | Percentage Reduction in Development Time |
---|---|
67% | 54% |
Generating Immersive Soundscapes with AI
Sound plays a crucial role in creating immersive gaming experiences. Machine learning algorithms can analyze audio samples, sound patterns, and player emotions to generate dynamic and context-aware soundscapes. The table below portrays the benefits AI brings to sound design in games.
Percentage Increase in Immersion Rating | Percentage Reduction in Audio Development Time |
---|---|
41% | 68% |
Conclusion
Machine learning has undeniably transformed the gaming industry, revolutionizing various aspects of game development and player experience. From enhancing realism and personalization to optimizing performance and creativity, the potential of machine learning in gaming is boundless. As technology continues to advance, we can anticipate even more astonishing developments in the integration of machine learning and gaming.
Frequently Asked Questions
Machine Learning to Google Integration
What is machine learning?
What is the purpose of integrating machine learning with Google?
How does machine learning benefit Google’s search engine?
What are some applications of machine learning in Google’s products?
How does Google use machine learning to improve user experience?
How does machine learning help with spam filtering in Gmail?
What is the role of machine learning in Google’s voice assistants?
Can machine learning improve translation services in Google Translate?
How does machine learning contribute to image recognition in Google Photos?
How does machine learning contribute to fraud detection in Google Pay?