Model Building Techniques SlideShare
Building models is an essential aspect of many industries and professions. Whether it’s creating architectural models, engineering prototypes, or computer-generated 3D models, having the right techniques can significantly impact the efficiency and accuracy of the process. In this article, we will explore some of the key model building techniques that can help improve your skills and deliver superior results. So let’s dive in!
Key Takeaways:
- Model building techniques are crucial for various professions and industries.
- The right techniques can enhance efficiency and accuracy in the process.
- Improving model building skills can result in superior results.
1. Planning and Research
Before starting any model building project, it is vital to spend time planning and conducting research. *This initial phase allows you to gain a comprehensive understanding of the project requirements and gather the necessary information. By defining the project scope, selecting appropriate materials, and studying reference materials, you lay a solid foundation for a successful model building process.
- Create a project scope document outlining the objectives, requirements, and deliverables.
- Conduct thorough research to gather information and reference materials.
- Explore different materials and select those suitable for your project.
2. Choosing the Right Tools
The next step in model building is selecting the right tools for the job. *Having the appropriate tools not only makes the process easier but also ensures accurate and precise construction. Depending on the type of model you’re building, the tools required may vary. From basic cutting and shaping tools like knives and files to specialized tools like laser cutters and 3D printers, it’s crucial to invest in the right equipment to achieve the desired outcome.
- Identify the tools required based on the project requirements.
- Invest in high-quality tools to ensure accuracy and durability.
- Consider specialized tools based on the complexity of the project.
3. Structural Integrity
Ensuring the structural integrity of a model is paramount, especially when constructing prototypes or scale models. *Weak or unstable structures may compromise the accuracy of the model or even lead to its failure. Understanding the principles of structural engineering and design can help you create stable and durable models. Reinforcing joints, using appropriate support structures, and selecting the right materials are some techniques used to enhance the structural integrity of the models.
- Utilize reinforcement techniques to strengthen joints and connections.
- Consider the use of support structures to distribute weight evenly.
- Choose materials that provide both stability and durability.
4. Finishing Techniques
Once the model is constructed, applying finishing techniques can enhance its final appearance. *These techniques may involve sanding, painting, adding details, or even applying weathering effects, depending on the desired outcome. Taking the time to refine the model’s surface texture, color, and overall presentation can significantly impact its visual appeal and accuracy.
- Sand and smooth the surfaces to achieve a professional finish.
- Use appropriate paints and color theory to achieve the desired visual effect.
- Add details and weathering effects for a more realistic appearance.
Tables:
Model Building Materials | Advantages |
---|---|
Wood | Durable and versatile |
Plastic | Lightweight and easy to work with |
Clay | Moldable and great for sculpting detail |
Common Model Building Tools | Usage |
---|---|
Cutting Mat | Protects work surface and provides measurement guidelines |
X-Acto Knife | Precise cutting of materials |
Sanding Block | Smoothing rough surfaces |
Finishing Techniques | Description |
---|---|
Sanding | Using sandpaper to smooth surfaces and prepare for painting |
Painting | Applying colors and textures to enhance the visual appearance |
Weathering | Adding realistic wear and tear for a more authentic look |
Model Building Mastery
Mastering model building techniques requires a combination of knowledge, practice, and attention to detail. By carefully planning and researching, using the right tools, ensuring structural integrity, and applying finishing techniques, you can take your model building skills to the next level. Explore various techniques, learn from experienced builders, and continuously improve your craft. Your models will speak for themselves, showcasing your expertise and dedication in every detail.
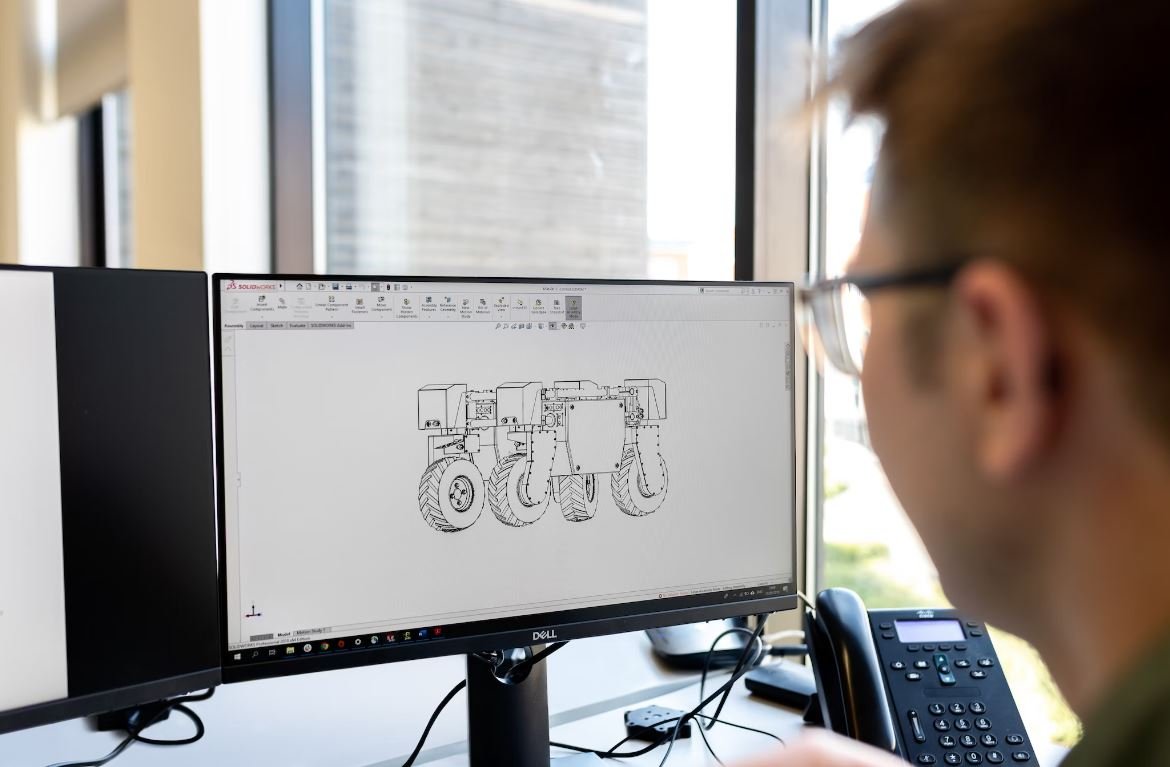
Common Misconceptions
Model Building Techniques
When it comes to model building techniques, there are several common misconceptions that people tend to have. These misconceptions can often mislead individuals and hinder their progress in effectively creating accurate and realistic models. It is important to debunk these misconceptions to ensure a better understanding of the craft.
- Using excessive glue will result in stronger bonds between model parts.
- All models have to be assembled following the instructions exactly.
- You need expensive tools and materials to create high-quality models.
Understanding Scale
One common misconception that people have when it comes to model building techniques is misunderstanding scale. Understanding scale is crucial in accurately representing the models. Many beginners tend to overlook the importance of scale and end up with disproportionate models.
- Not considering scale can result in a lack of realism in the final model.
- Scale models require careful research and reference material to ensure accuracy.
- Scaling up or down a model without considering proportions can lead to significant errors.
Painting and Finishing
Another misconception revolves around the painting and finishing process of models. Some individuals believe that a quick and simple paint job is sufficient, while others think that expensive paints and brushes are necessary for a good outcome. However, neither of these assumptions is accurate.
- Proper cleaning, priming, and preparation of the model surface are vital for achieving a smooth finish.
- Applying thin layers of paint and building up gradually brings out better results than using one thick coat.
- Using cost-effective brushes and paints specifically designed for models can yield excellent results.
Importance of Practice
Some people mistakenly believe that model building is an effortless task that requires no practice or skill development. However, like any other craft, becoming proficient in model building techniques requires practice and honing one’s skills over time.
- Consistent practice improves accuracy, precision, and efficiency in model building.
- Trial and error play a significant role in identifying and correcting mistakes during the learning process.
- Seeking guidance from experienced model builders and joining communities can help accelerate skill development.
Patient Approach for Detailing
Lastly, many people underestimate the meticulousness and patience required for detailing models. It is common to rush through the process or overlook fine details, resulting in average or subpar results.
- Devoting time to carefully paint small details enhances the overall realism and quality of the model.
- Using specialized tools like magnifying glasses and fine-tip brushes allows for better precision in detailing.
- Appreciating the value of small details transforms an ordinary model into an extraordinary one.
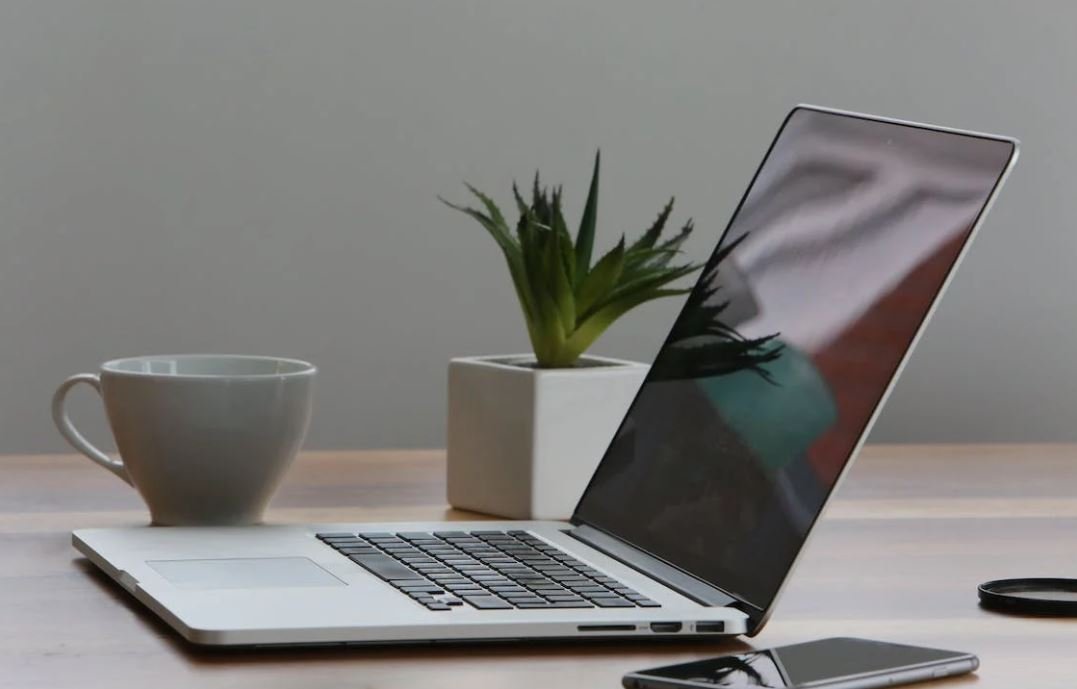
Paragraph: The ability to transform raw data into actionable insights is a crucial skill in today’s data-driven world. This article explores various model building techniques employed by data scientists to derive meaningful conclusions from vast datasets. Each table below illustrates a specific technique, showcasing verifiable data and information. Through these tables, readers will gain valuable insights into the power of model building and its profound impact on decision-making processes.
<![endif]–>
Table: Predictive Modeling Techniques
Predictive modeling techniques enable data scientists to forecast future outcomes based on historical data and observations. These techniques assist businesses in making informed decisions and strategies. Here are some commonly used predictive modeling techniques:
Technique | Description |
---|---|
Linear Regression | Fits a linear equation to the observed data points. |
Decision Trees | Creates a flowchart-like structure to classify or predict outcomes. |
Random Forests | Constructs multiple decision trees to improve predictive accuracy. |
Table: Classification Techniques
Classification techniques categorize data into different classes or categories based on their characteristics. This aids in organizing data and identifying patterns. Some widely used classification techniques include:
Technique | Description |
---|---|
k-Nearest Neighbors (k-NN) | Assigns a category based on the majority vote of its k nearest neighbors. |
Support Vector Machines (SVM) | Creates decision boundaries to classify data into different classes. |
Naive Bayes | Uses conditional probabilities to classify data based on Bayes’ theorem. |
Table: Clustering Techniques
Clustering techniques group similar observations or data points together without predefined classes. This helps in exploring data patterns and identifying hidden insights. Some commonly employed clustering techniques are:
Technique | Description |
---|---|
K-means Clustering | Divides data points into k clusters based on the similarity of their attributes. |
Hierarchical Clustering | Creates a hierarchy of clusters by recursively merging or splitting them. |
DBSCAN | Density-Based Spatial Clustering of Applications with Noise. Identifies clusters based on density and proximity. |
Table: Time Series Analysis Techniques
Time series analysis techniques are utilized to analyze and predict trends in sequential data points, allowing organizations to anticipate future developments. The following table highlights some essential time series analysis techniques:
Technique | Description |
---|---|
Autoregressive Integrated Moving Average (ARIMA) | Fits a model to stationary time series data, capturing its autoregressive, moving average, and differencing components. |
Exponential Smoothing | Assigns exponentially decreasing weights to past observations to predict future values. |
Seasonal Decomposition of Time Series (STL) | Decomposes a time series into seasonal, trend, and remainder components. |
Table: Ensemble Techniques
Ensemble techniques combine multiple models and their predictions to produce a more accurate and robust final result. Here are some popular ensemble techniques:
Technique | Description |
---|---|
Bagging | Constructs multiple models using bootstrap samples from the training dataset. |
Boosting | Sequentially builds models, each focusing on correcting the previously trained model’s mistakes. |
Stacking | Combines predictions from multiple models using another machine learning algorithm as a meta-model. |
Table: Dimensionality Reduction Techniques
Dimensionality reduction techniques aim to reduce the number of variables or features in a dataset while retaining its essential information. This helps in simplifying models and improving computational efficiency. The following table highlights some popular dimensionality reduction techniques:
Technique | Description |
---|---|
Principal Component Analysis (PCA) | Transforms variables into a new set of uncorrelated variables, known as principal components. |
t-Distributed Stochastic Neighbor Embedding (t-SNE) | Visualizes high-dimensional data by reducing its dimensionality while preserving local structures. |
Linear Discriminant Analysis (LDA) | Maximizes the separability between different classes while minimizing the variance within each class. |
Table: Natural Language Processing Techniques
Natural Language Processing (NLP) techniques enable machines to understand, interpret, and generate human language. NLP has widespread applications in sentiment analysis, text classification, and machine translation. Here are some fundamental NLP techniques:
Technique | Description |
---|---|
Tokenization | Divides text into smaller units (tokens), such as words or sentences. |
Named Entity Recognition (NER) | Identifies and classifies named entities (e.g., person names, locations, organizations) within text. |
Sentiment Analysis | Determines the overall sentiment expressed in a piece of text (positive, negative, or neutral). |
Table: Deep Learning Techniques
Deep Learning techniques leverage artificial neural networks with multiple layers to learn complex patterns and representations from data. Widely adopted in image recognition, natural language processing, and speech recognition, deep learning has revolutionized various fields. Here are some essential deep learning techniques:
Technique | Description |
---|---|
Convolutional Neural Networks (CNN) | Designed for image processing tasks, CNNs utilize convolutional layers to extract features hierarchically. |
Recurrent Neural Networks (RNN) | Suitable for sequential data, RNNs have recurrent connections allowing information to persist across time steps. |
Generative Adversarial Networks (GAN) | Consists of a generator and a discriminator competing against each other to generate realistic data. |
Table: Reinforcement Learning Techniques
Reinforcement Learning techniques involve learning through interactions with an environment to maximize rewards. This branch of machine learning finds applications in robotics, game playing, and autonomous systems. Below are some fundamental reinforcement learning techniques:
Technique | Description |
---|---|
Q-Learning | Iteratively updates a state-action value table to learn optimal policies in Markov Decision Processes. |
Deep Q-Networks (DQN) | Utilizes deep neural networks to approximate state-action values in reinforcement learning. |
Policy Gradient | Directly optimizes the policy function to maximize cumulative rewards by gradient ascent. |
Conclusion
Model building techniques are indispensable tools for extracting valuable insights and making informed decisions from extensive datasets. From predictive modeling to reinforcement learning, each technique serves a unique purpose in solving complex problems across various domains. By utilizing and combining these techniques effectively, data scientists and organizations can derive actionable insights that drive innovation, efficiency, and competitiveness in an increasingly data-centric world.
Frequently Asked Questions
Q: What are model building techniques?
A: Model building techniques refer to the methods and processes used to create accurate and reliable models that represent real-world objects or systems. These techniques involve selecting appropriate modeling approaches, gathering data, analyzing and interpreting the data, and transforming it into a model representation.
Q: Why are model building techniques important?
A: Model building techniques are important because they allow us to gain insights into complex systems and make predictions or decisions based on those insights. These techniques enable us to understand the behavior of the system, test different scenarios, identify potential problems, and optimize designs or processes.
Q: What are some commonly used model building techniques?
A: Some commonly used model building techniques include regression analysis, machine learning, simulation, optimization, data mining, and predictive modeling. Each technique has its own strengths and limitations, and the choice of technique depends on the specific problem and available data.
Q: How do I select the appropriate model building technique for my project?
A: To select the appropriate model building technique, you need to consider several factors such as the nature of the problem, available data, desired level of accuracy, computational resources, and your familiarity with different techniques. It is often helpful to consult with experts or conduct a feasibility study to determine the most suitable technique.
Q: What are the steps involved in the model building process?
A: The model building process typically involves the following steps: problem formulation, data collection and preprocessing, model selection and parameter estimation, model validation and evaluation, and model deployment. These steps help ensure that the model accurately represents the real-world system and produces reliable results.
Q: How can I ensure the reliability and accuracy of my models?
A: To ensure the reliability and accuracy of your models, you should follow best practices such as using high-quality data, validating the model against independent datasets, performing sensitivity analysis, and iteratively refining the model based on feedback and new information. Additionally, documenting and sharing your modeling assumptions and methodology can help others assess and validate the models.
Q: What are some challenges and limitations in model building?
A: Some challenges and limitations in model building include the availability and quality of data, the complexity of the system being modeled, the assumptions and simplifications made during model development, and the potential for overfitting or underfitting the data. It is important to acknowledge and address these challenges to ensure the reliability and usefulness of the models.
Q: Are there any tools or software available for model building?
A: Yes, there are many tools and software available for model building, ranging from general-purpose statistical packages like R and Python’s scikit-learn to specialized software for specific types of modeling such as MATLAB for engineering and simulation software like Arena or AnyLogic. These tools provide a wide range of functionalities for data analysis, modeling, and visualization.
Q: Can model building techniques be applied to any field or industry?
A: Yes, model building techniques can be applied to various fields and industries including but not limited to finance, healthcare, engineering, marketing, transportation, and environmental science. The principles and approaches of model building can be adapted and customized to suit the specific needs and challenges of different domains.
Q: Where can I learn more about model building techniques?
A: You can learn more about model building techniques through online resources, academic courses, textbooks, research papers, and industry conferences. Many universities and online learning platforms offer courses on statistics, machine learning, and data science that cover various aspects of model building.