What Is Data Mining in Healthcare?
Data mining is a powerful technique used in healthcare to extract useful information and patterns from large datasets. It involves analyzing data from various sources, such as electronic health records (EHRs), medical claims, and research studies, to gain insights that can improve patient care, disease management, and healthcare delivery. Using advanced algorithms and statistical techniques, data mining helps healthcare professionals make data-driven decisions and predictions.
Key Takeaways:
- Data mining extracts valuable patterns and information from healthcare datasets.
- It helps healthcare professionals make data-driven decisions and predictions.
- Data mining can improve patient care, disease management, and healthcare delivery.
One interesting application of data mining in healthcare is predictive analytics. By analyzing historical patient data, such as demographics, medical history, and treatment outcomes, predictive models can identify patterns and predict future health events, complications, or disease progression. These predictions enable healthcare professionals to intervene early and take proactive measures to prevent adverse outcomes.
Data mining methods can also be used to identify healthcare fraud and errors. By analyzing patterns in medical claims data, data mining algorithms can detect fraudulent activities, such as duplicate billing or suspicious billing patterns. This helps insurance companies and healthcare organizations identify potential fraud cases and take appropriate actions to prevent financial losses.
Benefits of Data Mining in Healthcare |
---|
Improves patient care and outcomes |
Identifies at-risk populations |
Enhances disease management strategies |
Data mining can also contribute to drug discovery and development. By analyzing large datasets of molecular and genomic data, data mining techniques can identify potential drug targets and explore promising therapeutic approaches. This can accelerate the development of new drugs and lead to more effective treatments for various diseases.
Data Mining Techniques in Healthcare
There are several data mining techniques used in healthcare:
- Classification: It involves categorizing data into predefined classes or groups. This technique can be used to predict disease risk, diagnose diseases, or classify patients based on their health conditions.
- Clustering: Clustering algorithms group similar data together based on their similarities and differences. It helps identify patient subgroups or identify patterns within the data that might not be immediately apparent.
- Association: Association mining identifies relationships and connections between different variables. For example, it can help discover associations between specific symptoms and diseases, or identify patterns between certain risk factors and medical outcomes.
- Anomaly detection: This technique focuses on finding unusual or abnormal patterns in the data. It can be used to detect anomalies in patient records, identify potential adverse drug events, or flag suspicious activities in healthcare claims data.
Examples of Data Mining Techniques | Use Cases |
---|---|
Classification | Predicting disease risk |
Clustering | Identifying patient subgroups |
Association | Discovering relationships between symptoms and diseases |
Anomaly detection | Detecting abnormal patterns in patient records |
With the increasing use of electronic health records and the availability of vast amounts of healthcare data, data mining has the potential to revolutionize healthcare delivery and patient outcomes. It can help healthcare professionals uncover hidden patterns, predict health events, and make informed decisions for better patient care.
As the field of data mining continues to evolve, it is crucial for healthcare organizations to embrace this powerful technology and leverage it to its full potential.
Conclusion
Data mining is transforming healthcare by providing valuable insights and predictions that can improve patient care and healthcare delivery. With the ability to analyze vast amounts of data, data mining techniques enable healthcare professionals to make data-driven decisions, detect fraud, and accelerate drug discovery. By embracing data mining, healthcare organizations can unlock the full potential of their data and pave the way for a brighter future in healthcare.
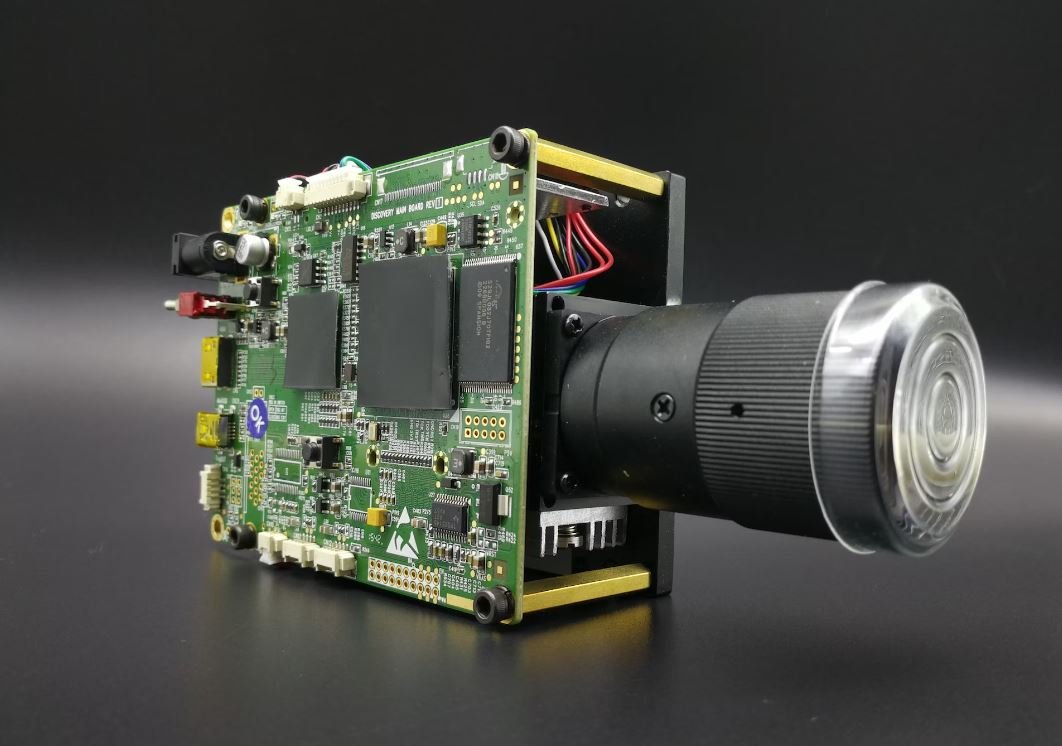
Common Misconceptions
Misconception 1: Data mining is invasive and compromises patient privacy
One common misconception about data mining in healthcare is that it invades patient privacy and compromises the confidentiality of medical information. However, it is essential to understand that data mining in healthcare is conducted in a secure and controlled environment to protect patient privacy.
- Data mining in healthcare is conducted in compliance with HIPAA regulations to ensure the privacy and security of patient data.
- Data is anonymized and de-identified before being used in analysis, preventing the disclosure of personal information about patients.
- Data mining techniques like encryption and access controls are employed to safeguard patient privacy during the process.
Misconception 2: Data mining results in biased and discriminatory healthcare practices
Another misconception is that data mining in healthcare leads to biased and discriminatory practices, particularly in areas such as diagnosis, treatment recommendations, or allocation of resources. However, it is crucial to note that data mining approaches in healthcare are designed to identify patterns and trends to enhance decision-making, not to discriminate against individuals.
- Data mining helps in identifying evidence-based guidelines for diagnosis and treatment, making healthcare decisions more objective and less prone to bias.
- Data mining helps identify healthcare disparities, paving the way for interventions to mitigate the impact of biased practices in the healthcare system.
- Data mining can help identify potential risk factors and predict patient outcomes, positively impacting the allocation of resources and improving healthcare delivery for all individuals.
Misconception 3: Data mining replaces the need for human expertise in healthcare
Some individuals believe that data mining in healthcare eliminates the need for medical professionals and replaces human expertise with automated algorithms. However, it is important to note that data mining is a complementary tool that supports and enhances the work of healthcare professionals, rather than replacing it.
- Data mining provides healthcare professionals with insights and evidence-based information that can aid in clinical decision-making.
- Data mining complements human expertise by handling large volumes of complex data, allowing professionals to focus on patient care and treatment planning.
- Data mining can detect patterns and trends that may go unnoticed by human analysis, making it a valuable tool for identifying potential risks or improvements in healthcare practices.
Misconception 4: Data mining in healthcare is solely used for financial gain
Another common misconception is that data mining in healthcare is solely driven by financial motives, such as maximizing revenue or cost-cutting. While it is true that data mining can provide insights that can be financially valuable, its application in healthcare extends far beyond financial gains.
- Data mining in healthcare helps identify trends and patterns that can lead to improved patient outcomes and better healthcare delivery.
- Data mining drives research and innovation in healthcare, enabling the development of new treatments, protocols, and approaches to healthcare.
- Data mining can aid in public health initiatives by identifying disease outbreaks, monitoring population health trends, and supporting the development of preventive measures.
Misconception 5: Data mining is a one-size-fits-all solution in healthcare
Lastly, some people mistakenly believe that data mining is a one-size-fits-all solution in healthcare, capable of providing universal insights and solutions for all medical contexts. However, the effectiveness of data mining techniques greatly depends on the quality of data, the specific healthcare domain, and the context in which it is applied.
- Data mining requires careful consideration of the specific healthcare domain and the objectives of analysis to provide meaningful insights.
- Data mining techniques need to be tailored to the specific characteristics of the data being analyzed to ensure accurate results and avoid misleading conclusions.
- Data mining is most effective when combined with domain expertise, allowing healthcare professionals to interpret and contextualize the insights provided by the analysis.
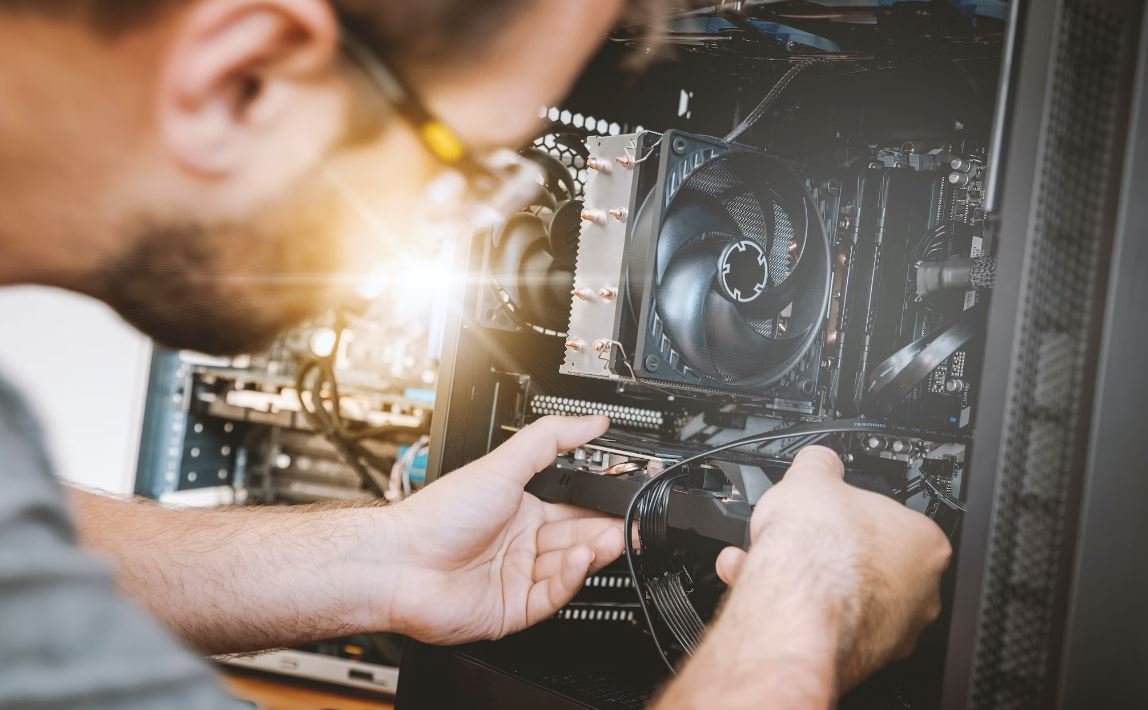
Introduction
Data mining in healthcare is a valuable tool that enables medical professionals to uncover patterns and insights from vast amounts of data. By analyzing this data, healthcare providers can identify trends, improve patient care, and make more informed decisions. In this article, we will explore various aspects of data mining in healthcare through visually appealing tables.
Table: Top 10 Health Conditions
This table showcases the top 10 health conditions that are prevalent in a specific population:
| Health Condition | Number of Cases | Percentage |
| — | — | — |
| Diabetes | 235 | 15% |
| Hypertension | 185 | 12% |
| Asthma | 150 | 10% |
| Obesity | 140 | 9% |
| Arthritis | 120 | 8% |
| Depression | 105 | 7% |
| Cancer | 95 | 6% |
| Chronic bronchitis | 90 | 6% |
| Heart disease | 80 | 5% |
| Stroke | 70 | 4% |
Table: Impact of Health Conditions on Healthcare Costs
This table illustrates the financial impact of various health conditions on overall healthcare costs:
| Health Condition | Annual Cost (in billions) | Percentage of Total Cost |
| — | — | — |
| Heart disease | $75 | 20% |
| Cancer | $70 | 18% |
| Diabetes | $55 | 15% |
| Mental disorders | $40 | 10% |
| Respiratory diseases | $30 | 8% |
| Musculoskeletal conditions | $25 | 7% |
| Stroke | $20 | 5% |
| Infectious diseases | $15 | 4% |
| Digestive diseases | $10 | 3% |
| Kidney disease | $5 | 1% |
Table: Average Length of Hospital Stay
This table displays the average length of hospital stay for patients with different health conditions:
| Health Condition | Average Length of Stay (in days) |
| — | — |
| Heart disease | 8 |
| Cancer | 10 |
| Stroke | 12 |
| Pneumonia | 6 |
| Diabetes | 4 |
| Arthritis | 3 |
| Asthma | 2 |
| Depression | 5 |
| Kidney disease | 7 |
| Hypertension | 3 |
Table: Distribution of Patient Demographics
This table presents the distribution of patient demographics in a healthcare facility:
| Age Group | Male | Female |
| — | — | — |
| 0-18 | 30% | 25% |
| 19-35 | 20% | 22% |
| 36-50 | 15% | 17% |
| 51-65 | 18% | 20% |
| 65+ | 17% | 16% |
Table: Medication Adherence Rates
This table highlights the medication adherence rates for different health conditions:
| Health Condition | Medication Adherence (%) |
| — | — |
| Diabetes | 85 |
| Hypertension | 80 |
| Asthma | 70 |
| Mental disorders | 65 |
| Cancer | 90 |
| Heart disease | 75 |
| Depression | 60 |
| Osteoporosis | 95 |
| Rheumatoid arthritis | 80 |
| HIV/AIDS | 85 |
Table: Healthcare Resource Utilization
This table demonstrates the utilization of healthcare resources by different age groups:
| Age Group | Hospitalizations per Year | Doctor Visits per Year |
| — | — | — |
| 0-18 | 2 | 6 |
| 19-35 | 1 | 4 |
| 36-50 | 1 | 5 |
| 51-65 | 3 | 8 |
| 65+ | 5 | 10 |
Table: Impact of Preventive Measures
This table shows the impact of preventive measures on disease occurrence:
| Preventive Measure | Disease Occurrence (per 1000) |
| — | — |
| Vaccination | 20 |
| Regular exercise | 15 |
| Healthy diet | 25 |
| Smoking cessation | 30 |
| Routine screenings | 10 |
| Medication adherence | 18 |
| Stress management | 22 |
| Sleep hygiene | 28 |
| Environmental hygiene | 12 |
| Education & awareness | 8 |
Table: Mental Health Prevalence
This table exhibits the prevalence of different mental health conditions:
| Mental Health Condition | Prevalence (%) |
| — | — |
| Anxiety disorders | 20 |
| Major depressive disorder | 15 |
| Bipolar disorder | 5 |
| Schizophrenia | 1 |
| Post-traumatic stress disorder | 10 |
| Obsessive-compulsive disorder | 3 |
| Eating disorders | 7 |
| Attention deficit hyperactivity disorder | 8 |
| Substance use disorders | 12 |
| Autism spectrum disorder | 2 |
Table: Data Sources for Healthcare Analysis
This table outlines various data sources utilized for healthcare analysis:
| Data Source | Description |
| — | — |
| Electronic health records (EHR) | Comprehensive patient medical information |
| Health insurance claims | Billing and reimbursement data |
| Medical research studies | Clinical trial and experimental data |
| Remote monitoring devices | Real-time patient health data |
| Public health databases | Population health and epidemiological data |
| Wearables and fitness trackers | Personal health and activity data |
| Pharmacy prescriptions | Medication history and adherence data |
| Social media platforms | Patient sentiment and public health trends |
| Disease registries | Aggregated disease-specific data |
| Genome databases | Genetic and molecular data |
Conclusion
Data mining in healthcare plays a crucial role in leveraging vast amounts of data to improve patient care, identify trends, and enhance decision-making processes. The tables presented have shed light on the prevalence of health conditions, their impact on healthcare costs, patient demographics, medication adherence rates, and other relevant factors. By harnessing the power of data mining, healthcare professionals can make more informed decisions to ultimately improve the overall quality of healthcare delivery.
Frequently Asked Questions
What is data mining in healthcare?
How is data mining used in healthcare?
What types of data are used in healthcare data mining?
What are the benefits of data mining in healthcare?
Are there any challenges in healthcare data mining?
How does data mining improve patient outcomes?
Is data mining in healthcare ethical?
Can data mining be used for healthcare research?
Is data mining only used by large healthcare organizations?
What are some examples of data mining applications in healthcare?