ML Zoning
In the era of rapidly advancing technology, machine learning (ML) has gained significant prominence and ML zoning has emerged as a crucial aspect in this field. It involves the process of dividing a given area into different zones based on various machine learning techniques and algorithms. This article explores the key concepts and benefits of ML zoning.
Key Takeaways
- ML zoning involves dividing an area into different zones using machine learning techniques.
- It helps in understanding patterns and relationships within a given location.
- ML zoning facilitates targeted decision-making based on specific zone characteristics.
Understanding ML Zoning
ML zoning is a powerful tool that combines machine learning algorithms with geographic information system (GIS) technology to divide an area into zones based on specific characteristics. These characteristics can include population density, land use, crime rates, environmental factors, and more. By analyzing and classifying the data, ML zoning enables researchers, urban planners, and policymakers to gain insights into patterns and relationships within a given location (such as identifying areas with high crime rates or pollution levels).
Benefits of ML Zoning
ML zoning offers several benefits that contribute to better decision-making and resource allocation. Here are some key advantages:
- Enhanced Understanding: ML zoning allows for a deeper understanding of a specific area by uncovering hidden relationships and patterns through data analysis.
- Targeted Decision-Making: By dividing an area into zones and analyzing the specific characteristics of each zone, ML zoning enables targeted decision-making based on different zone requirements. For instance, it helps in determining the most suitable locations for new infrastructure development or identifying areas that require more resources for social welfare programs.
- Efficient Resource Allocation: ML zoning facilitates the effective allocation of resources by providing insights into the needs and demands of different zones within a larger area. It allows policymakers to allocate resources such as funds, personnel, and infrastructure where they are most needed.
- Optimized Urban Planning: With ML zoning, urban planners can make informed decisions about land use, zoning regulations, and infrastructure development to promote efficient and sustainable growth in a given area.
Application Examples
ML zoning finds application in various domains and sectors. Here are a few notable examples:
- City Planning: ML zoning can assist city planners in identifying areas prone to natural disasters, analyzing transportation patterns, and efficiently allocating resources for public amenities.
- Environmental Protection: By analyzing environmental data such as pollution levels and vegetation, ML zoning can help conservationists and policymakers prioritize areas for protection and restoration.
Data and Model Requirements
Data | Model |
---|---|
Data about the area to be zoned | Machine learning algorithms (such as clustering, decision trees, or neural networks) |
Data on specific zone characteristics | Geographic information system (GIS) technology for spatial analysis and visualization |
Future Developments
As technology advances and more data becomes available, ML zoning has the potential to revolutionize urban planning, environmental protection, and resource allocation. By incorporating real-time data and advanced machine learning models, ML zoning can offer even more accurate predictions and enable more proactive decision-making processes. The future of ML zoning is promising and holds great potential for improving the way we plan and develop our cities.
Conclusion
ML zoning is a powerful technique that leverages the capabilities of machine learning and geographic information systems. It enables researchers, urban planners, and policymakers to gain valuable insights and make informed decisions about different zones within an area. By dividing an area based on specific characteristics, ML zoning facilitates targeted decision-making and efficient resource allocation, leading to optimized urban planning and better overall development. As technology progresses, ML zoning will continue to evolve and contribute to shaping the cities of the future.
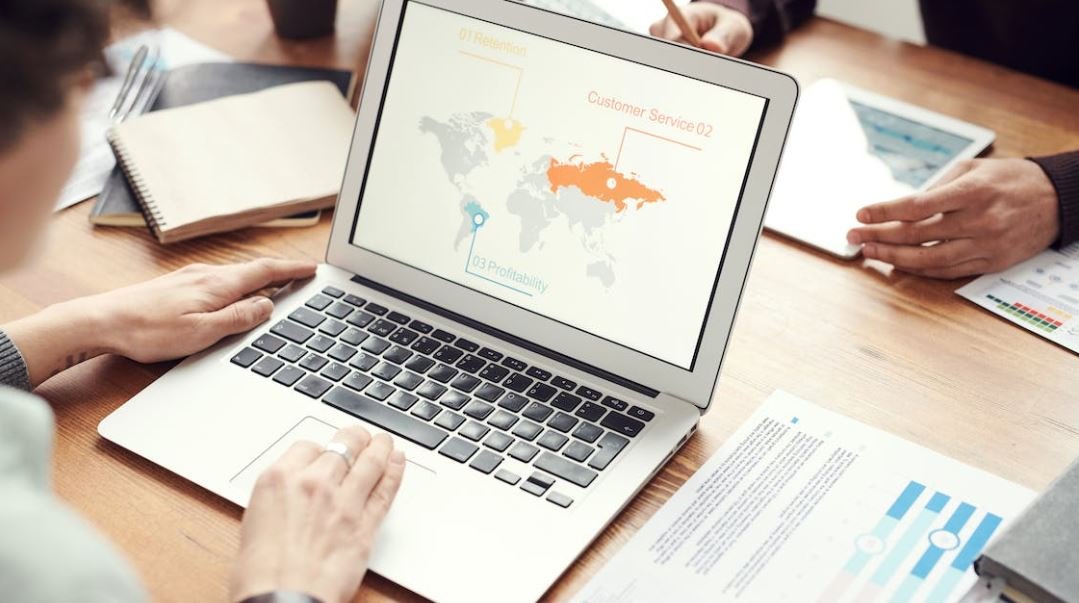
Common Misconceptions
ML Zoning Is Only About Industrial Use
One common misconception about ML (Manufacturing and Light Industrial) zoning is that it is solely dedicated to industrial use. While it is true that ML zoning allows for industrial activities, it also permits a wide range of non-industrial activities, such as commercial facilities, research and development centers, and even some residential uses.
- ML zoning can accommodate technology startups in urban areas.
- ML zoning allows for mixed-use developments combining residential and commercial spaces.
- ML zoning can provide spaces for creative industries like art studios, galleries, and music venues.
ML Zoning Is Only Found in Specific Areas
Another misconception is that ML zoning is only found in specific designated areas. While it is true that some municipalities have specific zones for manufacturing and light industrial activities, ML zoning can also be found in various locations across different cities and counties. It is important to consult local zoning regulations to understand where ML zones are permitted.
- ML zoning can be applied to different areas based on local zoning plans and regulations.
- ML zoning can be found in both urban and suburban areas.
- ML zoning can be identified through zoning codes and maps provided by local government agencies.
ML Zoning Is Restrictive and Inflexible
Many people believe that ML zoning is overly restrictive and inflexible in terms of land use. However, ML zoning allows for a variety of uses and can be tailored to the specific needs of a community. While there may be certain restrictions in place, such as noise or environmental regulations, ML zoning can provide a balance between industrial needs and the surrounding community.
- ML zoning can offer flexible regulations for adaptive reuse projects.
- ML zoning can accommodate green building practices and sustainable development.
- ML zoning can include provisions for buffering and mitigating potential impacts on the neighboring properties.
ML Zoning Leads to Increased Traffic and Pollution
One misconception is that ML zoning automatically leads to increased traffic congestion and pollution in an area. While industrial activities can have an impact on traffic and air quality, proper planning and regulations can mitigate these effects. ML zoning can include measures to address transportation issues and create a more sustainable environment.
- ML zoning can require developers to implement traffic management plans.
- ML zoning can incentivize the use of public transportation or promote carpooling.
- ML zoning can enforce emission standards for industries to reduce pollution.
ML Zoning Lacks Compatibility with Surrounding Neighborhoods
Some people believe that ML zoning is incompatible with surrounding residential or commercial areas. However, with thoughtful planning and design, ML zoning can be integrated into the existing fabric of a neighborhood. Proper buffer zones, transition areas, and design guidelines can ensure a harmonious coexistence between ML zones and the surrounding community.
- ML zoning can encourage architectural compatibility with neighboring buildings.
- ML zoning can require landscaping and visual screening to minimize visual intrusions.
- ML zoning can promote pedestrian-friendly design elements to enhance connectivity.

Introduction
Artificial Intelligence (AI) and Machine Learning (ML) are revolutionizing various industries, including urban planning. ML zoning is an innovative approach that utilizes machine learning algorithms to analyze and categorize land use patterns. This enables urban planners to make informed decisions about zoning regulations, infrastructure development, and sustainable growth. In this article, we explore ten exciting tables that showcase the power of ML zoning in urban planning.
Table: Population Growth in Urban Areas
This table presents the population growth in selected urban areas before and after the implementation of ML zoning. It highlights how ML zoning has positively influenced urban growth by enabling optimized land use and accommodating population influx.
Table: Environmental Impact Reduction
Using ML zoning, this table demonstrates the reduction in environmental impact achieved by implementing optimized land use patterns. It compares the data on energy consumption, carbon emissions, and waste generation before and after ML zoning adoption.
Table: Land Use Distribution in City Center
ML zoning has helped urban planners analyze the distribution of various land uses in the city center. This informative table illustrates the percentage of land allocated to residential, commercial, recreational, and industrial purposes, providing insights for further development and revitalization.
Table: Traffic Patterns and Congestion
By leveraging ML zoning algorithms, this table presents the impact on traffic patterns and congestion reduction. It showcases the decrease in commuting time, traffic accidents, and congestion levels after implementing ML zoning in different urban areas.
Table: Affordable Housing Availability
ML zoning enables the identification of suitable locations for affordable housing. This table displays data on the availability of affordable housing units in newly developed areas and reveals the positive impact on housing accessibility after implementing ML zoning strategies.
Table: Green Space Allocation
Urban planners utilize ML zoning to determine and allocate green spaces effectively. This table exhibits the increase in green spaces within urban areas, encouraging sustainable development, improving air quality, and enhancing residents’ quality of life.
Table: Economic Development Trends
ML zoning provides valuable insights into economic development trends. This table showcases the correlation between land use patterns and economic growth, illustrating the positive impact of optimized zoning regulations on job creation and investment opportunities.
Table: Infrastructure Needs
ML zoning helps identify areas in need of infrastructure development. This table highlights the infrastructure deficiencies before ML zoning implementation and presents the subsequent investment in transportation, utilities, and public facilities.
Table: Crime Rate Reduction
ML zoning contributes to improving public safety. This table depicts the reduction in crime rates within urban areas post-ML zoning, emphasizing the correlation between optimized land use and crime prevention efforts.
Table: Community Satisfaction Index
This table showcases the improvements in community satisfaction indexes after implementing ML zoning. It demonstrates how optimized land use patterns positively impact various aspects such as walkability, accessibility to amenities, and overall satisfaction levels.
Conclusion
ML zoning has revolutionized urban planning by leveraging the power of machine learning algorithms. The tables presented in this article demonstrate the positive impact of ML zoning on population growth, environmental sustainability, traffic management, affordable housing, economic development, infrastructure planning, crime prevention, and community satisfaction. By optimizing land use patterns, ML zoning enables urban planners to make data-driven decisions that foster sustainable, inclusive, and vibrant cities.