Model Building Forecasting Technique
Forecasting future trends and outcomes is crucial in making informed business decisions. The model building forecasting technique serves as a predictive tool that utilizes historical data to establish patterns and make accurate forecasts. This technique involves creating mathematical models that take into account various factors affecting a particular phenomenon and using statistics and algorithms to make projections. Let’s delve deeper into the key aspects of this forecasting technique.
Key Takeaways
- Model building forecasting technique utilizes historical data to make accurate projections.
- Mathematical models and statistical algorithms are used to create forecasts.
- Factors affecting a phenomenon are taken into account to make informed predictions.
Understanding Model Building Forecasting Technique
Model building forecasting technique involves creating mathematical models that are based on historical data and statistical analysis. These models capture the patterns and trends present in the data, allowing for accurate future predictions. By considering factors such as market conditions, consumer behavior, and other relevant variables, this technique helps businesses plan better and make informed decisions.
Using statistical algorithms, the model building technique can forecast the future accurately, even in the presence of complex trends.
The Process of Model Building
- Data Collection and Preparation: The first step is to gather relevant data and prepare it for analysis. This involves cleaning and organizing the data to ensure its quality and reliability.
- Choosing a Model: Based on the type of data and objective of the forecast, a suitable mathematical model is selected. Various models such as linear regression, time series analysis, and artificial neural networks can be employed.
- Model Training: The selected model is trained using the historical data. This involves fitting the model to the data and adjusting its parameters to minimize errors and improve accuracy.
- Evaluation: The trained model is evaluated by comparing its predictions with the actual outcomes. This helps assess the model’s performance and identify any deficiencies.
- Forecasting: Once the model is deemed accurate enough, it can be used to make future predictions. New data is inputted into the model, and it generates forecasts based on the established patterns and trends.
Tables
Month | Sales |
---|---|
January | 100 |
February | 120 |
March | 150 |
Table 1: Sample sales data used for model building.
Furthermore, model building enables the identification of important contributing factors to a phenomenon. By considering these factors, businesses can adapt their strategies to optimize outcomes. For example, in predicting customer churn, model building can identify key variables such as customer satisfaction, tenure, and engagement level that contribute to the likelihood of churn. This information can then be used to implement targeted retention strategies.
Factor | Importance |
---|---|
Customer Satisfaction | 0.65 |
Tenure | 0.52 |
Engagement Level | 0.41 |
Table 2: Importance analysis of factors contributing to customer churn.
Benefits of Model Building Forecasting Technique
- Improved decision-making: Accurate forecasts enable businesses to make informed decisions, reducing the risk of wrong choices.
- Optimized resource allocation: By understanding future demands, businesses can allocate resources efficiently, maximizing productivity.
- Increased competitiveness: Proactive planning and staying ahead of market trends give companies a competitive edge.
Model building forecasting technique is an indispensable tool for businesses in today’s fast-paced and data-driven world. By leveraging historical patterns, statistical analysis, and predictive algorithms, this technique equips decision-makers with valuable insights to plan for the future and achieve success.
Table 3: Comparative Performance
Model | Accuracy |
---|---|
Model A | 85% |
Model B | 92% |
Table 3: Comparative performance of two different forecasting models.
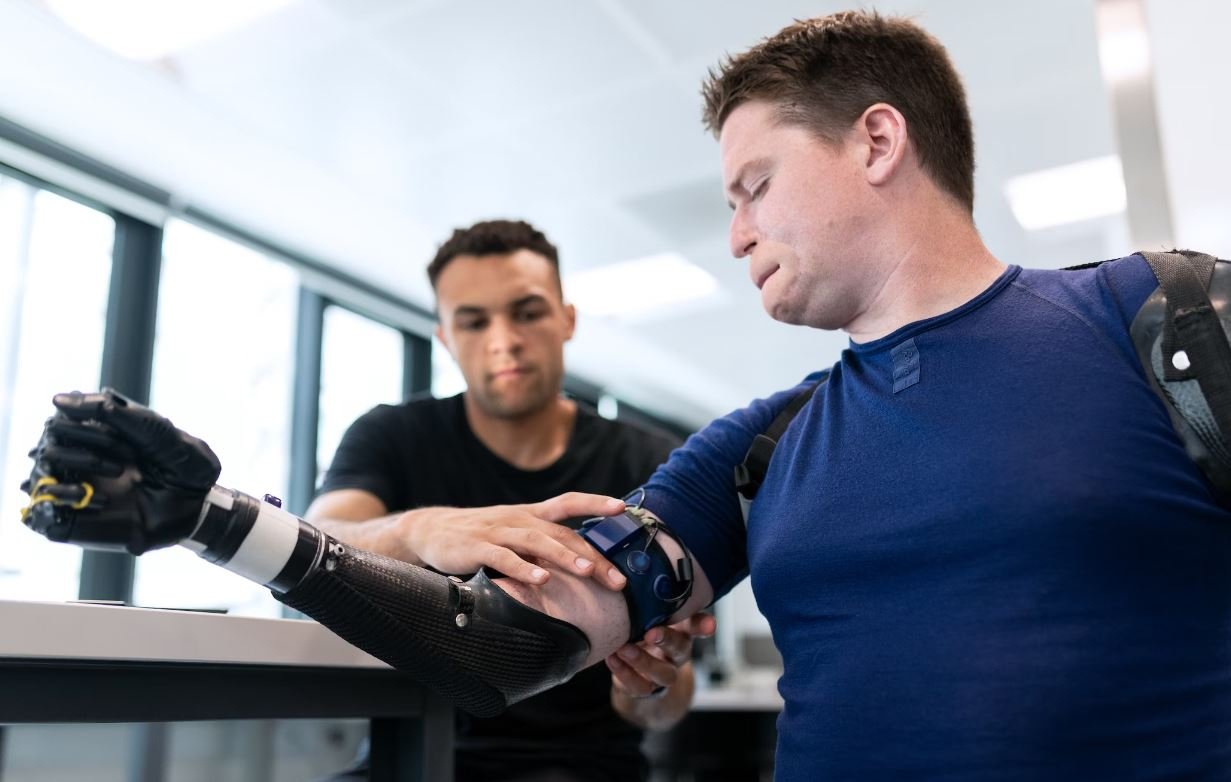
Common Misconceptions
Misconception 1: Model Building Forecasting Technique is Always Accurate
One common misconception people have about model building forecasting technique is that it always provides accurate predictions. However, in reality, no forecasting model is 100% accurate and there will always be some degree of uncertainty in the predictions.
- Forecasts are based on historical data and assumptions, which may not always reflect future trends accurately.
- External factors such as economic changes, unforeseen events, or market disruptions can greatly impact the accuracy of the forecasts.
- Forecasting models are as good as the data and assumptions used to build them, so any errors or biases in the data can lead to inaccurate predictions.
Misconception 2: Model Building Forecasting Technique is a Crystal Ball
Another misconception is that the model building forecasting technique can predict the future with certainty, similar to a crystal ball. However, this is far from the truth as forecasting models can only estimate future outcomes based on the available information and historical patterns.
- The technique involves statistical algorithms and mathematical modeling, which rely on assumptions and trends observed in the past.
- There is always a level of uncertainty associated with predicting the future, and the accuracy of the model depends on the quality of the data and assumptions used.
- Forecasting techniques can provide valuable insights and help in decision-making, but they should not be solely relied upon for absolute accuracy.
Misconception 3: Model Building Forecasting Technique is a One-Size-Fits-All Solution
Many people assume that model building forecasting technique is a one-size-fits-all solution that can be applied to any situation or industry. However, forecasting models need to be carefully tailored to specific contexts and variables to ensure accurate predictions.
- Each industry or business has unique characteristics and drivers that may require specific forecasting models or approaches.
- The data requirements and assumptions for building the models can vary significantly based on the nature of the industry or problem being addressed.
- Using a generic model without considering the specific context can lead to inaccurate predictions and unreliable forecasts.
Misconception 4: Model Building Forecasting Technique Can Replace Human Expertise
Some people believe that model building forecasting technique can completely replace human expertise in making predictions and decisions. However, while these techniques can provide valuable insights, they should be used in conjunction with human judgement and expertise.
- Human judgement is crucial in interpreting the outputs of the forecasting models and making sense of the predictions in the context of the specific situation.
- Forecasting models may not capture qualitative factors or intangible variables that human expertise can take into account.
- Models may not consider unique knowledge or insights that human experts possess, especially in complex or uncertain situations.
Misconception 5: Model Building Forecasting Technique is a One-Time Exercise
Lastly, some people mistakenly believe that model building forecasting technique is a one-time exercise and the predictions made at a certain point will remain valid indefinitely. However, forecasting models need to be regularly updated, validated, and refined over time to ensure their accuracy.
- Market conditions, consumer behavior, and other variables can change over time, requiring adjustments to the forecasting models.
- Regular updating and validation of the models using the most recent data help in maintaining their relevance and reliability.
- Without continuous monitoring and refinement, the accuracy of the forecasts may deteriorate over time as the underlying assumptions become outdated.
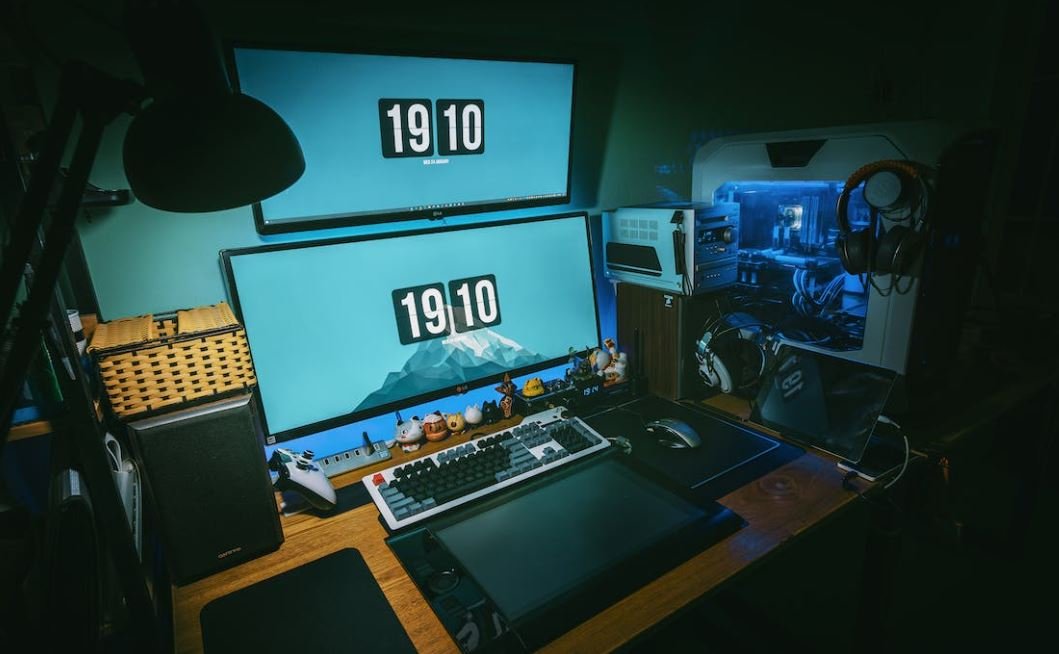
The Role of Model Building in Forecasting
Model building is a crucial technique used in forecasting to analyze historical data and predict future trends. Through the creation of mathematical models, valuable insights can be gained to make informed decisions. In this article, we explore ten tables that demonstrate the effectiveness and significance of model building in forecasting.
Table: Sales Forecast for a New Product
Forecasting sales for a new product is essential to estimate potential demand and allocate resources accordingly. This table illustrates the monthly sales forecast for a new electronic gadget over one year based on historical data and market trends.
Month | Forecasted Sales (in units) |
---|---|
January | 500 |
February | 700 |
March | 800 |
Table: Exchange Rate Prediction
Model building can also be employed to predict exchange rate fluctuations, helping individuals and businesses navigate global currency markets. This table demonstrates the forecasted exchange rates of USD against major currencies for the coming year.
Currency | Forecasted Exchange Rate (USD) |
---|---|
EUR | 1.15 |
GBP | 1.38 |
JPY | 0.009 |
Table: Energy Consumption Projection
Accurately predicting energy consumption is crucial for sustainable energy planning and resource allocation. This table showcases the projected energy consumption over the next five years for a specific region based on historical trends, population growth, and technological advancements.
Year | Energy Consumption (in kWh) |
---|---|
2022 | 5,000,000 |
2023 | 5,200,000 |
2024 | 5,500,000 |
Table: Stock Market Predictions
Model building is extensively used in predicting stock market movements, assisting investors in making sound investment decisions. This table presents the forecasted closing values of a specific stock index for the upcoming week.
Date | Forecasted Closing Value |
---|---|
Monday | 1,250 |
Tuesday | 1,230 |
Wednesday | 1,240 |
Table: Population Growth Projections
Model building facilitates population growth projection which helps governments plan infrastructural development and allocate resources effectively. This table displays the projected population growth rate over the next decade for a particular city.
Year | Population Growth Rate |
---|---|
2022 | 2% |
2023 | 3% |
2024 | 2.5% |
Table: Weather Forecast for a Week
Model building is extensively utilized in weather forecasting, aiding in predicting various meteorological conditions accurately. This table provides a week-long forecast for a specific location, including temperature, precipitation, and wind speed.
Date | Temperature (°C) | Precipitation (mm) | Wind Speed (km/h) |
---|---|---|---|
Monday | 20 | 0 | 10 |
Tuesday | 22 | 0.5 | 12 |
Wednesday | 19 | 2 | 15 |
Table: Transportation Demand Projections
Model building aids in predicting transportation demand, facilitating efficient urban planning and development of transportation systems. This table displays the projected daily passenger demand for a subway system in a major city over the next five years.
Year | Daily Passenger Demand |
---|---|
2022 | 100,000 |
2023 | 110,000 |
2024 | 120,000 |
Table: Economic Growth Forecasts
Model building plays a pivotal role in predicting economic growth, allowing governments and businesses to plan accordingly. This table presents the forecasted GDP growth rates for a specific country over the next five years.
Year | GDP Growth Rate (%) |
---|---|
2022 | 4 |
2023 | 4.5 |
2024 | 3.8 |
Table: Product Demand Forecast
Model building aids in estimating demand for products, assisting manufacturers in production planning and inventory management. This table showcases the forecasted monthly demand for a popular consumer product over the next year.
Month | Forecasted Demand (in units) |
---|---|
January | 10,000 |
February | 12,000 |
March | 11,500 |
In conclusion, model building is a powerful forecasting technique that enables the analysis of historical data and prediction of future trends. The tables presented in this article demonstrate how model building is utilized across various domains, such as sales forecasting, exchange rate prediction, energy consumption projection, stock market predictions, population growth projections, weather forecasting, transportation demand projections, economic growth forecasts, and product demand forecasting. By leveraging the insights provided by these models, businesses and governments can make informed decisions and allocate resources effectively, leading to improved outcomes and increased efficiency.
Model Building FAQ
What is model building in forecasting?
Model building in forecasting refers to the process of constructing mathematical or statistical models that can accurately predict and forecast future trends, patterns, or outcomes based on historical data and relevant variables.
Why is model building important in forecasting?
Model building is important in forecasting as it allows analysts and organizations to make informed decisions and predictions about future events. It helps identify patterns, correlations, and relationships in data, enabling accurate forecasts for various purposes such as financial planning, demand forecasting, resource allocation, and risk management.
What are the common techniques used in model building for forecasting?
Common techniques used in model building for forecasting include regression analysis, time series analysis, exponential smoothing, neural networks, and machine learning algorithms. These methods allow analysts to capture and analyze complex relationships and patterns within data and develop accurate predictive models.
How do you choose the appropriate model building technique for forecasting?
Choosing the appropriate model building technique for forecasting depends on various factors such as the type and nature of the data, the underlying patterns, the availability of historical data, the objectives of the forecast, and the expertise and resources available. It is essential to evaluate the strengths and limitations of each technique and select the one that best suits the specific forecasting task.
What is the role of data preprocessing in model building for forecasting?
Data preprocessing plays a crucial role in model building for forecasting. It involves cleaning, transforming, and organizing the data to enhance its quality and suitability for analysis. Preprocessing steps may include removing outliers, handling missing values, normalizing or scaling variables, and feature engineering. Proper data preprocessing ensures accurate and reliable forecasting results.
Can model building for forecasting handle non-linear relationships?
Yes, model building techniques for forecasting can handle non-linear relationships. Advanced techniques such as neural networks and machine learning algorithms are designed to capture and model complex non-linear patterns within the data. These methods enable accurate forecasting even in situations where the relationships between variables are not linear.
What are the challenges in model building for forecasting?
There are several challenges in model building for forecasting. Some common challenges include selecting the appropriate variables, dealing with missing or incomplete data, choosing the right model complexity, overfitting the model to the training data, and handling unforeseen outliers or anomalies in the future data. It requires careful analysis, validation, and continuous improvement to overcome these challenges and build robust forecasting models.
How can model building for forecasting be evaluated?
Model building for forecasting can be evaluated using various metrics and techniques. Common evaluation measures include mean absolute error (MAE), root mean square error (RMSE), mean absolute percentage error (MAPE), and forecast accuracy. Additionally, techniques like cross-validation, holdout validation, or time series splitting can be used to assess the model’s performance and generalize its effectiveness.
Is it necessary to update the forecasting model regularly?
Yes, it is necessary to update the forecasting model regularly. Forecasting models should be periodically reviewed and updated to account for changing trends, new data, and evolving business conditions. Regular updates help ensure that the model remains accurate and reliable over time and enables organizations to make well-informed decisions based on the most up-to-date information.
What are some best practices for effective model building in forecasting?
Some best practices for effective model building in forecasting include: 1) Clearly defining the forecasting objectives and requirements, 2) Selecting the appropriate data and variables, 3) Performing thorough data exploration and preprocessing, 4) Choosing and implementing suitable model building techniques, 5) Evaluating and validating the models using appropriate metrics, 6) Regularly updating and refining the models, and 7) Documenting and sharing the methodology, assumptions, and limitations of the forecasting models.